Long Short-Term Memory networks, or LSTMs for short, can be applied to time series forecasting.
There are many types of LSTM models that can be used for each specific type of time series forecasting problem.
In this tutorial, you will discover how to develop a suite of LSTM models for a range of standard time series forecasting problems.
The objective of this tutorial is to provide standalone examples of each model on each type of time series problem as a template that you can copy and adapt for your specific time series forecasting problem.
After completing this tutorial, you will know:
- How to develop LSTM models for univariate time series forecasting.
- How to develop LSTM models for multivariate time series forecasting.
- How to develop LSTM models for multi-step time series forecasting.
This is a large and important post; you may want to bookmark it for future reference.
Kick-start your project with my new book Deep Learning for Time Series Forecasting, including step-by-step tutorials and the Python source code files for all examples.
Let’s get started.
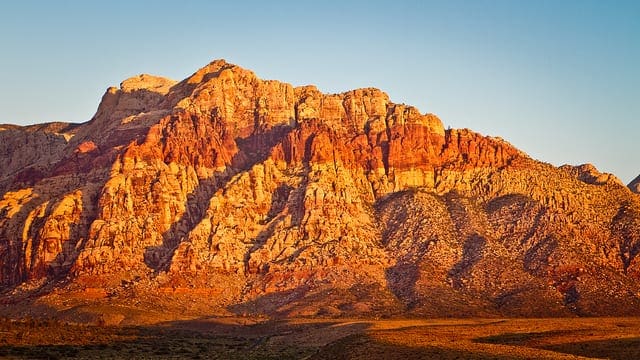
How to Develop LSTM Models for Time Series Forecasting
Photo by N i c o l a, some rights reserved.
Tutorial Overview
In this tutorial, we will explore how to develop a suite of different types of LSTM models for time series forecasting.
The models are demonstrated on small contrived time series problems intended to give the flavor of the type of time series problem being addressed. The chosen configuration of the models is arbitrary and not optimized for each problem; that was not the goal.
This tutorial is divided into four parts; they are:
- Univariate LSTM Models
- Data Preparation
- Vanilla LSTM
- Stacked LSTM
- Bidirectional LSTM
- CNN LSTM
- ConvLSTM
- Multivariate LSTM Models
- Multiple Input Series.
- Multiple Parallel Series.
- Multi-Step LSTM Models
- Data Preparation
- Vector Output Model
- Encoder-Decoder Model
- Multivariate Multi-Step LSTM Models
- Multiple Input Multi-Step Output.
- Multiple Parallel Input and Multi-Step Output.
Univariate LSTM Models
LSTMs can be used to model univariate time series forecasting problems.
These are problems comprised of a single series of observations and a model is required to learn from the series of past observations to predict the next value in the sequence.
We will demonstrate a number of variations of the LSTM model for univariate time series forecasting.
This section is divided into six parts; they are:
- Data Preparation
- Vanilla LSTM
- Stacked LSTM
- Bidirectional LSTM
- CNN LSTM
- ConvLSTM
Each of these models are demonstrated for one-step univariate time series forecasting, but can easily be adapted and used as the input part of a model for other types of time series forecasting problems.
Data Preparation
Before a univariate series can be modeled, it must be prepared.
The LSTM model will learn a function that maps a sequence of past observations as input to an output observation. As such, the sequence of observations must be transformed into multiple examples from which the LSTM can learn.
Consider a given univariate sequence:
1 |
[10, 20, 30, 40, 50, 60, 70, 80, 90] |
We can divide the sequence into multiple input/output patterns called samples, where three time steps are used as input and one time step is used as output for the one-step prediction that is being learned.
1 2 3 4 5 |
X, y 10, 20, 30 40 20, 30, 40 50 30, 40, 50 60 ... |
The split_sequence() function below implements this behavior and will split a given univariate sequence into multiple samples where each sample has a specified number of time steps and the output is a single time step.
1 2 3 4 5 6 7 8 9 10 11 12 13 14 |
# split a univariate sequence into samples def split_sequence(sequence, n_steps): X, y = list(), list() for i in range(len(sequence)): # find the end of this pattern end_ix = i + n_steps # check if we are beyond the sequence if end_ix > len(sequence)-1: break # gather input and output parts of the pattern seq_x, seq_y = sequence[i:end_ix], sequence[end_ix] X.append(seq_x) y.append(seq_y) return array(X), array(y) |
We can demonstrate this function on our small contrived dataset above.
The complete example is listed below.
1 2 3 4 5 6 7 8 9 10 11 12 13 14 15 16 17 18 19 20 21 22 23 24 25 26 27 |
# univariate data preparation from numpy import array # split a univariate sequence into samples def split_sequence(sequence, n_steps): X, y = list(), list() for i in range(len(sequence)): # find the end of this pattern end_ix = i + n_steps # check if we are beyond the sequence if end_ix > len(sequence)-1: break # gather input and output parts of the pattern seq_x, seq_y = sequence[i:end_ix], sequence[end_ix] X.append(seq_x) y.append(seq_y) return array(X), array(y) # define input sequence raw_seq = [10, 20, 30, 40, 50, 60, 70, 80, 90] # choose a number of time steps n_steps = 3 # split into samples X, y = split_sequence(raw_seq, n_steps) # summarize the data for i in range(len(X)): print(X[i], y[i]) |
Running the example splits the univariate series into six samples where each sample has three input time steps and one output time step.
1 2 3 4 5 6 |
[10 20 30] 40 [20 30 40] 50 [30 40 50] 60 [40 50 60] 70 [50 60 70] 80 [60 70 80] 90 |
Now that we know how to prepare a univariate series for modeling, let’s look at developing LSTM models that can learn the mapping of inputs to outputs, starting with a Vanilla LSTM.
Need help with Deep Learning for Time Series?
Take my free 7-day email crash course now (with sample code).
Click to sign-up and also get a free PDF Ebook version of the course.
Vanilla LSTM
A Vanilla LSTM is an LSTM model that has a single hidden layer of LSTM units, and an output layer used to make a prediction.
We can define a Vanilla LSTM for univariate time series forecasting as follows.
1 2 3 4 5 6 |
... # define model model = Sequential() model.add(LSTM(50, activation='relu', input_shape=(n_steps, n_features))) model.add(Dense(1)) model.compile(optimizer='adam', loss='mse') |
Key in the definition is the shape of the input; that is what the model expects as input for each sample in terms of the number of time steps and the number of features.
We are working with a univariate series, so the number of features is one, for one variable.
The number of time steps as input is the number we chose when preparing our dataset as an argument to the split_sequence() function.
The shape of the input for each sample is specified in the input_shape argument on the definition of first hidden layer.
We almost always have multiple samples, therefore, the model will expect the input component of training data to have the dimensions or shape:
1 |
[samples, timesteps, features] |
Our split_sequence() function in the previous section outputs the X with the shape [samples, timesteps], so we easily reshape it to have an additional dimension for the one feature.
1 2 3 4 |
... # reshape from [samples, timesteps] into [samples, timesteps, features] n_features = 1 X = X.reshape((X.shape[0], X.shape[1], n_features)) |
In this case, we define a model with 50 LSTM units in the hidden layer and an output layer that predicts a single numerical value.
The model is fit using the efficient Adam version of stochastic gradient descent and optimized using the mean squared error, or ‘mse‘ loss function.
Once the model is defined, we can fit it on the training dataset.
1 2 3 |
... # fit model model.fit(X, y, epochs=200, verbose=0) |
After the model is fit, we can use it to make a prediction.
We can predict the next value in the sequence by providing the input:
1 |
[70, 80, 90] |
And expecting the model to predict something like:
1 |
[100] |
The model expects the input shape to be three-dimensional with [samples, timesteps, features], therefore, we must reshape the single input sample before making the prediction.
1 2 3 4 5 |
... # demonstrate prediction x_input = array([70, 80, 90]) x_input = x_input.reshape((1, n_steps, n_features)) yhat = model.predict(x_input, verbose=0) |
We can tie all of this together and demonstrate how to develop a Vanilla LSTM for univariate time series forecasting and make a single prediction.
1 2 3 4 5 6 7 8 9 10 11 12 13 14 15 16 17 18 19 20 21 22 23 24 25 26 27 28 29 30 31 32 33 34 35 36 37 38 39 40 41 42 |
# univariate lstm example from numpy import array from keras.models import Sequential from keras.layers import LSTM from keras.layers import Dense # split a univariate sequence into samples def split_sequence(sequence, n_steps): X, y = list(), list() for i in range(len(sequence)): # find the end of this pattern end_ix = i + n_steps # check if we are beyond the sequence if end_ix > len(sequence)-1: break # gather input and output parts of the pattern seq_x, seq_y = sequence[i:end_ix], sequence[end_ix] X.append(seq_x) y.append(seq_y) return array(X), array(y) # define input sequence raw_seq = [10, 20, 30, 40, 50, 60, 70, 80, 90] # choose a number of time steps n_steps = 3 # split into samples X, y = split_sequence(raw_seq, n_steps) # reshape from [samples, timesteps] into [samples, timesteps, features] n_features = 1 X = X.reshape((X.shape[0], X.shape[1], n_features)) # define model model = Sequential() model.add(LSTM(50, activation='relu', input_shape=(n_steps, n_features))) model.add(Dense(1)) model.compile(optimizer='adam', loss='mse') # fit model model.fit(X, y, epochs=200, verbose=0) # demonstrate prediction x_input = array([70, 80, 90]) x_input = x_input.reshape((1, n_steps, n_features)) yhat = model.predict(x_input, verbose=0) print(yhat) |
Running the example prepares the data, fits the model, and makes a prediction.
Note: Your results may vary given the stochastic nature of the algorithm or evaluation procedure, or differences in numerical precision. Consider running the example a few times and compare the average outcome.
We can see that the model predicts the next value in the sequence.
1 |
[[102.09213]] |
Stacked LSTM
Multiple hidden LSTM layers can be stacked one on top of another in what is referred to as a Stacked LSTM model.
An LSTM layer requires a three-dimensional input and LSTMs by default will produce a two-dimensional output as an interpretation from the end of the sequence.
We can address this by having the LSTM output a value for each time step in the input data by setting the return_sequences=True argument on the layer. This allows us to have 3D output from hidden LSTM layer as input to the next.
We can therefore define a Stacked LSTM as follows.
1 2 3 4 5 6 7 |
... # define model model = Sequential() model.add(LSTM(50, activation='relu', return_sequences=True, input_shape=(n_steps, n_features))) model.add(LSTM(50, activation='relu')) model.add(Dense(1)) model.compile(optimizer='adam', loss='mse') |
We can tie this together; the complete code example is listed below.
1 2 3 4 5 6 7 8 9 10 11 12 13 14 15 16 17 18 19 20 21 22 23 24 25 26 27 28 29 30 31 32 33 34 35 36 37 38 39 40 41 42 43 |
# univariate stacked lstm example from numpy import array from keras.models import Sequential from keras.layers import LSTM from keras.layers import Dense # split a univariate sequence def split_sequence(sequence, n_steps): X, y = list(), list() for i in range(len(sequence)): # find the end of this pattern end_ix = i + n_steps # check if we are beyond the sequence if end_ix > len(sequence)-1: break # gather input and output parts of the pattern seq_x, seq_y = sequence[i:end_ix], sequence[end_ix] X.append(seq_x) y.append(seq_y) return array(X), array(y) # define input sequence raw_seq = [10, 20, 30, 40, 50, 60, 70, 80, 90] # choose a number of time steps n_steps = 3 # split into samples X, y = split_sequence(raw_seq, n_steps) # reshape from [samples, timesteps] into [samples, timesteps, features] n_features = 1 X = X.reshape((X.shape[0], X.shape[1], n_features)) # define model model = Sequential() model.add(LSTM(50, activation='relu', return_sequences=True, input_shape=(n_steps, n_features))) model.add(LSTM(50, activation='relu')) model.add(Dense(1)) model.compile(optimizer='adam', loss='mse') # fit model model.fit(X, y, epochs=200, verbose=0) # demonstrate prediction x_input = array([70, 80, 90]) x_input = x_input.reshape((1, n_steps, n_features)) yhat = model.predict(x_input, verbose=0) print(yhat) |
Note: Your results may vary given the stochastic nature of the algorithm or evaluation procedure, or differences in numerical precision. Consider running the example a few times and compare the average outcome.
Running the example predicts the next value in the sequence, which we expect would be 100.
1 |
[[102.47341]] |
Bidirectional LSTM
On some sequence prediction problems, it can be beneficial to allow the LSTM model to learn the input sequence both forward and backwards and concatenate both interpretations.
This is called a Bidirectional LSTM.
We can implement a Bidirectional LSTM for univariate time series forecasting by wrapping the first hidden layer in a wrapper layer called Bidirectional.
An example of defining a Bidirectional LSTM to read input both forward and backward is as follows.
1 2 3 4 5 6 |
... # define model model = Sequential() model.add(Bidirectional(LSTM(50, activation='relu'), input_shape=(n_steps, n_features))) model.add(Dense(1)) model.compile(optimizer='adam', loss='mse') |
The complete example of the Bidirectional LSTM for univariate time series forecasting is listed below.
1 2 3 4 5 6 7 8 9 10 11 12 13 14 15 16 17 18 19 20 21 22 23 24 25 26 27 28 29 30 31 32 33 34 35 36 37 38 39 40 41 42 43 |
# univariate bidirectional lstm example from numpy import array from keras.models import Sequential from keras.layers import LSTM from keras.layers import Dense from keras.layers import Bidirectional # split a univariate sequence def split_sequence(sequence, n_steps): X, y = list(), list() for i in range(len(sequence)): # find the end of this pattern end_ix = i + n_steps # check if we are beyond the sequence if end_ix > len(sequence)-1: break # gather input and output parts of the pattern seq_x, seq_y = sequence[i:end_ix], sequence[end_ix] X.append(seq_x) y.append(seq_y) return array(X), array(y) # define input sequence raw_seq = [10, 20, 30, 40, 50, 60, 70, 80, 90] # choose a number of time steps n_steps = 3 # split into samples X, y = split_sequence(raw_seq, n_steps) # reshape from [samples, timesteps] into [samples, timesteps, features] n_features = 1 X = X.reshape((X.shape[0], X.shape[1], n_features)) # define model model = Sequential() model.add(Bidirectional(LSTM(50, activation='relu'), input_shape=(n_steps, n_features))) model.add(Dense(1)) model.compile(optimizer='adam', loss='mse') # fit model model.fit(X, y, epochs=200, verbose=0) # demonstrate prediction x_input = array([70, 80, 90]) x_input = x_input.reshape((1, n_steps, n_features)) yhat = model.predict(x_input, verbose=0) print(yhat) |
Note: Your results may vary given the stochastic nature of the algorithm or evaluation procedure, or differences in numerical precision. Consider running the example a few times and compare the average outcome.
Running the example predicts the next value in the sequence, which we expect would be 100.
1 |
[[101.48093]] |
CNN LSTM
A convolutional neural network, or CNN for short, is a type of neural network developed for working with two-dimensional image data.
The CNN can be very effective at automatically extracting and learning features from one-dimensional sequence data such as univariate time series data.
A CNN model can be used in a hybrid model with an LSTM backend where the CNN is used to interpret subsequences of input that together are provided as a sequence to an LSTM model to interpret. This hybrid model is called a CNN-LSTM.
The first step is to split the input sequences into subsequences that can be processed by the CNN model. For example, we can first split our univariate time series data into input/output samples with four steps as input and one as output. Each sample can then be split into two sub-samples, each with two time steps. The CNN can interpret each subsequence of two time steps and provide a time series of interpretations of the subsequences to the LSTM model to process as input.
We can parameterize this and define the number of subsequences as n_seq and the number of time steps per subsequence as n_steps. The input data can then be reshaped to have the required structure:
1 |
[samples, subsequences, timesteps, features] |
For example:
1 2 3 4 5 6 7 8 9 10 |
... # choose a number of time steps n_steps = 4 # split into samples X, y = split_sequence(raw_seq, n_steps) # reshape from [samples, timesteps] into [samples, subsequences, timesteps, features] n_features = 1 n_seq = 2 n_steps = 2 X = X.reshape((X.shape[0], n_seq, n_steps, n_features)) |
We want to reuse the same CNN model when reading in each sub-sequence of data separately.
This can be achieved by wrapping the entire CNN model in a TimeDistributed wrapper that will apply the entire model once per input, in this case, once per input subsequence.
The CNN model first has a convolutional layer for reading across the subsequence that requires a number of filters and a kernel size to be specified. The number of filters is the number of reads or interpretations of the input sequence. The kernel size is the number of time steps included of each ‘read’ operation of the input sequence.
The convolution layer is followed by a max pooling layer that distills the filter maps down to 1/2 of their size that includes the most salient features. These structures are then flattened down to a single one-dimensional vector to be used as a single input time step to the LSTM layer.
1 2 3 4 |
... model.add(TimeDistributed(Conv1D(filters=64, kernel_size=1, activation='relu'), input_shape=(None, n_steps, n_features))) model.add(TimeDistributed(MaxPooling1D(pool_size=2))) model.add(TimeDistributed(Flatten())) |
Next, we can define the LSTM part of the model that interprets the CNN model’s read of the input sequence and makes a prediction.
1 2 3 |
... model.add(LSTM(50, activation='relu')) model.add(Dense(1)) |
We can tie all of this together; the complete example of a CNN-LSTM model for univariate time series forecasting is listed below.
1 2 3 4 5 6 7 8 9 10 11 12 13 14 15 16 17 18 19 20 21 22 23 24 25 26 27 28 29 30 31 32 33 34 35 36 37 38 39 40 41 42 43 44 45 46 47 48 49 50 51 |
# univariate cnn lstm example from numpy import array from keras.models import Sequential from keras.layers import LSTM from keras.layers import Dense from keras.layers import Flatten from keras.layers import TimeDistributed from keras.layers.convolutional import Conv1D from keras.layers.convolutional import MaxPooling1D # split a univariate sequence into samples def split_sequence(sequence, n_steps): X, y = list(), list() for i in range(len(sequence)): # find the end of this pattern end_ix = i + n_steps # check if we are beyond the sequence if end_ix > len(sequence)-1: break # gather input and output parts of the pattern seq_x, seq_y = sequence[i:end_ix], sequence[end_ix] X.append(seq_x) y.append(seq_y) return array(X), array(y) # define input sequence raw_seq = [10, 20, 30, 40, 50, 60, 70, 80, 90] # choose a number of time steps n_steps = 4 # split into samples X, y = split_sequence(raw_seq, n_steps) # reshape from [samples, timesteps] into [samples, subsequences, timesteps, features] n_features = 1 n_seq = 2 n_steps = 2 X = X.reshape((X.shape[0], n_seq, n_steps, n_features)) # define model model = Sequential() model.add(TimeDistributed(Conv1D(filters=64, kernel_size=1, activation='relu'), input_shape=(None, n_steps, n_features))) model.add(TimeDistributed(MaxPooling1D(pool_size=2))) model.add(TimeDistributed(Flatten())) model.add(LSTM(50, activation='relu')) model.add(Dense(1)) model.compile(optimizer='adam', loss='mse') # fit model model.fit(X, y, epochs=500, verbose=0) # demonstrate prediction x_input = array([60, 70, 80, 90]) x_input = x_input.reshape((1, n_seq, n_steps, n_features)) yhat = model.predict(x_input, verbose=0) print(yhat) |
Note: Your results may vary given the stochastic nature of the algorithm or evaluation procedure, or differences in numerical precision. Consider running the example a few times and compare the average outcome.
Running the example predicts the next value in the sequence, which we expect would be 100.
1 |
[[101.69263]] |
ConvLSTM
A type of LSTM related to the CNN-LSTM is the ConvLSTM, where the convolutional reading of input is built directly into each LSTM unit.
The ConvLSTM was developed for reading two-dimensional spatial-temporal data, but can be adapted for use with univariate time series forecasting.
The layer expects input as a sequence of two-dimensional images, therefore the shape of input data must be:
1 |
[samples, timesteps, rows, columns, features] |
For our purposes, we can split each sample into subsequences where timesteps will become the number of subsequences, or n_seq, and columns will be the number of time steps for each subsequence, or n_steps. The number of rows is fixed at 1 as we are working with one-dimensional data.
We can now reshape the prepared samples into the required structure.
1 2 3 4 5 6 7 8 9 10 |
... # choose a number of time steps n_steps = 4 # split into samples X, y = split_sequence(raw_seq, n_steps) # reshape from [samples, timesteps] into [samples, timesteps, rows, columns, features] n_features = 1 n_seq = 2 n_steps = 2 X = X.reshape((X.shape[0], n_seq, 1, n_steps, n_features)) |
We can define the ConvLSTM as a single layer in terms of the number of filters and a two-dimensional kernel size in terms of (rows, columns). As we are working with a one-dimensional series, the number of rows is always fixed to 1 in the kernel.
The output of the model must then be flattened before it can be interpreted and a prediction made.
1 2 3 |
... model.add(ConvLSTM2D(filters=64, kernel_size=(1,2), activation='relu', input_shape=(n_seq, 1, n_steps, n_features))) model.add(Flatten()) |
The complete example of a ConvLSTM for one-step univariate time series forecasting is listed below.
1 2 3 4 5 6 7 8 9 10 11 12 13 14 15 16 17 18 19 20 21 22 23 24 25 26 27 28 29 30 31 32 33 34 35 36 37 38 39 40 41 42 43 44 45 46 47 |
# univariate convlstm example from numpy import array from keras.models import Sequential from keras.layers import LSTM from keras.layers import Dense from keras.layers import Flatten from keras.layers import ConvLSTM2D # split a univariate sequence into samples def split_sequence(sequence, n_steps): X, y = list(), list() for i in range(len(sequence)): # find the end of this pattern end_ix = i + n_steps # check if we are beyond the sequence if end_ix > len(sequence)-1: break # gather input and output parts of the pattern seq_x, seq_y = sequence[i:end_ix], sequence[end_ix] X.append(seq_x) y.append(seq_y) return array(X), array(y) # define input sequence raw_seq = [10, 20, 30, 40, 50, 60, 70, 80, 90] # choose a number of time steps n_steps = 4 # split into samples X, y = split_sequence(raw_seq, n_steps) # reshape from [samples, timesteps] into [samples, timesteps, rows, columns, features] n_features = 1 n_seq = 2 n_steps = 2 X = X.reshape((X.shape[0], n_seq, 1, n_steps, n_features)) # define model model = Sequential() model.add(ConvLSTM2D(filters=64, kernel_size=(1,2), activation='relu', input_shape=(n_seq, 1, n_steps, n_features))) model.add(Flatten()) model.add(Dense(1)) model.compile(optimizer='adam', loss='mse') # fit model model.fit(X, y, epochs=500, verbose=0) # demonstrate prediction x_input = array([60, 70, 80, 90]) x_input = x_input.reshape((1, n_seq, 1, n_steps, n_features)) yhat = model.predict(x_input, verbose=0) print(yhat) |
Note: Your results may vary given the stochastic nature of the algorithm or evaluation procedure, or differences in numerical precision. Consider running the example a few times and compare the average outcome.
Running the example predicts the next value in the sequence, which we expect would be 100.
1 |
[[103.68166]] |
Now that we have looked at LSTM models for univariate data, let’s turn our attention to multivariate data.
Multivariate LSTM Models
Multivariate time series data means data where there is more than one observation for each time step.
There are two main models that we may require with multivariate time series data; they are:
- Multiple Input Series.
- Multiple Parallel Series.
Let’s take a look at each in turn.
Multiple Input Series
A problem may have two or more parallel input time series and an output time series that is dependent on the input time series.
The input time series are parallel because each series has an observation at the same time steps.
We can demonstrate this with a simple example of two parallel input time series where the output series is the simple addition of the input series.
1 2 3 4 5 |
... # define input sequence in_seq1 = array([10, 20, 30, 40, 50, 60, 70, 80, 90]) in_seq2 = array([15, 25, 35, 45, 55, 65, 75, 85, 95]) out_seq = array([in_seq1[i]+in_seq2[i] for i in range(len(in_seq1))]) |
We can reshape these three arrays of data as a single dataset where each row is a time step, and each column is a separate time series. This is a standard way of storing parallel time series in a CSV file.
1 2 3 4 5 6 7 |
... # convert to [rows, columns] structure in_seq1 = in_seq1.reshape((len(in_seq1), 1)) in_seq2 = in_seq2.reshape((len(in_seq2), 1)) out_seq = out_seq.reshape((len(out_seq), 1)) # horizontally stack columns dataset = hstack((in_seq1, in_seq2, out_seq)) |
The complete example is listed below.
1 2 3 4 5 6 7 8 9 10 11 12 13 14 |
# multivariate data preparation from numpy import array from numpy import hstack # define input sequence in_seq1 = array([10, 20, 30, 40, 50, 60, 70, 80, 90]) in_seq2 = array([15, 25, 35, 45, 55, 65, 75, 85, 95]) out_seq = array([in_seq1[i]+in_seq2[i] for i in range(len(in_seq1))]) # convert to [rows, columns] structure in_seq1 = in_seq1.reshape((len(in_seq1), 1)) in_seq2 = in_seq2.reshape((len(in_seq2), 1)) out_seq = out_seq.reshape((len(out_seq), 1)) # horizontally stack columns dataset = hstack((in_seq1, in_seq2, out_seq)) print(dataset) |
Running the example prints the dataset with one row per time step and one column for each of the two input and one output parallel time series.
1 2 3 4 5 6 7 8 9 |
[[ 10 15 25] [ 20 25 45] [ 30 35 65] [ 40 45 85] [ 50 55 105] [ 60 65 125] [ 70 75 145] [ 80 85 165] [ 90 95 185]] |
As with the univariate time series, we must structure these data into samples with input and output elements.
An LSTM model needs sufficient context to learn a mapping from an input sequence to an output value. LSTMs can support parallel input time series as separate variables or features. Therefore, we need to split the data into samples maintaining the order of observations across the two input sequences.
If we chose three input time steps, then the first sample would look as follows:
Input:
1 2 3 |
10, 15 20, 25 30, 35 |
Output:
1 |
65 |
That is, the first three time steps of each parallel series are provided as input to the model and the model associates this with the value in the output series at the third time step, in this case, 65.
We can see that, in transforming the time series into input/output samples to train the model, that we will have to discard some values from the output time series where we do not have values in the input time series at prior time steps. In turn, the choice of the size of the number of input time steps will have an important effect on how much of the training data is used.
We can define a function named split_sequences() that will take a dataset as we have defined it with rows for time steps and columns for parallel series and return input/output samples.
1 2 3 4 5 6 7 8 9 10 11 12 13 14 |
# split a multivariate sequence into samples def split_sequences(sequences, n_steps): X, y = list(), list() for i in range(len(sequences)): # find the end of this pattern end_ix = i + n_steps # check if we are beyond the dataset if end_ix > len(sequences): break # gather input and output parts of the pattern seq_x, seq_y = sequences[i:end_ix, :-1], sequences[end_ix-1, -1] X.append(seq_x) y.append(seq_y) return array(X), array(y) |
We can test this function on our dataset using three time steps for each input time series as input.
The complete example is listed below.
1 2 3 4 5 6 7 8 9 10 11 12 13 14 15 16 17 18 19 20 21 22 23 24 25 26 27 28 29 30 31 32 33 34 35 36 37 |
# multivariate data preparation from numpy import array from numpy import hstack # split a multivariate sequence into samples def split_sequences(sequences, n_steps): X, y = list(), list() for i in range(len(sequences)): # find the end of this pattern end_ix = i + n_steps # check if we are beyond the dataset if end_ix > len(sequences): break # gather input and output parts of the pattern seq_x, seq_y = sequences[i:end_ix, :-1], sequences[end_ix-1, -1] X.append(seq_x) y.append(seq_y) return array(X), array(y) # define input sequence in_seq1 = array([10, 20, 30, 40, 50, 60, 70, 80, 90]) in_seq2 = array([15, 25, 35, 45, 55, 65, 75, 85, 95]) out_seq = array([in_seq1[i]+in_seq2[i] for i in range(len(in_seq1))]) # convert to [rows, columns] structure in_seq1 = in_seq1.reshape((len(in_seq1), 1)) in_seq2 = in_seq2.reshape((len(in_seq2), 1)) out_seq = out_seq.reshape((len(out_seq), 1)) # horizontally stack columns dataset = hstack((in_seq1, in_seq2, out_seq)) # choose a number of time steps n_steps = 3 # convert into input/output X, y = split_sequences(dataset, n_steps) print(X.shape, y.shape) # summarize the data for i in range(len(X)): print(X[i], y[i]) |
Running the example first prints the shape of the X and y components.
We can see that the X component has a three-dimensional structure.
The first dimension is the number of samples, in this case 7. The second dimension is the number of time steps per sample, in this case 3, the value specified to the function. Finally, the last dimension specifies the number of parallel time series or the number of variables, in this case 2 for the two parallel series.
This is the exact three-dimensional structure expected by an LSTM as input. The data is ready to use without further reshaping.
We can then see that the input and output for each sample is printed, showing the three time steps for each of the two input series and the associated output for each sample.
1 2 3 4 5 6 7 8 9 10 11 12 13 14 15 16 17 18 19 20 21 22 23 |
(7, 3, 2) (7,) [[10 15] [20 25] [30 35]] 65 [[20 25] [30 35] [40 45]] 85 [[30 35] [40 45] [50 55]] 105 [[40 45] [50 55] [60 65]] 125 [[50 55] [60 65] [70 75]] 145 [[60 65] [70 75] [80 85]] 165 [[70 75] [80 85] [90 95]] 185 |
We are now ready to fit an LSTM model on this data.
Any of the varieties of LSTMs in the previous section can be used, such as a Vanilla, Stacked, Bidirectional, CNN, or ConvLSTM model.
We will use a Vanilla LSTM where the number of time steps and parallel series (features) are specified for the input layer via the input_shape argument.
1 2 3 4 5 6 |
... # define model model = Sequential() model.add(LSTM(50, activation='relu', input_shape=(n_steps, n_features))) model.add(Dense(1)) model.compile(optimizer='adam', loss='mse') |
When making a prediction, the model expects three time steps for two input time series.
We can predict the next value in the output series providing the input values of:
1 2 3 |
80, 85 90, 95 100, 105 |
The shape of the one sample with three time steps and two variables must be [1, 3, 2].
We would expect the next value in the sequence to be 100 + 105, or 205.
1 2 3 4 5 |
... # demonstrate prediction x_input = array([[80, 85], [90, 95], [100, 105]]) x_input = x_input.reshape((1, n_steps, n_features)) yhat = model.predict(x_input, verbose=0) |
The complete example is listed below.
1 2 3 4 5 6 7 8 9 10 11 12 13 14 15 16 17 18 19 20 21 22 23 24 25 26 27 28 29 30 31 32 33 34 35 36 37 38 39 40 41 42 43 44 45 46 47 48 49 50 |
# multivariate lstm example from numpy import array from numpy import hstack from keras.models import Sequential from keras.layers import LSTM from keras.layers import Dense # split a multivariate sequence into samples def split_sequences(sequences, n_steps): X, y = list(), list() for i in range(len(sequences)): # find the end of this pattern end_ix = i + n_steps # check if we are beyond the dataset if end_ix > len(sequences): break # gather input and output parts of the pattern seq_x, seq_y = sequences[i:end_ix, :-1], sequences[end_ix-1, -1] X.append(seq_x) y.append(seq_y) return array(X), array(y) # define input sequence in_seq1 = array([10, 20, 30, 40, 50, 60, 70, 80, 90]) in_seq2 = array([15, 25, 35, 45, 55, 65, 75, 85, 95]) out_seq = array([in_seq1[i]+in_seq2[i] for i in range(len(in_seq1))]) # convert to [rows, columns] structure in_seq1 = in_seq1.reshape((len(in_seq1), 1)) in_seq2 = in_seq2.reshape((len(in_seq2), 1)) out_seq = out_seq.reshape((len(out_seq), 1)) # horizontally stack columns dataset = hstack((in_seq1, in_seq2, out_seq)) # choose a number of time steps n_steps = 3 # convert into input/output X, y = split_sequences(dataset, n_steps) # the dataset knows the number of features, e.g. 2 n_features = X.shape[2] # define model model = Sequential() model.add(LSTM(50, activation='relu', input_shape=(n_steps, n_features))) model.add(Dense(1)) model.compile(optimizer='adam', loss='mse') # fit model model.fit(X, y, epochs=200, verbose=0) # demonstrate prediction x_input = array([[80, 85], [90, 95], [100, 105]]) x_input = x_input.reshape((1, n_steps, n_features)) yhat = model.predict(x_input, verbose=0) print(yhat) |
Note: Your results may vary given the stochastic nature of the algorithm or evaluation procedure, or differences in numerical precision. Consider running the example a few times and compare the average outcome.
Running the example prepares the data, fits the model, and makes a prediction.
1 |
[[208.13531]] |
Multiple Parallel Series
An alternate time series problem is the case where there are multiple parallel time series and a value must be predicted for each.
For example, given the data from the previous section:
1 2 3 4 5 6 7 8 9 |
[[ 10 15 25] [ 20 25 45] [ 30 35 65] [ 40 45 85] [ 50 55 105] [ 60 65 125] [ 70 75 145] [ 80 85 165] [ 90 95 185]] |
We may want to predict the value for each of the three time series for the next time step.
This might be referred to as multivariate forecasting.
Again, the data must be split into input/output samples in order to train a model.
The first sample of this dataset would be:
Input:
1 2 3 |
10, 15, 25 20, 25, 45 30, 35, 65 |
Output:
1 |
40, 45, 85 |
The split_sequences() function below will split multiple parallel time series with rows for time steps and one series per column into the required input/output shape.
1 2 3 4 5 6 7 8 9 10 11 12 13 14 |
# split a multivariate sequence into samples def split_sequences(sequences, n_steps): X, y = list(), list() for i in range(len(sequences)): # find the end of this pattern end_ix = i + n_steps # check if we are beyond the dataset if end_ix > len(sequences)-1: break # gather input and output parts of the pattern seq_x, seq_y = sequences[i:end_ix, :], sequences[end_ix, :] X.append(seq_x) y.append(seq_y) return array(X), array(y) |
We can demonstrate this on the contrived problem; the complete example is listed below.
1 2 3 4 5 6 7 8 9 10 11 12 13 14 15 16 17 18 19 20 21 22 23 24 25 26 27 28 29 30 31 32 33 34 35 36 37 |
# multivariate output data prep from numpy import array from numpy import hstack # split a multivariate sequence into samples def split_sequences(sequences, n_steps): X, y = list(), list() for i in range(len(sequences)): # find the end of this pattern end_ix = i + n_steps # check if we are beyond the dataset if end_ix > len(sequences)-1: break # gather input and output parts of the pattern seq_x, seq_y = sequences[i:end_ix, :], sequences[end_ix, :] X.append(seq_x) y.append(seq_y) return array(X), array(y) # define input sequence in_seq1 = array([10, 20, 30, 40, 50, 60, 70, 80, 90]) in_seq2 = array([15, 25, 35, 45, 55, 65, 75, 85, 95]) out_seq = array([in_seq1[i]+in_seq2[i] for i in range(len(in_seq1))]) # convert to [rows, columns] structure in_seq1 = in_seq1.reshape((len(in_seq1), 1)) in_seq2 = in_seq2.reshape((len(in_seq2), 1)) out_seq = out_seq.reshape((len(out_seq), 1)) # horizontally stack columns dataset = hstack((in_seq1, in_seq2, out_seq)) # choose a number of time steps n_steps = 3 # convert into input/output X, y = split_sequences(dataset, n_steps) print(X.shape, y.shape) # summarize the data for i in range(len(X)): print(X[i], y[i]) |
Running the example first prints the shape of the prepared X and y components.
The shape of X is three-dimensional, including the number of samples (6), the number of time steps chosen per sample (3), and the number of parallel time series or features (3).
The shape of y is two-dimensional as we might expect for the number of samples (6) and the number of time variables per sample to be predicted (3).
The data is ready to use in an LSTM model that expects three-dimensional input and two-dimensional output shapes for the X and y components of each sample.
Then, each of the samples is printed showing the input and output components of each sample.
1 2 3 4 5 6 7 8 9 10 11 12 13 14 15 16 17 18 19 20 |
(6, 3, 3) (6, 3) [[10 15 25] [20 25 45] [30 35 65]] [40 45 85] [[20 25 45] [30 35 65] [40 45 85]] [ 50 55 105] [[ 30 35 65] [ 40 45 85] [ 50 55 105]] [ 60 65 125] [[ 40 45 85] [ 50 55 105] [ 60 65 125]] [ 70 75 145] [[ 50 55 105] [ 60 65 125] [ 70 75 145]] [ 80 85 165] [[ 60 65 125] [ 70 75 145] [ 80 85 165]] [ 90 95 185] |
We are now ready to fit an LSTM model on this data.
Any of the varieties of LSTMs in the previous section can be used, such as a Vanilla, Stacked, Bidirectional, CNN, or ConvLSTM model.
We will use a Stacked LSTM where the number of time steps and parallel series (features) are specified for the input layer via the input_shape argument. The number of parallel series is also used in the specification of the number of values to predict by the model in the output layer; again, this is three.
1 2 3 4 5 6 7 |
... # define model model = Sequential() model.add(LSTM(100, activation='relu', return_sequences=True, input_shape=(n_steps, n_features))) model.add(LSTM(100, activation='relu')) model.add(Dense(n_features)) model.compile(optimizer='adam', loss='mse') |
We can predict the next value in each of the three parallel series by providing an input of three time steps for each series.
1 2 3 |
70, 75, 145 80, 85, 165 90, 95, 185 |
The shape of the input for making a single prediction must be 1 sample, 3 time steps, and 3 features, or [1, 3, 3]
1 2 3 4 5 |
... # demonstrate prediction x_input = array([[70,75,145], [80,85,165], [90,95,185]]) x_input = x_input.reshape((1, n_steps, n_features)) yhat = model.predict(x_input, verbose=0) |
We would expect the vector output to be:
1 |
[100, 105, 205] |
We can tie all of this together and demonstrate a Stacked LSTM for multivariate output time series forecasting below.
1 2 3 4 5 6 7 8 9 10 11 12 13 14 15 16 17 18 19 20 21 22 23 24 25 26 27 28 29 30 31 32 33 34 35 36 37 38 39 40 41 42 43 44 45 46 47 48 49 50 51 |
# multivariate output stacked lstm example from numpy import array from numpy import hstack from keras.models import Sequential from keras.layers import LSTM from keras.layers import Dense # split a multivariate sequence into samples def split_sequences(sequences, n_steps): X, y = list(), list() for i in range(len(sequences)): # find the end of this pattern end_ix = i + n_steps # check if we are beyond the dataset if end_ix > len(sequences)-1: break # gather input and output parts of the pattern seq_x, seq_y = sequences[i:end_ix, :], sequences[end_ix, :] X.append(seq_x) y.append(seq_y) return array(X), array(y) # define input sequence in_seq1 = array([10, 20, 30, 40, 50, 60, 70, 80, 90]) in_seq2 = array([15, 25, 35, 45, 55, 65, 75, 85, 95]) out_seq = array([in_seq1[i]+in_seq2[i] for i in range(len(in_seq1))]) # convert to [rows, columns] structure in_seq1 = in_seq1.reshape((len(in_seq1), 1)) in_seq2 = in_seq2.reshape((len(in_seq2), 1)) out_seq = out_seq.reshape((len(out_seq), 1)) # horizontally stack columns dataset = hstack((in_seq1, in_seq2, out_seq)) # choose a number of time steps n_steps = 3 # convert into input/output X, y = split_sequences(dataset, n_steps) # the dataset knows the number of features, e.g. 2 n_features = X.shape[2] # define model model = Sequential() model.add(LSTM(100, activation='relu', return_sequences=True, input_shape=(n_steps, n_features))) model.add(LSTM(100, activation='relu')) model.add(Dense(n_features)) model.compile(optimizer='adam', loss='mse') # fit model model.fit(X, y, epochs=400, verbose=0) # demonstrate prediction x_input = array([[70,75,145], [80,85,165], [90,95,185]]) x_input = x_input.reshape((1, n_steps, n_features)) yhat = model.predict(x_input, verbose=0) print(yhat) |
Note: Your results may vary given the stochastic nature of the algorithm or evaluation procedure, or differences in numerical precision. Consider running the example a few times and compare the average outcome.
Running the example prepares the data, fits the model, and makes a prediction.
1 |
[[101.76599 108.730484 206.63577 ]] |
Multi-Step LSTM Models
A time series forecasting problem that requires a prediction of multiple time steps into the future can be referred to as multi-step time series forecasting.
Specifically, these are problems where the forecast horizon or interval is more than one time step.
There are two main types of LSTM models that can be used for multi-step forecasting; they are:
- Vector Output Model
- Encoder-Decoder Model
Before we look at these models, let’s first look at the preparation of data for multi-step forecasting.
Data Preparation
As with one-step forecasting, a time series used for multi-step time series forecasting must be split into samples with input and output components.
Both the input and output components will be comprised of multiple time steps and may or may not have the same number of steps.
For example, given the univariate time series:
1 |
[10, 20, 30, 40, 50, 60, 70, 80, 90] |
We could use the last three time steps as input and forecast the next two time steps.
The first sample would look as follows:
Input:
1 |
[10, 20, 30] |
Output:
1 |
[40, 50] |
The split_sequence() function below implements this behavior and will split a given univariate time series into samples with a specified number of input and output time steps.
1 2 3 4 5 6 7 8 9 10 11 12 13 14 15 |
# split a univariate sequence into samples def split_sequence(sequence, n_steps_in, n_steps_out): X, y = list(), list() for i in range(len(sequence)): # find the end of this pattern end_ix = i + n_steps_in out_end_ix = end_ix + n_steps_out # check if we are beyond the sequence if out_end_ix > len(sequence): break # gather input and output parts of the pattern seq_x, seq_y = sequence[i:end_ix], sequence[end_ix:out_end_ix] X.append(seq_x) y.append(seq_y) return array(X), array(y) |
We can demonstrate this function on the small contrived dataset.
The complete example is listed below.
1 2 3 4 5 6 7 8 9 10 11 12 13 14 15 16 17 18 19 20 21 22 23 24 25 26 27 28 |
# multi-step data preparation from numpy import array # split a univariate sequence into samples def split_sequence(sequence, n_steps_in, n_steps_out): X, y = list(), list() for i in range(len(sequence)): # find the end of this pattern end_ix = i + n_steps_in out_end_ix = end_ix + n_steps_out # check if we are beyond the sequence if out_end_ix > len(sequence): break # gather input and output parts of the pattern seq_x, seq_y = sequence[i:end_ix], sequence[end_ix:out_end_ix] X.append(seq_x) y.append(seq_y) return array(X), array(y) # define input sequence raw_seq = [10, 20, 30, 40, 50, 60, 70, 80, 90] # choose a number of time steps n_steps_in, n_steps_out = 3, 2 # split into samples X, y = split_sequence(raw_seq, n_steps_in, n_steps_out) # summarize the data for i in range(len(X)): print(X[i], y[i]) |
Running the example splits the univariate series into input and output time steps and prints the input and output components of each.
1 2 3 4 5 |
[10 20 30] [40 50] [20 30 40] [50 60] [30 40 50] [60 70] [40 50 60] [70 80] [50 60 70] [80 90] |
Now that we know how to prepare data for multi-step forecasting, let’s look at some LSTM models that can learn this mapping.
Vector Output Model
Like other types of neural network models, the LSTM can output a vector directly that can be interpreted as a multi-step forecast.
This approach was seen in the previous section were one time step of each output time series was forecasted as a vector.
As with the LSTMs for univariate data in a prior section, the prepared samples must first be reshaped. The LSTM expects data to have a three-dimensional structure of [samples, timesteps, features], and in this case, we only have one feature so the reshape is straightforward.
1 2 3 4 |
... # reshape from [samples, timesteps] into [samples, timesteps, features] n_features = 1 X = X.reshape((X.shape[0], X.shape[1], n_features)) |
With the number of input and output steps specified in the n_steps_in and n_steps_out variables, we can define a multi-step time-series forecasting model.
Any of the presented LSTM model types could be used, such as Vanilla, Stacked, Bidirectional, CNN-LSTM, or ConvLSTM. Below defines a Stacked LSTM for multi-step forecasting.
1 2 3 4 5 6 7 |
... # define model model = Sequential() model.add(LSTM(100, activation='relu', return_sequences=True, input_shape=(n_steps_in, n_features))) model.add(LSTM(100, activation='relu')) model.add(Dense(n_steps_out)) model.compile(optimizer='adam', loss='mse') |
The model can make a prediction for a single sample. We can predict the next two steps beyond the end of the dataset by providing the input:
1 |
[70, 80, 90] |
We would expect the predicted output to be:
1 |
[100, 110] |
As expected by the model, the shape of the single sample of input data when making the prediction must be [1, 3, 1] for the 1 sample, 3 time steps of the input, and the single feature.
1 2 3 4 5 |
... # demonstrate prediction x_input = array([70, 80, 90]) x_input = x_input.reshape((1, n_steps_in, n_features)) yhat = model.predict(x_input, verbose=0) |
Tying all of this together, the Stacked LSTM for multi-step forecasting with a univariate time series is listed below.
1 2 3 4 5 6 7 8 9 10 11 12 13 14 15 16 17 18 19 20 21 22 23 24 25 26 27 28 29 30 31 32 33 34 35 36 37 38 39 40 41 42 43 44 |
# univariate multi-step vector-output stacked lstm example from numpy import array from keras.models import Sequential from keras.layers import LSTM from keras.layers import Dense # split a univariate sequence into samples def split_sequence(sequence, n_steps_in, n_steps_out): X, y = list(), list() for i in range(len(sequence)): # find the end of this pattern end_ix = i + n_steps_in out_end_ix = end_ix + n_steps_out # check if we are beyond the sequence if out_end_ix > len(sequence): break # gather input and output parts of the pattern seq_x, seq_y = sequence[i:end_ix], sequence[end_ix:out_end_ix] X.append(seq_x) y.append(seq_y) return array(X), array(y) # define input sequence raw_seq = [10, 20, 30, 40, 50, 60, 70, 80, 90] # choose a number of time steps n_steps_in, n_steps_out = 3, 2 # split into samples X, y = split_sequence(raw_seq, n_steps_in, n_steps_out) # reshape from [samples, timesteps] into [samples, timesteps, features] n_features = 1 X = X.reshape((X.shape[0], X.shape[1], n_features)) # define model model = Sequential() model.add(LSTM(100, activation='relu', return_sequences=True, input_shape=(n_steps_in, n_features))) model.add(LSTM(100, activation='relu')) model.add(Dense(n_steps_out)) model.compile(optimizer='adam', loss='mse') # fit model model.fit(X, y, epochs=50, verbose=0) # demonstrate prediction x_input = array([70, 80, 90]) x_input = x_input.reshape((1, n_steps_in, n_features)) yhat = model.predict(x_input, verbose=0) print(yhat) |
Note: Your results may vary given the stochastic nature of the algorithm or evaluation procedure, or differences in numerical precision. Consider running the example a few times and compare the average outcome.
Running the example forecasts and prints the next two time steps in the sequence.
1 |
[[100.98096 113.28924]] |
Encoder-Decoder Model
A model specifically developed for forecasting variable length output sequences is called the Encoder-Decoder LSTM.
The model was designed for prediction problems where there are both input and output sequences, so-called sequence-to-sequence, or seq2seq problems, such as translating text from one language to another.
This model can be used for multi-step time series forecasting.
As its name suggests, the model is comprised of two sub-models: the encoder and the decoder.
The encoder is a model responsible for reading and interpreting the input sequence. The output of the encoder is a fixed length vector that represents the model’s interpretation of the sequence. The encoder is traditionally a Vanilla LSTM model, although other encoder models can be used such as Stacked, Bidirectional, and CNN models.
1 2 |
... model.add(LSTM(100, activation='relu', input_shape=(n_steps_in, n_features))) |
The decoder uses the output of the encoder as an input.
First, the fixed-length output of the encoder is repeated, once for each required time step in the output sequence.
1 2 |
... model.add(RepeatVector(n_steps_out)) |
This sequence is then provided to an LSTM decoder model. The model must output a value for each value in the output time step, which can be interpreted by a single output model.
1 2 |
... model.add(LSTM(100, activation='relu', return_sequences=True)) |
We can use the same output layer or layers to make each one-step prediction in the output sequence. This can be achieved by wrapping the output part of the model in a TimeDistributed wrapper.
1 2 |
.... model.add(TimeDistributed(Dense(1))) |
The full definition for an Encoder-Decoder model for multi-step time series forecasting is listed below.
1 2 3 4 5 6 7 8 |
... # define model model = Sequential() model.add(LSTM(100, activation='relu', input_shape=(n_steps_in, n_features))) model.add(RepeatVector(n_steps_out)) model.add(LSTM(100, activation='relu', return_sequences=True)) model.add(TimeDistributed(Dense(1))) model.compile(optimizer='adam', loss='mse') |
As with other LSTM models, the input data must be reshaped into the expected three-dimensional shape of [samples, timesteps, features].
1 2 |
... X = X.reshape((X.shape[0], X.shape[1], n_features)) |
In the case of the Encoder-Decoder model, the output, or y part, of the training dataset must also have this shape. This is because the model will predict a given number of time steps with a given number of features for each input sample.
1 2 |
... y = y.reshape((y.shape[0], y.shape[1], n_features)) |
The complete example of an Encoder-Decoder LSTM for multi-step time series forecasting is listed below.
1 2 3 4 5 6 7 8 9 10 11 12 13 14 15 16 17 18 19 20 21 22 23 24 25 26 27 28 29 30 31 32 33 34 35 36 37 38 39 40 41 42 43 44 45 46 47 48 |
# univariate multi-step encoder-decoder lstm example from numpy import array from keras.models import Sequential from keras.layers import LSTM from keras.layers import Dense from keras.layers import RepeatVector from keras.layers import TimeDistributed # split a univariate sequence into samples def split_sequence(sequence, n_steps_in, n_steps_out): X, y = list(), list() for i in range(len(sequence)): # find the end of this pattern end_ix = i + n_steps_in out_end_ix = end_ix + n_steps_out # check if we are beyond the sequence if out_end_ix > len(sequence): break # gather input and output parts of the pattern seq_x, seq_y = sequence[i:end_ix], sequence[end_ix:out_end_ix] X.append(seq_x) y.append(seq_y) return array(X), array(y) # define input sequence raw_seq = [10, 20, 30, 40, 50, 60, 70, 80, 90] # choose a number of time steps n_steps_in, n_steps_out = 3, 2 # split into samples X, y = split_sequence(raw_seq, n_steps_in, n_steps_out) # reshape from [samples, timesteps] into [samples, timesteps, features] n_features = 1 X = X.reshape((X.shape[0], X.shape[1], n_features)) y = y.reshape((y.shape[0], y.shape[1], n_features)) # define model model = Sequential() model.add(LSTM(100, activation='relu', input_shape=(n_steps_in, n_features))) model.add(RepeatVector(n_steps_out)) model.add(LSTM(100, activation='relu', return_sequences=True)) model.add(TimeDistributed(Dense(1))) model.compile(optimizer='adam', loss='mse') # fit model model.fit(X, y, epochs=100, verbose=0) # demonstrate prediction x_input = array([70, 80, 90]) x_input = x_input.reshape((1, n_steps_in, n_features)) yhat = model.predict(x_input, verbose=0) print(yhat) |
Note: Your results may vary given the stochastic nature of the algorithm or evaluation procedure, or differences in numerical precision. Consider running the example a few times and compare the average outcome.
Running the example forecasts and prints the next two time steps in the sequence.
1 2 |
[[[101.9736 [116.213615]]] |
Multivariate Multi-Step LSTM Models
In the previous sections, we have looked at univariate, multivariate, and multi-step time series forecasting.
It is possible to mix and match the different types of LSTM models presented so far for the different problems. This too applies to time series forecasting problems that involve multivariate and multi-step forecasting, but it may be a little more challenging.
In this section, we will provide short examples of data preparation and modeling for multivariate multi-step time series forecasting as a template to ease this challenge, specifically:
- Multiple Input Multi-Step Output.
- Multiple Parallel Input and Multi-Step Output.
Perhaps the biggest stumbling block is in the preparation of data, so this is where we will focus our attention.
Multiple Input Multi-Step Output
There are those multivariate time series forecasting problems where the output series is separate but dependent upon the input time series, and multiple time steps are required for the output series.
For example, consider our multivariate time series from a prior section:
1 2 3 4 5 6 7 8 9 |
[[ 10 15 25] [ 20 25 45] [ 30 35 65] [ 40 45 85] [ 50 55 105] [ 60 65 125] [ 70 75 145] [ 80 85 165] [ 90 95 185]] |
We may use three prior time steps of each of the two input time series to predict two time steps of the output time series.
Input:
1 2 3 |
10, 15 20, 25 30, 35 |
Output:
1 2 |
65 85 |
The split_sequences() function below implements this behavior.
1 2 3 4 5 6 7 8 9 10 11 12 13 14 15 |
# split a multivariate sequence into samples def split_sequences(sequences, n_steps_in, n_steps_out): X, y = list(), list() for i in range(len(sequences)): # find the end of this pattern end_ix = i + n_steps_in out_end_ix = end_ix + n_steps_out-1 # check if we are beyond the dataset if out_end_ix > len(sequences): break # gather input and output parts of the pattern seq_x, seq_y = sequences[i:end_ix, :-1], sequences[end_ix-1:out_end_ix, -1] X.append(seq_x) y.append(seq_y) return array(X), array(y) |
We can demonstrate this on our contrived dataset.
The complete example is listed below.
1 2 3 4 5 6 7 8 9 10 11 12 13 14 15 16 17 18 19 20 21 22 23 24 25 26 27 28 29 30 31 32 33 34 35 36 37 38 |
# multivariate multi-step data preparation from numpy import array from numpy import hstack # split a multivariate sequence into samples def split_sequences(sequences, n_steps_in, n_steps_out): X, y = list(), list() for i in range(len(sequences)): # find the end of this pattern end_ix = i + n_steps_in out_end_ix = end_ix + n_steps_out-1 # check if we are beyond the dataset if out_end_ix > len(sequences): break # gather input and output parts of the pattern seq_x, seq_y = sequences[i:end_ix, :-1], sequences[end_ix-1:out_end_ix, -1] X.append(seq_x) y.append(seq_y) return array(X), array(y) # define input sequence in_seq1 = array([10, 20, 30, 40, 50, 60, 70, 80, 90]) in_seq2 = array([15, 25, 35, 45, 55, 65, 75, 85, 95]) out_seq = array([in_seq1[i]+in_seq2[i] for i in range(len(in_seq1))]) # convert to [rows, columns] structure in_seq1 = in_seq1.reshape((len(in_seq1), 1)) in_seq2 = in_seq2.reshape((len(in_seq2), 1)) out_seq = out_seq.reshape((len(out_seq), 1)) # horizontally stack columns dataset = hstack((in_seq1, in_seq2, out_seq)) # choose a number of time steps n_steps_in, n_steps_out = 3, 2 # covert into input/output X, y = split_sequences(dataset, n_steps_in, n_steps_out) print(X.shape, y.shape) # summarize the data for i in range(len(X)): print(X[i], y[i]) |
Running the example first prints the shape of the prepared training data.
We can see that the shape of the input portion of the samples is three-dimensional, comprised of six samples, with three time steps, and two variables for the 2 input time series.
The output portion of the samples is two-dimensional for the six samples and the two time steps for each sample to be predicted.
The prepared samples are then printed to confirm that the data was prepared as we specified.
1 2 3 4 5 6 7 8 9 10 11 12 13 14 15 16 17 18 19 20 |
(6, 3, 2) (6, 2) [[10 15] [20 25] [30 35]] [65 85] [[20 25] [30 35] [40 45]] [ 85 105] [[30 35] [40 45] [50 55]] [105 125] [[40 45] [50 55] [60 65]] [125 145] [[50 55] [60 65] [70 75]] [145 165] [[60 65] [70 75] [80 85]] [165 185] |
We can now develop an LSTM model for multi-step predictions.
A vector output or an encoder-decoder model could be used. In this case, we will demonstrate a vector output with a Stacked LSTM.
The complete example is listed below.
1 2 3 4 5 6 7 8 9 10 11 12 13 14 15 16 17 18 19 20 21 22 23 24 25 26 27 28 29 30 31 32 33 34 35 36 37 38 39 40 41 42 43 44 45 46 47 48 49 50 51 52 |
# multivariate multi-step stacked lstm example from numpy import array from numpy import hstack from keras.models import Sequential from keras.layers import LSTM from keras.layers import Dense # split a multivariate sequence into samples def split_sequences(sequences, n_steps_in, n_steps_out): X, y = list(), list() for i in range(len(sequences)): # find the end of this pattern end_ix = i + n_steps_in out_end_ix = end_ix + n_steps_out-1 # check if we are beyond the dataset if out_end_ix > len(sequences): break # gather input and output parts of the pattern seq_x, seq_y = sequences[i:end_ix, :-1], sequences[end_ix-1:out_end_ix, -1] X.append(seq_x) y.append(seq_y) return array(X), array(y) # define input sequence in_seq1 = array([10, 20, 30, 40, 50, 60, 70, 80, 90]) in_seq2 = array([15, 25, 35, 45, 55, 65, 75, 85, 95]) out_seq = array([in_seq1[i]+in_seq2[i] for i in range(len(in_seq1))]) # convert to [rows, columns] structure in_seq1 = in_seq1.reshape((len(in_seq1), 1)) in_seq2 = in_seq2.reshape((len(in_seq2), 1)) out_seq = out_seq.reshape((len(out_seq), 1)) # horizontally stack columns dataset = hstack((in_seq1, in_seq2, out_seq)) # choose a number of time steps n_steps_in, n_steps_out = 3, 2 # covert into input/output X, y = split_sequences(dataset, n_steps_in, n_steps_out) # the dataset knows the number of features, e.g. 2 n_features = X.shape[2] # define model model = Sequential() model.add(LSTM(100, activation='relu', return_sequences=True, input_shape=(n_steps_in, n_features))) model.add(LSTM(100, activation='relu')) model.add(Dense(n_steps_out)) model.compile(optimizer='adam', loss='mse') # fit model model.fit(X, y, epochs=200, verbose=0) # demonstrate prediction x_input = array([[70, 75], [80, 85], [90, 95]]) x_input = x_input.reshape((1, n_steps_in, n_features)) yhat = model.predict(x_input, verbose=0) print(yhat) |
Running the example fits the model and predicts the next two time steps of the output sequence beyond the dataset.
We would expect the next two steps to be: [185, 205]
Note: Your results may vary given the stochastic nature of the algorithm or evaluation procedure, or differences in numerical precision. Consider running the example a few times and compare the average outcome.
It is a challenging framing of the problem with very little data, and the arbitrarily configured version of the model gets close.
1 |
[[188.70619 210.16513]] |
Multiple Parallel Input and Multi-Step Output
A problem with parallel time series may require the prediction of multiple time steps of each time series.
For example, consider our multivariate time series from a prior section:
1 2 3 4 5 6 7 8 9 |
[[ 10 15 25] [ 20 25 45] [ 30 35 65] [ 40 45 85] [ 50 55 105] [ 60 65 125] [ 70 75 145] [ 80 85 165] [ 90 95 185]] |
We may use the last three time steps from each of the three time series as input to the model and predict the next time steps of each of the three time series as output.
The first sample in the training dataset would be the following.
Input:
1 2 3 |
10, 15, 25 20, 25, 45 30, 35, 65 |
Output:
1 2 |
40, 45, 85 50, 55, 105 |
The split_sequences() function below implements this behavior.
1 2 3 4 5 6 7 8 9 10 11 12 13 14 15 |
# split a multivariate sequence into samples def split_sequences(sequences, n_steps_in, n_steps_out): X, y = list(), list() for i in range(len(sequences)): # find the end of this pattern end_ix = i + n_steps_in out_end_ix = end_ix + n_steps_out # check if we are beyond the dataset if out_end_ix > len(sequences): break # gather input and output parts of the pattern seq_x, seq_y = sequences[i:end_ix, :], sequences[end_ix:out_end_ix, :] X.append(seq_x) y.append(seq_y) return array(X), array(y) |
We can demonstrate this function on the small contrived dataset.
The complete example is listed below.
1 2 3 4 5 6 7 8 9 10 11 12 13 14 15 16 17 18 19 20 21 22 23 24 25 26 27 28 29 30 31 32 33 34 35 36 37 38 39 40 41 42 43 |
# multivariate multi-step data preparation from numpy import array from numpy import hstack from keras.models import Sequential from keras.layers import LSTM from keras.layers import Dense from keras.layers import RepeatVector from keras.layers import TimeDistributed # split a multivariate sequence into samples def split_sequences(sequences, n_steps_in, n_steps_out): X, y = list(), list() for i in range(len(sequences)): # find the end of this pattern end_ix = i + n_steps_in out_end_ix = end_ix + n_steps_out # check if we are beyond the dataset if out_end_ix > len(sequences): break # gather input and output parts of the pattern seq_x, seq_y = sequences[i:end_ix, :], sequences[end_ix:out_end_ix, :] X.append(seq_x) y.append(seq_y) return array(X), array(y) # define input sequence in_seq1 = array([10, 20, 30, 40, 50, 60, 70, 80, 90]) in_seq2 = array([15, 25, 35, 45, 55, 65, 75, 85, 95]) out_seq = array([in_seq1[i]+in_seq2[i] for i in range(len(in_seq1))]) # convert to [rows, columns] structure in_seq1 = in_seq1.reshape((len(in_seq1), 1)) in_seq2 = in_seq2.reshape((len(in_seq2), 1)) out_seq = out_seq.reshape((len(out_seq), 1)) # horizontally stack columns dataset = hstack((in_seq1, in_seq2, out_seq)) # choose a number of time steps n_steps_in, n_steps_out = 3, 2 # covert into input/output X, y = split_sequences(dataset, n_steps_in, n_steps_out) print(X.shape, y.shape) # summarize the data for i in range(len(X)): print(X[i], y[i]) |
Running the example first prints the shape of the prepared training dataset.
We can see that both the input (X) and output (Y) elements of the dataset are three dimensional for the number of samples, time steps, and variables or parallel time series respectively.
The input and output elements of each series are then printed side by side so that we can confirm that the data was prepared as we expected.
1 2 3 4 5 6 7 8 9 10 11 12 13 14 15 16 17 18 19 20 21 22 |
(5, 3, 3) (5, 2, 3) [[10 15 25] [20 25 45] [30 35 65]] [[ 40 45 85] [ 50 55 105]] [[20 25 45] [30 35 65] [40 45 85]] [[ 50 55 105] [ 60 65 125]] [[ 30 35 65] [ 40 45 85] [ 50 55 105]] [[ 60 65 125] [ 70 75 145]] [[ 40 45 85] [ 50 55 105] [ 60 65 125]] [[ 70 75 145] [ 80 85 165]] [[ 50 55 105] [ 60 65 125] [ 70 75 145]] [[ 80 85 165] [ 90 95 185]] |
We can use either the Vector Output or Encoder-Decoder LSTM to model this problem. In this case, we will use the Encoder-Decoder model.
The complete example is listed below.
1 2 3 4 5 6 7 8 9 10 11 12 13 14 15 16 17 18 19 20 21 22 23 24 25 26 27 28 29 30 31 32 33 34 35 36 37 38 39 40 41 42 43 44 45 46 47 48 49 50 51 52 53 54 55 |
# multivariate multi-step encoder-decoder lstm example from numpy import array from numpy import hstack from keras.models import Sequential from keras.layers import LSTM from keras.layers import Dense from keras.layers import RepeatVector from keras.layers import TimeDistributed # split a multivariate sequence into samples def split_sequences(sequences, n_steps_in, n_steps_out): X, y = list(), list() for i in range(len(sequences)): # find the end of this pattern end_ix = i + n_steps_in out_end_ix = end_ix + n_steps_out # check if we are beyond the dataset if out_end_ix > len(sequences): break # gather input and output parts of the pattern seq_x, seq_y = sequences[i:end_ix, :], sequences[end_ix:out_end_ix, :] X.append(seq_x) y.append(seq_y) return array(X), array(y) # define input sequence in_seq1 = array([10, 20, 30, 40, 50, 60, 70, 80, 90]) in_seq2 = array([15, 25, 35, 45, 55, 65, 75, 85, 95]) out_seq = array([in_seq1[i]+in_seq2[i] for i in range(len(in_seq1))]) # convert to [rows, columns] structure in_seq1 = in_seq1.reshape((len(in_seq1), 1)) in_seq2 = in_seq2.reshape((len(in_seq2), 1)) out_seq = out_seq.reshape((len(out_seq), 1)) # horizontally stack columns dataset = hstack((in_seq1, in_seq2, out_seq)) # choose a number of time steps n_steps_in, n_steps_out = 3, 2 # covert into input/output X, y = split_sequences(dataset, n_steps_in, n_steps_out) # the dataset knows the number of features, e.g. 2 n_features = X.shape[2] # define model model = Sequential() model.add(LSTM(200, activation='relu', input_shape=(n_steps_in, n_features))) model.add(RepeatVector(n_steps_out)) model.add(LSTM(200, activation='relu', return_sequences=True)) model.add(TimeDistributed(Dense(n_features))) model.compile(optimizer='adam', loss='mse') # fit model model.fit(X, y, epochs=300, verbose=0) # demonstrate prediction x_input = array([[60, 65, 125], [70, 75, 145], [80, 85, 165]]) x_input = x_input.reshape((1, n_steps_in, n_features)) yhat = model.predict(x_input, verbose=0) print(yhat) |
Running the example fits the model and predicts the values for each of the three time steps for the next two time steps beyond the end of the dataset.
We would expect the values for these series and time steps to be as follows:
1 2 |
90, 95, 185 100, 105, 205 |
Note: Your results may vary given the stochastic nature of the algorithm or evaluation procedure, or differences in numerical precision. Consider running the example a few times and compare the average outcome.
We can see that the model forecast gets reasonably close to the expected values.
1 2 |
[[[ 91.86044 97.77231 189.66768 ] [103.299355 109.18123 212.6863 ]]] |
Further Reading
- Long short-term memory, Wikipedia.
- Deep Learning for Time Series Forecasting (my book)
Summary
In this tutorial, you discovered how to develop a suite of LSTM models for a range of standard time series forecasting problems.
Specifically, you learned:
- How to develop LSTM models for univariate time series forecasting.
- How to develop LSTM models for multivariate time series forecasting.
- How to develop LSTM models for multi-step time series forecasting.
Do you have any questions?
Ask your questions in the comments below and I will do my best to answer.
This tutorial is so helpful to me. Thank you very much!
It will be more helpful in the real projects if the dataset is split into batches. Hope you will mention this in the future.
Keras will split the dataset into batches.
I think this blog ( https://machinelearningmastery.com/use-different-batch-sizes-training-predicting-python-keras/) may answer my question. I will do more research. Thanks a lot.
Great!
Thank you
Hi!
i would like to cite your book “Deep Learning for Time Series Forecasting: Predict the Future
with MLPs, CNNs and LSTMs in Python.” Is there an appropriate format for doing this?
Yes, see here:
https://machinelearningmastery.com/faq/single-faq/how-do-i-reference-or-cite-a-book-or-blog-post
Hi Jason,
I want please an example of Sliding window-based support vector regression for prediction.
have you this example .
Thanks a lot
Thanks for the suggestion.
Hi Jason, It was a great tutorial
I have a question :
IN Multiple Parallel inputs, the output of the LSTM Encdoer 0Decoder model will be 3D, how do we transform it back to 2D? I am asking this because I have performed scaling on the data using minmaxscaler() and it expects the input to be a 2d array.
In order to compare the predicted values with the original values, I need to perform inverse scaling, but I am stuck at how to reshape the 3d input and output back to 2d without losing any data.
You might need to write custom code to collect values for each variable before inverting the scale.
Hello Jason,
Thank you so so much for your post, it was super helpful. For the multiple timesteps output LSTM model, I am wondering what will be the difference of the performance between model-1 and model-2? Model-1 is your multiple timesteps output LSTM model, for example, we input last 7 days data features, and the output is the next 5 days prices. Model-2 is the simple 1-timstep output LSTM model, where the input is last 7 days data features, output is the next day price. Then we use our predicted price as the new input to predict future prices until we predict all next 5 days prices.
I am wondering what are the key differences between those 2 strategies to predict the next 5 days prices? What are the advantages and disadvantages of those 2 LSTM models?
Thank you,
Good question, the differences really depend on the choice of model and complexity of the dataset.
This post compares the different approaches:
https://machinelearningmastery.com/multi-step-time-series-forecasting/
Hey Jason,
Thanks for the blogs. They are really helpful and I have learned a lot from machinelearningmastery.
This blog about LSTM is very informative, but I have a question
I have a set of amplitude scans, and I want to predict next scan (many to one problem). So my data is of (6,590) and the result should be (1,590). 590 are the amplitude values in the scan.
A. Is it possible to address this problem with LSTM and
B. Even if possible how much accurate do you think the system might perform given the number of time steps and features it is predicting.
Thanks
You’re welcome.
Try it and see. This framework will help:
https://machinelearningmastery.com/how-to-develop-a-skilful-time-series-forecasting-model/
annemarieke-de.haan@unilever.com
Thanks Jason for this good tutorial. I have a question. When we have two different time series, 1 and 2. Time series 1 will influence time series 2 and our goal is to predict the future value of time series 2. How can we use LSTM for this case?
I call this a dependent time series problem. I given an example of how to model it on this post:
https://machinelearningmastery.com/how-to-develop-lstm-models-for-time-series-forecasting/
The link is the link of the current page, Do you mean that?
Yes, I give an example above.
Thanks Jason for this good tutorial, I have read your tutorial for a long time , I have a question. How to use LSTM model forecasting Multi-Site Multivariate Time Series, such as EMC Data Science Global Hackathon dataset, thank you very much!
I have advice for multi-site forecasting here:
https://machinelearningmastery.com/faq/single-faq/how-to-develop-forecast-models-for-multiple-sites
Thank you for sharing. I found that the results of time series prediction using LSTM are similar to the results of one step behind the original sequence. What do you think?
Sounds like the model has learned a persistance model and may not be skillful.
I have some question?
If I have model from LSTM,I want to know percent of accurate of new prediction.
How to know percent accurate for new forcast?
Thank you
If your model is predicting a class label, you can specify the accuracy measure as a metric in the call to compile() then use the evaluate() model to calculate the accuracy.
You can learn how for an MLP in this post which will be the same for an LSTM:
https://machinelearningmastery.com/tutorial-first-neural-network-python-keras/
Thanks a lot! I have read your websites for a long time!
I have a question, in “Time Series Prediction with LSTM Recurrent Neural Networks in Python with Keras” you said that:
“LSTMs are sensitive to the scale of the input data, specifically when the sigmoid (default) or tanh activation functions are used. It can be a good practice to rescale the data to the range of 0-to-1, also called normalizing. ”
So why don’t you normalize input here?
Because you used relu? Because the data is increasing (so we can’t normalize the future input)? Or because you just give us an example?
Do you suggest normalizing here?
It would be a good idea to prepare the data with normalization or similar here.
I chose not to because it seems to confuse more readers than it helps. Also, choice of relu does make the model a lot more robust to unscaled data.
Thanks for a great article. Minor typo or confusion:
For the Multiple input case in Multivariate series, if we use three time steps and
10,15
20,25
30,35
as our inputs, shouldn’t the output (predicted val used for training) be
85
instead of 65?
In the chosen framing of the problem, we want to predict the output at t not t+1, given inputs up to and including t.
You can choose to frame the problem differently if you like. It is arbitrary.
You can also reference ‘Multiple Parallel …’
So you can find the differences in function ‘split_sequences’
if you want to predict 85, you can change the code to:
if end_ix > len(sequences)-1:
break
seq_x, seq_y = sequences[i:end_ix, :-1], sequences[end_ix, -1]
Notice ‘len(sequences)-1’, and ‘sequences[end_ix, -1]’
Thanks sooooo much Jason.
It helped me a lot.
I’m happy to hear that.
Hi Jason,
Thanks for this nice blog! I am new to LSTM in time-series, and I need your help.
Most info on internet is for a single time series and for next-step forecasting. I want to produce 6 months ahead forecast using previous 15 months for 100 different time series, each of length 54 months.
So, there is 34 windows for each time-series if we use sliding windows. So, my initial X_train has a shape of (3400,15). Then. I am reshaping my X_train [samples, timesteps, features] as follows: (3400, 15, 1). Is this reshaping correct? In genera, how can we choose “timesteps” and “features” arguments in this multi-input multi-step forecast?
Also, how can I choose “batch_size” and “units”? Since I want 6 months ahead forecast, my output should be a matrix with dimensions (100,6). I chose units=6, and batch_size=1. Are these numbers correct?
Thanks for your help!
Looks good.
Time steps is really problem specific – e.g. how much history do you need to make a prediction. Perhaps test with your data.
Batch size and units – again, depends on your problem. Test. 6 units is too few. Start with 100, try 500, 1000, etc. Batch size of 1 seems small, perhaps also try 32, 64, etc.
Let me know how you go.
Hi Jason,
Thanks for your response.
I don’t understand “6 units is too few”. In documentation of lstm functions in R, units is defined as “dimensionality of the output space”. Since I need an output with 6 columns (6 months forecast), I define units=6. Any other number does not produce the output I want. Is there anything wrong in my interpretation?
I recommend using a Dense layer as the output rather than the outputting from the LSTM directly.
Then dramatically increase the capacity of the model by increasing the number of LSTM units.
Hii Jason that’s great tutorial. I have time series data of the size 2245 where timings of bus from starting station to destination station. I want to find the pattern is it possible through LSTM WITHOUT THE CATEGORICAL RESPONSES.
Perhaps start here:
https://machinelearningmastery.com/start-here/#deep_learning_time_series
Bidirectional LSTM works better than LSTM. Can you please explain the working of bidirectional LSTM. Since we do not know future values. How do we do prediction?
It has two LSTM layers, one that processes the sequences forwards, and one that processes it backwards.
You can learn more here:
https://machinelearningmastery.com/develop-bidirectional-lstm-sequence-classification-python-keras/
In the last encoder-decoder model, if I have different features of input and output, is it correct that I change the code like this?
model = Sequential()
model.add(LSTM(200, activation=’relu’, input_shape=(n_steps_in, n_features_in)))
model.add(RepeatVector(n_steps_out))
model.add(LSTM(200, activation=’relu’, return_sequences=True))
model.add(TimeDistributed(Dense(n_features_out)))
model.compile(optimizer=’adam’, loss=’mse’)
I’m sure I understand, what do you mean exactly?
I am sorry for not expressing my question clearly.
In the last part of your tutorial, you gave an example like this:
[[10 15 25]
[20 25 45]
[30 35 65]]
[[ 40 45 85]
[ 50 55 105]]
Then, you introduced the Encoder-Decoder LSTM to model this problem.
If I want to use the last three time steps from each of the three time series as input to the model and predict the next two time steps of the third time series as output. Namely, my input and output elements are like the following. The shapes of input and output are (5, 3, 3) and (5, 2, 1) respectively.
[[10 15 25]
[20 25 45]
[30 35 65]]
[[85]
[105]]
When I define the Encoder-Decoder LSTM model, the code will be like this:
model = Sequential()
model.add(LSTM(200, activation=’relu’, input_shape=(3,3)))
model.add(RepeatVector(2))
model.add(LSTM(200, activation=’relu’, return_sequences=True))
model.add(TimeDistributed(Dense(1)))
model.compile(optimizer=’adam’, loss=’mse’)
Is it correct?
Thank you very much!
It looks correct, but I don’t have the capacity to test the code to be sure.
Thank you!
I test the code, and I want to show you what I got.
I assume the input sequence:
in_seq1 = np.arange(10,1000,10)
in_seq2 = np.arange(15,1005,10)
Define the prediction input:
x_input = np.array([[960, 965, 1925], [970, 975, 1945], [980, 985, 1965]])
I expect the output values would be as follows:
[ [1985] [2005] ]
And the model forecasts: [ [1997.1425] [2026.6136] ]
I think this means that the model can work.
Nice work! Now you can start tuning the model to lift skill.
how we can test these examples if have big excel data set?and its time series data, kindly refer to a link?
Save as a CSV file then use code in this post to prepare it for modeling:
https://machinelearningmastery.com/convert-time-series-supervised-learning-problem-python/
Can Multivariate time series apply to cnn-lstm model?
Yes, I have a good beginner example here:
https://machinelearningmastery.com/how-to-develop-lstm-models-for-time-series-forecasting/
I want to predict visibility on one airport for the next 120 hours.
I already build a LSTM to predict the visibility for the next hour, solely based on visibility observation. (Basically, the network learned that persistance is a good algorithm.)
My next step is to include a weather model forecast of say humidity as input.
I have then as input:
visibility observation on the airport (past and present)
prediction of humidity for the next 120 hours.
I have trouble to combine these two information.
Do you have suggestions?
What trouble are you having exactly?
let’s say:
Input : last 120 h of measured visibility
weather forcast for the next 120 h
Output: visibility prediction for the next 120 h
Implementation:
make visibility prediction every hour for the next 120 h
I have trouble to see how the LSTM will update its state every hour, since it will only get as new information a measured visibility for the last hour, and not about the full 120 h prediction.
I must say that I’m a newbie in ML.
The model is only aware of the data that you provide it.
Thanks a lot for your post. Your work is a great resource on forecasts with lstm!
Assume, I have dependent time series (heating costs and temperature) and I want to predict the dependent (heating costs), how could I implement temperature predictions (from other weather forecasts) into my model for heating cost predictions?
Do you know of any common approaches to this? Or any papers on how to handle external forecasts for independent variables?
I recommend this process generally:
https://machinelearningmastery.com/how-to-develop-a-skilful-time-series-forecasting-model/
Hi Jason,
I think I saw you mentioning the activation function ‘relu’ usually works better than ‘tanh’ in LSTM model. But, I forget I saw this in which post. I don’t find any post from your blog that focuses on how to choose the activation function. So, I submit this question under this post and hope you don’t mind.
Is it true that ‘relu’ often works better than ‘tanh’ in your experience? If you have any post talking about activation function, please give me the title or URL.
Thank you very much!
It really depends on the dataset, I have found LSTMs with relu more robust on some problems.
Thank you! So, the way I can make sure which activation function is the best for my dataset is to enumerate and see the results?
Yes. It will almost certainly be relu or tanh.
This is awesome for someone starting out with LSTM.
All the content on your site is amazing, I really appreciate it. Thank you.
Thanks!
Hi Jason,
Still lovin’ your work!
1 question: can you please explain the purpose of the out_seq series in the Multiple Parallel Series example?
Many thanks,
Andrew
It is the output sequence, dependent upon the input sequences.
Correct me if I’m wrong, but isn’t the prediction the output? I mean, besides the way you obtained the out_seq sequence in the first place, it’s no different than in_seq1 or in_seq2. It could even be considered an engineered feature that expands the data.
Prediction is the output of the model.
Perhaps I don’t follow your question?
another great article, Jason! I’m trying to get started on a project that is similar to the LSTM model described in this article: https://medium.com/bcggamma/using-deep-learning-to-predict-not-just-what-but-when-fae6515acb1b
I’d greatly appreciate your input on how to develop an LSTM model that can predict ‘what’ a consumer may buy and ‘when’ they will buy it;
Based on your article, it looks like the right model to choose would be Multiple Parallel Input and Multi-Step Output. Would you agree or do you think i should choose a different model? Any pointers or links to relevant articles would help!
Thanks,
I’d encourage you to prototype and explore a suite of different framings of the problem in order to discover what works best for your specific dataset.
I have used your code to get started, at the last step I am getting a below error-
NameError: name ‘to_list’ is not defined
Could you please help, I am not sure what am i missing here.
Thanks for your help
Ensure you have copied all of the code from the tutorial, I have more suggestions here:
https://machinelearningmastery.com/faq/single-faq/why-does-the-code-in-the-tutorial-not-work-for-me
Hi Jason,
Thanks for taking time out, I have copied your code line by line and checked couple of times as well. Example is from Vanila LSTM.
Checks done-
I was getting some error, then I followed stack overflow and downgraded my keras to Version: 2.1.5
I searched stack overflow and related questions and even posted my questions there.
Your help is appreciated.
I recommend using the latest version of Keras and TensorFlow.
Please, have you an example of LSTM encoder-decoder with the train / test-evaluation partitions.
I tried but it does not work like this:
# split into samples
trainX, trainy = split_sequence(train, n_steps_in, n_steps_out)
testX, testy = split_sequence(test, n_steps_in, n_steps_out)
# reshape
trainX = trainX.reshape((trainX.shape[0], trainX.shape[1], n_features))
testX = testX.reshape((testX.shape[0], testX.shape[1], n_features))
….
# fit model
model.fit(trainX, trainy, epochs=5, verbose=2)
# make predictions
trainPredict = model.predict(trainX)
testPredict = model.predict(testX)
# calculate root mean squared error
trainScore = math.sqrt(mean_squared_error(trainY[0], trainPredict[:,0]))
print(‘Train Score: %.2f RMSE’ % (trainScore))
testScore = math.sqrt(mean_squared_error(testY[0], testPredict[:,0]))
print(‘Test Score: %.2f RMSE’ % (testScore))
thank you very much
I may, you can use the search box to look at all tutorials that use the encoder-decoder pattern.
Hi Jason,
Thanks for this tutorial. I am quite new to the time series forecasting with LSTM. I have a question about the part “Multiple Parallel Input and Multi-Step Output”. The output data shape is (5,2,3). I mean the each instance on the output is not just a sequence, It is a sequence of sequence. And you have show the example there with Encoder and Decoder. I just want to implement one of the methods of Stacked or Bidirectional LSTM. But I am not sure which number I should put the Dense layer. For example, in the previous examples, the output shape is like (6,2) and It is obvious we should put 2 for the Dense layer. But I can not figure out the right thing for the Stacked LSTM. Do you have any example tutorial for this?
Kind Regards,
Gunay
With multi-step output, the number of nodes in the output layer must match the number of output time steps.
With multivariate multi-step, a vanilla or bidirectional LSTM is not suited. You could force it, but you will need n x m nodes in the output for n time steps for m time series. The time steps of each series would be flattened in this structure. You must interpret each of the outputs as a specific time step for a specific series consistently during training and prediction.
I don’t have an example, it is not an ideal approach.
Thank you!
Is there any alternative structure for this kind of problems except Encoder-Decoder?
Yes, the one I described. There may be others, it is good to brainstorm and prototype approaches.
Thanks for your great tutorial. I just wonder should we avoid using bidirectional LSTM for time series data? Does it mean we use future data to train the past model parameters?
No, it means the model will process the input sequence forwards and backwards at the same time.
Hi Jason,
I faced one problem and just interesting maybe you did it before. I have the forecasting problem as like Multiple Input Multi-Step Output but a little bit different. Let’s just assume, my input(which are features dataset) and output (target we want to forecast) datasets have historic data. And I should forecast one week ahead for the target. But I have also the one week ahead forecasted input dataset(which is forecasted by another system). I should use both the historic input and one week ahead forecasted input to forecast one week ahead output. But I do not know how I should use that one week ahead forecasted input data during the learning process. Can you give me any hint?
Perhaps use a model with two heads, one for the historical data and one for the other forecast?
The functional API will help you to design a model of this type:
https://machinelearningmastery.com/keras-functional-api-deep-learning/
What if we want to predict anything for the next 20 upcoming days! Here sequentially we have to predict for 20 days. How can we apply LSTM here?
Yes, although the further you predict into the future, the more error the model will make.
This is called multi-step forecasting, there are many examples, perhaps start here:
https://machinelearningmastery.com/start-here/#deep_learning_time_series
HI Jason, thanks for all the tutorials. They are really helpful. I am looking to try and implement an LSTM that returns a sequence, and had read this tutorial – https://machinelearningmastery.com/multi-step-time-series-forecasting-long-short-term-memory-networks-python/
One thing I am having trouble understanding is how to really shape the input data and get a sequence output using Tensorflow / Keras. I am looking to predict the sequence T – T+12 hours using T-1 – T-48 hours. So predicting the next 12 hours from the last 48 hours in 1 hour increments. Each hour of data has a dozen or so features for that time step. From what I have read of yours so far it seems as if each of the 48 previous time steps should be considered features of the time step T to predict a sequence for the next 12 hours. And so basically, from what I gather, I would end up with the input for Timestep T having 576 columns (48 time steps, each with 12 features) – I mean does that seem right? I am also a bit unsure of what particular model I should use… is it going to be a multi-step, multi-input network… just a bit confused on the jargon as well and maybe thats why I’m having trouble figuring out what I need to do.
Looking at some of your books too, but not sure what might be the right one to help guide me through a problem like this.
Thanks,
Aaron
Perhaps this will help:
https://machinelearningmastery.com/faq/single-faq/what-is-the-difference-between-samples-timesteps-and-features-for-lstm-input
Thanks! That definitely makes sense now from the input shape standpoint. If I have 20 samples with 48 timesteps and 12 features the input shape would be [20, 48, 12]
For the output however, looking through the Keras docs https://keras.io/layers/recurrent/, I am trying to get a return sequence. Would I be using a 3D tensor? (batch_size, timesteps, units) where it would look like (20, 12, 1)? Since I am trying to find 1 value at each of the 12 time steps for the sample size of 20
Thanks again!
Aaron
I don’t recommend returning a sequence from the LSTM itself, instead use an encoder-decoder model:
https://machinelearningmastery.com/start-here/#lstm
Why don’t you recommend returning a sequence from the LSTM? If I was using the below encoder-decoder model from another one of your posts, what would the output of the first LSTM be?
model = Sequential()
model.add(LSTM(…, input_shape=(…)))
model.add(RepeatVector(…))
model.add(LSTM(…, return_sequences=True))
model.add(TimeDistributed(Dense(…)))
Generally the output sequence from an LSTM is the activation of the nodes from each step in the input sequence. It is unlikely to capture anything meaningful.
It is better to interpret these activations or the final activations with more LSTM or Dense layers, and the output a sequence of the same or different lengths using a separate model.
Hi there,
I love this tutorial, all of your tutorials actually but this one I have found the most helpful. Questions about the MIMO LSTM output shape has come up a few times, and I am also having trouble with it.
I am trying to use a Dense layer as my final layer as you suggest, passing it n_steps_out as an argument. I am predicting 3 variables and n_steps_out is 10.
Keras complains that it is expecting the dense layer to have 2 dimensions, but I am passing it an array with shape (n_samples,n_steps_out,n_features)
Can you help me make sense of this?
Thank you
I would recommend a model with a time distributed wrapper or decoder for multivariate multi-step output, so you can output one vector for each time step.
Hi Jason,
I have a question: are LSTM suitable for predicting based on a test set with the same nature of inputs as of train set ? Like in other cases of prediction where you will be having input signals in train set, that the model will work on. plus the memory based on the fact that entries are ordered.
I trained an LSTM on a CNN model acting on ordered images, to predict a timeserie. on test set I have the following ordered set of images by time. I guess there is no concept of horizon here, how should I improve my model, and what starting point in predicting test set in this case?
Many thanks.
I would recommend modeling the raw time series directly, instead of images of the time series.
Hello Jason,
Many thanks for the helpful article..
I have tried to copy the code “Multiple Parallel Input and Multi-Step Output” and run it exactly the same without any changing but I got a different results than the one you got.
[ [
[147.56306 167.8626 312.92883]
[185.38152 205.36024 385.96536] ] ]
Is there any reason for that?
Best regards,
Tayson
Yes, this is due to the stochastic nature of the learning algorithm, more here:
https://machinelearningmastery.com/faq/single-faq/why-do-i-get-different-results-each-time-i-run-the-code
Hi Jason,
How would you handle building the LSTM model for time series data with irregular time intervals (e.g. Jan 1, Jan 2, Jan 4, Jan 7, Jan 13, Jan 14, etc…)?
It appears this model presupposes a regular time-interval spacing.
You could fill the “missing” days with zeros or impute them with, say, the mean of the last 3 values, but I would like to know how to make the LSTM model without filling/imputing the time series data. How would you handle this?
Thanks, and great lesson.
Yes, I would try many approaches and compare results, such as:
– model as is
– normalize interval with padding
– upsample/downsample to new intervals
– etc.
Follow-up to this question
Holding number of features constant
Are the various combination of models above able to cope when the number of time-steps per each Sample is variable?
Or do the underlying model assumptions break in some way?
Yes, you can either pad all samples to the same length or use a dynamic RNN. Assumptions of the model hold for both cases.
If we are forecasting in monthly buckets and using 5 years of data, how do we know how many months of data to have on each row?
Perhaps perform a sensitivity analysis of the model to see how history impacts model performance.
There will be a sweet spot for a given dataset.
Thanks Jason! If the history has distinct patterns for each quarter, should we have 3 months in each row? How would the results differ when we keep 12 months on each row versus 3 months on each row versus 1 month on each row?
Depends on the dataset, I recommend testing to discover the specific answers with your data and model.
Hi Jason,
I am trying to predict high and low value of a time series in next X days, my output layer in RNN is :
model.add(Dense(2, activation=’linear’))
so basically output vector is [y_high, y_low], the model works pretty well however it sometimes outputs y_low > y_high, which of course doesn’t make any sense, is there a way to enforce model so that condition y_high >= y_low is always met.
Interesting, perhaps you simplify the problem and predict a value in a discrete ordinal interval, e.g. each category is a blocks of values?
I was trying to modify loss function but I am unable to access y_pred individual members, I don’t even know whether it’s ultimately possible.
I don’t follow, why not? What is the error?
Hi Jason, a colleague and I are thinking of trying an LSTM model for time series forecasting. We are faced with over a thousand potential predictors, and would like to select only a smaller number for the final model. In particular, I have recently become fascinated by SHAP values; e.g., see this informal blog post by Scott Lundberg himself, in the context of XGBoost.
https://towardsdatascience.com/interpretable-machine-learning-with-xgboost-9ec80d148d27
Tantalizingly, Scott L. demonstrates SHAP values in the context of an LSTM model here:
https://slundberg.github.io/shap/notebooks/deep_explainer/Keras%20LSTM%20for%20IMDB%20Sentiment%20Classification.html
But that is using text input (sentiment classification in the IMDB data set), which involves an Embedding layer just before the LSTM layer. For a non-text problem like time series forecasting, we would exclude the Embedding layer. But doing so breaks the code.
Do you have any suggestions how SHAP values might be used in the context of LSTMs for time series forecasting (not text processing)? If not, do you have any suggestions for feature selection in that context?
Thanks!
I don’t know what SHAP is, sorry.
Hi, Joe. I am running into the exact same topic. Have you found a way to implement SHAP to multivariate timeseries forecasting?
Hi Jason,
Thanks for this useful tutorial.
I am confused to inverse scaling of my data after splitting it into the form:
x(data_length, n_step, feature)
Because the scaler only can be used in 2D condition.
What I want to do is evaluate rmse between prediction and true values, so I have to
inverse transform data. Could you please tell me how to deal with this problem?
Yes, I show how here:
https://machinelearningmastery.com/machine-learning-data-transforms-for-time-series-forecasting/
Hi Jason,
Firstly, I must say you have a fabulous chunk of articles on ML/DL. Thanks for helping out the community at large.
Coming to LSTMs, I am stuck in one problem from last few days. Here is how it goes –
I have 3 columns namely customer id and basket_index and timestamp. For every customer, each row represents one time stamp. Lets say there are 3 customers with variable time stamps. First one is having 30 time stamps, 2nd is having 25 and 3rd is having 50. So, the total number of rows are 105. Now for the column basket index, each row signifies a list of product keys bought by any customer on a particular timestamp. Here is the snapshot of the dataset –
CustomerID basket_index timestamp predicted_basket
111 [1,2,3] 1 [4,5]
111 [4,5] 2 [9,7]
111 [9,7] 3 [3,5,6,1]
.
.
222 [6,2,3] 1 [1,0,2,5]
222 [1,0,2,5] 2 [7,5]
.
.
333
.
. and so on..
Now, since every customer has a different time series,
1) How to pass everything into one network?
2) Do I have to build multiple LSTM models (one for each customer) in this case?
3) Also, I am creating an embedding layer for both customer and product keys (taking mean for every basket). How to specify how many steps back does every time series look in such cases?
4) How should I specify batch size in this case?
Your help will be really appreciated. Thanks!
Great question, I have some suggestions here that might help:
https://machinelearningmastery.com/faq/single-faq/how-to-develop-forecast-models-for-multiple-sites
Generally, I would encourage you to try to learn across customers.
Thanks Jason for nice post.
One question hopes to get your guide: For a LSTM work, we can’t stop on say the model is good but most important is how to use the good model outcome.
For example flu or not for patients. Now I want to predict the flu for future half year (Jun-2019 to Dec-2019) but what I have is history data (I have past 4 years those people’s flu data and target on that model is half year from 6-1-2018 to 12-31-2018).
How can I apply history LSTM outcome to predict future?
Can I get a list of important features from the history model with some value(like a weight) and apply this to my future data?
Or can i get the list of important feature from a good fit LSTM model and those features are important than other features?
Appreciate your guide!
This is a common question that I answer here:
https://machinelearningmastery.com/make-predictions-long-short-term-memory-models-keras/
Hi Jason,
Amazing work! Thanks sharing us your knowledge, this tutorial was so helpfull.
I’m new in ML/DL, i’m trying to predict sales in a company for future six months using LSTM. But i have an issue, i’m not sure about how to get more than 1 next step from your code using just one x vector by input. I’m using a monthly time step
Could you help me to understand a little bit better how to get it?
There are many examples of multi-step forecasting that you can use, including in the above tutorial.
There are also more examples here:
https://machinelearningmastery.com/start-here/#deep_learning_time_series
Dear Sir,
Thanks for your sharing example. I have collected traffic information like (Road property, weather, datetime,adjacent road speed, target road speed and more) for predicting road speed. Currently, I have prepared my code using Vanilla LSTM model for one step as well as multi-step-ahead prediction. Can you suggest me for which below model will be best for road speed prediction with higher accuracy?
Models are:
Data Preparation
Vanilla LSTM
Stacked LSTM
Bidirectional LSTM
CNN LSTM
ConvLSTM
I am waiting for your response.
Thanks,
Azad
I recommend testing a suite of model types and model configurations in order to discover what works best for your specific dataset, you can learn more here:
https://machinelearningmastery.com/how-to-develop-a-skilful-time-series-forecasting-model/
hi Jason, im using vanilla LSTM for forecasting,and i want to forecast 10 days ahead using this code
# Forecat real future
# Number of desired forecast in the future
L=10
#creat inputs and output empty matrices for future forecasting
Future_input=np.zeros((L,3))
Future=np.zeros((L,1))
#add last 3 forecast as input for forecasting next day (tommorow)
Future_input[0,:]=[predict[-3],predict[-2],predict[-1]]
#create 3 dimension input for LSTM inputs
Future_input= np.reshape(Future_input,(Future_input.shape[0],1,Future_input.shape[1]))
#predict tommorrow value
Future[0,0]=model.predict(np.expand_dims(Future_input[0],axis=0))
#Loop to predict next 9 days values
for i in range (0,9):
Future_input[i+1,0,:]=np.roll(Future_input[i,0,:], -1, axis=0)
Future_input[i+1,0,2]=Future[i,0]
Future[i+1,0]=model.predict(np.expand_dims(Future_input[i],axis=0))
#print 10 day ahead values
print(Future)
can it be like that?
Sorry, I cannot debug your code.
If you need more help with multi-step forecasting, see this:
https://machinelearningmastery.com/faq/single-faq/how-do-you-use-lstms-for-multi-step-time-series-forecasting
Hi, do you have any tips for implementing univariate ConvLSTM for two-dimensional spatial-temporal data? I’m trying to input 10 time steps of 55 x 55 images for single-step time series forecasting.
The following error code appears:
“ValueError: Error when checking target: expected dense_10 to have 2 dimensions, but got array with shape (10, 55, 55)”
Sorry, I don’t have a tutorial on the topic.
Dear Sir,
i have sequence 1247 data and i want to forecast 30 next, so the data would be 1277.
i follow this tutorial, but it just can 1 or 2 forecast. and i follow this tutorial
https://machinelearningmastery.com/how-to-develop-lstm-models-for-multi-step-time-series-forecasting-of-household-power-consumption/
but i get little confusion. so you have any advise to me?
its stock price data actually.
Stock prices are not predictable:
https://machinelearningmastery.com/faq/single-faq/can-you-help-me-with-machine-learning-for-finance-or-the-stock-market
Amazing Tutorial, thank you.
I have a question, is there a model where the outputs can influence each other?
I.e. you have multiple sequences all which move independently but can influence the others?
Thank you
Thanks.
Yes, an encoder-decoder model that outputs a time step for each series in concert might be such an approach.
Awesome. Great Explanation as always. I have always got rather frustrated and confused over the shape of data going into Keras models. So I relied upon your tutorials to make it clear.
Anyway using your examples I have been able demonstrate use of LSTM in predicting simple 2-D ballistics prediction calculations. I have used your code to help me here.
https://github.com/JulesVerny/BallisticsRNNPredictions
Pygame is required to animate the simulations
Well done, your project is very cool!
Dear Prof,
Imagine I have raw text containing only words ‘N1,N2,N3,………….,N1000’ in a shuffled format , i.e, 1 million words, each of which can belong to any of these 1000 words.
I want to select the number of time steps =5, and predict the next word.
Eg: An input of [N1,N6,N5,N88,N32] would be followed by ‘N73′.
Now, assume that I have tokenized all the 1000 possible words into numbers.
This is a scenario with 1000 possible output classes.
So should I replace model.add(Dense(1)) with model.add(Dense(1000,activation=’softmax’)) ?
If not, what is the main change I need to make, as compared to your univariate stacked LSTM code ?
If the words are shuffled, then there would be no structure for a model to learn.
Dear Jason!
I’m trying to use stacked lstm for this problem – Multiple Parallel Input and Multi-Step Output.
However I’m not sure how the final Dense layer should look like. Could you give me some hints, please?
Perhaps start with the example in the above post and then add an additional LSTM layer?
Which example do you mean? I can’t find any example for Multiple parallel input and multi step output LSTM, which uses stacked LSTM layers instead of encoder decoder.
Yes, under the section “Multivariate Multi-Step LSTM Models”
Specifically the subsection “Multiple Parallel Input and Multi-Step Output”
The examples can be adapted to use any models you wish.
Thanks Jason for detailed explanation.
Could you please tell how can we add hyperparameters for tuning “Forget Gate”, Input Gate” and “Output Gate” in LSTM compile or fit methods or is it done internally and we can’t control these gates?
They are not tuned.
How to predict multiple such inputs, x_input = array([[70,75,145], [80,85,165], [90,95,185],…,[200,205,405]]),Expect the next output, [210,215,425],
See this input in the article,x_input = array([[70,75,145], [80,85,165], [90,95,185]]),Predict such results,[[101.76599 108.730484 206.63577 ]],But it doesn’t seem to matter why you need to enter such a sequence.in_seq1 = array([10, 20, 30, 40, 50, 60, 70, 80, 90]),in_seq2 = array([15, 25, 35, 45, 55, 65, 75, 85, 95])
thanks
I believe there is a few multi-time step models listed above that will provide a good starting point.
Hi Jason,
Thanks for the article.
I was working with your code and planning to implement in my work, but I have noticed a different behavior. If I compile and run the code different times, it gives different result each time although I didn’t change anything in your code. I have tried with your example data and run several times and each time I got different results. I tried with my own dataset and the result is the same.
Now I am confused to implement LSTM in my work.
Could you please clarify this behavior?
Yes, this is to be expected. You can learn more here:
https://machinelearningmastery.com/faq/single-faq/why-do-i-get-different-results-each-time-i-run-the-code
Hi jason,
Say we have 3 variates(X).. and 1 dependent (Y)
The relation of 2 variate in X is like for 3 lags and 1 variate is 30 lag.
What is your advice when we have to model in such case?
I have many examples, including a few above.
I also have a tutorial here that might help as a starting point:
https://machinelearningmastery.com/how-to-develop-lstm-models-for-multi-step-time-series-forecasting-of-household-power-consumption/
Hi Jason,
Thanks for the very informative tutorial. Can you please throw more light on how to come up with confidence intervals for the predicted value
Do you mean prediction intervals instead of confidence intervals?
Perhaps start here:
https://machinelearningmastery.com/prediction-intervals-for-machine-learning/
Hi Jason
Thanks for your helpful tutorial
Could you please tell how can we predict the futures that we don’t have its data available
for example, I finalized my LSTM model, how can I predict the values on 2050
Yes, I show how in this post:
https://machinelearningmastery.com/make-predictions-long-short-term-memory-models-keras/
Thanks for the article.However, I have a problem that every prediction results are different, such as Multiple Parallel Series,The first time is [[101.25582 106.49429 207.8928 ]],The second time it became [[101.82945 107.527626 209.8016 ]],Why is this?
thanks
This is a common question that I answer here:
https://machinelearningmastery.com/faq/single-faq/why-do-i-get-different-results-each-time-i-run-the-code
I recommend that you try to run the example a few times.
I want to restate my question…
Suppose we are trying to model a water bucket that was 1 open inlet at the top and 2 outlets at the side one near the top and one near the bottom.
this will mean that the outlet at the top can release when the water is really good..
the outlet near the bottom has release which is exponential function of water above it.
now say such systems are in paralell(one above another, say2) and series(say 2, the final outlet from each parallel series join at the final output.) (Total 4 buckets).
can this be modeled by LSTM?
I have done this analytically…results are ok ..
tyring to use lstm for this ,,,
Perhaps.
You can use this framework to explore different framings of your problem:
https://machinelearningmastery.com/how-to-define-your-machine-learning-problem/
Hello Jason,
to the step:
# define input sequence
in_seq1 = array([10, 20, 30, 40, 50, 60, 70, 80, 90])
in_seq2 = array([15, 25, 35, 45, 55, 65, 75, 85, 95])
I want to ask how I can load a fully column out of a dataset.
I don´t want to insert each value because I have more than 22 million rows. After that I want to split into sequences of 200-400 time steps.
To the step:
out_seq = array([in_seq1[i]+in_seq2[i] for i in range(len(in_seq1))])
I don´t have a right mathematical equation. I want to predict the output without any knowledge about the relationship between the input signals.
I hope you can help me.
Kind regards
Ali
I have many examples, perhaps start here:
https://machinelearningmastery.com/start-here/#deep_learning_time_series
Hi Jason,
Thanks for these explanations and sample codes!
I was interested in the example you have provided for multi-variate version of LSTM. You have provided an example of a simple addition case. How can this be extended to instances where there are multiple inputs, but an exact relation between the inputs are not known even though it is known that the inputs are correlated? Thanks much for your guidance!
The model will learn the relationship, addition was just for demonstration.
Thanks Jason! That’s perfect.
In that case, what should the statement “out_seq = array([in_seq1[i]+in_seq2[i] for i in range(len(in_seq1))])” be replaced by, since we don’t know the exact relation between the variables? Thanks again!
Observations across multiple input time series are aligned to time steps based on their time of observation.
Perhaps this will make things clearer:
https://machinelearningmastery.com/faq/single-faq/what-is-the-difference-between-samples-timesteps-and-features-for-lstm-input
Thanks Jason. I shall read the content on that link.
Cheers,
Sree.
Hello, thank you again. I think my previous question could be made more clear.
I would like to use the vector output approach for a mimo lstm, making multi step predictions into the future similar to your encoder/decoder example.
I have tried using the split_sequences method from the encoder/decoder example with the vector output example and the dimensions dont work out. I end up with a value error
ValueError: Error when checking target: expected dense_2 to have 2 dimensions, but got array with shape (5, 2, 3)
I greatly appreciate your help, I have been struggling with this for a while. I would imagine the output should be a matrix (number of features X prediction horizon) so I think there is something conceptually I am not understanding.
Thank you, and thank you for all of the wonderful tutorials
Gideon
Perhaps start wit the code example you want to use and slowly change it for your needs.
If the data size does not match the models expectations, you will need to change the data shape or change the model’s expectations.
I will toil away some more, but I just want to be sure it is possible to use a dense layer/vector output approach for Multiple Parallel Input and Multi-Step Output LSTM in Keras.
Thanks again for your time.
Gideon
It is possible to use a Dense for multi-step multivariate output without a decoder or timedistributed wrapper layer, it is just ugly.
E.g. the output would be a vector with n x m nodes, where n is number of variates and m is the number of steps.
Ive figured it out, and its not too ugly and exactly what I needed. I was unaware of the Reshape layer in Keras.
from keras.layers import Reshape
…
model.add(Dense(n_steps_out*n_features))
model.add(Reshape((n_steps_out,n_features)))
Thank you again for your help. I am buying your book right now.
Cheers
Gideon
Nice work.
Hi Gideon,
I was struggling around something similar and applying your solution, solved all the matters! Do you have any more documentation on this?
Hello Jason,
Great article, very useful. I want to use LSTM to predict sun irradiance 12 hours ahead using 8 features (including sun irradiance) of the last 24 hours as inputs. Thus, it would be a multivariate multi-step LSTM where the output is a sequence of 12 timesteps. I have 8 years of data and I want to use first 6 for training and last 2 for testing. I have some questions:
1) Should I overlap the input sequences?
2) Should I use a vector output model or an encoder-decoder model?
I recommend testing both approaches and use data to make the decision, e.g. choose the model that gives the best result.
hai jason,
the article was very much helpful.
can you just tell me which approach should I take if I have two columns in my dataset .
one is time in ddmmyyyy format and the other is stock price.
I have the data for last 12 months.
I want to predict the stock price for 4 upcoming months.
how can I do the same.
one more doubt is that if the column for time is not actually having a same interval in between them, then is there anything more that I should do to or consider for predicting the 4 upcoming months stock price
You can drop the date if all observations have a consistent interval.
Stock prices are not predictable:
https://machinelearningmastery.com/faq/single-faq/can-you-help-me-with-machine-learning-for-finance-or-the-stock-market
Regardless, I would recommend this process:
https://machinelearningmastery.com/how-to-develop-a-skilful-time-series-forecasting-model/
So after dropping the dates column I guess I would have to go for a univariate multistep lstm model. right?
And what should be done if seasonality comes into picture?
and one more doubt is that when I am predicting the four upcoming months in a multistep , will the model consider the predicted value of 3rd month while predicting the fourth month or will the model consider the predicted value of 2rd month while predicting the third month and likewise ?
Try a suite of models, and compare to a linear model or naive model to confirm they have skill.
If you have seasonality, try modeling with and without the seasonality and compare performance.
Try multiple approaches for multi-step prediction, e.g. direct, recursive, etc:
https://machinelearningmastery.com/multi-step-time-series-forecasting/
If you have seasonality, try modeling with and without the seasonality and compare performance.
what does this mean? I didn’t get you
my training data will anyway contain the seasonality if my original dataset has seasonality right?
how will I be able to make a model without seasonality?
is there a additional parameter or feature in LSTM for seasonality?
You can remove seasonality from a dataset via seasonal differencing:
https://machinelearningmastery.com/remove-trends-seasonality-difference-transform-python/
In Multiple Input Series,
(7, 3, 2) (7,)
[[10 15]
[20 25]
[30 35]] 65
[[20 25]
[30 35]
[40 45]] 85
[[30 35]
[40 45]
[50 55]] 105
[[40 45]
[50 55]
[60 65]] 125
[[50 55]
[60 65]
[70 75]] 145
[[60 65]
[70 75]
[80 85]] 165
[[70 75]
[80 85]
[90 95]] 185
1. How many lstm block will be here in this example( x=7)
if batch size = 3,is the number of lstm block equal to the number of x in the batches?
or the number of timesteps?
2.are timesteps, neurons and batchsize all hyperparameter? how do we optimize them
No, the number of blocks in the first hidden layer is unrelated to the length of the input sequences.
See this:
https://machinelearningmastery.com/faq/single-faq/what-is-the-difference-between-samples-timesteps-and-features-for-lstm-input
thanks..
Then what is the total number of LSTM blocks?
for every epoch, are the weights reinitialized and states are reset?
The number of LSTM units is specified in each hidden LSTM layer.
LSTM states are reset at the end of every batch.
sorry but i dont get this?
In model.add(LSTM(50, activation=’relu’, input_shape=(n_steps, n_features)))
input_shape here is equal to an input to each LSTM node right?
and here 50 means,, h(hidden layer) is a vector of 50*1 right?
my question is the number of individual LSTM nodes(block) equal to number of samples in the a batch?
Yes, the shape defines the shape of each input sample (time steps and features).
Yes, 50 refers to units in the first hidden layer.
The number of units and sample shape are both unrelated to the batch size. Unless you are working with a stateful LSTM, in which case the input shape must also specify the batch size.
Does that help?
yeah.. one followup question
[10 15]
[20 25]
[30 35]] 65
here is it like many to one ?
this feeds as xt (single input) right?
in this case what is the size of weight ?
Yes, multivariate multistep input to one output.
how does this input concatenate with hidden layer … i cannot visualize this..
i was thinking the input were a vector[n*1]
Each node in the hidden layer gets the complete put sequence.
Thank you so much..
[10 15]
[20 25]
[30 35]] 65
so in this case ,,, what is the size of xt and weight matrix?
You can calculate it based on the number of nodes in your network.
Thank you jason.. you have so kind and helpful..
The number of cells is equal to the number of fixed time steps.
The blogs says so. I am very confused with number of cells and what controls it.
https://stackoverflow.com/questions/37901047/what-is-num-units-in-tensorflow-basiclstmcell#39440218
Sorry for trouble
Not in the Keras implementation.
Dear Jason,
Thank you for writing all these awesome tutorials!
My question:
As I understood it, a LSTM network learns the information in a time-series by backpropagation through a specific length (in time) at which the LSTM cells are unrolled during training.
So, while training it is necessary to define the number of timesteps provided in the training data. But shouldn’t it be possible to use the (trained) network with ANY number of input timesteps to make a prediction (because of the recurrent nature in which the LSTM cells work)?
Am I getting something wrong here from the beginning?
Thank you for hints on this
Philipp
Dear Jason,
I am currently working on a disease outbreak prediction model. I have 4 years of data with over 100 input variables and each year has got 365 data points. I would like to create a LSTM model that will be able to predict the future outbreak (whether thr will be an outbreak-1 or no outbreak-0) based on the given input variables. For example, given 7 days of data points, i would like to predict the occurance of outbreak (whether 0 or 1) on the 8th day.
However, i am not sure on which LSTM model will best fit my case. Will ‘multiple input multi-step output) be the best approach? Your guidance will be much appreciated.
Thank you
Perhaps you can model it as a time series classification problem.
The tutorials here will help you to get started:
https://machinelearningmastery.com/start-here/#deep_learning_time_series
Hi Jason,
Can you please provide some pointers that will help us in minimizing the step-loss during model fitting….
Thanks
Yes, here are some suggestions:
https://machinelearningmastery.com/start-here/#better
Dear Jason,
Thank you for your tutorials. They are really useful for us.
I’ve one question about LSTM. I have different time series more than one (for example 100). I need to train network with 100 different time series. and test 10 different time series. Which method should I use?
Thanks for your helps.
I recommend this process:
https://machinelearningmastery.com/how-to-develop-a-skilful-time-series-forecasting-model/
Hi Jason,
Thank you for sharing. I wonder if there is a way to set timestep > 1 without doing subsequence sampling as you did in data preparation, e.g. convert a 9-by-1 time series to a 6-by-3 data set. After the conversion, the 3-feature dataset is no more time dependent. You are able to use any kind of ML models (say OLS) to predict y. So why LSTM? Should LSTM be able to select (forget) previous information without this conversion?
LSTM does have the benefit that it can remember across samples.
This may or may not be useful, and is often not useful for simple autoregressions.
Thank you for your quick reply. In your example, if I do a subsequence sampling and convert
[10, 20, 30, 40, 50, 60, 70, 80, 90] to
[[10, 20, 30],
[40, 50, 60],
[70, 80, 90]] (no replacement between each subsequence)
and run LSTM(input_shape=(3,1)), is that the same as I run LSTM(batch_input_shape=(3,1,1), stateful=True) on the origin time series (9-by-1)?
No, as each “sample” will cause an output from the model, e.g. 1 sample with 3 time steps vs 3 samples with 1 time step.
More on samples vs timesteps here:
https://machinelearningmastery.com/faq/single-faq/what-is-the-difference-between-samples-timesteps-and-features-for-lstm-input
Thank you, Jason!
No problem.
For a classification LSTM, using a Seed I get the same classification matrix each time I run it. However, when I vary the batch size in model.predict, I get the following:
Prediction Batch Sizes:
32 = Different Classification Matrix on each repeat
Batch size in predictions is merely for ram managment. Correct? If yes, what do you think Dr. Jason would cause these irregularities ?
No batch size impacts the learning algorithm:
https://machinelearningmastery.com/how-to-control-the-speed-and-stability-of-training-neural-networks-with-gradient-descent-batch-size/
Hi Jason,
Sorry I didn’t explain my concern well. I was referring to the Batch Size parameter that we mention in “model.predict i.e. predicting” and not while training. I agree that batch size during training will have an impact. During prediction, the default size is 32 as defined by keras but when I change that to anything but 32 I get a different classification matrix even though I use a seed. When I leave the batch size as default, my seed is able to produce the same results.
Recall that with the LSTM, the state is reset at the end of each batch. This explains why you are getting different results for the same model with different inference batch sizes.
Hi Jason,
Thanks for the tutorial.
I’d like to apply this example to a real case.
I have to forecast how much money will be withdrawn every day from a group of ATMs.
Currently I am using a time series for every ATM. (100 ATMs = 100 time series).
Wich method do you think could be better from this tutorial ?
I need to use historical information and external information such as holidays, day of week, etc.
Thanks in advance.
I recommend following this framework:
https://machinelearningmastery.com/how-to-develop-a-skilful-time-series-forecasting-model/
Hi Jason, I want to use some kind of machine learning method to demonstrate that there is a relationship between the score gap of two basketball teams and the demand for a taxi outside the stadium.
I have time series of pick-ups near a stadium. I have the score gap time series between two basketball teams.
What I want to achieve is that training a machine learning model that could tell me, based on the taxi pick-ups at time t, what is the taxi pick-ups at time t+1.
I also want to see if I also have the score gap at time t, can I improve my prediction accuracy of pick-ups at time t+1.
Which machine learning model should I use?
thank you so much!
Why not just measure statistical correlation between the observations?
https://machinelearningmastery.com/how-to-use-correlation-to-understand-the-relationship-between-variables/
Thank you very much for your reply!
Yes, this could work for finding a relationship.
but what if I want to forecast the number of pick-ups at t+1. Can LSTM or ARIMA do this job?
Yes, but perhaps test a suite of methods and discover what works best for your specific dataset.
Hi Jason,
Thanks for the tutorial.
Suppose I have several time series showing cumulative bookings for different trains last year. I don’t want to forecast but just classify those time series to see if some of them have similar patterns. Can I include all those series into one LSTM model? Is there any risks when doing so?
Thanks in advance.
Sure, it means you are learning/modeling across books. Sounds reasonable.
Thanks Jason!
So is it the same as multivariate LSTM? Sorry I’m new to modelling so still find things confusing
Probably not, each example is a separate sample or input-output pair for the model to learn from.
Hi Jason,
thanks for the nice tutorial!
I have a dataset with 3000 univariate timeseries (i.e. 3000 samples) and each sample has 4000 timesteps. When i use [samples, time steps, features]=[3000, 4000, 1] the code is extremely slow and with bad performance.
On the other hand, if instead [3000, 4000, 1] i write [3000, 1, 4000] the code is very fast and with great performance.
But is the reshape [3000, 1, 4000] correct? I mean according to the rule [samples, time steps, features] and given the fact that each of my samples have 4000 timesteps and for each time step there is one feature the correct should be [3000, 4000, 1].
So is [3000, 1, 4000] correct? And if it is not (logically it is not) why it works much better than [3000, 4000, 1] ?
Thanks in advance
I would recommend not using more than 200 to 400 time steps per sample. Perhaps you can truncate your data?
I did also an experiment and i truncated my data and used as input [samples, time steps, features]=[3000, 400, 1]. It was quicker but i got a mean accuracy 42% (in 10 random splits).
As i told you in my previous post when i exchange timesteps with features namely when i use [3000, 1, 4000] i get an accuracy 90%.
But giving 1 timestep means that i don’t exploit the memory, whis is the characteristic of lstm?
I am confused as to whether i should use [3000, 1, 4000], which is very quick and gives very good results but maybe it is not very correct? Or it is correct as if i used [3000, 400, 1](if i truncated my data to 400)
The state of the LSTM is reset at the end of each batch by default, so you can get some across-sample memory.
I recommend testing a suite of different configurations to see what works well or best for your specific dataset. I cannot know what will work well, you must discover the answer.
Hello Jason,
I am quite new to ML and LSTMs. I have a scenario where I intend to train a model using my hourly sensor values. For eg
12-1-2019 12:00:00 12
12-1-2019 13:00:00 16
…
12-5-2019 12:00:00 14
Once I am done with my training I intend to predict values every hour and compare the values with live sensor values….I am planning to use LSTM and which approach do you recommend me ?
I recommend this framework:
https://machinelearningmastery.com/how-to-develop-a-skilful-time-series-forecasting-model/
Jason, this is very useful. Im try to to do some prediction around IT incidents. based on historic data i want to predict what type incident i can expect next month/week/day. do you have anything similar done if so request to share pls
Perhaps you can model it as a time series classification, e.g. what event is predicted in this interval.
The tutorials here might help as a starting point:
https://machinelearningmastery.com/start-here/#deep_learning_time_series
Hi Jason,
Thanks for answering my question in your other tutorials. I have a minor doubt suppose my data has a continuous time series(non stationary) & other categorical variables (which are already encoded). Under that circumstance what is the best way to difference the data ? Because categorical data are not differenced but they have to be used while training the model.
The function written above differences all the variables irrespective of whether they are continuous or categorical. It would be great if you can help.
Difference the real-values only, and only if they are non-stationary.
Hi Jason,
I copied and pasted your first example from Multi-Step LSTM Models, the one with the vector output of two values and the input being one.
You report as an output the values:
input [[70 80 90]]
output [[100.98096 113.28924]]
but with those parameters I cannot get any closer than
input [[70 80 90]]
output [[122.678955 139.9465 ]]
This you use the parameters you report? Is this so dependant on architecture?
Results are dependent upon the model, the model configuration and the data, the performance is also stochastic, subject to random variance.
Hi, thanks for your reply.
I understand that, that’s why I am asking,
I have same model, same model config. same data, and the stochasticity should be symmetrically distributed (?). Then I assume that the results you report are not from the parameters you have in the code examples.
My data is non stationary & there are seasonality every 7 days ( as evident from the ADF tests & ETS plots) & a first order differencing makes it stationary.
I totally get that I have to difference only the real values & that is what I have been aiming to do . But the reason I asked this question because the moment I difference the real values its get shifted by one place so if the original data has 100 observations the differenced data will have 99 observations (with a first order differencing). But the categorical data which cannot be differenced remains to be the same 100. How do I deal with this ?
You discard the first observation and the difference value corresponds to the categorical value at the same time step.
I think I have been able to solve the issue thanks Jason for addressing my query
I’m happy to hear that.
in the Vector Output Model section,
I copied your code and tried, the actual answer is not correct as of the expected [100, 110], they are actually [110, 120].
Perhaps try running the example a few times? It can very given the stochastic nature of the learning algorithm.
never get any chance to around [100, 110]. I ran many times, the output is always around [110, 120] with some variations.
no kidding 🙂 you can try that part of codes. The output looks ridiculous.
Intersting.
Is Keras/TensorFlow/Python up to date?
Hi Jason, I am doing an electrical demand forecast and am trying to build a model which predicts the demand for the following 24 hours given the last 90 hours. I have implemented two types: a 24 step prediction and a recursively defined prediction, which predicts the next hour and then uses the previous 89 true values and the new predicted value to predict the next value, and so on. I am wondering which method you believe to be the best(if either) and any tips for improving my model as depending on the time of year the forecast can vary massively with accuracy. I currently have an LSTM(50) connected to a Dense(20) connected to an output Dense(1) for both cases.
Any help would be greatly appreciated. Thank you. Matthew
Well done, very cool!
I recommend testing each method and use the one with the lowest error.
Also, get creative and test a suite of other configurations. Ensure your test harness is robust and reliable so that you can trust the decisions you make.
Hello, this example was nice to follow and seemed little more simpler than other LSTM examples because of no pre-processinhg transformations (normalization, standardization, making data into stationary, etc.). However, should I perform these pre-processing transformations in general for time series prediction? Should I do such thing for this kind of examples too even though the dataset is simple?
Yes, test to see if the data preparation improves model performance.
I keep it out of examples for brevity.
#In Multiple Parallel Series
I have defined the input like this
# define input sequence
in_seq1 = array([10, 20, 30, 40, 50, 60, 70, 80, 90])
in_seq2 = array([15, 25, 35, 45, 55, 65, 75, 85, 95])
x_input = array([[70,75,1,4], [80,85,165,5], [90,95,185,6]])
n_steps=4
n_features=X.shape[2]
how the input is looping to obtain output as follows: [[ 72.74373 106.51455 251.78499]]?
Can you give a clear idea what does n_steps=4, n_features=X.shape[2] really means and how does it function?
Yes, perhaps this will help you to understand the input shape:
https://machinelearningmastery.com/faq/single-faq/what-is-the-difference-between-samples-timesteps-and-features-for-lstm-input
Please, is there a method to find the correct parameter of an ANN model: LSTM, MLP (hidden layer number, activation function, loss function ..)
what does it mean when my train and validation loss curves are parallel while the Train Score and Test Score are small?
Is there a method to optimize all these results?
Yes, see this post:
https://machinelearningmastery.com/faq/single-faq/how-many-layers-and-nodes-do-i-need-in-my-neural-network
Thanks for the tutorial. I have applied the multistep, multivariate logic to my own dataset. Namely, I have 12 look-back, 12 look-ahead and 41 features (all having exact look-back as the main variable of interest). Trying the TimeDistributed code snippet gave me progressively increasing RMSE. Is this due to the nature of my time series or is it a sign of mistake done during construction of the model? It is hard to tell for you but maybe you can share your take on this issue. Thanks
It could be either.
Perhaps try fewer features and evaluate impact?
Perhaps try different models and evaluate impact?
Thanks II tried encoder-decoder and stack LSTM. Both gives me increasing RMSE for further look-aheads.It is understandable for encoder-decoder as it uses the output as an input (so associated error also comes with the prediction and builds up over time) but not sure why I see the same thing with the stack lstm. Anyways, thanks again for the response and the post!
Also, one quick related question. You use “-1” in multi step future multivariate split_sequence models (such as n_steps_out-1 etc.). This reduces the number of resulting features by one when compared to other split_sequence snippets. I tested it with the other multistep split_sequence code you shared above. Not sure but are’nt we supposed to have the same number of features? Thanks
Thanks.
Well done on the improvement!
Sir Plz! Suggest me good learning sources about my project ( carbon emission forcasting using LSTM).
Start here:
https://machinelearningmastery.com/start-here/#deep_learning_time_series
Thank you for the great tutorial. Is it possible to get the probability of prediction(in percentage) or second best prediction out of these models? Thank you)
Yes, model.predict() will return a probability on classification tasks.
More details here:
https://machinelearningmastery.com/how-to-make-classification-and-regression-predictions-for-deep-learning-models-in-keras/
Hi, Jason
Your tutorial helps me a lot, thank you very much!
And I have a question that how to adjust the learning rate of the LSTM network in the CNN-LSTM code you’ve mentioned above.
I’m looking forward to your reply, thank you!
(The reply I left in https://machinelearningmastery.com/how-to-develop-lstm-models-for-multi-step-time-series-forecasting-of-household-power-consumption/?unapproved=494293&moderation-hash=2b6d045a4e1ff047d0720753b2b1e418#comment-494293 is in wrong place, sorry about that)
You can learn more about how to tune the learning rate (generally) here:
https://machinelearningmastery.com/understand-the-dynamics-of-learning-rate-on-deep-learning-neural-networks/
This is amazing. I love the blog
Thanks Luis.
Thank for this nice explanation.
I have a problem when reshaping the data for multiple output architecture.
the architecture is:
outputs=[]
main_input = Input(shape= (seq_length,feature_cnt), name=’main_input’)
lstm = LSTM(32,return_sequences=True)(main_input)
for _ in range((5)):
prediction = LSTM(8,return_sequences=False)(lstm)
out = Dense(1)(prediction)
outputs.append(out)
model = Model(inputs=main_input, outputs=outputs)
model.compile(optimizer=’rmsprop’,loss=’mse’)
and when reshaping the y using:
y=y.reshape((len(y),5,1))
I got a reshaping error:
ValueError: Error when checking model target: the list of Numpy arrays that you are passing to your model is not the size the model expected. Expected to see 5 array(s), but instead got the following list of 1 arrays: [array([[0.35128802, 0.01439778, 0.60109704, 0.52722118, 0.25493708],
would you please help?
Perhaps define what you want the output shape to be, e.g. n samples with m time steps, then confirm your data has that shape, or if not set that shape?
You use “model.add(TimeDistributed(MaxPooling1D(pool_size=2)))” and write “max pooling layer that distills the filter maps down to 1/4 of their size”. A typo or is there a different reason explaining the use of 2 vs. 4 here?
Sorry for the confusion.
If the map is 8×8 and we apply a 2×2 pooling layer, then we get a 4×4 out, e.g. 1/4 the area (64 down to 16).
For time series, if we have 1×8 and apply a 1×2 pooling, we get 1×4, you’re right. 1/2 the size, not 1/4 as in image data.
Fixed. Thnaks!
Hi Jason,
first of all, thanks for that awesome introduction into LSTM-Models.
There is just one thing i don’t get.
In the section “Multiple Input Series” you used the following example:
[[ 10 15 25]
[ 20 25 45]
[ 30 35 65]
[ 40 45 85]
[ 50 55 105]
[ 60 65 125]
[ 70 75 145]
[ 80 85 165]
[ 90 95 185]]
As you mentioned the first two entries in the arrays refer to the two time series and the last one to the corresponding target variable. To train the LSTM you split the data into input and output samples like:
[[10 15]
[20 25]
[30 35]] 65
Why do I drop the first two target entries (25 and 45). Isn’t that information my network loses for training? Why don’t we use each (single) sample like x = [10 15] y[25] to train the time series. Isn’t it easier to lern the series if i have the target for each step?
Good question.
We must create samples of inputs and outputs.
Some of the input at the beginning of the dataset don’t have enough prior data to recreate an input, therefore must be removed.
Good work, However, you should provide the library imports, to make it easier for beginners.
All library inputs are provided in the “complete example” listed in the post.
Sorry for the confusion.
Hello Sir, I am so happy with your illustration, I have a problem with how to do forecasting based on your demonstration. I will be happy to get your email
Hi, Jason,I need to predict a hundred thousand sequences like this[10, 20, 30, 40, 50, 60, 70, 80, 90], how do I do it, do I do it in cycles, one by one, I do it in cycles, it feels like it’s going to take longer
If the model is read only and you are not dependent upon state across samples, you can run the model in parallel on different machines and prepare batches of samples for each model to make predictions.
Hi, I am very happy to have this LSTM example to have a practice.
I have a problem as follows:
I have 300 excel workbooks of which each excel sheet has 3 values…..
the 3 values will be in this format [1.02,2.20,1.0]; [2.9,3.5,3.3];…….like this 300 sets.
Now i want to train and test my model with the data from 300 excel workbooks as input and the model has to predict the 301th set for example: [5,3.3,2.4] depending on the sequence of previous values.
Note: the output shouldn’t be the probability set from the 300 sets, the output should be a new set.
Can you suggest me any solution to this problem?
Perhaps you can use some custom code to extract all of the data from the excel files into a csv file ready for modeling?
How to construct parallel three lstms, and then add a DNN in series.
You can use one LSTM with 3 variables or 3 LSTMs and concat the outputs together.
See the functional API:
https://machinelearningmastery.com/keras-functional-api-deep-learning/
Hi Jason,
Thanks for this wonderful post. I have been trying to digest LSTM’s (metaphorically) and one particular aspect was not clear to me. I know the general structure of LSTM’s but I’m having hard time to understand:
model.add(LSTM(50, activation=’relu’, input_shape=(n_steps, n_features)))
When ReLU is set as an activation function, but not in the output layer, what exactly happens behind the scenes? To make myself clear, I am aware of the gates and their respective activation functions: sigmoid and tanh. But if we set ReLU like above, does that mean that each unit/LSTM cell outputs a hidden state –> pass it to a ReLu –> pass it to the next unit/LSTM cell?
Thanks!
Yes, that is correct. It controls the output gate, not the internal gates which are governed by a sigmoid.
hello Mr Jason Brownlee please my dataset is in matrics form, i want convert it to fit into GRU or LSTM sequential model,
If your matrix represents a sequence, you can reshape it for your model. This will help:
https://machinelearningmastery.com/faq/single-faq/what-is-the-difference-between-samples-timesteps-and-features-for-lstm-input
If not, an RNN would not be appropriate.
Hi Jason,
A problem is involved in my mind, If it is possible, I want to know your opinion.
What will happen if we use both lstm and gru layers simultaneously in the model? Does this make sense?
For example this architecture:
model=Sequential()
model.add(GRU(256 , input_shape = (x.shape[1], x.shape[2]) , return_sequences=True))
model.add(LSTM(256))
model.add(Dense(64))
model.add(Dense(1))
Because I used this model and I got good results compared to using each one separately.
You can, but why?
Hello Jason and community,
I have a question. My dataset has 27 features. 26 of them I want to use as input and the last one as output (this feature is also the last column in my dataset). I use the multiple input multi-step output code from above. After using the function “def split_sequences(sequences, n_steps_in, n_steps_out)”, I split the dataset into train and test sets and choose a number of time steps for n_steps_in and n_steps out. After transforming from 2D to 3D with “split_sequences(train, n_steps_in, n_steps_out)” I printed the shape of train_X, train_y, test_X and test_y. The results are:
(14476887, 25, 26) (14476887, 20) (7130386, 25, 26) (7130386, 20)
My three questions are:
1.) Does python count from 0 upwards, so that 0 is my first feature or does it count from 1 upwards?
2.) Does python work from left to right, so that the left feature in the csv file is my first feature and so on?
3.) Is the shape above (7130386, 20) equal to (7130386, 20, 1) or why is it 2D?
I hope that I could explain my problem and the questions good enough.
Many thanks in advance.
Ali
Yes, array indexes start at 0.
Yes, arrays run from left to right.
Yes, you can transform (7130386, 20) to (7130386, 20, 1) directly. They are the same thing.
Hello Jason,
thank you so much for the answer. I have to other questions:
I take the ‘split a multivariate sequence into samples’ code from above:
def split_sequences(sequences, n_steps_in, n_steps_out):
X, y = list(), list()
for i in range(len(sequences)):
# find the end of this pattern
end_ix = i + n_steps_in
out_end_ix = end_ix + n_steps_out-1
# check if we are beyond the dataset
if out_end_ix > len(sequences):
break
# gather input and output parts of the pattern
seq_x, seq_y = sequences[i:end_ix, :-1], sequences[end_ix-1:out_end_ix, -1]
X.append(seq_x)
y.append(seq_y)
return array(X), array(y)
After that I split the dataset into train and test sets:
train_size = int(len(values) * 0.67)
test_size = len(values) – train_size
train, test = values[0:train_size,:], values[train_size:len(values),:]
print(len(train), len(test))
The result is:
14476930 7130429
The next step is to define the number of time steps:
n_steps_in, n_steps_out = 25, 20
train_X, train_y = split_sequences(train, n_steps_in, n_steps_out)
test_X, test_y = split_sequences(test, n_steps_in, n_steps_out)
print(train_X, train_y, test_X and test_y)
The result is:
(14476887, 25, 26) (14476887, 20) (7130386, 25, 26) (7130386, 20)
The last point is to create and fit the LSTM network:
n_features = 26
model = Sequential()
model.add(LSTM(50, input_shape=(n_steps_in, n_features)))
A lot of code, sorry for that. Now the short questions:
I want to predict the last column (column 27) in my csv-file. The first 26 are the input features (columns).
1.) Where in the codes above do I explicitly define my input features and my output feature?
2.) Do I have to explicitly use n_features in the code ‘model.add(LSTM(50, input_shape=(n_steps_in, n_features)))’. My aim is to train the model with the input features and the output feature and test it only with the test data without the output feature. The output feature shall be predicted.
Is the code with n_features = 26 in my case wrong?
Sorry that I bother you with this banal questions but I have not enough experience.
Many thanks in advance.
Ali
Not sure I understand, sorry.
Perhaps start with a solid understanding of features here:
https://machinelearningmastery.com/faq/single-faq/what-is-the-difference-between-samples-timesteps-and-features-for-lstm-input
Hi Jason,
Thanks for your post.
I would like to use a network architecture like:
cnn = Sequential([
Conv1D(filters=16, kernel_size=4, strides=2, activation=’relu’, input_shape=(n_steps, n_features)),
BatchNormalization(),
MaxPooling1D(pool_size=2)
])
model = Sequential()
model.add(cnn)
model.add(LSTM(50, activation=’relu’))
model.add(Dense(1))
The reason is that when the true model is path dependency, longer look back period should be used, but it is not very efficient for LSTM dealing with large time step, so I use CNN to reduce the length of time step and encode some predictive information.
Is this make sense to you?
Do you think pre-train would make some contribution in stacked network structure?
Joe
Don’t put stock into my speculations, perhaps try it and see?
Dear Jason,
Thank you for your great tutorial. I just have a question:
As I understood from your explanations, for bidirectional neural networks we need both past and future input data to predict the current time step. So, in case of univariate LSTM, when we are going to predict the energy use of current time as example, we need to know the energy use of future? This is abit confusing to me. Would you please explain about it.
Thank you
No, the future is predicted from the past.
Or you can frame your prediction problem any way you wish.
Thank you for your answer. Can you explain a bit more to make it clear? Because as I just checked the mathematical formulation of Bidirectional RNNs, I see that there is a hidden state of the next time step as the input: ( x(t), h(t-1) and h(t+1) are used to calculate y(t) ).
So, when there is a hidden state from the next time step as the input, how is t possible to just use the past data in univariate bidirectional RNN?
Thank you in advance for your guidance
Perhaps this will help:
https://machinelearningmastery.com/develop-bidirectional-lstm-sequence-classification-python-keras/
Actually I applied the bidirectional layer but I got much higher error than typical LSTM network. Is it possible or I am doing wrong?
When I write 50 neurons it means that each single layer of bidirectional has 50 neurons or it would be the summation of two layers?
Bidirectional may require more training.
Each direction has 50.
Hi, I have question regarding data normalization (scaling values between specific number such as [0,1]). Should I perform it before making the dataset supervised form as in this example? Or after the supervised form?
I noticed that if I do after, the columns looks little different from each other because the scaling are done via columns only. Here is example output if done after:
t-1 t t+1
-1.000000 -1.000000 -0.870529
-1.000000 -0.869976 -0.895359
-0.869976 -0.894799 -0.897133
-0.894799 -0.896572 -0.901271
Is this problematic to forecast via LSTM?
Yes, scaling should happen first.
Hi Jason.
Great Tutorial. I have electronic health record data which has multivariate time series inputs. Is it better to use normal LSTM or bidirectional LSTM for prediction?
Thanks
I would encourage you to test a range of models, linear, ml, mlp, cnn and lstms and discover what works best for your specific dataset.
This will help:
https://machinelearningmastery.com/how-to-develop-a-skilful-time-series-forecasting-model/
Hi Jason,
i am trying to train my model to forecast a 144 data points (1 day) (10 minutes for each values (load forecast for a home)) based on 5 days (=144*5 values) (i have more data but till now i didnt find a good result so i training my model by less amount of data.. it takes so long)
there a seasonality each day.. so i chose the n_input to be 144.
i am varying the batch size from 1 to 6… and the epochs from 25 to 150,
but my problem is: each time i get a result, i have one of these problems:
1- values converge to a constant (i thought maybe it is underfitting)… so i try to reduce batch size and increase epochs
2- when i do so.. i always get a loss value of n.a.n and then i get no predictions from the model….
can you please recommend something?
thank you so much!!!!
i appreciate it!
You can discover how to diagnose the performance of your model and improve performance here:
https://machinelearningmastery.com/start-here/#better
thank you,
but i mean not the values of the loss.. the values of the forecast.. they converge to a constant, and when i increase the number of epochs.. there are better results but then many times, starting from epoch n. 150 or so.. the loss is n.a.n.. and there are no predictions…
I see.
i searched more in the internet… i am thinking maybe i am dealing with the exploding gradient problem…
I see, this may help:
https://machinelearningmastery.com/how-to-avoid-exploding-gradients-in-neural-networks-with-gradient-clipping/
Hello Jason,
i still have another question:
is it better to forecast 144 values through the dense(144) at once?
or
like what i am doing.. i am forecasting only 1 value and then append my history with it:
history.append(yhat_sequence)
…
add.dense(1)
Thank you so Much!!!
Perhaps compare a few approaches for your dataset and discover what works best.
Hello Jason,
Thanks for this great tutorial and dive into LSTMs.
For Multiple Parallel Input and Multi-Step Output you also mention that it is possible to use the vector version of LSTMs. I cannot get my head around it how that model should look like.
model = Sequential()
model.add(LSTM(100, activation=’relu’, return_sequences=True, input_shape=(n_steps_in, n_features)))
model.add(LSTM(100, activation=’relu’, return_sequences=True))
# What is needed here??? dim to n_steps_out
model.add(TimeDistributed(Dense(n_features)))
model.compile(optimizer=’adam’, loss=’mse’)
The above architecture doesn’t what I intend. In the end there should be an output of dim (batch_size, n_steps_out, n_features) but what I achieve is (batch_size, 100, n_feautres) or an error. So how to make the above architecture work without the encoder-decoder version of your snippets?
Thanks a lot for all of your hard work
Perhaps you can use the example in the post as a starting point?
Hi Jason,
Really interesting article!!
Actually I have a doubt, I am currently trying to forecast sales of business based on the discounting burn. So, The future dependent variable values are usually fixed, is there any code which deals with such a problem.
Thanks and Regards
Perhaps you can adapt one of the examples here:
https://machinelearningmastery.com/start-here/#deep_learning_time_series
Dear Jason,
I have a question about Multivariate LSTM Models.
In the Multiple Input Series, your input is
80, 85
90, 95
100, 105
And you’re trying to predict the output of 205.
In the Multiple Parallel Series, your input is
70, 75, 145
80, 85, 165
90, 95, 185
And you are trying to predict the output of
[100, 105, 205]
My question is, in the first model, you know more information about the output in the past that you are not passing on to the model.
So, the actual input should be
80, 85, 165
90, 95, 185
100, 105, X
Where we are trying to predict X
Similarly, in the second model let us assume that you know the first two fields 100 and 105 and you only want to predict the 205.
70, 75, 145
80, 85, 165
90, 95, 185
100, 105, X
Again we are unnecessarily trying to predict some known values.
Is there a model where I can use all available information from the previous time series and try to predict X?
I learnt a lot from this post and the above question is something I am trying to answer. Thanks a lot for sharing your knowledge. It is helping us a lot.
Yes, you can frame the problem anyway you wish.
In your proposed framing, you could use a new token to indicate missing and then use a Masking input layer.
Or a multiple input model with a separate input for the dependent variables and the univariate series that your predicting.
Perhaps experiment and see what model you prefer and what works best for your specific dataset.
Thank you Jason. Is there any reference model that you can point me to where you have explained how the above has been done?
Great question.
I don’t think so off hand. I might have to create one.
Can you please give an example code for handling missing data and using Masking layer?
I am trying to eliminate training with samples where even 1 point has missing values in case of multivariate (multiple series)
Yes, see this tutorial:
https://machinelearningmastery.com/handle-missing-timesteps-sequence-prediction-problems-python/
Hi Jason,
I was implementing the cross-validation method for the LSTM Encoder-Decoder model, I wanted to ask you if it is better that at each step I recreate the class or I can use the old one calling the fit method.
Thanks and Regards
Typically cross-validation is not valid for sequence prediction, instead you must use walk-forward validation:
https://machinelearningmastery.com/backtest-machine-learning-models-time-series-forecasting/
Hi Jason,
If I have to train my model in such a manner that I have the data like :
Input are two columns i.e temperature and pressure i.e. the first 25 perc data and output are also two column temperature and pressure i.e. the 75 perc data remaining one.
My goal is to predict the temperature and pressure together by giving little input and receiving greater output to LSTM
. If i train my model by giving input [x,y] can I predict [x,y] but I do not want to give time stamp. Which method should I follow?
I have already made my data according to your blog and I am now confused hot to train the model without time steps
I recommend following this framework:
https://machinelearningmastery.com/how-to-develop-a-skilful-time-series-forecasting-model/
The tutorials here will be a helpful starting point:
https://machinelearningmastery.com/start-here/#deep_learning_time_series
Hi Jason,
Why you have not used the minmax scaler over here while training the input sequence in the LSTM model?
Good question, I skipped scaling to keep the example simpler – e.g. for brevity.
Thank you very much and I have one more question if I have 200000 data points and I have to make time steps for them maybe dividing the data into 5 time steps and giving 40,000 points in each of the timestep for LSTM will it be a good training? or you can suggest something for this? So, that I can prepare the data properly.
I have a multivariate data of 2 variables and want to predict both of them. So, basically 2 inputs and 2 outputs but do I have to make them supervised first as they are temperature and viscosity and they are dependent on each other with respect to time.
So, should I supervise them first or I can directly use multivariate time series for the prediction by dividing the data into 5 time steps and predicting 2 outputs.
Do you provide any consultations also?
Perhaps this post will help:
https://machinelearningmastery.com/prepare-univariate-time-series-data-long-short-term-memory-networks/
And the suggestions here:
https://machinelearningmastery.com/faq/single-faq/what-is-the-difference-between-samples-timesteps-and-features-for-lstm-input
Regarding consulting:
https://machinelearningmastery.com/faq/single-faq/can-you-do-some-consulting
Hi,
can you please tell me how to visualize the results. As, when I am reshaping the array it is not able to get reshaped into 2 dimension from 3D.
Thank you and have a nice day!
You can use matplotlib via the plot() function to create a line plot.
This will help with reshaping:
https://machinelearningmastery.com/faq/single-faq/what-is-the-difference-between-samples-timesteps-and-features-for-lstm-input
I think I did not reframe my question properly. My question is for example: I trained my LSTM model with 300 n_step_in and 300 n_steps_out. Now, after the training, yhat has a shape (20000, 300,2) . So, when I am reshaping it to 2D so as to see the results it is giving me an error and is not able to reshape it back.
Perhaps this post will help you with reshaping arrays:
https://machinelearningmastery.com/index-slice-reshape-numpy-arrays-machine-learning-python/
Hi can i add an extra layer under this one and if yes how should i do that?
model.add(LSTM(200, activation=’relu’, input_shape=(n_timesteps, n_features)))
Thanks in advance.
This tutorial shows how to create a stacked LSTM:
https://machinelearningmastery.com/stacked-long-short-term-memory-networks/
Hi, can you please tell me is this type of prediction only suitable for sequential data?
Sequence prediction is appropriate for data comprised of sequences. You can learn more here:
https://machinelearningmastery.com/sequence-prediction-problems-learning-lstm-recurrent-neural-networks/
Hi Jason,
If I have unsupervised data and I make it supervised for the training in the LSTM model.
My question is that when we make the data supervised and we give input data points and we predict the output data points, but the output is just the n+1 point of input and at last we are only predicting 1 point from the whole data. Basically we are giving the model all the points in the training only. What is the model actually doing?
The model learns a function that takes input points and predicts the next point.
but what if I want the model to get not all data as input points and just few input points to predict the remaining data? then what strategy is used?
You control what data goes in and out of the model.
Prepare the data you want to feed in and make a prediction.
The examples above will provide a template you can use to start with and adapt for your problem.
Yes i want to apply it at time series
I recommend the related tutorials here:
https://machinelearningmastery.com/start-here/#deep_learning_time_series
Sorry you are talking about time series, what if there is a date with time (I didn’t see the feature of date and time in your created data)
Date and time are removed from the dataset and the series of observations is worked with directly.
Hi Jason,
I have really enjoyed many of your articles over the last half year. Question on your output vector model using stacked LSTM model. Under the hood, what type of architecture is being used here for 3 input time-steps and 2 output time-steps. I’m sure it is a many-to-many problem, but can you help me with the exact visual connection? Is the first output time-step laid out directly over the second time-step of the input series?
Good question.
For a model that take a sequence input and outputs one time step which happens to be a vector – I would classify that as many to one model:
https://machinelearningmastery.com/models-sequence-prediction-recurrent-neural-networks/
Great read, that was a nice nugget of knowledge. Thank you I appreciate you work.
Thanks.
Hi Jason, thanks for your great posts and prompt replies. On a Multi-Step LSTM Models when I loaded my dataset I first noticed that the number of steps should be a number divisible by the length of the dataset (i.e. if my data is 1239 rows, a step in number of 59 is suitable since 1239/59 = 21). In fact trying with non-divisible numbers assigned to n_steps_in would result in nan loss values when fitting the model. I was indeed able to run all the way 50 epochs using 59 over 1239, however something I cannot explain happened: after re-running the code without making any changes, the loss on the various epochs (after setting the verbose to 1) jumped back to nan. Running it again it would start populating some values and along the way end up in nan.. It is very erratic and unpredictable and to end up all epochs looks like a lucky test, Could you help me to understand what is wrong? Thanks!
Yes, it might help to scale your data prior to modeling.
Yes, you are correct, as always. Scaling not only did not return nan but also made each epoch faster to run. Thanks Jason!
Well done.
Thanks Jason. I apologize if this was addressed somewhere in the list of comments but in the case of predicting a continuous variable, how would you compare the performance of LSTM vs. another algorithm such as Random Forest?
Other than comparing the actual value vs. predicted value from both models, is there a separate way to assess accuracy of both models?
Use the same test harness, that is the same evaluation methodology like walk forward validation:
https://machinelearningmastery.com/backtest-machine-learning-models-time-series-forecasting/
Often RMSE or MAE are great metrics to compare.
Thanks Jason,
all your work is clear! thank you very much. I have some questions if you please. What are the differences between all LSTM models you applied above ? is there and performance trade-off between them? because you repeat the sentence that we can use any of them for time series forecasting.
on the other hand, im working in the domain of wireless channel prediction. its a complex number problem. So can I split it into real and Imag parts and apply your LSTM models for each part separately and then concatenate the output results?
Good question.
Not so much a performance trade-off as different framings of the problem, or different problem types.
The goal was to show you how flexible the method is and that you should adapt it to your problem, not your problem to the method.
Not sure about imaginary numbers in neural nets or Keras, sorry.
I wanted to know how to approach this problem. Let’s say we have a time series with 2 features, ranging from 0 to n as:
[a0, b0], [a1, b1], [a2, b2] upto [an, bn]
The output of the series would be,
[a0 b0], [a1, b1], [a2, b2] -> [a3]
The issue is [b3] also play an important role in determining [a3].
My question is how do I incorporate this so that, I am able to use a0, a1, a2, b0, b1, b2, b3 to feed into the model and predict [a3].
Good question, start here:
https://machinelearningmastery.com/convert-time-series-supervised-learning-problem-python/
And then here:
https://machinelearningmastery.com/faq/single-faq/what-is-the-difference-between-samples-timesteps-and-features-for-lstm-input
Great tutorial.
I have a question related to lstm model for time series forecasting problem. I have dataset with four input features like 78, 153.23, 77.25, 4.33.
The first input ordering difference is like 78,80,87,96….so on.
The other inputs ordering is well like 77.25,77.35,77.40….
I have used lstm model with one previous timestamp as input to predict the next timestamp which predict well on the last three input but poor for the first one.i.e.
Actual: 78, 153.23, 77.25, 4.33
Predicted: 82, 153.01, 77.02, 4.12
How i tunned this model for good result of first input?
You can discover suggestions on how to diagnose and tune deep learning models here:
https://machinelearningmastery.com/start-here/#better
hi Jason,
I want to make a model to predict the Inflow to a reservoir, with past rainfall data, temperature data, and also past inflow data.
i want the model to be able to predict the inflow for a week ahead (7 timesteps) when given the past week’s, rainfall and temperature data.
what model should i use for this?
Great question, follow this process:
https://machinelearningmastery.com/how-to-develop-a-skilful-time-series-forecasting-model/
thank you jason. i read through the process and some other links on your site and decided that a multivariate multi-step lstm would help.
Is there specific link for that? because i only found univariate multistep lstm.
The above tutorial is a great starting place that you can adapt to your problem.
Hi Jason,
Can I put time in the X axis to predict wind speed on Y axis?
Best Regards
Sure. You would discard time and model wind speed directly.
sorry, i did not understand that. Should I discard time or I can use it to train my model so as to predict the wind speed.
The time column is typically discarded when observations are spaced at consistent intervals.
but what if the time is not consistent then?
Then see this:
https://machinelearningmastery.com/faq/single-faq/how-do-i-handle-discontiguous-time-series-data
Hi Jason,
In the “Multiple Parallel Input and Multi-Step Output” example, you stated that it could be done with the vector output method, or the encoder/decoder, and proceeded to demonstrate the encoder/decoder.
I’ve been wondering how the example would look in vector output form. Would the target, y, for each sample need to be merged into a single 1D array, or vector?
For example,
If y for one sample looks like:
[a1,b1,c1],
[a2,b2,c2],
…
[an,bn,cn]
Would we reshape it into something that looks like this?
[a1,b1,c1,a1,b2,c2,…,an,bn,cn]
Probably one long 1d vector with all time steps that you can then choose to interpret anyway you wish (e.g. by the structure of the expected/target y).
I’ve setup all the example in a Google Colab: https://colab.research.google.com/drive/16nsMXFDmzgdpsSY_p1ZljN5ZDzq9u6jY#scrollTo=xgSwSfpE3-GO&forceEdit=true&sandboxMode=true
I’d rather you didn’t.
hey,
How can we se the root mean square error in the training of the model here
Best Regards,
Kannu
Here is an example:
https://machinelearningmastery.com/custom-metrics-deep-learning-keras-python/
Hello Jason,
I am trying to use the CNN-LSTM for forecasting
The split sequences gives an output of
(175196, 4, 4) (175196, 1)
Where 175196 is the samples, 4 is number of steps and 4 is the features ( variables)
Then i reshape the input vector as directed in the tutorial, but when i run the model
I get this error:
at: TypeError Traceback (most recent call last)
in ()
22 model.add(TimeDistributed(MaxPooling1D(pool_size=2)))
23 model.add(TimeDistributed(Flatten()))
—> 24 model.add(LSTM(50, activation=’relu’))
25 model.add(Dense(1))
26 model.compile(optimizer=’adam’, loss=’mse’)
TypeError: while_loop() got an unexpected keyword argument ‘maximum_iterations’
I know it is hard to debug in this manner! but any idea what could be wrong here ?
Do other Keras examples work for you?
It is possible that there is a fault with your Keras/TF installation?
Yes Other keras examples work, CNN, Multi-Headed CNN etc.
You were right 🙂 I updated to higher version of tensorflow and keras and it worked! thanks!
Happy to hear that.
That is surprising, not sure I have good advice sorry.
Perhaps try simplifying the example and see what the case of the fault could be on your workstation?
Hi Jason,
Thank you for this interesting article. Can I create one model for all sites with LSTM ? That means if we have for example a group of persons and every person has its time series data with different features, LSTM model can learn from all these time series for once?
Best regards
Sure. Here are some suggestions:
https://machinelearningmastery.com/faq/single-faq/how-to-develop-forecast-models-for-multiple-sites
many thanks for your help
You’re welcome.
Hi Jason, how do we chose n_steps in the split_sequence() ? or we should consider n_steps as an hyper parameters or it can be set by an statistical test? Thank you for work jason. i am following ur site from past 2 yrs. ur content is best in the ml community.
A hyperparameter.
Thanks, I deeply appreciate your support!
Hi Jason,
i have some questions in LSTM model.
First, it is the LSTM input x definition. In the time series forecast case, we divide input data into some portion by batchsize parameters. Later, these 2D portion data were transformed into 3D tensor data and feed to model for training. After all portions feed to the model and complete forward/backward propagation, the 1 epoch routine is completed. My question is : in the x[t] input time, the LSTM model input x refers to only first portion of x data or the all portions data ?
Second, what is the LSTM_unit parameter definition ? My understanding is the number of the LSTM input x vector’s element. For example, if have 10 input, the LSTM_unit should be 10 to capture all the input vector. But, it is not always requiring the higher numbers such as 20, so on.
Third, is there any “feature importance” example in the LSTM now? I am looking forward and quite frustration this moment. Could LSTM and XGBoost have sample feature importance result ?
many thank
X refers to the input samples, for a definition see this:
https://machinelearningmastery.com/faq/single-faq/what-is-the-difference-between-samples-timesteps-and-features-for-lstm-input
A unit is like a node in an MLP. Each unit gets the entire input sequence as input. The number of nodes is unrelated to the number of input time steps.
Not that I’m aware.
Hi Jason, I run the first example, but it was failed. It shows: TypeError: Input ‘b’ of ‘MatMul’ Op has type float32 that does not match type int32 of argument ‘a’. Do you know what the problem is?
Sorry to hear that, I have some suggestions here:
https://machinelearningmastery.com/faq/single-faq/why-does-the-code-in-the-tutorial-not-work-for-me
Thank you, Jason. I have solved the problem. The reason is, I installed the tensorflow 2.0 + keras 2.2.4, but these two are not matched, so I use tensorflow.keras instead of keras. I added a command “x_input = x_input.astype(‘float32’)” in the code, and it run swiftly. Another way is to install the tensorflow version 1.15.0, and no problem occurs.
Happy to hear that you solved the problem.
You can use Keras 2.3. with TensorFlow 2.0, or Keras 2.2 with TensorFlow 1.15.
Hi Jason,
I have a problem to modelize and i think lstm network are the most adapted models to do it.
I want to predict the true trajectory of an airplane before it takes off. I have to set of data, the first is the trajectories announced before departure (the fake), and the second is the trajectory announced after landing (the true).
I want to predict the true, giving the fake.
I have a list of array, each array represent a flight made by a plane. Each flight is represented by different variable and after an interpolation i have 50 observations points by flight.
At each point we can observe a vector of our variables like latitude, longitude ect ..
Let assume i have N variables like that.
I have 2200 flights, so my input data is an array with (2200,50,N) shape.
I already tried a little model but oddly the model seems to follow the fake trajectory and not the true.
Do you have an idea of what architecture i can use ?
Thank you a lot
Perhaps test a suite of different approaches and discover what works best for your specific dataset?
Yeah this what i am doing, but maybe you can help with the last layer, i think the error comes from there.
As i said I have a vector (50,N) shape wich represent a flight with 50 points and N features, and i want to predict a (50,2) vector wich is 50 points with (latitude longitude).
I cannot use dense layer at the end of the model because it does not return the right shape.
Encoder-decoder with 2 nodes in the output layer and 50 in the repeat vector layer – this would achieve the desired output.
Hello,
Thanks for your tutorials; they are amazing! I’m having the following pitfall by implementing your ideas: I use your “split_sequences” in order to prepare the network input and, accordingly, I train my network and save the model. When I use the same input in the trained model and plot it, I get a very weirdo plot, like the many times over ploted lines. Do you mind what is my problem?
Thanks.
I don’t know off hand, sorry.
Hi Jason,
I’m building a Multiple Parallel Input and Multi-Step Output model, and I’m curious why you repeat the same LSTM output in
model.add(RepeatVector(n_steps_out))
? The alternative that I was thinking is using the keras functional API, training n_steps_out LSTMs from the input, concatenating the output of these LSTMs, and feeding it into the next LSTM. so it would look something like thisinput = Input(shape=(n_steps_in,n_features))
concat_layers = []
for i in range n_steps_out:
concat_layers.concat(LSTM(200,activation=’relu’))(input)
x = tf.keras.layer.Concatenate(concat_layers)
x = LSTM(200,activation=’relu’,return_sequences=True)(x)
x = TimeDistributed(Dense(n_features)))(x)
model=Model(input,x)
model.compile(optimizer=’adam’, loss=’mse’)
The biggest drawback that I can see is there will be a ton more parameters, but are there other issues that I’m missing? For instance, does this get rid of some relationship between the different timesteps that the previous model maintains better?
Thanks!
The reason is because it is an encoder-decoder model where the same encoding of the input is used in the generation of each output time step.
Perhaps try it and see? It’s could to test a suite of different models in order to discover what works best for your specific dataset.
Dear Jason,
thanks for the tutorial, that is very helpful! However, i am having a hard time to understand the input shape given in the CNN LSTM example below:
X, y = split_sequence(raw_seq, n_steps)
# reshape from [samples, timesteps] into [samples, subsequences, timesteps, features]
n_features = 1
n_seq = 2
n_steps = 2
X = X.reshape((X.shape[0], n_seq, n_steps, n_features))
# define model
model = Sequential()
model.add(TimeDistributed(Conv1D(filters=64, kernel_size=1, activation=’relu’), input_shape=(None, n_steps, n_features)))
…
Here, X is first reshaped into 4 dimensions, however, the input_shape defined and used in the model Conv1D layer is 3 dimensions. Is the None used in “input_shape=(None, n_steps, n_features)” referring to the “n_seq” dimension of X or the number of samples of X…?
And then, the data used to fit and predict are again 4 dimensions…
could you please kindly explain a bit? I am really confused …
thanks a lot!
Yes, the CNN must process sub sequences and then groups of processed subsequences are passed to the LSTM.
Accturally, each piece of X is 3 dimentions(n_seq, n_steps, n_features) and every time the model accepts one piece of X in this CNN-LSTM case.
I think the None refers to the n_seq but the n_seq is expressed through using TimeDistributed(), so there is a None to stand the place of the first dimentions.
Hi jason,
What if we had a dataset of every day of a years sales data and we wanted to predict say for example 10 days sales based on the sales data of previous 30 days? What should be the form of output that we get? and also the code for getting the predicted value? Is it model.predict(X_test)?
You could predict the 10 days directly:
https://machinelearningmastery.com/faq/single-faq/how-do-you-use-lstms-for-multi-step-time-series-forecasting
Hey Jason, I am halfway through and reading this stuff is pure joy! Thank you for your tremendous efforts and making this available! I’ve become an instand fan of your site.
Thanks Arne!
Hi Jason,
one practical question in LSTM. If the input data sets have the various range, how to deal with the LSTM forecast model ? For example, if input vector one spans 0~100, vector two spans 0~0.5, could we still put these two input vectors together to compile the model? I use SHAP package to analyze the weight. In this case, vector one is always very strong rather than vector two. In mathematical view, this result is correct. how do you think in this case?
jasper
You can try normalizing or standardizing each variable prior to modeling, or try using a relu activation.
This might help:
https://machinelearningmastery.com/machine-learning-data-transforms-for-time-series-forecasting/
Hi Jason,
Thank you for such a detailed explanation. I am having an issue with scaling data for a multistep multivariate lstm problem. I am taking data of last 14/21 days to predict for the next 7 days. Can you please give any idea what is the proper way of scaling data using MinMax for these type of problems, as I am lost in the shapes of matrices.
Thanks, I’m happy it helped!
Yes, see this:
https://machinelearningmastery.com/machine-learning-data-transforms-for-time-series-forecasting/
Thank you. I know how scaling works and I have implemented it in single step forecasting. However, when it comes to multistep, we actually split the data and it becomes 3 dim after using the split_sequency function. Which means we have 3 dim matrices for X and Y.
Scaler doesn’t work on 3 dim matrices.
If I do scaling before splitting, I will end up with a matrix dimension that I can’t retrieve after prediction and thus will be stuck without doing the inverse_transform for scaling. I will appreciate your help in this matter
Yes, it is sticky. You may have to write some custom code as the libraries don’t accomodate it.
Perhaps try using relu and no scaling, at least as a starting point.
Hi Jason,
I want to introduce the attention mechanism to the Encoder-Decoder model
for regression problem (with Multiple Input). Is there any other article that can help me solve this problem?
I hope to cover this topic in the future.
Hi Jason.
Is there some simple method to add attention to the Encoder_Decoder Model in this article?
I’ve trid to use AttentionWrapper class to achieve it, but I’m failed, because It’s hard for me to do it during a short time. So can you give me some guide?
Thank you!
The TensorFlow 2 API provides attention layers.
Hi Jason,
Thank you so much for this valuable tutorial. Really appreciate it.
Jason, I’m bit new to DL with RNN. I have two small doubts to get cleared. In my question I want to predict how many steps (i.e:- step counts) a participant walk tomorrow depending on the previous step counts. For this we have collected step counts of large number of participants for n number of days.
Is this a univariate problem where each participant step count is taken as a univariate sequence and train the model? AND do you think RNN is a good move to this problem?
Do I have to scale the sequences of each and everyone’s step counts (by taking the each participants current mean and sd) Or can’t I use the raw count?
Thank you so much in advanced again. All the best for your future work too!!!!
San
You’re welcome.
Sounds like univariate, this will help:
https://machinelearningmastery.com/taxonomy-of-time-series-forecasting-problems/
It is a good idea to scale data prior to modeling, perhaps try fitting on raw data first to get started.
Thank you Jason !!!. Please keep up the good work !!!
Thanks!
Thanks a lot Jason for sharing such a knowledgeable article,
I have a doubt in my case,
for the last- Multiple Parallel Input and Multi-Step Output
I am trying to predict next 6 or 12 hours data, as of now trying to predict next 6 hours data training with n_steps_in- 72 and expecting n_steps_out- 6 with 6 features
but I am getting output as nan
Please see if I am doing something wrong..
def split_sequences(sequences, n_steps_in, n_steps_out):
X,y = list(), list()
pt = progress_timer(description= ‘Split Sequences’, n_iter=len(sequences))
for i in range(len(sequences)):
# find the end of this pattern
end_ix = i + n_steps_in
out_end_ix = end_ix + n_steps_out
# check if we are beyond the dataset
if out_end_ix > len(sequences):
break
# gather input and output parts of the pattern
seq_x, seq_y = sequences[i:end_ix, :], sequences[end_ix:out_end_ix, :]
X.append(seq_x)
y.append(seq_y)
pt.update()
pt.finish()
return array(X), array(y)
dataset = df_104902.values
# choose a number of time steps
n_steps_in, n_steps_out = 72, 6
# covert into input/output
X, y = split_sequences(dataset, n_steps_in, n_steps_out)
# the dataset knows the number of features, e.g. 2
n_features = X.shape[2]
# define model
model = Sequential()
model.add(LSTM(200, activation=’relu’, input_shape=(n_steps_in, n_features)))
model.add(RepeatVector(n_steps_out))
model.add(LSTM(200, activation=’relu’, return_sequences=True))
model.add(TimeDistributed(Dense(n_features)))
model.compile(optimizer=’adam’, loss=’mse’)
# fit model
model.fit(X, y, epochs=30, verbose=0)
# demonstrate prediction
x_input = array(df_104902[-72:])
x_input = x_input.reshape((1, n_steps_in, n_features))
yhat = model.predict(x_input, verbose=0)
print(yhat)
Output is coming-
[[[nan nan nan nan nan nan]
[nan nan nan nan nan nan]
[nan nan nan nan nan nan]
[nan nan nan nan nan nan]
[nan nan nan nan nan nan]
[nan nan nan nan nan nan]]]
nan output is not good.
Perhaps check the scale of your input data and normalize or standardize prior to fitting the model?
also my X and y shape is – (20875, 72, 6) and (20875, 6, 6) respectively.
and x_input is (1, 72, 6)
I have the same problem with standardised data…it reduced number of features to 1,i assume coz hstack is not working and used concatenation instead…any suggestions
This might help:
https://machinelearningmastery.com/faq/single-faq/why-does-the-code-in-the-tutorial-not-work-for-me
Saludos Jason, una consulta, como puedo validar el método de predicción. He visto en otros ejemplos que la serie lo dividen en dos partes: en entrenamiento y prueba, y en este caso no lo hace, a que se debe eso ?
This most common approach is to use cross-validation:
https://machinelearningmastery.com/k-fold-cross-validation/
Dear Jason,
First of all, thank you so much for your time and great contents.
Second, I studied your website for long time. I have a question: I have developed a model which predict the price of shares, my model can predict X_test data as well, now how can I forecast sequences(future times) does not happened?
You’re welcome.
Call model.predict(newData) to make predictions on new data.
newData are not available, i.e. the future days does not happened and not available, how do I prepare them for the model?
You must design and train your model based on the data you will have available at the time a prediction is required.
For example, if you have 7 days prior data at the time of prediction when predicting the next week, then design your model around that and train it on that type of data.
Then when you start using your model on new data, you will have the data available.
Dear Jason,
Thank you so much for your time and attention. I will try your approach.
You’re welcome.
Dear Jason,
Thank you so much for your time and attention
I was wondering if I can use time as univariate sequence.
Regards
Sure.
Hello Jason,
my model will learn from the past Forcast data and past actual AC Power data.
my Input is the future 7 days Forecast as csv file.
my goal is to predict the AC Power data based on the input.
I dont know how to apply what I want to you model here.
can you please help me?
Perhaps start here:
https://machinelearningmastery.com/how-to-develop-a-skilful-time-series-forecasting-model/
Thank you so much. I was struggling to understand LSTM.
Your work helped me a lot.
You’re welcome, I’m happy to hear that.
Dear Jason,
Thank you for your contributions. You have helped me a lot in the start of deep learning.
I have a question. I am working on a model and surprisingly the predicted output shape is different from the target shape of training data
Traning: X (12000, 12, 8), Y (12000,)
Test: X (3000, 12, 8); Y (3000,)
pred = model.predict (X (3000, 12, 8))
and pred shape is (3000, 12, 1) but I was expecting (3000,)
what am i doing wrong?
Please help me
Perhaps double check the structure of your model, e.g. the output layer/model.
Dear Jason,
thanks for the tutorial, that is very helpful! However, I use data normalization method for input data(10,20,30…) carry out your Multi-Step LSTM Models, it happens error. I dont konw how to resolve it. Pls see the belowing program. Thanks!
from numpy import array
from keras.models import Sequential
from keras.layers import LSTM
from keras.layers import Dense
import numpy as np
import matplotlib.pyplot as plt
import pandas as pd
import collections
# split a univariate sequence into samples
def split_sequence(sequence, n_steps_in, n_steps_out):
X, y = list(), list()
for i in range(len(sequence)):
# find the end of this pattern
end_ix = i + n_steps_in
out_end_ix = end_ix + n_steps_out
# check if we are beyond the sequence
if out_end_ix > len(sequence):
break
# gather input and output parts of the pattern
seq_x, seq_y = sequence[i:end_ix], sequence[end_ix:out_end_ix]
X.append(seq_x)
y.append(seq_y)
return array(X), array(y)
# define input sequence
training_set = np.array([10, 20, 30, 40, 50, 60, 70, 80, 90])
training_set = training_set.reshape(-1,1)
from sklearn.preprocessing import MinMaxScaler
sc = MinMaxScaler(feature_range = (0, 1))
raw_seq = sc.fit_transform(training_set)
print(raw_seq)
# choose a number of time steps
n_steps_in, n_steps_out = 3, 2
# split into samples
X, y = split_sequence(raw_seq, n_steps_in, n_steps_out)
# reshape from [samples, timesteps] into [samples, timesteps, features]
n_features = 1
X = X.reshape((X.shape[0], X.shape[1], n_features))
# define model
model = Sequential()
model.add(LSTM(40, activation=’relu’, return_sequences=True, input_shape=(n_steps_in, n_features)))
model.add(LSTM(40, activation=’relu’))
model.add(Dense(n_steps_out))
model.compile(optimizer=’adam’, loss=’mse’)
# fit model
print(‘X: \n’,X)
print(‘y: \n’,y)
model.fit(X, y, epochs=60, verbose=0)
# demonstrate prediction
#x_input = array([70, 80, 90])
x_input = np.array([70, 80, 90])
x_input= x_input.reshape(-1,1)
x_input = sc.transform(x_input)
x_input = x_input.reshape((1, n_steps_in, n_features))
yhat = model.predict(x_input, verbose=0)
yhat = sc.inverse_transform(yhat)
print(100,110)
print(yhat)
I’m eager to help, but I don’t have the capacity to debug your code, sorry.
This might be helpful:
https://machinelearningmastery.com/machine-learning-data-transforms-for-time-series-forecasting/
Thank you so much.
I have resolved the problem.
Thank for your tutorial.
I’m happy to hear that.
X, y = split_sequence(raw_seq, n_steps)
# reshape from [samples, timesteps] into [samples, subsequences, timesteps, features]
n_features = 1
n_seq = 2
n_steps = 2
X = X.reshape((X.shape[0], n_seq, n_steps, n_features))
# define model
model = Sequential()
model.add(TimeDistributed(Conv1D(filters=64, kernel_size=1, activation=’relu’), input_shape=(None, n_steps, n_features)))
model.add(TimeDistributed(MaxPooling1D(pool_size=2)))
model.add(TimeDistributed(Flatten()))
model.add(LSTM(50, activation=’relu’))
The code in your post, use CNN+LSTM for Univariate Models above.
I am confused in the numbers of n_seq, why is 2. And Can I consider the n_seq as the times_step of LSTM?
The configuration is arbitrary, you can change it you anything you like.
Do we have any ways to find the best configuration of n_seq ?
And can I consider the n_seq as the times_step of LSTM? I mean the first subsequence is the first step as input of LSTM, and the second subsequence is the second step as input of LSTM?
If my understanding is wrong, please correct me!
It is a good idea to test different values.
Yes, see this:
https://machinelearningmastery.com/faq/single-faq/what-is-the-difference-between-samples-timesteps-and-features-for-lstm-input
Thank you for your great tutorial!
BTW I found a more pythonic way to write the split_sequence() function.
Regards,
Thanks for sharing.
Hello Mr. Jason,
Please, I have a technical question about the LSTM model.
The LSTM is defined with default activation functions such as:
3 sigmoid for the input gate, the foget gate and the output gate.
and 2 tanh for updating the internal states of the recurrent layer.
In your code:
In your code:
#########################
# define model
model = Sequential()
model.add(LSTM(50, activation=’relu’, return_sequences=True, input_shape=(n_steps, n_features)))
model.add(LSTM(50, activation=’relu’))
model.add(Dense(1))
#########################
Have you changed the sigmoid with a relu or a tanh?
Yes.
Thanks for the wonderful explanation. I have query regarding which category my dataset and requirement falls into.
i want to forecast number of defects for each of the 3 parts.
i have dataset like : Part (a,b,c are components of that Tool)
date Part Tools shipped num of defects(of parts)
2019-01-01 part a 2 0
2019-01-01 part b 1 2
2019-01-01 part c 2 2
2019-01-08 part a 2 0
2019-01-08 part b 1 1
2019-01-08 part c 2 1
2019-01-15 part a 2 0
2019-01-15 part b 1 1
2019-01-15 part c 2 3
i want to forecast what will be the number of defects of all parts in next 2 weeks for example.
Tools shipped column has relationship with number of defects.I have future data for Tools shipped too. so output desired :
2019-01-22 part a 2 ??
2019-01-22 part b 2 ??
2019-01-22 part c 2 ??
tools shipped for a particular week is constant
Sounds like a time series forecasting problem. See this:
https://machinelearningmastery.com/taxonomy-of-time-series-forecasting-problems/
Hi Jason,
Thank you for such an informative tutorial. I am planning on implementing LSTM for a multivariate time series data. The input dimension is (1000*7*24) and the output is (1000*30). I wanted to understand how can I decide how many layers and units to use. Similarly the batch size which would be appropriate in this case. It would be great you could comment on some of standard heuristics or point to some reliable resource for the same.
Good question, see this:
https://machinelearningmastery.com/faq/single-faq/how-many-layers-and-nodes-do-i-need-in-my-neural-network
Hi Jason,
Great tutorial, it really helped my get on my feet and started.
I have a question on Multiple Parallel series. Does parallel mean the input features and the output are treated as independent across columns?
To be more specific, using a 3 feature vector and 4 steps as input:
[ [F1_t1, F2_t1, F3_t1],
[F1_t2, F2_t2, F3_t2],
[F1_t3, F2_t3, F3_t3],
[F1_t4, F2_t4, F3_t4] ]
to predict:
[F1_t5, F2_t5, F3_t5]
does F1(t1 tot t4) have no effect on prediction F2_t5 or F3_t5 ?
Also, how would you go about combining Multiple input and Multiple parallel series in a case where the the input is a N-feature vector and using 3 timesteps, predict M-features (many-to-one), where M < N (and M-features included in the N-features) .
And on a separate note, any literature suggestions for using this with categorical data? I tried encoding to numerical, but they are not treated as categories
No, parallel inputs mean separate input variables measured over time. Perhaps this will help:
https://machinelearningmastery.com/taxonomy-of-time-series-forecasting-problems/
There are examples of multi-input and multi-output in the above tutorial.
Yes, try ordinal and one hot encode and compared to an embedding:
https://machinelearningmastery.com/how-to-prepare-categorical-data-for-deep-learning-in-python/
Hi Jason,
I am new for the LSTM, can you put a related picture of topology for each type’s visualization?
Yes, see this:
https://machinelearningmastery.com/models-sequence-prediction-recurrent-neural-networks/
Hi Jason,
Thank you very much your effort and for offering us your great tutorials. I enjoy a lot!
I do not have much experience with LSTM so I get already problems with definitions which are problably clear for most of the readers. For Vanilla LSTM you say you use 50 LSTM units. Does it mean you have 1 LSTM whose Input is 3 dimensional and the output 50 dimensional or you actually have 50 LSTM accepting 3 dimensional vectors and 1 dimensional outputs?
Yes, 50 units, each of which takes the full input and produce an output.
Hi Jason, where you state
Vanilla LSTM for univariate time series forecasting and make a single prediction.
is it possible to predict more than a single variable? How would I modify to make 5 value predictions?Yes, there are multi-step examples in the above tutorial.
Also, see this:
https://machinelearningmastery.com/faq/single-faq/how-do-you-use-lstms-for-multi-step-time-series-forecasting
Brilliant post. Very enlightening.
Thanks!
Hi Jason
I need help i am working on project for HAR for video dataset, could you help me making model
which use cnn-lstm .
Perhaps this will give you ideas:
https://machinelearningmastery.com/cnn-long-short-term-memory-networks/
Hi, Jason.
Great job, I have build a model, that performed well, but when I close the program, open and run again doesn’t perform equal, but when I restart the PC does work properly, I am running in CPU, what could be causing this problem? , how do avoid this from happening?, your answer will be most appreciated
I have not heard of this kind of problem before, sorry.
Perhaps try posting your experience on stackoverflow?
Thanks
You’re welcome.
Hello Jason
I was wondering how could we know the accuracy and have some sort of validation_data (the parameter used in model.fit).
This to obtain the loss and accuracy curves for training and validation
Could you please give me some guide on this
Thanks a lot
I recommend using walk-forward validation described here:
https://machinelearningmastery.com/backtest-machine-learning-models-time-series-forecasting/
This is the procedure used the majority of the tutorials here:
https://machinelearningmastery.com/start-here/#deep_learning_time_series
Hello Jason,
Thanks for the valuable efforts
Do you think that TS Deep Learning has proved itself successful when applied to stock market forecasting?
No.
See this:
https://machinelearningmastery.com/faq/single-faq/can-you-help-me-with-machine-learning-for-finance-or-the-stock-market
And this:
https://machinelearningmastery.com/findings-comparing-classical-and-machine-learning-methods-for-time-series-forecasting/
When I train my model it has a two-dimension output – it is (none, 1) – corresponding to the time series I’m trying to predict. But whenever I load the saved model in order to make predictions, it has a three-dimensional output – (none, 40, 1) – corresponding to the reshaping of the network input training dataset. What is wrong?
Here is the code:
df = np.load(‘Principal.npy’)
# Conv1D
#model = load_model(‘ModeloConv1D.h5’)
model = autoencoder_conv1D((2, 20, 17), n_passos=40)
model.load_weights(‘weights_35067.hdf5’)
# summarize model.
model.summary()
# load dataset
df = df
# split into input (X) and output (Y) variables
X = f.separar_interface(df, n_steps=40)
# THE X INPUT SHAPE (59891, 17) length and attributes, respectively ##
# conv1D input format
X = X.reshape(X.shape[0], 2, 20, X.shape[2])
# Make predictions
test_predictions = model.predict(X)
## test_predictions.shape = (59891, 40, 1)
test_predictions = model.predict(X).flatten()
##test_predictions.shape = (2395640, 1)
plt.figure(3)
plt.plot(test_predictions)
plt.legend(‘Prediction’)
plt.show()
This will help with shape – it applies to LSTMs and 1s CNNs:
https://machinelearningmastery.com/faq/single-faq/what-is-the-difference-between-samples-timesteps-and-features-for-lstm-input
Hello,
Thank you very much for your reply. Anyway, it didn’t help. I’ve changed the input size of my Conv1D from (2, 20, 17) to (40, 1, 17), but it didn’t accept – it tells me that it has negative dimension. I don’t understand why it doesn’t happen when training the network but does when I use the saved model to predict.
Layer (type) Output Shape Param #
=================================================================
time_distributed_14 (TimeDis (None, 4, 1, 24) 4104
_________________________________________________________________
time_distributed_15 (TimeDis (None, 4, 1, 24) 0
_________________________________________________________________
time_distributed_16 (TimeDis (None, 4, 1, 48) 9264
_________________________________________________________________
time_distributed_17 (TimeDis (None, 4, 1, 48) 0
_________________________________________________________________
time_distributed_18 (TimeDis (None, 4, 1, 64) 12352
_________________________________________________________________
time_distributed_19 (TimeDis (None, 4, 1, 64) 0
_________________________________________________________________
time_distributed_20 (TimeDis (None, 4, 64) 0
_________________________________________________________________
lstm_3 (LSTM) (None, 100) 66000
_________________________________________________________________
repeat_vector_2 (RepeatVecto (None, 40, 100) 0
_________________________________________________________________
lstm_4 (LSTM) (None, 40, 100) 80400
_________________________________________________________________
time_distributed_21 (TimeDis (None, 40, 1024) 103424
_________________________________________________________________
dropout_2 (Dropout) (None, 40, 1024) 0
_________________________________________________________________
dense_4 (Dense) (None, 40, 1) 1025
=================================================================
Perhaps there is a bug in your code.
I am happy to make some suggestions:
– Consider aggressively cutting the code back to the minimum required. This will help you isolate the problem and focus on it.
– Consider cutting the problem back to just one or a few simple examples.
– Consider finding other similar code examples that do work and slowly modify them to meet your needs. This might expose your misstep.
– Consider posting your question and code to StackOverflow.
Let me tell how I’ve solved, provisorily, the problem:
I’ve used your split_sequences() for multivariate and 40 steps. Therefore, for dataset was taking the ith+40 steps and later ith+1+40 steps and so on. It always has the last item of each subsequence as a new one, all the rest equals the past subsequence.
The output layer, for some reason that I still couldn’t figure out, is making a prediction of every subsequence. Then I design a function that takes the first item of each subsequence.
def separador_output(sequence):
X = list()
for i in range(len(sequence)):
x = sequence[i][30]
X.append(x)
return np.array(X)
As a result, I’ve got the 1-Dimension time-series I was trying to reproduce.
I sharing that because I still believe that there should be a manner of doing this without introduce such function as above.
Best regards!
Perhaps write your own function for preparing your data how you need it?
This might prove to be a useful starting point:
https://machinelearningmastery.com/convert-time-series-supervised-learning-problem-python/
Thank you for the excellent article!
I am trying to perform an LSTM model of time series data following the strategy you outline in tis article.
I have one input (feature) at multiple timepoints in the past, and I use your code “split_sequence()” to split the univariate sequence into multiple samples, each with a specified number of time steps and a single output.
I have to standardize my “train” dataset for which I had planned on using StandardScaler (per your other excellent articles including: https://machinelearningmastery.com/how-to-develop-lstm-models-for-time-series-forecasting/). I am performing the standardization prior to performing the SPLIT into multiple samples for the LSTM. This seems straightforward (although please comment if you think this plan is inappropriate.)
The complication is that at any given timepoint, my single input feature actually has multiple values, each derived from any one of many “related but independent” sources. While I can perform the LSTM on each source separately, I would like to try maximizing my sample size by performing the LSTM on the aggregate of all of the sources (since the sources seem to follow similar behavior to each other, but not necessarily within the same time window). Or at least I would like to see what the results of that aggregated model looks like. My only question is: does it make more sense to perform the data input standardization separately for each source (so each source is standardized to mean of zero and SD of 1, and has equal weighting in the model), versus standardizing once across all sources in the aggregated data.
(I am relatively new to machine learning, so I apologize if my question is a bit naive.)
Thank you for your thoughts.
Jim
Each feature or “time series” variable will need to be scaled separately.
I understand “feature”.
By ‘”time series” variable’: Do you mean each of the individual sequences created by the “split_sequence()” function in your example is scaled separately?
Thank you!
No, each series in the original data.
You can learn more here:
https://machinelearningmastery.com/faq/single-faq/what-is-the-difference-between-samples-timesteps-and-features-for-lstm-input
Hey, Jason Thanks for your helpful blog. could you please help me on a case?
my data includes a fixed size of input as (1, 16, 2) . but output is different in number of timesteps. i mean that one may be like (1,2,2) or other may be (1, 20, 2). i thought to use Encoder-Decoder format. but the problem is determining dimension of “repeatVector()”. how should i do that?
is it possible to adjust its size for each input?
Perhaps try padding all output sequences to the same length and use an encoder-decoder model to that length.
Sir please can you explain
Why in multiple input series the input shape is (3,2) while in multiple parallel series it is (3,3)?
This tutorial will help you understand input shape for LSTMs:
https://machinelearningmastery.com/faq/single-faq/what-is-the-difference-between-samples-timesteps-and-features-for-lstm-input
what about EarlyStopping, ModelCheckpoint, and ReduceLROnPlateau functions with lstm. And also i want to update my model with receiving data. i mean i want to train my model after every new data. how can i do it.
Yes, try them and see if they lift performance.
Don’t you need to test whether the data fits?
Sorry, what do you mean exactly?
Hey Jason, very well written!
I have a question on your 1DConv LSTM network below:
model.add(TimeDistributed(Conv1D(filters=64, kernel_size=1, activation=’relu’), input_shape=(None, n_steps, n_features)))
model.add(TimeDistributed(MaxPooling1D(pool_size=2)))
model.add(TimeDistributed(Flatten()))
model.add(LSTM(50, activation=’relu’))
model.add(Dense(1))
I’m wondering what the intuition behind applying a convolution with a 1D kernel on a sequence of data is? What does this involve – is this equivalent to taking a single value as a feature, to represent the input sequence?
Thanks for this resource!
Thanks.
It attempts to extract patterns from the sequence.
Will it not just be applying the same constant filter across the whole sequence equally, transforming it by some constant?
Each filter will extract different patterns from the sequence – in an analogy to filters extracting patterns from an image.
Hi Jason,
What are the limits of LSTM models on multistep prediction length?, like if we have N samples and M features and we are predicting K future samples of a 1-D variable. Is there a way to relate N, M and K? or to have a quick rule of thumb on how large K can go before it doesn’t make sense anymore?
Thanks!
The further you predict into the future, the more errors will compound.
Harder problems are more challenging to forecast.
That is about as general as we can go – you will need test specific models on specific datasets to learn more.
Hi Jason,
is there a method to choose the best n-steps-in? knowing that I need to make a prediction of 3 days, and I have a data of 1 year (8760 observations).
Test different values for your dataset and use the configuration that results in the best average performance.
Sir can u write the same code for functional api
Thanks for the suggestion, perhaps this will help:
https://machinelearningmastery.com/keras-functional-api-deep-learning/
thank you Dr.Jason,
it is very helpful as always.
i applied for my dataset
https://www.kaggle.com/abdulmeral/rnn-4-models-for-lstm
Well done!
omg omg omg omg omg, I just graduate last year and i did my internship. there i learn the great wonders of machine learning. for 1 year i have look at so many tutorial saying a this and that blah blah blah then it takes me a couple of hours to try to run that nonsense. Then i saw this, omg I cant stop saying that. this is what i want. this is it, i just want a simple code that i can run my self, i don’t need the million lines of explanation. i just want to know what works. you sir are my hero. Thank you so much from the bottom of my heart, i really feel that i don’t deserve this kindness of free usable knowledge. Thank you Dr Jason Brownlee my hero.
Well done!
Professor Brownlee , What makes you put relu activation function on LSTM? When I tested my own project, the loss value increased astronomically (e.g. Loss: 2382585115.4067 Acc: 0.23)
When I removed relu function on LSTM. It run as charm. Could you explain it more about this topic?
I find relu works well in lstm when we don’t scale inputs.
If it doesn’t work well for your data, don’t use it. Find what works best on your project.
Hi professor Brownlee,
Thank you for this excelent work.
I’m thinking why when i use “Multiple Input Multi-Step Output” and select just 1 n_steps_out i don’t get the same result has i just make a simple Multiple Input Series predicting the next output?
Shouldn’t get the same result?
Thank you,
The models and data are very small in these cases, they are to show you how to use them, not actually solve the tiny prediction tasks.
Hello everyone.
Thanks to Mr.Jason Brownlee
I reviewed some examples of the site (airplane passengers and shampoo) and I am totally confused the concept of timesteps with features.
here we assume that data is like:
X, y
10, 20, 30 40
20, 30, 40 50
30, 40, 50 60
and then it is concluded that the timesteps is 3 and features is 1.
but in shampoo sales prediction we always change the data shape into
X = X.reshape(X.shape[0], 1, X.shape[1])
no matter how many lags we took in the model. it means we assume the timesteps to be 1 and features to be equal the number of lags.
I ll appreciated if anyone can help me understand those concepts.
Yes, this is a tricky topic, I believe this will help:
https://machinelearningmastery.com/faq/single-faq/what-is-the-difference-between-samples-timesteps-and-features-for-lstm-input
Hi Jason, thanks for so nice article.
I have a large set of number sequences, labeled as “good” or “bad”. I need to build a model, so given a new sequence, it can classify as “good” or “bad” based on training. I’m not sure what model to use, because I need to classify, not to predict next value.
It’s like classifying dogs and cats from pictures, but instead of pictures I have sequence of numbers where the order matters.
Thank you!
That sounds like a great project!
The tutorials on sequence classification here will help you to get started:
https://machinelearningmastery.com/start-here/#deep_learning_time_series
Thank you so much for this. Preparing data is always a great task. I have a chat dataset which I want to use to create a chatbot. How should I prepare data for the encoder-decoder model?
Good question, it depends on the specifics of your model.
Perhaps prepare sequences of integers (ordinal encoded words) for input and output sequences per sample.
You can see examples here:
https://machinelearningmastery.com/start-here/#nlp
Hi Jason, thank you so much for this article. I really liked this and learned many things.
I have a time series data, I have 60 input data points and I have to predict 1 output at the last layer of LSTM, so basically I want my lstm to be
first_day_data–>lstm_unit1–>second_day_data–>lstm_unit2–>….60th_day_data–>lstm_unit60–>denseLayer–>output.
is something like this,
data is – [1,2,3,4….60] and output only single value for ex. 5.6. How to construct this model using keras ?
This will help you prepare your data:
https://machinelearningmastery.com/faq/single-faq/what-is-the-difference-between-samples-timesteps-and-features-for-lstm-input
Hi Jason,
If I have a time series with say 600000 time steps as output and 3 time serieses for 3 features all of them also of the same length. Then I form sequences (say 60 consecutive features at a time, first sequence will be 1-60, second will be 2-61 third will be 3-62 and so on) from my data and now I want to split it into training and testing sets.
If I shuffle the sequences (within a sequence, all the points will still be chronologically sequential) and then split the data into 80 – 20 train test split, is that ok or would it lead to data leakage into testing?
Yes.
Hi Jason, sorry for my ambiguity in the question. You said yes for
1. Ok to shuffle or
2. data leak into testing?
Thank you so much!
If you shuffle sequences, then train a model, it will likely lead to data leakage and an optimistic evaluation of model performance.
We must use walk-forward validation in most cases:
https://machinelearningmastery.com/backtest-machine-learning-models-time-series-forecasting/
Thank you Jason! ????
You’re welcome.
Also, thank you for this and all the other articles!
You’re welcome!
Is there some tutorial for LSTM + Time series in R?
With best regards
Sorry, I don’t have examples of LSTMs in R.
Hi! Great job with this website! It is very useful.
I have a question: Does the order of input train samples matter?
Example:
product_id | day_1 | day_2 | day_3 | day_4 | day_5 | day_6
1 | 10 | 3 | 2 | 5 | 9 | 10
2 | 11 | 5 | 2 | 4 | 3 | 2
3 | 14 | 8 | 5 | 0 | 2 | 14
4 | 10 | 0 | 1 | 5 | 1 | 1
train dataset:
[10,3,2,5] -> [9,10] #item 1
[11,5,2,4] -> [3,2] #item 2
[14,8,5,0] -> [2,14] #item 3
[10,0,1,5] -> [1,1] #item4
(to be more precise I have x products with sales for z days and I want to make each of the products a train sample, but by doing this the days will be repeated for each product is it correct? or should I build a train sample to contain all items?) * I mention that I’ve implemented the sliding window approach to build the dataset for each item
Thanks!
Yes, the order of samples probably matters both in splitting data for train/eval and within the training and test set themselves.
At the model.fit the samples are automatically shuffle, so in this case the order of samples(items)[in train] still matter?
hi Jason,
Could you provide your opinion on this usecase – I am working on a multivariate, multi-step time series problem to forecast sales for each of the cities. I understand from your tutorials how to use LSTM with vector output on such a problem but how do I handle the forecasting by cities? one way I read is to build separate models for each of the cities and then model concatenate at the end. what are your thoughts? do you have a post on it that I can refer to?
Your blog has been a “go to” solution for all my problems. Thanks for sharing knowledge and keeping it simple!
Good question, this will give you some ideas:
https://machinelearningmastery.com/faq/single-faq/how-to-develop-forecast-models-for-multiple-sites
Thanks a lot for the article, can you please explain briefly how to do the same in Java using Deeplearning4j library
Sorry, I don’t have any exampels for Java.
Hey Jason
Thanks for the wonderful article. Can you help me with the data reshaping for the Multiple Parallel Series for a CNN LSTM model? It would be great if you could provide a python function fro the same. As a beginner, it is a bit tricky to understand the data shapes needed for the different models. Thanks
Yes, see this:
https://machinelearningmastery.com/faq/single-faq/what-is-the-difference-between-samples-timesteps-and-features-for-lstm-input
Hi Jason ,
What should we do when using string data as input ?
I get the error that string data could not be converted to float type.
how can i solve this problem?
If the string represents a categorical input, you can encode it:
https://machinelearningmastery.com/how-to-prepare-categorical-data-for-deep-learning-in-python/
Otherwise, you can use a bag of words model or a embedding:
https://machinelearningmastery.com/start-here/#nlp
Hi, Jason. Thank you for your helpful blog and post.
I’m doing a project to predict COVID-19 growth in countries/regions. My plan is to use data of a handful of chosen countries in training and do the prediction with only one country (dataset: https://github.com/datasets/covid-19/blob/master/data/time-series-19-covid-combined.csv). Is this possible with the knowledge exposed in the post? If yes, which type of time series I’ll have to apply? Univariate? Multivariate? Multi-step?
Best regards,
Higo
To be a little more specific:
I wanna use the “Confirmed”, “Recovered” and “Deaths” to predict “Cases” (and eventually “Deaths”).
The growth rate can be modelled directly with an exponential function, use the GROWTH() function in excel.
Jason, thanks for the reply, but I don’t think I expressed myself in the best way.
What I intend to do on my project is to train an LSTM with data from confirmed cases, recovered patients and deaths from a certain set of countries and try to predict the number of cases in another country. The dataset is that on my first comment.
For example: training the LSTM with data from Australia, Costa Rica, Greece, Hungary and Israel (from 2020-01-22 to 2020-06-15) and trying to predict the number of cases in Brazil (here i would like to try two approaches: a validation with predictions in the same range 2020-01-22 to 2020-06-15, and another aimed at predicting future cases, beyond the date 2020-06-15).
Which of the approaches exposed in the article should I use? It is not yet clear to me which would be the best.
Thanks in advance.
That sounds like a great project, I think this might give you some ideas:
https://machinelearningmastery.com/faq/single-faq/how-to-develop-forecast-models-for-multiple-sites
Good day sir,
I would like to know, how can I get the next week’s forecasting results in the vanilla LSTM model. In this site example, we only get single forecast value.
Can you help me in this senario.?
See this:
https://machinelearningmastery.com/make-predictions-long-short-term-memory-models-keras/
Hi Jason,
Thank you for the valuable post. I have a question regarding multi-step LSTM model. I was trying to apply CNN-LSTM for the multi-step model, but I am a bit confused on reshaping [sample, timesteps] into [sample, subsequences, time steps, features].
The example code for the stacked LSTM is
X = X.reshape((X.shape[0], X.shape[1], n_features))
but in case of CNN-LSTM, we need the number of subsequence for the CNN model. But whenever I input n_seq=2 and run the code
X = X.reshape((X.shape[0], n_seq, X.shape[1], n_features))
, the error occurred: ValueError: cannot reshape array of size 15 into shape (5,2,3,3)
Would you please help me resolve the problem?
Thank you in advance.
You’re welcome.
You may need to experiment with different input shapes that are divisible by the number of timesteps in each sample.
Hi and thank you for great explanation
I have another situation, lets say I have
20 33
30 43
40 53
50 63
60 ?
so I need to predict a time series but with help of another that I already have, whats the best approach?
I recommend that you test a suite of different models and configs and discover what works best for your dataset, this may help:
https://machinelearningmastery.com/how-to-develop-a-skilful-time-series-forecasting-model/
Hi Jason, Thank you so much fr all your work!
It is a blessing to have such a talented educator as you to teach the practical side of ML.
I used this tutorial to create a timeforecast for COVID 19.
I was wondering, can i use different data generators (in my specific practice case: coutries) to learn the behavior?
In your example of the shampoo sales: Can i use different companies sales numbers to predict?
Or do i have to fit one net per data generator?
You’re welcome!
Great question, yes this will give you some ideas:
https://machinelearningmastery.com/faq/single-faq/how-to-develop-forecast-models-for-multiple-sites
Hello,
I am testing some forecasting algorithms including the LSTM model. From this, I wanted to seek its complexity in terms of memory and computing time.
So if you allow me, what complexities for the example of the univriate time series forecasting presented in the example above.
Thank you so much
Not sure off hand, sorry. You might have to check the literature if anyone has estimated the big-O for the method.
Hi Jason,
I have a litlle complictaed, but I think not so rare forecast Problem I’d like to solve.
Example description:
Lets say we do klimate-measurements at ground level but also at 15km hight. The last 2 years we started weatherbaloons every day to measure i.e. the pressure at 15 km hight. weather balloons are expensive and not really enviromental friendly, so we like to reduce the amount of weather balloons we need.
The idea:
from now on, we could start a weather balloon only every Sunday. The folowing 6 days we would predict the pressure at 15 km hight based on the current measurements of each day at ground level and on the Sundays we could ‘refocus’ our model using the real world measurement.
This sounds feasable to me, but I do not know where to start.
my first idea:
Not really what i want but possible:
put all the input data for last week together ((Sunday+)? Monday-Sunday) in one feature set and build a standard RNN to predict the pressure in 15km hight for Monday-Saturday. I think this would work, but then i would only get the values for last week. If I would like to have a estimation for today I to not see a way.
I think there are many processes you could optimise this way. Also in Industry where products of one batch often have rather equal properties. We could drastically reduce the prodcution time if we predict kalibration Measurements which take a long time to perform based on rather simple Measurements.
Do you have a idea how I could start building such a model? Do you know a good book with a similar example?
Chears,
Julian
That sounds like a fun project.
Generally, I would encourage you to prototype and evaluate each approach you can think of, rather than guess a priori what might be best – use results to guide you.
Thank you for your insightful work.
Why does the input shape contain the number of steps :
model.add(LSTM(50, activation=’relu’, input_shape=(n_steps, n_features)))
model.add(Dense(1))
It seems that the actual shape of the input can do the job:
model.add(LSTM(50, activation=’relu’, input_shape=(X.shape[1], n_features)))
Thanks again.
You can use either, as long as the model matches the data.
Great tutorial, your work has always been of help to me. I am trying to develop a predictive model for a belt drive. In this case, my time series data is not necessarily for forecasting but the trained model predicts the status of the belt drive based on new time series data. Is LSTM nevertheless optimal or do you have any two to three neural network you can recommend in this case?
You’re welcome.
Good question. I recommend testing a suite of algorithms and algorithm configurations in order to discover what works best for your specific dataset.
Hey Jason, I have very much enjoyed your tutorial! In your opinion, is there a ‘right’ amount to data points (e.g., rows) to feed into an LSTM model? I was thinking to use around 500000 – 1M data points and I was wondering if they are too much and what wold be the limitations between using a small dataset vs a very large one?
Thanks, love your website!
Thanks.
Good question, this might help:
https://machinelearningmastery.com/much-training-data-required-machine-learning/
Hello Jason,
Thanks for your great tutorials, they have been always very helpful.
I’m interested in the calculation process behind LSTM. I’m familiar with all formulas which are used in LSTM but I’m not sure what is the input at each calculation step in Vanilla LSTM example.
For example, let suppose that the input time series is [30, 40, 50]
So, at the first step, using C_{0} (cell memory), H_{0} (cell output) and number 30 (from time series above), we calculate C_{1} and H_{1}
Next, using C_{1}, H_{1} and 40 are calculated C_{2} and H_{2} and so on. Right?
I’m a little confused because in sentence time series, each word can be represented as a one-hot vector and in that example, the sentence would be time series of one-hot vectors and at each calculation step, the input in the formula would be one one-hot vector.
Regards, Enes
You’re welcome.
Good question, this will help:
https://machinelearningmastery.com/faq/single-faq/what-is-the-difference-between-samples-timesteps-and-features-for-lstm-input
And this:
https://machinelearningmastery.com/faq/single-faq/how-is-data-processed-by-an-lstm
Hi, Jason, thank you so much for your great tutorials.
I am using multiple-variables multiple-steps encoder-decoder LSTM. In my case, the input steps, output steps, and n_features are 150, 15, and 11, respectively. But I have a really large number of timesteps (~100,000).
So the input [100000, 150, 11] and output [100000,15, 11] are used to train. I set the epochs to 50 and got the model after 4h’s training. But I find that all the prediction result of this model keeps constant, i.e. [0, 15, 11], [1, 15, 11], [2, 15, 11], … are the same.
I will be grateful if you could give me some possible reasons that I should check.
Thank you!
That is too many time steps. I recommend splitting up the sequences:
https://machinelearningmastery.com/handle-long-sequences-long-short-term-memory-recurrent-neural-networks/
hi jason, great article!!!
I have a dataset with 3 years of historical precipitation and radiation data.
Which of the above models would be more logical to use so that I could predict both variables at the same time?
Is this enough data for a forecast?
How would I predict the next 30 days of the month from the last dataset date?
Sorry for so many questions!
Perhaps start with this framework:
https://machinelearningmastery.com/how-to-develop-a-skilful-time-series-forecasting-model/
Hello
I have an EEG(brain signal) dataset which i want to use for classification. .64 electrodes are attached to every subject(patient) and 5012 samples are recorded for every electrode. this way every subject has 64 series of 5012 samples and one class label for each subject. likewise there are 108 such subjects.
Can you suggest the right deep learning method that can be used for classification?
I recommend testing a suite of different framings of the problem, models, model configuration, and data preparations in order to discover what works best for your specific dataset.
The tutorials here will help you to get started:
https://machinelearningmastery.com/start-here/#deep_learning_time_series
I have a question about builind a test harness for testing LSTMs vs different other models.
My data is structured as follows:
Input: Information on weather, construction works, accidents in a road network
Output: cars passing a counter
Accidents that happened in the morning would affect traffic in the afternoon and traffic patterns that developed in the morning due to these accidents will as well. Hence I thought an LSTM could help. But I want to test against simpler models.
I would imagine model performance varies over the course of the day so my performance measure would be a graph showing the errors of the model over the course of the day as a distribution as the test set would include multiple days.
Where I am stuck is the training part: I selected a few characteristic days over the past years that I want to pass to the model. I assume that no effects spill over from one day to the next as there’s almost no traffic at night. So in effect my train data set consists of a number of days that shall be taken individually. That way I don’t have to pass years of data but can select typical days and only train on these. How do I pass these to the model and avoid at the same time that the “memory” takes info from previous days into consideration?
Should I just use one model.fit(X, y) where I add a dummy variable to the X representing the day? that doesn’t seem like good practice to me. If I do not point out the day specifically the model may think that the state of the neural network from the day before would affect the following day.
Or fit the model multiple times, e. g.
for day in sample_days:
model.test(X_day, y_day)
Sorry, mistake in the last code snippet. That would have to be:
for day in sample_days:
model.fit(X_day, y_day)
Perhaps you can use all prior data up to the day you want to test as training, then test on the hold out day. Repeat for each day you want to evaluate.
Hello Jason, thank you for this tutorial which is very useful. I’m working on panel data right now, i.e. I observe certain variables on several individuals at different times. I have a dataset of 719 individuals and 11 variables observed daily over 10 years (2010 to 2019).
Can we apply an LSTM model on these data?
If yes, how to prepare the data (reshape).
Thank you.
LSTM might be appropriate if each subject is a time series and you want to learn across subjects.
This will help you prepare the data:
https://machinelearningmastery.com/faq/single-faq/what-is-the-difference-between-samples-timesteps-and-features-for-lstm-input
Thank you Jason, Emmanuel. I read the link on data preperation – very useful. I have a question of clarification:
I have panel data on 200 different companies, each company belongs to a different sector of which there are 12 of these different sectors labelled numerically as 1-12.
For each company there are 8 different pieces of price information such as price, market capitalisation, volume, and so forth.
I then have a column of of future company stock price which is 10 days ahead. My aim is to predict this column.
The date range is from 2010 – 2012. Weekly, 104 dates for all 200 companies.
My understanding is that this means 200 samples, 104 timesteps, 9 features including the 10 day ahead stock price.
Would this mean I need to train 200 different models? How would you go about this problem if you were given this dataset?
Sorry if this is a daft question. I am new to ML.
Hi Guanta…You may want to consider multivariate LSTM models:
https://machinelearningmastery.com/multivariate-time-series-forecasting-lstms-keras/
Hi Jason,
Your answers were always inspiring for me. I am always thankful for that.
I have a question please.
I’m working on a stock price forecasting problem.
Assuming (t-current time, t+1 -future time), I prepared my data set as follows: Xt -> Yt+1, so that data links current feature inputs with future change in the price.
As I understand, RNNs try to map Yt -> Yt+1. In my case I cannot include (Yt+1, Yt+2,…) in the training set as upon using the trained model for forecasting; these will be unknown future values that cannot be fed into the model.
On the other hand, using a data set of Xt -> Yt does not hold the core information: the future price change of the stock, so as to be able to forecast it.
What would be your advice?
How can I make use of say: Xt-n, … ,Xt, Yt-n,…,Yt to forecast Yt+1 ?
Thanks.
I don’t think stock price is predictable:
https://machinelearningmastery.com/faq/single-faq/can-you-help-me-with-machine-learning-for-finance-or-the-stock-market
Thanks Jason, I know your opinion about stock price prediction, but I happened to collect data in this domain for learning purposes. I am trying to learn more about time series forecasting. I will be thankful if you could answer my above question as it is vital for me to better understand the use of RNNs.
Malik
Sorry, I don’t think I follow your question.
Recall, you have complete control over the choice of inputs and outputs of the model. Try a suite of different framings of the problem and discover what works well/best for your dataset.
Perhaps try modeling the target using any and all data you have available. Then, once you have something working, try removing/ablating data and review how it impacts model performance.
If you’re question is about the mechanics of preparing data, perhaps the function in this tutorial will be helpful:
https://machinelearningmastery.com/convert-time-series-supervised-learning-problem-python/
Hi Jason, I have time series eye data like diameter, number of blinks, duration of fixation and each features has different threshold like diameter is more than 3.5 means high cognitive load for eyes. Which LSTM can I use for this dataset to measure cognitive load? Or any other ML will fit for this problem?
I recommend testing a suite of different models and model configurations, not list lstms, in order to discover what works best for your specific dataset.
Hi Jason,
Thanks for your suggestions.
I don’t have ground truth data. I’m recording data using device and I’m thinking to ask user to label data for the last 2/3 min recording data. But it has downside to label many rows with same label. Is there any way to generate ground truth data?
Thanks
You can take each candidate answer as a separate row, or try consolidating each row using the mode or mean estimate.
Thank you Jason,
I love your articles so much I’ve bought several of your books which I find excellent.
I have a data prep question….
I’m training an LTSM multi-classification model;
I find that the classes in my training set (training data is chronologically before the val & test data) are very unbalanced.
I’m particularly interested in the minority classes (their accurate prediction is more important to me).
Given the dependent nature of timeseries observations and how I’m training in batches with each batch maintaining state (even in the stateless LSTM)…
Am I correct in saying that I cannot upsample or downsample the training data to balance the classes in the training dataset? (because either omitting or adding any data points, in this case there’s a datapoint for every day, would mess up the timeseries in a batch).
Do you have any advice for how I can balance out my training dataset?
Appreciate your insight,
Thanks,
Simon
Thank you deeply Simon!
Great question.
First, select an appropriate metric, not accuracy.
Second, try a cost-sensitive LSTM (and other neural nets). Try weights that balance the classes first, later try more agressive over-corrective weights and see if you can do better.
Finally, try simple duplication of input patterns for the minority class and add gaussian noise to the observations – e.g. a primitive form of random oversampling.
Let me know how you go.
Brilliant suggestions Jason, thank you!
I’ll try those out, I’m learning a lot from you and appreciate your explanations
You’re welcome.
Thank so much for your articles, I have been learning deep NN and LSTM, this helps me a lot to understand deep down and to build my own model for time series analysis.
You’re very welcome!
Hi Jason!
I always appreciate your blobs. They help me understand the deep idea of DNN with precious sample codes.
Now, I’m little struggling with CNN + LSTM model for Multivariate – Multistep time series forecasting problem.
I experimentally added CNN before LSTM layer and your blob made me notice that I needed TimeDistributed wrapper to layers before LSTM layers. To do so, I reshaped input as follows, as well as x validation set.
[Before adding CNN]
InputLayer(input_shape=(x_train.shape[1],x_train.shape[2]), batch_size=BATCH_SIZE))
x_train.shape[1]: time steps (e.g. 600)
x_train.shape[2]; # of features (e.g. 4, since it’s Multivariate)
batch_size: I specifed it as 128 or 256 since stateful=True in LSTM arg.
[Now]
InputLayer(input_shape=(x_train_multi.shape[1],x_train_multi.shape[2],x_train_multi.shape[3]), batch_size=BATCH_SIZE)
x_train.shape[1]: subsequences (e.g. 600)
x_train.shape[2]: time steps (e.g. 1)
x_train.shape[3]: # of features, No change.,
batch_size: No change.
I adjusted the ratio of [1]:[2], then found 600:1 is the best.
After all, the following is my current model snippet.
model.add(InputLayer( “AS [Now] ABOVE” ))
model.add(TimeDistributed(Conv1D(filters=200, kernel_size=3, strides=1, padding=”causal”, activation=”relu”)))
## model.add(TimeDistributed(MaxPooling1D(pool_size=2)))
model.add(TimeDistributed(Flatten()))
model.add(LSTM(150, stateful=True, return_sequences=True))
model.add(LSTM(150, stateful=True, return_sequences=False))
model.add(Dense(150, activation=’relu’))
model.add(Dense(8)) # forcast 8 time points
“fit()” works normally and the accuracy is almost the same as before I added CNN.
However, when I enable MaxPooling1D layer after Conv1D, the layer throws ValueError with regards to input shape. When I delete “padding=”causal”” from Conv1D arg, Conv1D also throws the same ValueError too.
I’m sorry for this long question, but if you see any wrong part especially about the input shape, please give me your comment.
Thank you.
Well done!
Sorry, I don’t have a good off the cuff answer for you, you will need to tune the model for your problem including ensuring the architecture is a good match for the shape of the data flowing through the model. I cannot debug the model for you.
Thank you for your reply, Jason!
Your comment cheers me up since I’m the only one who is doing ML in my office.
I found the cause of this ValueError. It is because the size of Maxpooling1D has to be more than “timesteps”. As I posted, I reshaped the original time step 600 into 600 x 1 ( subsequences x “timesteps” in [samples, subsequences, “timesteps”, features]).
It has to be 300 x *2*(or more) since the pooling size is *2*.
But no errors do not mean that it is correct. I hope this would work to fit.
Well done Shinichiro Imoto!
Hi, Jason, thank you for your tutorial. I have a question, I want to predict the flood, and my data is not continuous, like for the year, 2019, I have the data of part weeks of 5, 8 month, and for the year 2020, I have data of 3, 6 month. how should I do to make the prediction?
This may help:
https://machinelearningmastery.com/make-predictions-long-short-term-memory-models-keras/
Sorry Jason, I have read many times and alongside with some questions people asked above. I still don’t understand what’s the difference of using a RepeatVector comparing to LSTM with return_sequence = True? Is there any easy way to understand the major difference? Would like to understand when each method would be ideal to use.
Much appreciated!
Repeat vector uses the same single output vector from the encoder in the creation of each output step by the decoder.
Return sequences is the output of each input time step from the encoder.
Hi Jason,
Thank you for this wonderful post!
I have tried out multistep your example “Vector Output Model” with exactly the same numbers, same code. Some of the important data:
raw_seq = [10, 20, 30, 40, 50, 60, 70, 80, 90]
n_steps_in, n_steps_out = 3, 2
x_input = array([70, 80, 90])
print(yhat)
[[124.500435 137.70433 ]]
Normally yhat should be close to 100 and 110. Do you have an explanation what is happening or possibly going wrong?
By the way, I am running Keras ‘2.3.0-tf’. Re-running the model changes the numbers but it stays far away from 100 and 110.
Good question, see this:
https://machinelearningmastery.com/faq/single-faq/why-do-i-get-different-results-each-time-i-run-the-code
I am going back to your multi-step LSTM example.
You have the following parameters in the example:
raw_seq = [10, 20, 30, 40, 50, 60, 70, 80, 90]
n_steps_in, n_steps_out = 3, 2
I am wondering, how many multi-steps could be predicted maximally?
Imagine that I have a serie of 100 timepoints. What would a the max. reliable multi-step forecast and the most optimal split of X and y?
Hello Jason,
thank you very much for your great work. Please let me ask you two questions:
a) can I train (fit) a LSTM with a series with e.g. timestep =5, but predict the network with data with timestep = 1?
b) is there a relationship between quantity of timestep and quantity of hidden layers or neurons per layer?
Thanks
BR
Armin
I don’t see why not. You might have to re-define the model input layer after it is fit.
Yes, it varies for dataset and models. Run a sensitivity analysis to see how performance varies with model capacity in your case.
Thank you very much and greetings from Bavaria.
You’re welcome!
Thanks for the algorithms. I have a question about how optmize the LTSM hyperparameters. Is there some algorithm that do this?
Yes, a grid search or a random search are a good start.
I’ve difficulty in understanding LSTM input shape. For example. I’ve 50 videos out of these 25 are categorized as Awake (0) and 25 as Drowsy (1). I preprocessed them to extract Eye Aspect Ratio and Mouth Aspect Ratio as features every second.
Now my data has ( VideoFileName, Time Series, EAR, MAR, Label )
Video1 1 0.30 0.25 0
Video1 2 0.31 0.27 0
Video1 3 0.35 0.25 0
Video2 1 0.30 0.25 1
Video2 2 0.27 0.28 1
Video2 3 0.31 0.29 1
Video2 4 0.33 0.30 1
I extracted above data from first 3 and 4 seconds of two videos respectively as the length of videos may be different.
I’ve a very basic question here. How should I feed this data to LSTM? Any code example would be fine. I know input shape should be [Batch Size, Time Step, Features] but I’m confused how to feed this to LSTM should I feed each video’s data in a loop.
Please help me to clear my doubt.
This will help:
https://machinelearningmastery.com/faq/single-faq/what-is-the-difference-between-samples-timesteps-and-features-for-lstm-input
Hello Jason,
Great article!
just a quick question about the split sequence method for Multiple Input Multi-Step Output.
On this line, you select only the first two features in X and the last feature in Y.
seq_x, seq_y = sequences [i: end_ix,: -1], sequences [end_ix-1: out_end_ix, -1]
Why not include the 3 features in X?
That is to say, use the 3 features to predict only the 3rd.
Would that be a problem?
Thank you
You can structure the prediction problem any way you wish.
Perhaps this will help understand how to reshape data:
https://machinelearningmastery.com/time-series-forecasting-supervised-learning/
Thanks Jason for this great article!
You’re welcome.
Thanks you very much for this. I learned a lot from you different post, especially LSTM. Just wondering if you have recommendation using LSTM for anomaly detection? Thank you!
THanks.
Yes, you could use LSTMs for time series classification, this will help:
https://machinelearningmastery.com/faq/single-faq/how-do-i-model-anomaly-detection
Thanks for sharing this! I learned really well about LSTM models, and I am wondering why you used a Vanilla LSTM on ‘Multiple Input Series’ part, and why I cant use other models such as Stacked LSTM, Bidirectional LSTM, or ConvLSTM. Is it because of the dimensional of input?
In some cases yes, on other cases because one model performs better than the others for a given dataset.
Thanks for sharing, how would you model a regression problem to predict at a various arbitrary time steps? For example: predicting a inflection point where we are interested in where inflection occur and when is the time step it will happen. For example: The next predicted inflection point at 12345 occur at t+136.
Will it be the same multi time step LSTM model above, or is it a completely different problem, and how can we approach to this?
There are many ways to approach the problem, perhaps prototype a few and discover what works well/best for your dataset.
e.g. time series classification – is an event expected to occur in the next interval.
or multi-step forecast and use an if-statement to post-process the predictions.
etc.
First, thanks for this great article, I just found it on Linkedin.
Currently I am working on a project where I want to predict how many pieces of a material should be ordered for the next three month.
I have purchasing data of 20,000 materials (different time series) on monthly base which correlate to eachother ín case of seasonality but have very short time series (50-80 data points).
For example:
date | mat | amount | workload |
2020-08 | A | 20.0 | 0.8
Does it make sense to build a LSTM model for this kind of problem?
As a regressor I could implement the months (for seasonality) and also the workload for this month.
I could train the model with all time series and 80-90% of data points. The other 10-20% for test set)
Maybe another model is better? (S)ARIMA is only a univariate approach, so I can’t implement the workload.
Thank you!
Good question, I recommend evaluating a suite of different algorithms/configs and discover what works well or best for your dataset.
This framework may help:
https://machinelearningmastery.com/how-to-develop-a-skilful-time-series-forecasting-model/
Thank you for your response!
Do you have any approach how to handle time series data which is effected by covid-19.
For example: I have timeseries from 2017 to 2020 on monthly base.
For two months (April and March, 2020) the production went down, so that I have small values for this two months and also some high values in the following months, because production went up again (in total there are outliers over 4-6 months).
I have tried several approaches, but these outliers of covid-19 makes it hard to get good results in case of forecasting (training/testing). Also, like I mentioned before there are thousands of timeseries that are effected (differently but of course with some correlation)
Do you have any advices?
Yes, see this:
https://machinelearningmastery.com/faq/single-faq/how-can-i-use-machine-learning-to-model-covid-19-data
Is the model trained only with training data or for every prediction the actual data of the prediction is added to trainig data and the model retrained?
You can re-train the model as new observations become available if you like – both in walk forward validation and when the model is deployed.
Hi Jason, amazing article covering many of the shapes of the LSTM!
I have one question:
I am using PyTorch instead of Keras and would like to reproduce your vanilla LSTM. Could you please explain more about what is the ‘input’ parameter of the LSTM?
Thanks!
Thanks.
Perhaps this will help:
https://machinelearningmastery.com/pytorch-tutorial-develop-deep-learning-models/
Hi jason,
I am trying to build and LSTM for a time series data, unfortunately i am unable to reshape a 4D input data into 3D input data to fit my LSTM model. do you know how is this possible?
Good question, this will help:
https://machinelearningmastery.com/faq/single-faq/what-is-the-difference-between-samples-timesteps-and-features-for-lstm-input
Hi Jason, I am trying to develop a custom loss function for my LSTM mode which was based on yours, like:
model = Sequential()
model.add(LSTM(neurons, activation=’relu’, input_shape=(n_steps_in, n_features)))
model.add(RepeatVector(n_steps_out))
model.add(LSTM(neurons, activation=’relu’, return_sequences=True))
model.add(TimeDistributed(Dense(1)))
model.compile(optimizer=’adam’, loss=my_loss,run_eagerly=True)
The custom loss my_loss function receives from Keras the parameters (y_true,y_pred), in my understanding they should have shape like input_shape. However, regardless of the input shape I use, y_true comes with shape (32,1,1), even if I remove all layers and leave a bare Sequential model.
I am trying to understand the logic of this, googled around but so far nothing helped me to explain this.
This will help:
https://machinelearningmastery.com/faq/single-faq/what-is-the-difference-between-samples-timesteps-and-features-for-lstm-input
Actually I made some confusion in this question. Trying to explain again: imagine that I am trying to predict a series with a single (1-dimensional) value each time step, so y_true, y_pred should have shape (total_time_steps,1). However I always get shape (32,1,1), with values that have no remembrance to the actual values.
Sorry, I don’t understand what you’re asking exactly. Perhaps you can rephrase.
Hi Jason, thanks so much for the reply. I did a further search and found the answers to my problem. First, y_true, y_pred come with sizes defined by batch_size; second, by default, their values come shuffled, so I have to use shuffle=False. Third, and most important, I don’t know if what I am trying to do is even possible with Keras because all the operations in the loss function have to use tensor operations, otherwise the loss function cannot provide gradients to the optimiser. My intended loss function goes sequentially over each element of y_true and y_pread, compares each pair and updates an accumulation function not definable by custom algebraic/symbolic functions. It’s a bit large and too specific to share here, but if you are interested I can share the details of what I am trying to do.
Perhaps there’s an optimiser in Keras that does not require gradients, but not that I know of
Well done!
Hi Jason, I learn a lot from your articles. Could you please help on a network. I have an input of presumably (4, 10, 2). [(10,2) are time steps and features, respectively.] There are a lot of data in such a shape and for each one I propose to train a lstm and then make a Convolution layer among them. So by an Conv1D(1), I expect the output (3, 10, 2).
please correct me if I am wrong. I reshaped data into (1, 4,10,2). Then I used TimeDistributed wrapper for prediction. but then I am not able to make a convolution on shape[0] (I mean 4). what is get is convolution on the shape[2] (I mean 2). can you help me how to arrange data for the network or whether my network is true or not?
Typically you would use a CNN than an LSTM, not the other way around.
I have not tried LSTM-CNN, but I expect it would be challenging and you may need to debug the model yourself.
Hello Jason!
First off, thanks for being here for my machine learning journey! So I have a base scenario to check for understanding:
Context: I have supervised binary classification dataset on weather temperatures with 4 features. Target variable at time t is 0 or 1. 0 is if temperature at t+30 is down, 1 if up.
Framing the Problem for LSTM: Say timesteps is 60. So we take the previous 60 timesteps of data to predict 0 or 1 for t+1. In doing so, we can predict if the weather temperature is up or down in 30 days. Input shape would be (60, 4). I would have to chunk the training dataset and reshape it to be compatible with the (60, 4) input shape.
Is my understanding correct? Thank you!
You’re welcome.
Sounds about right, also see this help you confirm:
https://machinelearningmastery.com/faq/single-faq/what-is-the-difference-between-samples-timesteps-and-features-for-lstm-input
Sweet! Thanks 🙂
Follow up question: Is there an article that can provide guidelines in order to build a good base LSTM model (ie adding layers, how many layers, stacked or vanilla, # of units in each layer, etc)?
I feel like I’m shooting in the dark with loss values and accuracy not changing at all for every epoch.
This might help:
https://machinelearningmastery.com/faq/single-faq/how-many-layers-and-nodes-do-i-need-in-my-neural-network
Dear Jason,
The tutorial was really useful as ever is.
But I have not seen in your tutorials that you applied any **Bilstm** network for regression to predict ** Multivariate and Multi-step ahead** data.
I have created a Bilstm to forecast 9 features in terms of 3-time steps ahead.
model = Sequential()
model.add(Bidirectional(LSTM(200, return_sequences=True), activation=’relu’, input_shape=(n_steps_in, n_features)))
model.add(RepeatVector(n_steps_out))
model.add(Dropout(0.5))
model.add(Bidirectional(LSTM(100, activation=’relu’, return_sequences=False)))
model.add(Dense(3))
model.add(TimeDistributed(Dense(n_features)))
model.compile(optimizer=’adam’, loss=’mse’)
I am really eager to know whether the given model is correct or not.
Moreover, the output prediction is not well, so I would like to know the answer to some questions.
1- Is it common to use **Bilstm** for regression in the case of ** Multivariate and Multi-step ahead**??
2- what is the best model for regression in the case of ** Multivariate and Multi-step ahead**??
3- is the given model created correctly or not?
I am really sorry for writing too much, but I am really looking forward to get anwer.
Best
Mary
No, typically bidirectional LSTMs are not used in the encoder-decoder architecture, but I don’t see any reason why they couldn’t be used.
We cannot know the best model for a given dataset, the job of a machine learning practitioner is to use careful experiments and discvoer what works well or best.
Dear Jason,
I really appreciate your quick reply.
But I did not get the answer o this question:
1- Is the below architecture correct logically?
( I am a beginner in using Bilstm in regression, so I am not sure whether I made the layers correctly or not)?
model = Sequential()
model.add(Bidirectional(LSTM(200, return_sequences=True), activation=’relu’, input_shape=(n_steps_in, n_features)))
model.add(RepeatVector(n_steps_out))
model.add(Dropout(0.5))
model.add(Bidirectional(LSTM(100, activation=’relu’, return_sequences=False)))
model.add(Dense(3))
model.add(TimeDistributed(Dense(n_features)))
model.compile(optimizer=’adam’, loss=’mse’)
I have created a Bilstm to forecast 9 features in terms of 3-time steps ahead.
2- Is it common to use **Bilstm** for regression in the case of ** Multivariate and Multi-step ahead**??
I am really looking forward to see your clear answer, as I did not get the mean of your previous answer.
Best
Mary
I don’t have the capacity to review and comment on your model architecture:
https://machinelearningmastery.com/faq/single-faq/can-you-read-review-or-debug-my-code
LSTMs are used for sequence prediction, not regression. In numeric sequence prediction, bidirectional are rarely used – but if it gives the best results for your dataset, then use it.
Dear Jason,
I am grateful for your reply.
As you mentioned “LSTMs are used for sequence prediction, not regression”,
so do you have a tutorial post to introduce the best techniques for numeric sequence regression?
As I cannot differentiate between sequence prediction and sequence regression.
I want to predict 9 features in terms of 3-time steps ahead.
would you please introduce me to some useful methods?
Best
Mary
“regression” is a row of data without sequence.
“sequence prediction” or “sequence regression” are the same kind of thing. The above examples fall into this.
You cannot accurately refer to “sequence prediction” or “sequence regression” as “regression” as an LSTM cannot be used for the latter, but can be used for the former.
I hope that is clearer.
Dear Jason,
Thank you a lot for the time you spent answering that much clearly.
Best
Mary
You’re welcome.
i want to make an earthquake prediction using rnn (LSTM).I am getting difficulty to code . can you please help me
Is there a specific problem you are having that I can perhaps address?
can i get it from those code you have provided here? If yes then which LSTM should i follow?
I have no examples of “earthquake prediction”.
Perhaps you can start with a model listed above and adapt it for your specific dataset.
Many thanks
This is my situation: I have several companies. According to 20 measurable features varying from year to year. For ten years, we have a binary classification Fail/Succes.
My question is what model adequate for this problem to train the machine to predict a probable success or failure of a given company with its successive given features?
Many thanks
Perhaps try a suite of data preparation methods, models and model configurations in order to discover what works well or best for your dataset.
This process may help:
https://machinelearningmastery.com/start-here/#process
Many thanks
A googling led to your article
https://machinelearningmastery.com/how-to-develop-baseline-forecasts-for-multi-site-multivariate-air-pollution-time-series-forecasting/
Is it helpful?
I think only you can comment whether you find the linked article helpful.
Thanks Jason for the insights. I have one question regarding Convo_LSTM. It can extract the spatio-temporal features. How can we input the spatio data? Do we have the examples code of it?
Yes, convlstm is designed for patio-temporal data.
It takes a sequence of images as input.
Thanks a lot for your kind reply.
Do you mean to extract spatio temporal feature , we need to input a sequence of images as input rather than a sequence of values?
Yes.
Thank you for your great tutorial. I learned through this article but I have a question about the number of samples.
If [10, 20, 30, 40, 50, 60, 70, 80, 90] is one sample, I have about 10,000 samples that each sample is independent and has the same characteristics.
For instance, [10.01, 20, 30.035, 40.102, 50.1, 60, 70.364, 80.112, 90.623], [10.541, 20.983, 30.097, 40.152, 50.2, 60.942, 70.73, 80, 90.53], [10.543, 20.486, 30.897, 40, 50.766, 60.519, 70.132, 80.11, 90.445], …
In this case, I am wondering if there is a way to apply all 10,000 samples to training the model.
Thank you.
You can learn more about “samples” for LSTMs here:
https://machinelearningmastery.com/faq/single-faq/what-is-the-difference-between-samples-timesteps-and-features-for-lstm-input
Dear Jason,
I am not familiar with python. Do you have this tutorial in R?
Best
Yilma
Sorry I do not.
Hi Jason
regarding the case ‘Multiple Parallel Series’… my problem has 150k time series, and for each I need to predict the future value.
I guess this means that I will have 150k features.
So the input array for my LSTM NN will have dimensions [n_samples, n_steps, 150k].
The size of the array is too large! I get the error:
‘Unable to allocate 606. MiB for an array with shape (365, 3, 150000) and data type float32’.
What should I do? is this the right way to approach the problem?
Many thanks!
Yes, or perhaps you can use an alternate strategy:
https://machinelearningmastery.com/faq/single-faq/how-to-develop-forecast-models-for-multiple-sites
Also, you may want to work with a smaller sample of the data initially or run on a large machine like an AWS EC2 instance with tons of RAM.
Hi Jason
I want to train my LSTM NN with random samples taken from a timeseries.
Should I normalize the whole series or each sample individually?
Thanks
Ideally, data scaling is fit on training examples and applied to train and test examples.
This may help:
https://machinelearningmastery.com/machine-learning-data-transforms-for-time-series-forecasting/
Hi Jason,
I am intending to do my research work in electricity prices forecasting. I have electricity price data of 6 years from 2012 to 2017. And I need to forecast the value for 2017 using NEURAL NETWORK AUTO REGRESSIVE in R.The data set ranges from January 1st 2012 to December 31th 2017 (52608 observations, covering 2192 days). Each day of the data set comprises 24 observations, where each observation corresponds to a load period. For modeling and forecasting purposes,the data set is further divided into two sets:January 1st 2012 to December 31th 2016 (43848 observations, covering 1827 days) for identification and estimation of the models, and January 1st 2017 to December 31th 2017 (8760 observations, covering 365 days) for evaluating one-day-ahead out-of-sample forecasting accuracy of the models. I need your help. I’ve tried searching but couldn’t find a specific code of one day ahead forecasting with NN-AR .Can you kindly send me the code of neural network autoregression to make forecasts for one-day-ahead out-of-sample forecasting for the complete year 2017. I will be highly obliged for this favor. Thank you and have a nice day.
This will help you prepare your data for modeling:
https://machinelearningmastery.com/faq/single-faq/what-is-the-difference-between-samples-timesteps-and-features-for-lstm-input
This will help you understand how to make out of sample predictions:
https://machinelearningmastery.com/make-predictions-long-short-term-memory-models-keras/
I hope that helps as a first step.
Hi Jason,
Great book! Wish I understood more, but I’m on my way.
About the tutorial. You’ve stacked the output sequence with the input sequence, and I’m trying to understand how it differentiates x from y.
Let’s say, I have 10 input_seq and 1 out_seq how would you approach this?
I tried it myself with some random numbers, but the code predicts all values along the x-axis, which takes forever with LSTM. Should I stack the output)seq at the end of the input_seq’s.
Thanks in advance!
Thanks.
They are past observations of the target that we believe will help to predict future values of the target.
Hello I am new to machine learning and trying to wrap my head around some of the examples to find the best use cases for each.
In the section on ‘Multiple Parallel Series’ is this procesessed as multiple paralel univariable predictions or multiple multivariable predictions?
I am looking for a solution where it is the later. I was considering creating seperate multivariable models for each output but wondering if the parallel series might be the better way to go.
Multiple parallel univariate time series, which is a multivariate input time series.
Perhaps experiment with a few of the approaches and see what is a good fit for your data.
Hi Jason,
Really great and informative article. My first time working with LSTMs but the input format is really clear and has been easy to understand.
I am trying to adapt this to an a problem I am trying to solve. I am trying to predict net income from a financial income statement from 31 balance sheet and income statement items. I am using 3 years of quarterly data to predict this, thus a time step of 12. For each yhat, my x_train contains 12 lists for each quarter that contains the 31 independent balance sheet/ income statement variables being used to try and predict my yhat.
Thus due to the fact my y_train has a length of 63, my input data is 63 x 12 x 31. This is stored at a list of arrays, each with 12 lists containing the 31 variables values for each quarter. The LSTM model really doesn’t like this format and gives the error:
ValueError: Failed to find data adapter that can handle input: ( containing values of types {“”}), ( containing values of types {“”})
Do you have any advice as to how to format this input into my LSTM? Hope the question is clear and thanks for the help!
Thanks!
Preparing LSTM data can be very tricky, hang in there!
Perhaps these tips will help:
https://machinelearningmastery.com/faq/single-faq/what-is-the-difference-between-samples-timesteps-and-features-for-lstm-input
Hi Jason,
I have a question regarding Multivariate predictions.
Say for example I have two sets of multivariate datasets with parallel input series in both.
How can we use dataset (X) which is multivariate and has parallel input time series , to predict dataset (Y), which again is a multivariate dataset with parallel input series.
Looking forward to your response.
The above examples under “Multivariate LSTM Models” can be used as a starting point and adapted directly.
Hi Jason,
Do you have any example for univariate multi-step time series?
Thanks
Yes many, you can use the search box at the top of the page.
There is an issue with the line
model.add(LSTM(100, activation='relu', input_shape=(n_steps_in, n_features)))
from section ‘Vector Output Model
and 'Encoder-Decoder Model'
since the following exception is thrown
NotImplementedError: Cannot convert a symbolic Tensor (lstm/strided_slice:0) to a numpy array. This error may indicate that you’re trying to pass a Tensor to a NumPy call, which is not supported
`
How can this be resolved
Sorry to hear that you’re having trouble, are you able to check that you are using the latest version of Python, Keras and TensorFlow.
Also, these tips may help:
https://machinelearningmastery.com/faq/single-faq/why-does-the-code-in-the-tutorial-not-work-for-me
Hello Jason,
Thanks for this brilliant blog post. it has really been helpful to me.
However, I have got a real-world Spatio-temporal traffic dataset and I reckon that the procedure to model it as a supervised learning problem would be quite different from multivariate time series (as the order of the spatial variables matter).
As an example: take the Spatio-temporal matrix
T1 T2 T3 T4 T5 T6
S1 | 67 | 34 | 24 | 54 | 49 | 67 |
S2 | 61 | 55 | 23 | 42 | 53 | 78 |
S3 | 74 | 83 | 55 | 50 | 62 | 68 |
S4 | 48 | 73 | 78 | 56 | 61 | 78 |
S5 | 80 | 58 | 67 | 54 | 51 | 89 |
where the rows represent the spatial identity (the position of the detectors) and the columns represent the time interval for collection of the data.
In formulating this as a supervised learning problem with 5 time-step per sample and 1 step prediction made at S3, would this be a logical formulation?
Input:
T1 T2 T3 T4 T5
S1 | 67 | 34 | 24 | 54 | 49 |
S2 | 61 | 55 | 23 | 42 | 53 |
S3 | 74 | 83 | 55 | 50 | 62 |
S4 | 48 | 73 | 78 | 56 | 61 |
S5 | 80 | 58 | 67 | 54 | 51 |
Output:
68
Also, Since I am working with a real Spatio-temporal dataset, do I need to split the samples into subsequences when using the ConvLSTM module?
If No, for the example above, would this input to the ConvLSTM be correct:
[no of samples, time-step=5, rows=spatial, columns=temporal, features=1]
It’s hard to be prescriptive, perhaps experiment and see what works/makes sense for your dataset.
Hi, just a quick question I am working with a multiple multivariate timeseries. Will the structure remain the same as the Multiple Input Series model discussed above?
Yes, see this:
https://machinelearningmastery.com/faq/single-faq/what-is-the-difference-between-samples-timesteps-and-features-for-lstm-input
Hi Jason,
Thank you for such an informative tutorial.
But I’m having problems using the convLSTM module for multivariate time series prediction. I hope you can answer this for me, it is really important for me and I would appreciate it.
My topic is to learn the train dataset to perform outlier detection on the test dataset. If the test set has no outliers, the convLSTM module can predict well. However, when I add outliers, the predictions change and I can’t do outlier detection.I can’t explain it very well.
Only a simple example can be given.
Suppose a feature in my training set is [1, 2, 3, 4, 5, 6, 7]
And the corresponding test set is [8, 9, 10, 11, 11, 11, 14]
Ideally, the prediction generated by learning the train set would be [8, 9, 10, 11, 12, 13, 14], which is used to prove that there are 2 outliers in my test set.But the real situation is that I get predictions similar to[8, 9, 10, 11, 11.1241, 11.3661, 14].
Questions:
1). The data in the prediction set and the test set are too close to each other, so I can’t do outlier detection.
2). How to use the convLSTM module to perform multi-step prediction for multivariate sequences? Because I guess the reason for the first problem is that I am using the convLSTM module for single-step time series prediction.
You’re welcome.
Sorry, I don’t understand your first question sorry. Outlier detection would probably occur prior to modeling as a data prep step.
You can perform multi-step prediction a few ways – all described above, e.g. vector out for an encoder-decoder model each time step.
Hi Jason!
I would like to ask another question. After training a mulitivate lstm model, how do we know if the model is good or not?
You can evaluate the performance of the model on a hold out dataset and calculate a metric, then compare the metric to other methods including a naive method. This will help:
https://machinelearningmastery.com/faq/single-faq/how-to-know-if-a-model-has-good-performance
Hi Jason!
Thanks for your quick reply! However, I probably did not make myself understood. I was struggling with how to know the test error of my multivariate lstm model…
You can calculate test error for an LSTM using walk-forward validation described in this tutorial:
https://machinelearningmastery.com/backtest-machine-learning-models-time-series-forecasting/
Thanks so much! Jason!
You’re welcome.
It’s like YOU HAVE EVERYTHING! You are a treasure!
Thanks.
Hi Jason,
I’m currently working on stock price prediction. As of now, I’ve used historical data of the end-of-day ‘Closing’ prices ONLY as univariate sequences. My aim is further improve the model by giving it more than just old ‘closing’ prices. I want to give it open, high and low too. From your article, I could understand that I can achieve this using Multivariate sequences. I have gained so much knowledge from this and I can make my project even better.
Thanks a lot! I would be really happy if you can give me some tips!
These tutorials may also help:
https://machinelearningmastery.com/start-here/#deep_learning_time_series
Also, I’m pretty sure stock prices are not predictable:
https://machinelearningmastery.com/faq/single-faq/can-you-help-me-with-machine-learning-for-finance-or-the-stock-market
Yes, that’s true. Stock prices cannot be predicted but I’m trying to at least achieve a fair/legit movement in the chart for the future (at the back of my mind I feel, even this is not completely achievable).
Should I continue with this project or just be honest with my faculty saying it is not achievable?
You have the freedom to choose what you work on, I don’t want to interfere with that!
Hey Jason,
So, I’m using the Multistep Univariate method for my problem. Instead of splitting into only X, y; I have split the data into X_train, y_train & X_test, y_test. It’s obvious that X_test will be used to test the model and compare it to y_test for ERROR computing.
For example,
If my y_test looks like [[10],[20],[15],[21]…….[34]]
and my predicted y_test looks like [[11[,[19],[17],[20]…….[34]]
how do I compute mean_squared_error(y_test, pred_y_test)?
Collect predictions in a list or array and then calculate the error with predictions vs expected values.
You must use walk-forward validation to collect predictions, described here:
https://machinelearningmastery.com/backtest-machine-learning-models-time-series-forecasting/
Hello Jason,
Now I know how to develop a Multivariate Multistep forecasting model for the hourly weather forecasting task.
But in case we are also given a day ahead “weather guess” dataset, how can I use these guessed values in a model? do you know any tutorial or blog post?
In fact, we have a history of guesses and a history of actual values.
Then a day ahead guess is passed to us, and we should make an accurate prediction using the history of this guess entity and the actual values
Hi,
thank you for this nice tutorial.
I would like to know how to modify the multivariate multi-step forecasting in order to use keras’ SimpleRNN instead of LSTM.
In particular, I would like to use Elman RNN. I have read that it can be implemented by connecting one SimpleRNN layer with a TimeDistributed(Dense) layer, but it is not clear to me how to do
I have tried the following code:
model = Sequential()
model.add(SimpleRNN(100, return_sequences=True, input_shape=(n_steps_in, n_features)))
model.add(TimeDistributed(Dense(n_steps_out, activation=’tanh’)))
model.compile(optimizer=’rmsprop’, loss=’mse’)
# fit model
model.fit(X, y, epochs=200, verbose=0)
but fit() fails raising the error:
tensorflow.python.framework.errors_impl.InvalidArgumentError: Incompatible shapes: [6,3,2] vs. [6,2]
Thank you in advance
You’re welcome.
Sorry, I don’t have an example, perhaps use a little trial and error and discover how to make the required changes.
Hello Jason,
Is it possible to use LSTM without time. Just for coordinates. Input = coordinates, output = value (like temperature). For extrapolation task or interpolation.
Yes, but it is a bad application. Use Dense.
Actually, I am using your multivariate multi-step example (version with one LSTM layer), just replacing LSTM with SimpleRNN and Dense with RimeDistributed(Dense).
Apparently, the problem is the shape of the y data structure. I made the following change:
X, y = split_sequences(dataset, n_steps_in, n_steps_out)
y = y.reshape(y.shape[0],1,y.shape[1]) # <– added this one
print(X.shape, y.shape)
Now, the model design, train and test is:
# define model (Elman RNN)
model = Sequential()
model.add(SimpleRNN(100, activation="sigmoid", return_sequences=True, input_shape=(n_steps_in, n_features)))
model.add(TimeDistributed(Dense(n_steps_out, activation='tanh')))
model.compile(optimizer='rmsprop', loss='mse')
# fit model
model.fit(X, y, epochs=200, verbose=0)
# demonstrate prediction
x_input = array([[70, 75], [80, 85], [90, 95]])
x_input = x_input.reshape((1, n_steps_in, n_features))
yhat = model.predict(x_input, verbose=0)
print(yhat)
The resulting yhat is:
[[[1. 1.]
[1. 1.]
[1. 1.]]]
which is not good in shape and values. What am I still missing?
Sorry, I have not used “SimpleRNN” and “RimeDistributed”. I don’t know the cause of your problem.
Perhaps these tips will help:
https://machinelearningmastery.com/faq/single-faq/can-you-read-review-or-debug-my-code
RimeDistribuited was a typo, I actually meant: TimeDistributed
I was not asking to debug my code, of course.
Recently, I purchased a couple of your books, which unfortunately do not help me in solving theproblem. I thought you were at least able to provide useful hints – not just a link to the FAQ.
Nevermind, I will find the solution and publish it for free. 😀
No problem, I’m eager to hear how you go.
Hi Jason,
Thanks for the post. It is very helpful.
I created a LSTM model:
model = Sequential()
model.add(LSTM(20, activation=’relu’, return_sequences=True, input_shape=(5,12)))
model.add(Dense(20, activation=’relu’))
model.add(Dense(1, activation=’sigmoid’))
model.compile(optimizer=’adam’, loss=’binary_crossentropy’, metrics=[‘accuracy’])
As you can see the dimention of model output is (1,)
However after training when I run model prediction, I got multiple output:
predictions = model.predict_classes(X_test[0].reshape((1, 5, 12)))
predictions.shape, predictions
Output:
((1, 5, 1),
array([[[0],
[0],
[0],
[0],
[0]]]))
Yes, one output “vector” per sample.
I have a question, if i want to get back the test data used in the model in its original form , so as to plot it against the predicted values with the dates on the x-axis, is there a way to do it ?
You can inverse the transforms applied to the input data.
If you use sklearn to prepare data, you can do it via a inverse_transform() function.
If you prepare data manually, this will help:
https://machinelearningmastery.com/machine-learning-data-transforms-for-time-series-forecasting/
Hello Jason,
I have a question regarding creating samples. I want to create samples for the Closing price for 60 days window but give labels to them. Using this code
from numpy import array
# split a univariate sequence into samples
def split_sequence(sequence, n_steps_in, n_steps_out):
X, y = list(), list()
for i in range(len(sequence)):
# find the end of this pattern
end_ix = i + n_steps_in
out_end_ix = end_ix + n_steps_out
# check if we are beyond the sequence
if out_end_ix > len(sequence):
break
# gather input and output parts of the pattern
seq_x, seq_y = sequence[i:end_ix], sequence[end_ix:out_end_ix]
X.append(seq_x)
y.append(seq_y)
return array(X), array(y)
# define input sequence
raw_seq = df[‘Close’]
# choose a number of time steps
n_steps_in, n_steps_out = 60, 60
# split into samples
X, y = split_sequence(raw_seq, n_steps_in, n_steps_out)
# summarize the data
for i in range(len(X)):
print(X[i], y[i])
I am able to create X samples but for Y samples I want to give them labels 0 and 1. On the condition
X_T: T+1, T+2, …, T+60
Y_T: ==1, if the price increases by 6% before going down 3% within 3 trading days; ==0, otherwise.
How should I do this ?
It sounds like time series classification, if you need help, perhaps the examples here will be useful:
https://machinelearningmastery.com/start-here/#deep_learning_time_series
Hello Jason, Greetings.
Hope you are doing well. Thanks for the post. This is very useful. However, I have a doubt.
How do I choose the optimal time step for my data. or Shall I use ACF or PACF plot to choose the optimal time step? please advise. Thanks in advance
Perhaps ACF/PACF plots will help, perhaps grid search, perhaps trial and error.
Dear Jason,
I appreciate your instructive blog. I lean a lot from you.
I am trying to teach supervised to several LSTMs and then make a Max pooling between their hidden states. Can you help me whether there is such an ability in LSTMs embedded or I need to make it available by myself?
You may need to write some custom code or a custom layer.
Thanks for the reply, Jason.
You’re welcome.
Hello Jason, thanks for this great tutorial!
I have a question and I would be glad if you share your idea.
I have a dataset of frames obtained from a gameplay video and each frame (row) in the dataset has the following columns (in a simplified manner): time, bitrate_kbps, game stage (0: Exploration, 1: Combat)
As an example of random 6 adjacent frames:
2.2, 208, 1
2.3, 211, 1
2.5, 215, 1
2.6, 219, 0
2.7, 222, 0
2.9, 221, 1
My goal is to train a model (e.g. with LSTM) with this time-series data to be able to classify game stages according to the bitrate data. The model should be able to assign the correct game stage labels to the unlabeled time series of frames such: time, bitrate_kbps.
What kind of approach would be a good way to train such a model? Thanks!
Perhaps test a suite of data preparations, model types and model configurations in order to discover what works best.
If you want to use an LSTM, this will help:
https://machinelearningmastery.com/faq/single-faq/what-is-the-difference-between-samples-timesteps-and-features-for-lstm-input
Hi Jason and thanks for your posts!
In your multivariate multi-step stacked lstm example, If I had:
n_steps_in, n_steps_out = 3, 2
and for x_input another one line, so:
x_input = array([[[70, 75], [80, 85], [90, 95]],
[[100, 105], [110, 115], [120, 125]]])
then the output would be:
yhat = array([[182.84283, 212.43597],
[247.65134, 288.84436]], dtype=float32)
Now, let’s say that I have the dates information also
(so all this refers to data in certain dates by every day step).
So for the first data which is on 1/4/21
[[[ 70, 75],
[ 80, 85],
[ 90, 95]] the +1 day value is 182.84283 (2/4/21) and the +2 days is 212.43597 (3/4/21) ?
And for the next set of input which is on 2/4/21
[[100, 105],
[110, 115],
[120, 125]] the +1 day value is 247.65134 (3/4/21) and the +2 days is 288.84436 (4/4/21) ?
But on 3/4/21 I have two values now!
Please, if you want to clarify because I am confused!
Thank you!
You’re welcome.
Perhaps this will help you to get started:
https://machinelearningmastery.com/faq/single-faq/what-is-the-difference-between-samples-timesteps-and-features-for-lstm-input
Hi Jason,
So, since I have 2 samples and 3 timesteps:
1st sample
———–
[[[ 70, 75] -> 1/4/21
[ 80, 85] -> 2/4/21
[ 90, 95]] -> 3/4/21
the output is:
182.84283 is on 4/4/21 and 212.43597 on 5/4/21 , right?
2nd sample
———-
[[100, 105] -> 4/4/21
[110, 115] -> 5/4/21
[120, 125]] -> 6/4/21
the output is:
247.65134 is on 7/4/21 and 288.84436 on 8/4/21, right?
So,I am predicting for 4,5,7,8 of April?
Where is the prediction for 6/4 ?
You can frame the data any way you want.
I think it would be better to shift each sample down by one time step, instead of 3, but you can do whatever you think is best for your dataset and model. If you’re not sure, perhaps try a few different approaches and compare results.
Ok, but what if I have this frame as above?
3 steps in and 2 steps out. How to deal with the dates, that’s my problem.
My point is you can prepare your data so you have [3,4,5]->[6,7] if you want.
Do you have any work about Multiple Parallel Input, Multi-Step Output and Multiple Output for Time Series Forecasting?
The problem I have is that I have 6 features and I want to predict 3 with their respective test and training like the air pollution blog.
Perhaps you can adapt one of the above examples.
Hi Jason,
Thanks for your post, it was very helpful for me to start LSTM.
My problem is to predict a time series, say prices over time, and apart from the historic real prices, I also have some forecasted prices from another source, for the next n time intervals, and I want to use them as additional features.
To test the accuracy of the model, I substitute the forecasted prices with real price. Say I want to predict a price that follows pi: [3 1 4 1 5 9 2 6 7 ..], I use a data input structure look like this:
X[0,:,:] =
[[ 3 1 4]
[ 1 4 1]
[ 4 1 5]
[ 1 5 9]]
Y[0,:] = [5 9]
X[1,:,:] =
[[ 1 4 1]
[ 4 1 5]
[ 1 5 9]
[ 5 9 2]]
Y[1,:] = [9 2]
and so on,
As a test, I used a simple single layer LSTM + a dense layer as output.
model.add(LSTM(10, activation=’relu’, return_sequences=False, input_shape=(4, 3))
model.add(Dropout(0.1))
model.add(Dense(2))
But it seems the current configuration can not figure out there is a relationship between the diagonal element in the input, even the inputs already have the answer. The error is quite large.
Is there any LSTM or other model structure you see will be helpful?
Thank you very much!
MK
I recommend testing a suite of different data preparations, different model types and different model configurations in order to discover what works best for your dataset.
This may help:
https://machinelearningmastery.com/how-to-develop-a-skilful-time-series-forecasting-model/
Hello,
I want to know the significance of the number of steps we use.
In these examples the number of steps used are 3 ? does this mean that every time the LSTM is trained it looks only at the last 3 time steps ?
Does this mean that if we want the LSTM to look over temporal dependencies over a longer time period we need to increase the number of steps accordingly ?
I don’t understand this part.
The configuration was arbitary. I recommend tuning the problem representation and model for your specific dataset.
hello jason
please can you explain the function split_sequence i can’t understand how the function work …
please # gather input and output parts of the pattern
seq_x, seq_y = sequence[i:end_ix], sequence[end_ix] those too lines specifically
This explains the general idea:
https://machinelearningmastery.com/convert-time-series-supervised-learning-problem-python/
thank you so much … you saved my life <3
You’re welcome.
Hey Jason, Needed some help with my project.
I am working on a project to predict future demands.
Its as univariate forecasting. (only two columns i.e. Date and Demand)
I have trained my model for the year 2015-2016 (having the data only of both these year), and want to predict for the year 2017 (the next 365 days).
How can I do this
I recommend starting here:
https://machinelearningmastery.com/start-here/#timeseries
Then here:
https://machinelearningmastery.com/start-here/#deep_learning_time_series
Thanks for this great tutorial, Dr. Jason.
In the univariate LSTM model that uses CNN as feature , you use a kernel of size 1.
model.add(TimeDistributed(Conv1D(filters=64, kernel_size=1, activation=’relu’),
input_shape=(None, n_steps, n_features)))
model.add(TimeDistributed(MaxPooling1D(pool_size=2)))
model.add(TimeDistributed(Flatten()))
The configuration may have been chosen arbitrarily, but the model performed better with kernel size 1. What is the intuition behind this size?
Thanks
It may suggest the CNN is not adding any value to the model.
Hi Jason. I wanna use the last model ” Multiple Parallel Input and Multi-Step Output” for stock prediction, but I face this error: “AttributeError: module ‘tensorflow.python.framework.ops’ has no attribute ‘_TensorLike'”
The code that I have been using is as follows. I exactly copied the code and transformed my data to fit the model but I faced an error.
Thanks
import numpy as np
import pandas as pd
import matplotlib.pyplot as plt
%matplotlib inline
import yfinance as yf
from datetime import date
from dateutil.relativedelta import *
from copy import deepcopy
import pickle
import warnings
warnings.filterwarnings(“ignore”)
from numpy import array
from numpy import hstack
from keras.models import Sequential
from keras.layers import LSTM
from keras.layers import Dense
from keras.layers import RepeatVector
from keras.layers import TimeDistributed
stocks = [‘AAPL’,’TSLA’,’UPS’, ‘FDX’, ‘FB’]
today = date.today()
Initial_period = today + relativedelta(months=-24)
data = pd.DataFrame(columns=stocks)
for s in stocks:
dt = yf.download(s,Initial_period, today)
data[s]= dt.reset_index()[‘Close’].values
# split a multivariate sequence into samples
def split_sequences(sequences, n_steps_in, n_steps_out):
X, y = list(), list()
for i in range(len(sequences)):
# find the end of this pattern
end_ix = i + n_steps_in
out_end_ix = end_ix + n_steps_out
# check if we are beyond the dataset
if out_end_ix > len(sequences):
break
# gather input and output parts of the pattern
seq_x, seq_y = sequences[i:end_ix, :], sequences[end_ix:out_end_ix, :]
X.append(seq_x)
y.append(seq_y)
return array(X), array(y)
# choose a number of time steps
n_steps_in, n_steps_out = 50, 7
# covert into input/output
X, y = split_sequences(data.values, n_steps_in, n_steps_out)
print(X.shape, y.shape)
model = Sequential()
model.add(LSTM(200, activation=’relu’, input_shape=(n_steps_in, n_features)))
model.add(RepeatVector(n_steps_out))
model.add(LSTM(200, activation=’relu’, return_sequences=True))
model.add(TimeDistributed(Dense(n_features)))
model.compile(optimizer=’adam’, loss=’mse’)
Sorry to hear that, perhaps some of these tips will help:
https://machinelearningmastery.com/faq/single-faq/why-does-the-code-in-the-tutorial-not-work-for-me
Hi Jason, I have one question . Can you please check this question?
https://stackoverflow.com/questions/67467590/lstm-timesteps-and-features-selection
Thanks!
This is a common request that I answer here:
https://machinelearningmastery.com/faq/single-faq/can-you-comment-on-my-stackoverflow-question
Ok Jason, so
I am using 6 features and each feature has 7 timesteps, so I have:
feature1(t-7) feature2(t-7) feature3(t-7) … feature6(t-7)… feature5(t) feature6(t) .. feature5(t+1) feature6(t+1)
I am predicting the t and t+1 timesteps.
So, my input data is [?, 7, 42] (6 features * 7 timesteps).
Now, at first I was doing:
X_train = X_train.reshape((X_train.shape[0] , 1 , X_train.shape[1]))
and
nb_timesteps, nb_features = 7, X_train.shape[2]
I want to use 7 timesteps, but as you can see the input data has shape
[?, 1, 42] and not [?, 7, 42]
so, I show a warning about that.
How can I overcome this, if I want to use 7 timesteps?
My solution is to reshape data (after confirming that my length of data is a multiple of 7)
X_train = X_train.reshape((X_train.shape[0] , 7 , X_train.shape[1] // 7))
but now I am using 7 timesteps (ok I want that) and 6 features instead of 42.
I want to ask if this is ok. I mean, with this setup I am using the 6 features for only for the (t-7) step and at the same time I am using 7 timesteps.
Not sure I follow, sorry.
I believe this will help:
https://machinelearningmastery.com/faq/single-faq/what-is-the-difference-between-samples-timesteps-and-features-for-lstm-input
I was just saying that if I do reshape, the data is mixed up.
Then , what features should I place in the last dimension? [samples, timesteps, features].
Should I have all 42 features? (t-7),(t-6)…(t-1) ?
Or should I have 6 features ? And at what time reference? (t-7) , (t-6) .. (t-1)?
I try to avoid being descriptive as I never have all of the details of a reader’s dataset.
I guess it is a design decision, likely based on the native structure of the data you are working with.
The link I provided should help you think it through, otherwise prototype some approaches with pen and paper of some vanilla python and print() the results to see what makes sense.
Hi, I’ve got a silly question but I see variables named like nb_timesteps, nb_features. What does nb actually mean? Thanks!
Hi Sam…I do not see what you are referencing, however there would be significance to it as it is just part of variable name. In other words, you could also just call them…”nx_timesteps”, “ab_features” and the like.
Good evening, thanks for all the material you have published, as a newbie they have been a great help to me. In my case I am working on a time series problem, which consists of the disintegration of residential electrical energy. My problem can be summarized as follows: I have two time series as input, which can be interpreted in a certain way as the sum of the output series. I have the two input data series and 22 output time series. The objective is that once the model receives the two input series, it can reconstruct the 22 series that compose it. Please can you give me a guide between your tutorials and books which may be the most appropriate for my case. Can I reference the book that I purchase? Thank you.
I recommend starting with this framework:
https://machinelearningmastery.com/how-to-develop-a-skilful-time-series-forecasting-model/
Hi Jason,why do i use Encoder-Decoder Model for muti-step forecast(24steps) had bad result? it only can predict the trend for me ,can you help me? thank you very much
It may or may not give a good result for a given dataset. We cannot know beforehand.
Why do you define the input_shape as shape of 2D? What is the difference between input_shape and batch_input_shape?
LSTM input is 3D, this will help:
https://machinelearningmastery.com/faq/single-faq/what-is-the-difference-between-samples-timesteps-and-features-for-lstm-input
Good day Jason, first, thanks for the awesome tutorial.
Second, I have two doubts regarding RNN in general.
1) I have read in some forums that “each sample ‘should’ be of an integer type”, and in others they say that “RNN can deal with series of numbers, no matter the type”. Plus, the examples used in some of your other tutorials (https://machinelearningmastery.com/reshape-input-data-long-short-term-memory-networks-keras/), show some float numbers used inside each sample. Which type is “preferred” for working with RNN?
2) Related with the previous question, I am exploring the behaviour of some DNN architectures for binary classification. I have a mixed-type dataset (with both integer and float number), but I don’t know if I could use it “as is”, or turn them into some specific format (all integer, all float, if they are categories OHE, standardize / normalize)…
I think both question pretty much redundate with each other, but anyways, I want to make sure I am well understood.
Thanks beforehand for your thoughs about my query, and stay safe.
Hello Jason,
I just wanted to clarify of my ‘doubt # 2’, that I am focusing specifically to LSTM-RNN.
Thank you again.
Yes, generally RNNs should take small floats as input.
Try your model on the raw data and compare to scaled data and use whatever works best for you.
The code worked for me with the followin changes,
from tensorflow.keras.models import Sequential
from tensorflow.keras.layers import LSTM
from tensorflow.keras.layers import Dense
I had to install tensorflow==1.12.0 and keras==2.2.4 (my python version is 3.6.8, and I am on Windows 10, no anaconda!)
Hope this is helpful for other people facing problems regarding package incompatibility.
The code works with tf 2.4 and keras 2.4 directly.
Hi Jason,
I tried your CNN-LSTM model and I got an error message. The error message was “ValueError: Please initialize
TimeDistributed
layer with atf.keras.layers.Layer
instance. You passed: ”Would you like to help me solve this error? Thank you
I recommend using the Keras API directly instead of tf.keras.
I was thinking about making a model for multiple separate ( sale forecast of a shop for different product) using a single model. I have found different ways but they are not concrete .I have studied ESRNN lib from github but it seems my data magnitude is too low like :
product_id,date,count
1101,1-5-2020,1
1101,2-5-2020,4
1101,3-5-2020,0
1101,4-5-2020,0
1101,5-5-2020,4 ….
Is it possible to add embedded layer to parse the id then using the split_sequences method of yours to train a model that works for all product .
This might give you ideas:
https://machinelearningmastery.com/faq/single-faq/how-to-develop-forecast-models-for-multiple-sites
Thank you for answering my question.
Sir, I am really keen to learn details about this steps (you have referred).
Is there any other details options .where I can read few papers/books to learn more about this. eg I don’t have any idea about the Ensemble method .
I have used .
1. Hands-On Machine Learning with Scikit-Learn, Keras, and TensorFlow: Concepts, Tools, and Techniques to Build Intelligent Systems
**[1] Where I found the concept of adding a custom layer .But I am afraid for large number of product (sites in your cities weather example) I need an enormous network .And it may not work properly.
These tutorials will help:
https://machinelearningmastery.com/start-here/#deep_learning_time_series
Thanks Jason for the tutorial. I have a question regarding the Multiple Input Multi-Step Output. You use the last 3 timesteps of the 2 time series [(10, 15); (20, 25); (30, 35)] to predict the next 2 timesteps [65,85]. Basically the 65 is from the same timestep as the (30,35). So why would you want to predict a value from a timeslot that you have already observerd (otherwise you would not have the input (30,35))? Would it not make more sense to predict the next 2 timeslot after the time slot with the (30,35) which led to 65? So basically you should predict [85, 105] when having [(10, 15); (20, 25); (30, 35)] as input.
I’d appreciate every comment and would be quite thankful for your help.
We are evaluating the model using walk-forward validation.
Once you choose a model and config, you fit the model on all data and start making predictions on new data.
Perhaps this will help:
https://machinelearningmastery.com/make-predictions-long-short-term-memory-models-keras/
Thanks Jason for your answer, I know that you are using a walk-forward validation and I know how this works. This was not the point of my question. I am wondering why you forecast the values of the same timeslot for which you have the inputs? Normally you should forcast the values of the NEXT timeslot because this is – by definition – what a forecast is supposed to do.
You are forecasting the output (Timeseries_3) of Timeslot_3 which is 65 using – amongst others – the inputs of Timeslot_3 (Timeseries_1:30 and Timeseries_2: 35). For me this does not make sense. Surely it makes sense to forecast Timeseries_3 of Timeslot_4 because this is a future value while Timeseries_3 for Timeslot_3 is not a future value when you are in Timeslot_3.
So why do you not use Timeslot_1, Timeslot_2 and Timeslot_3 to forecast Timeslot_4 and Timeslot_5? You are using Timeslot_1, Timeslot_2 and Timeslot_3 to forecast Timeslot_3 and Timeslot_4
Timeslot_1: Timeseries_1: 10, Timeseries_2: 15, Timeseries_3: 25
Timeslot_2: Timeseries_1: 20, Timeseries_2: 25, Timeseries_3: 45
Timeslot_3: Timeseries_1: 30, Timeseries_2: 35, Timeseries_3: 65
That was the framing of the problem I was solving. You can frame the prediction problem anyway you like.
Ah okay. Thanks a lot for your tremendous help. I really appreciate it.
Hello,
The LSTM is well modeled my time series with acceptable errors.
However, the forecasting value (after the test set of my real time series) are very far from what is called normal data.
Is it normal?
can you tell me more.
Perhaps you need to prepare the data prior to modeling?
Perhaps you need to tune the model?
Perhaps the model is not appropriate for your dataset?
Hello,
I have about to use LSTM for a price prediction case, but i gave addition data like, Age, Region, Town, payment method, different date (First and last payment) and so on.
I want to know, if i will be able to use those those for LSTM model, This is my first project on NN.
Thank you
Perhaps try it and see.
Hi Jason, thank you for the informative and detailed tutorials! I noted that you use the ‘relu’ activation function for the LSTM layers instead of the default ‘tanh’ activation. May I ask why? Thank you!
Sometimes it is more effective.
Thank you very much for your reply! Sorry, but could you please clarify in what way it is more effective, and in what cases it might be preferred? Thank you!
I noticed empirically on some problems that using RELU for some simple univariate time series was more effective.
I recommend that you test a suite of model configurations and discover what works best for your specific dataset and model.
Thank you for your clarification!
You’re welcome.
Thank you for your informative post!
I have a question for ‘Multiple Input Multi-Step Output’ process.
when I trained, I’d like to add validation set.
is it a good way to add validation set?
and if it is, how can I set?
is it right to split train/validation/set disjointly??
Thank you in advance!
I don’t think using a validation set with an LSTM model is appropriate.
Can I ask why?.. I’m lack of information about CNN or LSTM yet…
Because we cannot perform walk-forward validation on future time steps and use the same time steps (or different future time steps) for validation.
Hi Jason,
In your example Multivariate Multi-Step LSTM Models->Multiple Input Multi-Step Output,
where you use n_steps_in, n_steps_out = 3, 2 , if we use for example sigmoid for the last layer and binary crossentropy loss:
n_steps_in, n_steps_out = 3, 3
X, y = split_sequences(dataset, n_steps_in, n_steps_out)
n_features = X.shape[2]
model = Sequential()
model.add((LSTM(5, activation=’relu’, return_sequences=True, input_shape=(n_steps_in, n_features))))
model.add(Dense(1, activation=’sigmoid’))
model.compile(optimizer=’adam’, loss=’binary_crossentropy’, metrics=[‘accuracy’])
model.fit(X, y, epochs=20, verbose=0, batch_size=1)
it runs ok.
BUT, if we use n_steps_in, n_steps_out = 3, 2, it gives:
ValueError: Dimensions must be equal, but are 2 and 3 for ‘{{node binary_crossentropy/mul}} = Mul[T=DT_FLOAT](binary_crossentropy/Cast, binary_crossentropy/Log)’ with input shapes: [1,2], [1,3].
Any ideas what is that and how to deal with it?
Thank you!
Sorry, it’s not clear what the issue may be. You may need to use a little trial and error in adapting the model for your specific use case.
hi Jason, thanks for the tutorial, that’s very helpful, I found that by changing the batch_size in the predict() method, the prediction values change (I used your # univariate stacked lstm example and just changed the batch_size in the predict() method below)….
yhat values are almost the same as yhat1 (because the default batch size 32 is similar to 41), but yhat2 values differ a lot from yhat1 and yhat…..since it is a stateless lstm, how come changing the batch size in predict method change the prediction values?
i really appreciate your time and help in advance 🙂
# univariate stacked lstm example
from numpy import array
from keras.models import Sequential
from keras.layers import LSTM
from keras.layers import Dense
from keras.utils import plot_model
# split a univariate sequence
def split_sequence(sequence, n_steps):
X, y = list(), list()
for i in range(len(sequence)):
# find the end of this pattern
end_ix = i + n_steps
# check if we are beyond the sequence
if end_ix > len(sequence)-1:
break
# gather input and output parts of the pattern
seq_x, seq_y = sequence[i:end_ix], sequence[end_ix]
X.append(seq_x)
y.append(seq_y)
return array(X), array(y)
# define input sequence
raw_seq = list(range(1,65))
# choose a number of time steps
n_steps = 2
# split into samples
X, y = split_sequence(raw_seq, n_steps)
# reshape from [samples, timesteps] into [samples, timesteps, features]
n_features = 1
X = X.reshape((X.shape[0], X.shape[1], n_features))
# define model
model = Sequential()
model.add(LSTM(50, activation=’relu’, return_sequences=True, input_shape=(n_steps, n_features)))
model.add(LSTM(50, activation=’relu’))
model.add(Dense(1))
model.compile(optimizer=’adam’, loss=’mse’)
# fit model
model.fit(X, y, epochs=200, verbose=0)
plot_model(model)
# demonstrate prediction
x_input = array(list(range(2,166)))
x_input = x_input.reshape((-1, n_steps, n_features))
yhat = model.predict(x_input, verbose=0, batch_size=41)
yhat1 = model.predict(x_input, verbose=0)
yhat2 = model.predict(x_input, verbose=0, batch_size=2)
and yhat != yhat2 != yhat1
The model will make different prediction each time it is fit, and this is to be expected:
https://machinelearningmastery.com/faq/single-faq/why-do-i-get-different-results-each-time-i-run-the-code
Once fit, it will generally make the same predictions each time, give or take interactions between samples within a batch.
em..just a follow up commet, the difference are quite minor (probably can be ignored):
yhat2[-1]
Out[3]: array([169.57353], dtype=float32)
yhat1[-1]
Out[4]: array([169.57355], dtype=float32)
yhat1[-2]
Out[5]: array([167.4769], dtype=float32)
yhat2[-2]
Out[6]: array([167.47688], dtype=float32)
yhat2[-4]
Out[7]: array([163.28676], dtype=float32)
yhat1[-4]
Out[8]: array([163.28674], dtype=float32)
Hmm, if the model is already fit, it may be the interactions between samples within a batch. That’s my best guess.
You could take control over when internal resets occur and find the best configuration for your problem (e.g. stateful=True).
https://machinelearningmastery.com/stateful-stateless-lstm-time-series-forecasting-python/
HI Jason,
Can you give me an idea on how to choose the time steps for lstm model used for fault detection and diagnosis of time series data with 7 faults and normal condition data labeled within data set. I have decided to go with 8 time steps since there are 8 types of conditions(7 faults and normal). 8 time series .
Finally i want to send last 10 data points to the predict function and return the condition( fault type or normal). Multiple data points as input, predicts the class label based on the input data points. A multi-class classification problem.
Thanks
Perhaps you can test a suite of configurations and discover what works best for your specific dataset.
Hi Jason,
This is a great article. Can we use LSTM to impute missing data in time series?
Yes, perhaps try it and compare results to other methods.
Hey Jason,
Thanks for these fantastic blogposts!
I used a lot of your inputs to develop the code for my thesis – Forecasting carbon market prices with Bayesian and Machine Learning methods. I performed 1step and 4step ahead forecasts with a multivariate (6 covariates), direct rolling window forecast with 3 models to compare:
1) normal linear regression
2) a shrinkage time varying parameter model (shrinkTVP in R)
3) LSTM model (from your blogposts)
I am still finalizing the results and will post them here to compare the performance between these models over time. I use weekly data from 2013-2020. Let me know if you are interested in something particular / if there is something that would help this community most.
Really big thank for the great resources – I am an economist and will continue to use all the resources here to advance econometric methods!
Well done!
Sharing may help other people using the same methods or working on the same problem.
Hi Jason,
Thank you for providing such a good article for us!
In the process of learning LSTM,I encountered some doubts.I hope to get your advice.
I find that the predicted value lags behind the actual value.It’s like the curve of the actual value make parallel movement to the curve of the predicted value.What is the cause of this phenomenon? Is there any solution?
I hope to hear from you soon.
This problem is common, see this:
https://machinelearningmastery.com/faq/single-faq/why-is-my-forecasted-time-series-right-behind-the-actual-time-series
Thank you very much for your reply.
If it’s just a lag in part of trend,rather than it’s not that the predicted value at time t+1 is exactly the same as the actual value at time t. Can I assume that the prediction I’m doing is not the Persistent Algorithm (the “naive” prediction)?
I have another question. How to determine the value of n_steps?
Not sure I follow, sorry.
You can try different window sizes for input and discover what works best for your specific model and dataset.
OK, thank you for your reply. I hope I can learn more from your article.
If you can learn more about lag, I hope you can tell me. I will be indebted forever.
You can vary the amount of lag used as input in order to discover what works well or best for your specific dataset and model.
Thank you very much. I will try as you say.
You’re welcome.
Hi Jason,
I have a question regarding the splitting of data for multivariate analysis.
According to the book Deep Learning for Time Series Forecasting Predict the Future with MLPs, CNNs and LSTMs in Python for the following example:
time, measure1, measure2
1, 0.2, 88
2, 0.5 89
3, 0.7 87
The data can be converted into supervised series as follows:
time, measure1, measure2
1, ?, 88
2, 0.2, 89
3, 0.5 87
4, 0.7, ?
Which means the first and last rows fall off.
However, in your multivariate example for this dataset and window = 3
[[10, 15, 25]
[ 20, 25, 45]
[ 30, 35, 65]
[40, 45, 85]
[50, 55, 105]
[60, 65, 125]
………………..]]
When given an input of:
10, 15
20, 25
30, 35
The output is :
65
85
Shouldn’t the output be [85, 105], assuming the first set data value [65] falls off as the case in the first example.
I also reran the same example with window size 1, and for the first row of data [10,15] the output was 25, but should it be 45 instead, given that there is no previous data to predict 25 and the first row should fall off ?
This is the split function I am using :
def msplit_sequence(sequences, n_steps_in, n_steps_out):
X, y = list(), list()
for i in range(len(sequences)):
# find the end of this pattern
end_ix = i + n_steps_in
out_end_ix = end_ix + n_steps_out-1
# check if we are beyond the dataset
if out_end_ix > len(sequences):
break
# gather input and output parts of the pattern
seq_x, seq_y = sequences[i:end_ix, :-1], sequences[end_ix-1:out_end_ix, -1]
X.append(seq_x)
y.append(seq_y)
return array(X), array(y)
Looking forward to your response.
Each problem has different requirements and expectations. You can define the input and output of your problem any way you like.
Good day
Searching Google came across your blog is very interesting, I’m a beginner just starting to learn prediction….
Needed to know , can this be done:
Race : 1
5 runners, 400m track race
1. At 100m , 10.5s / 200m, 19.8s/300m,30.1s/400m, 43.5s
Then runner 2,
Then runner 3,
Then runner 4
Then runner 5.
All with times at 100m, 200m,300m,400m individually performed
Can I predict who’ll be 1st/2nd/3rd/4th with new predicted times for each runner at 100m/200m/300m/400m intervals.
Race :2
Another scenario i have 400m race, only 300m sectional 30.1s then for each runner with their individual times achieved and final time 43.5 then for each runner have their 400m times achieved , can i still predict predicted intervals of each runner and each runners position at 100/200/300/400m is this possible ?
Can result be in this format ?
Example
100m – 5, 10.5s / 3, 10.3s /1, 09.9s/4, 10.2s / 2, 11.3
200m- same like 100m calculations
300m- same like 100m calculations
400m – same like 100m calculations
Appreciate your assistance….
Await your response
Thanks
Hi Dion…Please narrow your query to single question so that we may better assist you.
Hi Jason,
I’m new to Time Series Forecasting. I would appreciate your help. I am currently trying to predict how much a person drinks each day. I have timestamps every 30 minutes and a corresponding value that represents the drunk amount within those 30 minutes. You can already imagine I have a lot of 0 values in the middle. Moreover, a person only drinks from 8AM until 8PM but the data nevertheless spans the whole day (So always 0s from 8PM until 8AM the next day and 1 day is 48 entries). I have also another version of the dataset where the data spans only 8AM till 8PM (1 day is 24 entries).
I already tried Croston’s Method but I am trying to have a dynamic solution, I am trying to implement a Neural Network for this. Would you point me to the right direction? Will LSTMs for example work for intermittent data? Which version of the data would make the model less complicated?
Ps: Your blog is extremely helpful, thanks a lot.
Best,
Omar
I would recommend testing a suite of different framing of the problem, different models, different configurations until you find a technique that works well for your dataset.
I have a dataset timeseries forecasting that includes the categorical columns and numeric as well.
here is a sample of it
Date | categorical _fature_1 |categorical _fature_2| Feature_1_numeric | feature_2_numeric | price
1-1-2020 | USA | A | 5.5 | 7.6 | 100
1-1-2020 | USA | B | 8.3 | 1.7| 20
1-1-2020 | USA | C | 3.6 | 2.1 | 17
1-2-2020 | USA | D | 5.5 | 7.6 | 40
1-2-2020 | USA | E | 77.5 | 35 | 22
1-2-2020 | USA | F | 69.5 | 2 | 22
as you can see in the sample in the date lets pick up the **1-1-2020** we have multiple observations at the same date .
i want to predict the **Price** column as a **Y_label** and taking the **categorical _fature_1**, **categorical _fature_2**, **Feature_1_numeric**, and **Feature_2_numeric** as the **X_features**
so from my understanding as im using **multiple features** for time series Forecasting predicting the **Price** column this is called **Multivariate Time-Series Forecasting**
My Question is
1-how can i manage the multiple observations at the same time from the different features as we saw for example in **1-1-2020** we have **three** different observations
2-i believe if we have multiple observations at the same time/date then we have a new kind of Time-series forecasting what is it Multi-timestep Multivariate Time-Series Forecasting or what ???
thanks
Perhaps you can test different framings of the problem and discover what works well or best for you, e.g. multiple-input model vs treating the observations as separate time steps.
Hi Jason , thank you for your amazing tutorial. I have a dataset that contains test results and multiple features for multiple users. for example
date | user_Id | feature _1| feature _2| test_output
1-Jan-2020 | A | 5.5 | 7.6 | 100
2-Jan-2020 | A | 8.3 | 1.7 | 20
3-Jan-2020 | A | 3.6 | 2.1 | 17
1-Jan-2020 | B | 5.5 | 7.6 | 40
2-Jan-2020 | B | 77.5 | 35 | 22
3-Jan-2020 | B | 69.5 | 2 | 22
I want to predict the output for the next day, and I want to achieve it using LSTMs if possible and all suggestions are welcome.
I want to train my model with multiple users so that it can predict the output for any given user(unseen user) in the next day and i could not find a way to create/reshape my data before feeding it into LSTM
A quick way is to use groupby() in dataframe to create a subset on each user, then set target to be dataframe[“target”]=dataframe[“feature”].shift(-1) so you can see the next-period data as a column. Is that what you mean by reshape?
thank you for your reply
1- i want to understand and visualize the data preparation process (as in the examples above) before feeding it into the lstm model and how can i deal with such data as i mentioned it is related to multiple users.
2- shouldnt i add the output in the “next-period” column instead of the features ?
dataframe[“target”]=dataframe[“output”].shift(-1) ?
3- if i want to generally prepare my code to deal with multistep forecasting, what changes should i modify in any of the above illustrated examples
You’re correct for (2). For (1), I don’t see any issue with multiple users here. You still train the model the same way as long as you do not mix the data from different time series. For (3), that depends on your design. One way is to feed the LSTM output back into the input so we can predict for one more step, then repeat for yet one more step, etc.
regarding point 1 , can you explain what do you mean by(as long as you do not mix the data from different time series) and how can i make sure that i am not mixing the data during the training phase. in other words how can i make sure my model understands that there are multiple users that shares the same time series
Hi Jason:
I have a concern, in the case of using an LSTM for the forecast of time series of the Multiple Parallel Input and Multi-step Output type, Vector Output and Encoder-Decoder LSTM can be used, but, in both cases can also be used Vanilla LSTM, Stacked LSTM, Bidirectional LSTM, CNN-LSTM and ConvLSTM?.
Thanks for your attention.
Yes, there are different variations of LSTM. All have the feature that they can learn and remember the state, but each variant will have some subtle differences.
Hello Jason:
I would like to know, if I want to make the forecast for a time series of Multiple Parallel Input and Multi-step Output type, using an LSTM Encoder-Decoder, to obtain multivector output. Could I do the following?:
Configure the Encoder in any of the following ways:
Vanilla LSTM
Stacked LSTM
Bidirectional LSTM
CNN-LSTM
ConvLSTM
And, configure the Decoder in any of the following ways:
Vanilla LSTM
Stacked LSTM
Bidirectional LSTM
CNN-LSTM
ConvLSTM
And do any combination of LSTM Encoder-Decoder settings to get my multi-step, multi-vector forecast?
Or are there any of these combinations that I cannot do for an LSTM Encoder-Decoder?
Thanks for your attention.
All seems possible. Did you tried anything?
Hi Adrian, yes, now that you mention it, I’m testing each of these combinations.
Thank you so much.
Hello Adrian
Doing these tests, I would like to ask you… really in an LSTM Encoder-Decoder model could I really use a CNN-LTSM model or a ConvLSTM model with the Decoder?
I ask this because these two models use an input with specific characteristics and in the case of being used as Decoders, the input comes with a RepeatVector layer that does not correspond to the input form for a CNN-LSTM model or a ConvLSTM model.
Thanks for your attention.
Hi Jason,
I’m trying to learn how LSTMs actually work under the hood (as opposed to how to use them). One very confusing point is this: What exactly is an LSTM unit? There seems to be contradictory definitions in the literature. In particular, referring to your very first example in which you separate a 10-long integer sequence into six sets of three consecutive terms with the next term as the desired output, the best interpretation I have come up so far is that by a “unit” you mean a set of six LSTM cells wired in series, where each cell takes a 3-dimensional vector as input and outputs a scalar. Here a “cell” is the usual collection of 4 (or 3 depending again on murky definitions) gates. So there wold be a total of 6×50 = 300 cells all wired up in series, and all having the same set of affine parameters (weights and biases). Another unanswered question then is: what is the dimension of the state vector?
It would be great if you could notify my email when you respond, or better yet, copy your response to my email.
Thanks so much for any help!
In Keras, there are no cells, just units/nodes. Or a cell is a unit is a node.
Hello Adrian
Doing these tests, I would like to ask you… really in an LSTM Encoder-Decoder model could I really use a CNN-LTSM model or a ConvLSTM model with the Decoder?
I ask this because these two models use an input with specific characteristics and in the case of being used as Decoders, the input comes with a RepeatVector layer that does not correspond to the input form for a CNN-LSTM model or a ConvLSTM model.
Thanks for your attention.
Sorry I was in the wrong place to ask this question. I appreciate it being deleted from this place, because I already asked it in the correct question.
No worries Liliana!
Thank you for this clear and helpful tutorial.
Thanks a lot! Amazing tutorial.
Glad you like it!
def split_sequence(sequence, n_steps):
X, y = list(), list()
for i in range(len(sequence)):
# find the end of this pattern
end_ix = i + n_steps
# check if we are beyond the sequence
if end_ix > len(sequence)-1:
break
# gather input and output parts of the pattern
seq_x, seq_y = sequence[i:end_ix], sequence[end_ix]
X.append(seq_x)
y.append(seq_y)
return array(X), array(y)
# define input sequence
raw_seq = [2456, 1829, 2141, 1362, 1634, 1241, 1617, 1434, 2279, 1131,
1192, 1065, 725, 997, 1161, 2033, 1815, 1123, 1136, 929, 1340,
1476, 1962, 2199, 1276, 1351, 1201, 1078, 1397, 2181, 2042, 1117,
1284, 1114, 1416, 1163, 1931, 1753, 1073, 1168, 1022, 1251, 3167,
3958, 4002, 2033, 1362, 1099, 1506, 1614, 2838, 2569, 1708, 1536,
1443, 1734, 1970, 2755, 3101, 1790, 1223, 1369, 1651, 2101, 3255,
2559, 1711, 1738, 1612, 1878, 2064, 3504, 3855, 3425, 2829, 2846,
4503, 4300, 4099, 3829, 1694, 1633, 1579, 2404, 2520, 4544, 4435,
2227, 2173, 1690]
# choose a number of time steps
n_steps = 7
# split into samples
X, y = split_sequence(raw_seq, n_steps)
# reshape from [samples, timesteps] into [samples, subsequences, timesteps, features]
n_seq = 1
n_steps = 2
n_features = 1
X = X.reshape((X.shape[0], n_seq, n_steps, n_features))
# define model
model = Sequential()
model.add(TimeDistributed(Conv1D(filters=64, kernel_size=1, activation=’relu’), input_shape=(None, n_steps, n_features)))
model.add(TimeDistributed(MaxPooling1D(pool_size=2)))
model.add(TimeDistributed(Flatten()))
model.add(LSTM(50, activation=’relu’))
model.add(Dense(1))
model.compile(optimizer=’adam’, loss=’mse’)
# fit model
model.fit(X, y, epochs=500, verbose=0)
# demonstrate prediction
x_input = array([4300, 4099, 3829, 1694, 1633, 1579, 2404])
x_input = x_input.reshape((1, n_seq, n_steps, n_features))
yhat = model.predict(x_input, verbose=0)
print(yhat)
Sir, i tried replicating your code and change the n_steps to 7 but it gave me this valueerror ValueError: cannot reshape array of size 581 into shape (83,2,2,1). what should i do? sorry i am very new. thank you. 🙁
you redefined n_steps to 2 later on.
Jason, thank you for your great post.
I am just wondering whether this one can be used to predict non-parallel series problem
for example:
out_seq = array([in_seq1[i-10]+in_seq2[i-5] for i in range(len(in_seq1))])
I tried in_seq1, seq2 as random noise to pred out_seq. The whole purpose is to let the network to learn the hidden mapping btwn different lagging seq1/seq2. Result is not good. Any idea on how to tackle this kind of problem, or did I miss sth.
Garbage in garbage out. If your input is random noise, usually the result would not make sense.
Jason, thank you for your helpful post.
I am a phd student . I used Bidirectional LSTM with CNN to forecasting solar Energy . I got good accuracy when compared my result with another model with same dataset, but I need some advice to make contributions on model.
Hi Jason,
I focus on your website from 2018. Your website has benefited me a lot .Thank you very much for sharing these tutorials and code publicly.
I used convlstm for spatial -temporal forecast , I my dataset is [2880, 6], 6 is spatial dot, 2880 is time series.
n_features = 6
n_seq = 6
n_steps = 2
model.add(ConvLSTM2D(filters=6, kernel_size=(6,2), activation=’relu’, input_shape=(n_seq, 6, n_steps, n_featurs)))
But meet the error:
ValueError:
Input 0 of layer sequential is incompatible with the layer: expected ndim=5, found ndim=3. Full shape received: [None, 5, 6]
I can not find the a solution,would you like to give me any advice? Thanks !
ndim=5 because you set “input_shape=(n_seq, 6, n_steps, n_featurs)” and ndim=3 refers to you input dataset. I think you need to check how you shape your input and passed int the network.
This was a great tutorial, the most comprehensive one out there. Thank you for your work. I have one question, do you have a comparison between the time series prediction NN algorithims, is there any better than LSTM?
I don’t think any comparison would be absolutely fair, but more on which problem fits which model. For the question on LSTM, people have seen GRU as a faster alternative but not always better.
Hi Jason,
Thanks a lot for this wonderful tutorial. Extremely helpful for me!
I have a query regarding the input shape to LSTM model. I would like to provide 8 dimensional time series (i.e. 8 features) where each time sample has a label (or output) associated with it. So, I want the network to learn the mapping from the time series to label series (where time series features also have temporal dependencies). For example- let’s say I have 10000 x 8 length of input series, and 10000 x 1 is the corresponding output size. Now if I set time_steps=10, and feat_size=8, I will have (1000, 10,8) as size of input and (1000,10) as size of output. How can I train LSTM for this ? Should I set return_seq as True and it will take care of learning map from feat to corresponding label ? I am not sure if I am correct here and would like to know if this approach is fine. Thanks again!
If you set return_seq as True, your output is (1000,10) but if it is false, you still have (1000,1). The sequence length in LSTM just means for this many step you will reset the memory.
Thank you for this clear and helpful tutorial,
what if i need to work on csv data as input instead of sample data as above ?
For example, use pandas the read CSV and then extract a column as numpy array?
Hi Dalia…The following tutorial will guide you through some examples of loading csv data.
https://machinelearningmastery.com/basic-data-cleaning-for-machine-learning/
Regards,
Hi
This blog is super helpful, thank you!
I am really stuck on this matter and maybe you could help me?
I have 500 number of different observations in the shape of (100,2). (100 data points, 2 features)
I am reshaping my data to predict 5 time steps ahead based on past 3 time steps. so, after reshaping my data I have
input_shape = (94,3,2)
output_shape=(94,5,2)
but because I have 500 different observations I essentially have the data in the shape of,
input_shape = (500,94,3,2)
output_shape=(500,94,5,2)
the only way I could train my model is by using a for loop to feed each of the 500 observations.
is there a better way to do this?
You’re wrong on the shape here. Your LSTM is predicting with 3 steps and 2 features, then your input is (N,3,2). You should combine the 500 observations together.
Can you please tell me how did you consider the below values:
I understood it for 3 timesteps for input and 1 for output but not the below one’s.
n_steps_in, n_steps_out = 3, 2
n_features = X.shape[2]
For example you have data [10, 20, 30, 40, 50, …] it means you use [10, 20, 30] to predict [40, 50], hence you use 3 steps in input and 2 steps in output. In this case, each time step is a single number, hence the n_features is 1.
Hi Bharathi…Could you please post the exact code block you have questions about?
-Regards,
Hi Jason
Alex is my name :I’m looking for an algorithm such as Multi-Modal Deep Prediction Model using LSTM
Can you explain what do you mean by the multi-model prediction?
Hi Alex…Please explain more about what you are specifically trying to accomplish.
Hi Jason,
amazing post thanks a lot for it! super, super!
I would have a question if you do not mind.
I have a dataset of 100 financial indices.
I want to make prediction of 1 or more samples ahead (doesnt matter).
However, since my variables share some information (common variance) there is some redundancy therefore I would like to compress my dataset same as a PCA or a factor analysis does, but I want to use the LSTM Autoencoder (or how you call it here Encoder-Decoder Model).
The point is that I want to run the autoencoder as you coded here, however what I would keep at the end are the compressed variables at the bottleneck of the autoencoder (end of the encoder), so remove the decoder, and make a prediction only on those compressed set..
because i believe those compressed variables can represent better my dataset (removing redundancy)
This would be also useful for denoising (I would let the hyperparameter tuning to choose the dimension of the bottleneck).
Do you have a reference for coding this?
Or can you briefly indicate me please how to modify your Encoder-Decoder Model?
My idea is that the code you show here during the training will be the same but there must be a modification to add such as the number of dimention of the bottleneck (which I cannot see in your code), and the predict() which has to be run using the model without the decoder
Many thanks in advance
Luigi
Hi Luigi…I appreciate the kind words! I would be able to help you better if you could direct any questions to specific code listings and examples provided machinelearningmastery.com.
Regards,
Hi James,
thanks for willing to help me.
I found your post https://machinelearningmastery.com/lstm-autoencoders/
more relevant to my case so I will open/continue the discussion in there if you don’t mind
Thanks again for your offer to help, very kind
Luigi
Hi Luigi…You are very welcome! Yes, please feel free to continue the discussion in indicated post.
Regards,
Hello, Sir! Thanks for your explanation. I want to ask about ConvLSTM. Can I use it for weather data that have spatial and temporal features that have extention grib2 or nc? We can get spatial features from the longitude and latitude and temporal from the time. I want use it that data for predict the rain. And also, Can I use ConvLSTM for predict the probabilistic?
I hope you’ll answer my question, thank you Sir.
Hi Mocha…a CNN may also work well for your application.
https://machinelearningmastery.com/how-to-develop-convolutional-neural-network-models-for-time-series-forecasting/
Thanks for your answer, Sir!
But, can I still use Conv-LSTM or just LSTM? Because my data aren’t image, Sir.
How can I understand the way to build the model?
I mean, how many LSTM for example? How many dense layers? dropout?
I have a multivariate time series with 5 features
Hello Jesu…More nodes and layers means more capacity for the network to learn, but results in a model that is more challenging and slower to train.
You must find the right balance of network capacity and trainability for your specific problem.
There is no reliable analytical way to calculate the number of nodes or the number of layers required in a neural network for a specific predictive modeling problem.
My general suggestion is to use experimentation to discover what configuration works best for your problem.
This post has advice on systematically evaluating neural network models:
How to Evaluate the Skill of Deep Learning Models
Some further ideas include:
Use intuition about the domain or about how to configure neural networks.
Use deep networks, as empirically, deeper networks have been shown to perform better on hard problems.
Use ideas from the literature, such as papers published on predictive problems similar to your problem.
Use a search across network configurations, such as a random search, grid search, heuristic search, or exhaustive search.
Use heuristic methods to configure the network, there are hundreds of published methods, none appear reliable to me.
More information here:
How to Configure the Number of Layers and Nodes in a Neural Network
Regardless of the configuration you choose, you must carefully and systematically evaluate the configuration of the model on your dataset and compare it to a baseline method in order to demonstrate skill.
Hello Jason
Doing these tests, I would like to ask you… really in an LSTM Encoder-Decoder model could I really use a CNN-LTSM model or a ConvLSTM model with the Decoder?
I ask this because these two models use an input with specific characteristics and in the case of being used as Decoders, the input comes with a RepeatVector layer that does not correspond to the input form for a CNN-LSTM model or a ConvLSTM model.
Thanks for your attention.
Hi Liliana…You should try both and compare the results in my opinion. Also, it would be a good idea to try SARIMA. Sometimes it even outperforms newer deep learning methods!
https://machinelearningmastery.com/sarima-for-time-series-forecasting-in-python/
Yes, I have already tried it and I have the problem that I describe, that is to say that I cannot make the CNN-LSTM and the ConvLSTM serve as a Decoder due to the form of input they require, which is not like the one provided by the previous layer of the model which is a Repeat Vector layer, hence my question, actually, can I use these models as a Decoder?
Thanks for the advice, already use a VAR model.
I am attentive, thank you.
how about this,
i have a time series data (2 years) with one variable (amount per day). And i want to predict based on that data. How to do that?
*i’m 100% newbie
Hi Dwiki…The following are excellent resources:
https://machinelearningmastery.com/introduction-to-time-series-forecasting-with-python/
https://machinelearningmastery.com/deep-learning-for-time-series-forecasting/
Hello Jason, great tutorial as always!
I am having trouble finding any sensible result in my LSTM algorithm. I am trying to use Early Stopping and Model Checkpoint together but when I try to monitor validation accuracy for model checkpoint, validation accuracy becomes zero and does not improve over epochs. I changed the monitor parameter to validation loss and now validation loss seems to be very high. After model completes training, the results are zero for both train and test accuracies.
I am thinking if I made a mistake seperating the dataset into train and test datasets because in your article you mention that datasets should be in a certain format to use LSTM.
Hi,
Thank you so much for the thorough tutorial.
As for the out_seq, I see in almost all examples that is a summation of the input_seqs. I understand these are examples. But what if you know there is a dependency between the in and out seqs but u do NOT know what it is exactly. Then how do you set his up? Any tips? thanks
Hello, thanks for tutorial.
I tried to use a Vector Output to model your last example (Multiple Parallel Input and Multi-Step Output) instead of an encoder-decoder model, but I keep getting an error.
Here’s the code.
# split a multivariate sequence into samples
def split_sequences(sequences, n_steps_in, n_steps_out):
X, y = list(), list()
for i in range(len(sequences)):
# find the end of this pattern
end_ix = i + n_steps_in
out_end_ix = end_ix + n_steps_out
# check if we are beyond the dataset
if out_end_ix > len(sequences):
break
# gather input and output parts of the pattern
seq_x, seq_y = sequences[i:end_ix, :], sequences[end_ix:out_end_ix, :]
X.append(seq_x)
y.append(seq_y)
return array(X), array(y)
# define input sequence
in_seq1 = array([10, 20, 30, 40, 50, 60, 70, 80, 90])
in_seq2 = array([15, 25, 35, 45, 55, 65, 75, 85, 95])
out_seq = array([in_seq1[i]+in_seq2[i] for i in range(len(in_seq1))])
# convert to [rows, columns] structure
in_seq1 = in_seq1.reshape((len(in_seq1), 1))
in_seq2 = in_seq2.reshape((len(in_seq2), 1))
out_seq = out_seq.reshape((len(out_seq), 1))
# horizontally stack columns
dataset = hstack((in_seq1, in_seq2, out_seq))
# choose a number of time steps
n_steps_in, n_steps_out = 3, 2
# covert into input/output
X, y = split_sequences(dataset, n_steps_in, n_steps_out)
# the dataset knows the number of features, e.g. 2
n_features = X.shape[2]
model = Sequential()
model.add(LSTM(200, activation=’relu’,return_sequences=True, input_shape=(n_steps_in, n_features)))
model.add(LSTM(200, activation=’relu’, return_sequences=True))
model.add(TimeDistributed(Dense(n_features)))
model.compile(optimizer=’adam’, loss=’mse’)
model.fit(X, y, epochs=300, verbose=0)
# demonstrate prediction
x_input = array([[60, 65, 125], [70, 75, 145], [80, 85, 165]])
x_input = x_input.reshape((1, n_steps_in, n_features))
yhat = model.predict(x_input, verbose=0)
print(yhat)
Plz help !
Thanx in advance
Hi Kostas…Please clarify your question so that we may better assist you.
Thank you for the tutorial, but I have a question.
I tried implementing a Multiple Parallel Input and Multi-Step Output model by using a vector output model instead of a encoder-decoder (as you did at the end of your tutorial) but I keep getting some errors.
The code is presented below. Could you please help me out ?
Thanks in advance!
from numpy import array
from keras.models import Sequential
from keras.layers import LSTM
from keras.layers import Dense
from keras.layers import Bidirectional
from keras.layers import Flatten
from keras.layers import TimeDistributed
from keras.layers.convolutional import Conv1D
from keras.layers.convolutional import MaxPooling1D
from keras.layers import ConvLSTM2D
from numpy import hstack
from keras.layers import RepeatVector
# split a multivariate sequence into samples
def split_sequences(sequences, n_steps_in, n_steps_out):
X, y = list(), list()
for i in range(len(sequences)):
# find the end of this pattern
end_ix = i + n_steps_in
out_end_ix = end_ix + n_steps_out
# check if we are beyond the dataset
if out_end_ix > len(sequences):
break
# gather input and output parts of the pattern
seq_x, seq_y = sequences[i:end_ix, :], sequences[end_ix:out_end_ix, :]
X.append(seq_x)
y.append(seq_y)
return array(X), array(y)
# define input sequence
in_seq1 = array([10, 20, 30, 40, 50, 60, 70, 80, 90])
in_seq2 = array([15, 25, 35, 45, 55, 65, 75, 85, 95])
out_seq = array([in_seq1[i]+in_seq2[i] for i in range(len(in_seq1))])
# convert to [rows, columns] structure
in_seq1 = in_seq1.reshape((len(in_seq1), 1))
in_seq2 = in_seq2.reshape((len(in_seq2), 1))
out_seq = out_seq.reshape((len(out_seq), 1))
# horizontally stack columns
dataset = hstack((in_seq1, in_seq2, out_seq))
# choose a number of time steps
n_steps_in, n_steps_out = 3, 2
# covert into input/output
X, y = split_sequences(dataset, n_steps_in, n_steps_out)
# the dataset knows the number of features, e.g. 2
n_features = X.shape[2]
model = Sequential()
model.add(LSTM(200, activation=’relu’,return_sequences=True, input_shape=(n_steps_in, n_features)))
model.add(LSTM(200, activation=’relu’,return_sequences=True ))
model.add(TimeDistributed(Dense(2)))
model.compile(optimizer=’adam’, loss=’mse’)
model.summary()
# fit model
model.fit(X, y, epochs=300, verbose=0)
# demonstrate prediction
x_input = array([[60, 65, 125], [70, 75, 145], [80, 85, 165]])
x_input = x_input.reshape((1, n_steps_in, n_features))
yhat = model.predict(x_input, verbose=0)
print(yhat)
Hi Kostas…Thanks for asking.
I’m eager to help, but I just don’t have the capacity to debug code for you.
I am happy to make some suggestions:
Consider aggressively cutting the code back to the minimum required. This will help you isolate the problem and focus on it.
Consider cutting the problem back to just one or a few simple examples.
Consider finding other similar code examples that do work and slowly modify them to meet your needs. This might expose your misstep.
Consider posting your question and code to StackOverflow.
Thanks for the reply, but the code I posted is actually a copy of your last implementation, which is “Multiple Parallel Input and Multi-Step Output” implementation.
In your article, I quote :
“A vector output or an encoder-decoder model could be used. In this case, we will demonstrate a vector output with a Stacked LSTM.”
I tried using a Stacked LSTM instead of an encoder-decoder model, but I did not work, because I’m using three timesteps for training and I’m trying do predict a 2 timesteps series.
#Correction
Thanks for the reply, but the code I posted is actually a copy of your last implementation, which is “Multiple Parallel Input and Multi-Step Output” implementation.
In your article, I quote :
“We can use either the Vector Output or Encoder-Decoder LSTM to model this problem. In this case, we will use the Encoder-Decoder model”
I tried using a Stacked LSTM instead of an encoder-decoder model, but it did not work, because I’m using three timesteps for training and I’m trying do predict a 2 timesteps series.
How can I solve the issue plz ?
Hi Kostas…What error(s) are you encountering?
Dear Sir,
Thank you so much for this great tutorial.
I am working on a project that requires me to feed real-time IoT data (with four variables) to the vanilla LTSM model to enable me to predict an outcome.
Kindly provide me with a guide on this.
Thank you
Hi Emmy…Thanks for asking.
Sorry, I cannot help you with your project.
I’m eager to help, but I don’t have the capacity to get involved in your project at the level you need or at a level to do a good job.
I’m sure you can understand my position, as I get many of requests to help with projects each day.
Nevertheless, I am happy to answer any specific questions you have about machine learning.
Hello Jason,
Greetings.
Does RNN use one-hot encoding in each time step for time series data forecasting?
for instance, input=[10,20, 30]
In 1st time step input is [10, 0, 0],
In 2nd time step input is [0, 20, 0], and
In 3rd time step input is [0, 0, 30]
Isn’t it?
Thanks in advance.
Hello. First, thank you for your support to developers.
I am having a lot of trouble while I’m trying to estimate if my univariate data is forecastable or not.
What am I doing?:
1- Using StandartScale to scale my data
2- Using the “difference” method to make my data stationary.
3- Testing my data’s stationarity with null hypothesis.
My questions:
-When i use MinMax scaler my prediction being absoulute flat (tried relu,sigmoid,even None) Why do you think?.
-My validation loss increasing, then stabilizing.. why?
I can publish my code if you want,
Thanks in advance!
Hi McanP…The following resource may give you some ideas to improving your models:
https://machinelearningmastery.com/get-the-most-out-of-lstms/
Firstly, thanks for this blog. I am developing LSTM forecasting model for stock price. For company X LSTM model with 2 layers, epoch 5, batch size 1 works well with 10 future steps (Recursive Multi-step Forecast). I get RMSE between predicted and actual values less than 5. But the same model with company Y with same rows of data does not work well. RMSE is larger than 20. I am not able to figure out why this happens.
Apart from RMSE can you suggest method to check how accurate predictions are done by the model.
Hi Lochan…Machine learning model performance is relative, not absolute.
Start by evaluating a baseline method, for example:
Classification: Predict the most common class value.
Regression: Predict the average output value.
Time Series: Predict the previous time step as the current time step.
Evaluate the performance of the baseline method.
A model has skill if the performance is better than the performance of the baseline model. This is what we mean when we talk about model skill being relative, not absolute, it is relative to the skill of the baseline method.
Additionally, model skill is best interpreted by experts in the problem domain.
For more on this topic, see the post:
How To Know if Your Machine Learning Model Has Good Performance
When I feed the test dataset to the model for predictions, the model predicts with almost 0 variation from test data for the first 70% of test data. I am predicting only a single outcome and for the next outcome I am using the original test value, not my predicted value. Still, for the last 30% of data, the variation (or deviation) between test data and predicted data starts increasing. Plotting it, I found that for the last 30% of test dataset, the deviation between expected and predicted data is even bigger than 25 digits. No matter how big or small dataset I am using, results are always bad for last 30% predictions. What should I do to get more accurate predictions.
Hi Lochan…The following may help you get the most from your LSTM models:
https://machinelearningmastery.com/get-the-most-out-of-lstms/
Hi!
Thank you very much for this useful tutorial.
I have a question on the first example (Vanilla LSTM). You showed how to make one prediction, but how can I proceed in making more?
I mean, should I use the same model and then just pass as input the two last trained values plus the first prediction (if n_steps = 3, for instance)? Or should I retrain the model using the first prediction value as part of the new training set and go on like that?
Thanks for the help!
Ilenia
Hi Ilenia…Are you wanting to extend the forecast time period?
Hi James!
Yes, basically, that’s what I would like to do. Let’s say I want to forecast up to 3 future values, instead of just one, what should I do?
Thanks!
Hey , I’m new to LSTM. I have to start learning this for my fyp where I have to train model to predict future sensor values. Can you guide me how to start?, what are the pre-requisites and how I can do better? What language tool, software to use. I’m familiar with python and practicing on VS Code but not sure where to run all this?
Hi Javvv…there are many options, however one of the most straightforward is setting up a Anaconda environment:
https://machinelearningmastery.com/setup-python-environment-machine-learning-deep-learning-anaconda/
Hi Javvv…the following resource may help clarify:
https://stackoverflow.com/questions/52959685/how-to-get-the-prediction-of-new-data-by-lstm-in-python
Hi Jason,
Thank you for the tutorials. They are very helpful.
If I have multivariate time series, dependent time series, however, instead of predicting time series, I would like to get the target output from multiple input variables in the same time stamp,
For example, the first column is input variable 1, the 2nd column is the input variable 2, and the 3rd column is the target variable.
[[ 10 15 25]
[ 20 25 45]
[ 30 35 65]
[ 40 45 85]
[ 50 55 105]]
I would like to have the input of 10, 15 to output 25, 20, 25 to 45, 30, 35 to 65 etc.
Can I simply follow the examples you’d discussed in the “Multivariate LSTM Models” section, but set n_steps=1? Or there are other methods to deal with such situation?
Thank you
Hi James,
Awesome tutorial.
if I want to train the same model on several sequences, how would you do this ?
Thanks in advance for the answer.
Hi Mat…The following resource may help in terms of saving and loading a model that could be trained on new data.
https://machinelearningmastery.com/save-load-keras-deep-learning-models/
Thanks James for the link. I implemented the model and iterated it on several sequences.
LSTM is clearly very heavy (very long to iterate 100 epoch on only 1 sequence).
I have to find an other solution. But thanks for the support, I realy appreciated it and your blog is a huge source of information. Thanks for the work and the knwoledge you share, and congratulations.
Hi Jason, thanks for your tutorials.
Is it possible to train LSTM for different lookback values in different epochs/iterations? Kindly suggest your views
Hi Brijesh…the following may be of interest to you:
https://towardsdatascience.com/time-series-forecasting-with-recurrent-neural-networks-74674e289816
Thanks James! I mean to say: Instead of fixed lookback, is it possible that lstm-network learns the lookback value on its own?
Hi Jason
I am using LSTM for sequence to sequence modelling in computer networking scenario. I am considering multiple parallel series and multi-step forecasting. However, in my scenarios the number of input parallel series is not fixed. How can i handle this scenario? Kindly i need your guidance.
Regards
Hi skr…You may wish to approach the problem with an encoder/decoder model:
https://machinelearningmastery.com/develop-encoder-decoder-model-sequence-sequence-prediction-keras/
Hi Jason,
Thank you for this. I am new to LSTM, so this really helped me. I would like to ask a question. I have a small data of 24 time points with a clear trend of increase over time. Is it fine to use LSTM or should I go with classical time series methods such as ARIMA?
Thanks once again,
Hi Budha…My recommendation would be to apply ARIMA and an LSTM model and compare results. One is not necessarily the best option in all cases.
Thank you so much for the reply. I will definitely try both models. Love reading your tutorials.
In section, “Multiple Input Series”, very strange to see the result is not 100% precise? Because it should be very easy for the network to learn add operation? (The output is just the sum of current time step’s inputs)
Hi ewind…the following discussion may be helpful:
https://www.quora.com/Why-cant-machine-learning-deep-learning-algorithms-be-a-100-accurate-at-test-time
Interestingly, I could only replicate your results with Multi-Step LSTM Models when I increased the number of iterations, the length of the sampling time series and the size of the input data. Was that because I haven’t any GPU hardware, that TensorFlow would use ? BTW, in my current setup, TensorFlow is complaining about how Keras uses it.
Hi Jason, thank you for the tutorial. I have a question about the Multiple Parallel Input and Multi-Step Output.
The number of features is specified in the Dense output layer for MultiVariate-MultiStep-MultiParallel forecast, as in the last example above where the number of features in the input and output sequences are the same.
How is this done when the number of features for the input and output are not the same? Foremaple, i am using 15 input variables and only want to forecast 4 in a multistep forecast.
I will appreciate your response. Thank you
Hi Olaitan…I would recommend the following resource:
https://machinelearningmastery.com/feature-selection-with-real-and-categorical-data/
Hi Jason,
Thanks for your tutorial. It is very useful for me.
I have one question what if I have multiple series with different dimensions?
Thanks for your answer
Hi Andre…You are very welcome! With limited knowledge of your application, you may want to investigate ensemble learning:
https://machinelearningmastery.com/ensemble-machine-learning-with-python-7-day-mini-course/
Hi James,
Sorry I did’t explain it well. My doubt is about “Multiple Input Series”. I have data from multiple sites and I want to forecast the precipitation area of each site. These sites has same features but different time steps. I understood that LSTM can learn parallel input series. Can I apply it in this case too? If yes, how would you recommend I start?
Thank you
Hi Jason thank you so much for the tutorial I had one doubt
I have 2 series of x,y coordinates
s1 = [[x1,y1],[x2,y2],[x3,y3],[x4,y4],[x5,y5],[x6,y6],[x7,y7],[x8,y8]]
s2 = [[a1,b1],[a2,b2],[a3,b3],[a4,b4],[a5,b5],[a6,b6],[a7,b7],[a8,b8]]
I need to send both of them as inputs to lstm what would you suggest I should do? multiple input seies with more than one value in each instance..
Hi Pranav…You may wish to investigate multivariate LSTM models such as presented in the following tutorial:
https://machinelearningmastery.com/multivariate-time-series-forecasting-lstms-keras/
import numpy as np
from tensorflow.keras.models import Sequential
from tensorflow.keras.layers import Dense, LSTM
# Our Input data X
X = q_cqi
X = X.reshape(1, -1)[0]
X.shape
# Creating a window of 10
window_size = 10
X_train = []
y = []
inc = 0
for i in range(len(X) – window_size):
if inc + window_size + 2 > len(X):
break
row = [[a] for a in X[inc:inc + window_size]]
X_train.append(row)
idx = inc + window_size + 1
y.append(X[idx])
inc += 1
X = X_train
#converting list back into arrays
X=np.array(X)
y=np.array(y)
#Splitting data into train, test and validation
X_train, y_train = X[:25000], y[:25000]
X_val, y_val = X[25000:27200], y[25000:27200]
X_test, y_test = X[27200:], y[27200:]
n_steps=10
n_features=1
# define model
model = Sequential()
model.add(LSTM(128, return_sequences= True ,activation=’linear’, input_shape=(n_steps, n_features)))
model.add(LSTM(64 ,activation=’linear’))
model.add(Dense(32, ‘linear’))
model.add(Dense(16, ‘linear’))
model.add(Dense(1))
#Compiling the model
#model.compile(loss=MeanAbsoluteError(), optimizer=’Adam’,metrics=[RootMeanSquaredError()])
model.summary()
So above is my input data and my LSTM model. Now I am confused about how to generate the new data? what I mean when I create the new vector q_cqi again like this
# Our Input data X
X = q_cqi
X = X.reshape(1, -1)[0]
when I create the new vector q_cqi again like this, what would be the next step? how can i reshape it? do i need the target value y in this new data? how I can chose a data suppose from this input vector of length 35000 if I want to do predction on the last 1500 or first 1000 how could i do this?
what I mean when I create the new vector q_cqi again like this
# Our Input data X
X = q_cqi
X = X.reshape(1, -1)[0]
what would be the next step? how can I change the following section i.e. creating the window etc.?
window_size = 10
X_train = []
y = []
inc = 0
for i in range(len(X) – window_size):
if inc + window_size + 2 > len(X):
break
row = [[a] for a in X[inc:inc + window_size]]
X_train.append(row)
idx = inc + window_size + 1
y.append(X[idx])
inc += 1
X = X_train
Do I need the target value y? how I can chose the new input? Could you please answer how I can generate the new data and how to implement my trained model on the new data?
Hi James! Thank you for your great posts. I am working on a project. It is a regression problem and I am using LSTM model to predict the next value. I trained my LSTM model and test and validate it on the same data. Now I want to generate new data as the previous one but I am confused about this new data whether I will have the target value in this new data or not? also how can I reshape it to used it for my trained LSTM model. the following are my LSTM model and input data. my input vector is around 35000.
import numpy as np
from tensorflow.keras.models import Sequential
from tensorflow.keras.layers import Dense, LSTM
# Our Input data X
X = q_cqi
X = X.reshape(1, -1)[0]
X.shape
# Creating a window of 10
window_size = 10
X_train = []
y = []
inc = 0
for i in range(len(X) – window_size):
if inc + window_size + 2 > len(X):
break
row = [[a] for a in X[inc:inc + window_size]]
X_train.append(row)
idx = inc + window_size + 1
y.append(X[idx])
inc += 1
X = X_train
#converting list back into arrays
X=np.array(X)
y=np.array(y)
#Splitting data into train, test and validation
X_train, y_train = X[:25000], y[:25000]
X_val, y_val = X[25000:27200], y[25000:27200]
X_test, y_test = X[27200:], y[27200:]
n_steps=10
n_features=1
# define model
model = Sequential()
model.add(LSTM(128, return_sequences= True ,activation=’linear’, input_shape=(n_steps, n_features)))
model.add(LSTM(64 ,activation=’linear’))
model.add(Dense(32, ‘linear’))
model.add(Dense(16, ‘linear’))
model.add(Dense(1))
#Compiling the model
#model.compile(loss=MeanAbsoluteError(), optimizer=’Adam’,metrics=[RootMeanSquaredError()])
model.summary()
Thanks in advance
Hi Inam…You are very welcome! The following resource should add clarity:
https://machinelearningmastery.com/make-predictions-long-short-term-memory-models-keras/
Thank you James!
Hello I’ve been able to create an LSTM model for my fourth year project which is about forex price movement forecasting but the problem comes to when I want to try and implement it in real time. I trained the model on 30 minute data so the Idea was to make the model into an API with like 10-20 closing prices of a particular forex pair eg GBP/USD and the have the model predict at least 2 hours into the future i.e. 4 30 minute periods and then the API would return that. Thankyou in advance for your help.
Hi Francis…While we cannot recommend any particular model for your project, it would be helpful if you could elaborate on a specific question regarding our content so that we may better assist you.
Which is best for time series prediction like stock price prediction?
Hi Arun…While we cannot recommend any particular model for that purpose, you may find the following the following of interest:
https://machinelearningmastery.com/using-cnn-for-financial-time-series-prediction/
Hello James! I hope you will be. thanks for your great posts.
I am trying to plot perfromance evaluation of 2 methods (the LSTM and the Ideal)
I want to compare these two. Also I want to make a plot between the [e_DRNN1,thr_DRNN1]
bit-error-rate and achieved throughput. How could I do this? the following are my code with
the respected output for each method.
#Method LSTM
[e_DRNN1,thr_DRNN1]=e_short_pkts(p.L_pkt,gamma_real,gamma_DRNN1,p)
e_DRNN1,thr_DRNN1
(array([[0.00000000e+00, 9.83990470e-01, 4.78419178e-07, …,
0.00000000e+00, 1.62437153e-03, 4.77800111e-02]]),
array([[2.20861316, 0.05908644, 3.07646398, …, 3.5582583 , 4.1410422 ,
4.0731042 ]]))
#Method Ideal
[e_ideal,thr_ideal]=e_short_pkts(p.L_pkt,gamma_real,gamma_ideal,p)
e_ideal,thr_ideal
(array([[0. , 0.98399047, 0.97368655, …, 0.08990624, 0.15850721,
0.12215858]]),
array([[2.20861316, 0.05908644, 0.09711517, …, 3.89260928, 3.63755763,
3.79468346]]))
Thank you
awsm tutorial
Thank you Anwar for your feedback! We appreciate it!
Thank you very much for making it easy to understand James.
As a beginner I tried to get one output from 5 random sets of numbers , letting the model learn by itself.
How can I get single output from the 5 sets of input please?
Thank you very much anyway
Avi Ofek
Hi Avi…You are very welcome! If I understand your question, I would recommend that results be averaged as deep learning models are inherently “stochastic”.
https://machinelearningmastery.com/stochastic-optimization-for-machine-learning/
Hello Jason,
Thank you for this blog. It is helpful as always.
I have one doubt. How to prepare data for future prediction? let’s say I want to forecast energy consumption for the next 3 years in an hourly manner. For training data, we have a date and energy consumption hour wise. How do I prepare testing data where I only have a date?
Thank you
Hi Sagar…the benefit of LSTM and CNN models is that they “learn features” of an existing time series to make future predictions. These models perform “autoregression” as explained in the following resource:
https://machinelearningmastery.com/autoregression-models-time-series-forecasting-python/
Hi
Thanks for the tutorial. For the univariate series, is there a reason to use ConvLSTM2D and not ConvLSTM1D ?
Hi,
I did not really understand why it was necessary to use subsequences instead of the sequences in the CNN-LSTM model. Could you please detail that ?
Thanks
Hi mayan…The following resource may be of interest to you:
https://machinelearningmastery.com/cnn-long-short-term-memory-networks/
Hi again
In the ConvLSTM could we have used ConvLSTM1D instead of ConvLSTM2D ?
Hi mayan…The following may be of interest to you:
https://medium.com/neuronio/an-introduction-to-convlstm-55c9025563a7
Hi all, I am trying to find the solution to a simillar problem and I wonder if you can help.
I have panel data on 200 different stocks, each stock belongs to a different sector of which there are 12 different sectors hot encoded 1-12. For each stock there 8 different pieces of price information such as price, market capitalisation, volume, and so forth. I then have a a column of of future stock prices on which to train the mdoel.
Would this mean I need to train 200 different models? How would you go about this problem if you were given this dataset?
Sorry if this is a daft question. I am new to ML.
Hi Jason, massive fan of your work throughout the years.
Keeping it short as I assume you have hundreds of messages a day!
If one has a dataset on 400 patients’ health through time.
X variables are: Patient ID, Age Group (Binary i.e OLD 1 and Young 2), Distance walked during the day, Amount of calories eaten that day.
Y variable to be predicted is: Amount of non-fatal heart attacks.
My idea was that one could run 400 different LSTM time series models on each individual to predict the amount of non-fatal heart attacks.
My question is! These results would gain no information from the other predictions, is there a way you know of linking this information?
For example, if one was to train a model on an OLD patient, is there any way that the model can learn that OLD patients have tended to have more non-fatal heart attacks in the other regressions so the model incorporates more non-fatal heart attacks to this old patients predictions?
Maybe I am thinking about it wrong, please help!
Hi, is there a “multi parallel & multi inputs(features)” LSTM model? Thanks!
Hi frr…You may find the following resource of interest:
https://machinelearningmastery.com/multivariate-time-series-forecasting-lstms-keras/
Hi, I searched so much and even used chatGPT … but I’m so confused. I have data set of company and I should find a model for customer churn using LSTM. I have customer (showing by IDs) behavior of these customer in 12 months , I mean I know the churn label for ID : 1445 in first month , second month and so on. This data set has features like monthly_visit or age of customers or the sim_type or contract_ type and so on. How can I define the LSTM input and output. I like to say that I want to predict the churn for customer 1445 for month 12 based on month 11, 10,9 and 8 and then for the customer 1445 I want to predict month 11 based on 10,9,8 and 7 and so on and then jump into the next customer and do the same for him. How can I use LSTM for this problem? sorry for long explanation.
Hi Iman…Please narrow your query to a single question so that we may better assist you.
Sorry … Is it possible to predict customer churn using LSTM when you have monthly behavior of customers? I mean what’s the X(input) and y(output) for LSTM ?
Is it possible to use LSTM for customer churn prediction when you have monthly behavior of customer and the churn label of each month ? I mean what should be the X(input) and y (output) for LSTM ?
Is it possible to use LSTM for customer churn prediction ? what’s the X and y for LSTM model. note that I have the monthly behavior of each customers in 12 months.
Hi Mani…The following resource may be of interest:
https://towardsdatascience.com/churn-prediction-with-machine-learning-ca955d52bd8c
Hi ,I have a dataset that represent the monthly behavior of customers with 1million rows and 8 columns , I mean every 12 rows of dataset are for one customer and I want to predict churn model for these customers using LSTM. how should I make input and output for my LSTM model when I have dataset of monthly behavior of customers?
Hi David…I would recommend a multivariate lstm model for this purpose:
https://machinelearningmastery.com/multivariate-time-series-forecasting-lstms-keras/
Hi Jason,
Appreciate your guide for LSTM time series model. It is really helpful.
I have followed your step to make my own time series LSTM model but encountered a question.
At stage 1, I had multivariate single step forecasting.(simple LSTM model with 3 dense layers)
At stage 2, I converted it to multivariate multi-step forecasting by using Encoder-Decoder model.
But in doing so, my dense layer complexity dropped which I didn’t wanted.
Can you give any suggestion how to maintain complexity of dense layer while using Encoder-Decoder model?
Please see below in code and model summary
At stage 1(Simple LSTM model)
model = tf.keras.models.Sequential([
tf.keras.layers.Input(shape=(window_size, n_character)),
tf.keras.layers.LSTM(100, return_sequences=True),
tf.keras.layers.LSTM(100),
tf.keras.layers.Dense(100, activation=”relu”),
tf.keras.layers.Dense(100, activation=”relu”),
tf.keras.layers.Dense(n_outPut_charactor)
])
model.summary()
Model: “sequential”
________________________________________________________________
Layer (type) Output Shape Param #
=================================================================
lstm (LSTM) (None, 20, 100) 59600
lstm_1 (LSTM) (None, 100) 80400
dense (Dense) (None, 100) 10100
dense_1 (Dense) (None, 100) 10100
dense_2 (Dense) (None, 44) 4444
=================================================================
Total params: 164644 (643.14 KB)
Trainable params: 164644 (643.14 KB)
At stage 2 (Encoder- Decoder model)
model = tf.keras.models.Sequential([
tf.keras.layers.Input(shape=(window_size, n_character)),
tf.keras.layers.LSTM(100),
tf.keras.layers.RepeatVector(n_step_out),
tf.keras.layers.LSTM(100,return_sequences=True),
tf.keras.layers.TimeDistributed(tf.keras.layers.Dense(n_outPut_charactor,activation=’relu’)),
])
Model: “sequential”
_________________________________________________________________
Layer (type) Output Shape Param #
=================================================================
lstm (LSTM) (None, 100) 59600
repeat_vector (RepeatVecto (None, 3, 100) 0
r)
lstm_1 (LSTM) (None, 3, 100) 80400
time_distributed (TimeDist (None, 3, 44) 4444
ributed)
=================================================================
Total params: 144444 (564.23 KB)
Trainable params: 144444 (564.23 KB)
Hi Justin…You are very welcome! The following resource may be of interest to you:
https://machinelearningmastery.com/develop-encoder-decoder-model-sequence-sequence-prediction-keras/
Hi James,
I aim to develop an ML predictive model (forecasting) to predict the next failure time
I have the following data type:
-Failure date (dd/mm/yy)
-Failure time (11:00 am)
-Recovery data (dd/mm/yy)
-Recovery time (11:30 am)
-Operational delay (30 min)
-Age of equipment
-Number of Failures last time
Q:
1-Can you suggest models to be used for prediction
2- Is there an example of this type of prediction
3- How to per-processing the (date & time) Data
Regards,
Thank you, it seems that you explained the LSTM model implementation quite well but I cannot run your code. Why there is no intended block in the for loops and if loops?
Hi Julia…did you type the code or copy and paste it? There could be formatting issues resulting from the way in which the code was entered into your Python environment.
Hi James, thank you for your answer. I have found the way to properly copy the code by click toggle plain code.
Thank you Jason for the great resource. I have a question : I am trying to train an LSTM autoencoder model on a multivariate time series to detect anomalies using reconstruction error. I want to train the model on normal operating mode, and i have 2 years time of data. A fault occurs 4 months into the timeseries, so i have normal operating mode data before the fault and another normal data after the fault. How can use those two sub time series before and after the fault to train the model ? As far as i know, the timeseries should have a consistent time interval and without cuts in time. What do you suggest ? I was considering adding time features to the existing features fed to the model, explicitly feeding the Model with time information, other option would be maybe to update the model after training it on the first subseries before the fault and then updating it with the second time series after the fault, am not sure this is possible.
Thank you for your time again.
Hi Bassel…Some ideas can be found here:
https://carloalbertocarrucciu.medium.com/filling-large-gaps-in-time-series-using-forecasting-2f6db5f5286b
https://stats.stackexchange.com/questions/106358/gaps-in-time-series-and-time-series-validity
Hello Dr. Brownlee
thank you for putting this together! I really helped me understand the operations behind LSTM.
i have couple questions if you can
1. in vanilla/stackedetc LSTM you use “model.add(LSTM(50,” .. why 50? the keras LSTM doc specifies this field as “units: Positive integer, dimensionality of the output space.”, which makes me think we should use n_steps or n_features, but as i tried to run it with either of those two options the result was absolutely nowhere near what it should be
2. in Multiple Input Series > Multiple Input Series shouldnt the “Output” be 85 and not 65 since 85 is the output at the next timestep in the dataseries? similarly as 10,20,30 and output was 40?
Hi Martin…
Determining the input and output parameters of Long Short-Term Memory (LSTM) models is crucial for designing neural networks that can effectively process sequence data (e.g., time series, natural language text). LSTM models are a type of recurrent neural network (RNN) capable of learning long-term dependencies in data, making them suitable for tasks like language modeling, time series forecasting, and more.
### Input Parameters
1. **Input Shape:**
– The input shape to an LSTM layer is typically
(batch_size, time_steps, features)
:– **batch_size**: How many sequences you’re passing through the network at once. It can be left unspecified (None) during model definition for flexibility.
– **time_steps**: The length of the sequence, i.e., how many time steps or elements are in each sequence.
– **features**: The number of features in each time step. For instance, in text processing, it could be the size of the word embedding vector; in time series, the number of variables at each time step.
2. **Timesteps and Feature Selection:**
– Based on the problem, decide how many past observations (time steps) your model should consider for predicting the future value or next sequence element. This will define your window size or the sequence length.
– The features depend on the data available and the nature of the problem. For instance, in a stock price forecasting problem, features could include past prices, volume, and other technical indicators.
### Output Parameters
1. **Output Shape:**
– The output of an LSTM can be tailored based on the task:
– **Many-to-One**: For tasks like sentiment analysis, where the entire sequence maps to a single label. The output shape would be
(batch_size, units)
, where units refer to the number of LSTM units (neurons).– **Many-to-Many**: For tasks like machine translation or sequence generation, where each input time step corresponds to an output time step. This can be achieved by setting
return_sequences=True
in LSTM layers, resulting in an output shape of(batch_size, time_steps, units)
.– **Custom**: Using techniques like sequence-to-sequence models, where an encoder LSTM’s output is used as an input to a decoder LSTM, allowing for flexible input-output configurations.
2. **Number of Units:**
– This parameter defines the dimensionality of the output space of the LSTM layer, i.e., how many hidden states (neurons) each unit/time step should have. It is a crucial parameter to tune based on the complexity of the task and the amount of data available.
### Design Considerations
– **Sequence Padding:** If your input sequences have variable lengths, you’ll need to pad them to ensure they have the same length for batch processing.
– **Batch Size:** The choice of batch size can affect training dynamics and performance. Smaller batches might lead to faster convergence but can be noisier. Larger batches provide more stable but potentially slower convergence.
– **Statefulness:** Decide whether your LSTM model should remember its state (hidden states) across batches. Stateful LSTMs can be beneficial for time series data where the sequence continuity across batches is important.
### Practical Steps
1. **Preprocessing**:
– Normalize/standardize your input data.
– Convert text data into numerical form (e.g., embeddings for NLP tasks).
– Ensure sequences have a fixed length (padding/truncating where necessary).
2. **Model Definition**:
– Choose the appropriate architecture (e.g., stacked LSTMs, bidirectional LSTMs) based on your problem.
– Experiment with different numbers of units, batch sizes, and sequence lengths.
3. **Training**:
– Use a validation set to monitor performance and avoid overfitting.
– Adjust learning rate, optimization algorithm, and other hyperparameters as needed.
Determining the optimal input and output parameters for LSTM models often requires experimentation and is guided by the specific requirements and constraints of your application.
With your tutorials, It took me only a week to complete LSTM necessary knowledge for working on a real-world problem. Thank you si much!
Hi Mesabo…You are very welcome! Thank you for sharing your success!
Hello
I have 21 images(tiff file) that each of them has 60 bands. and each of them is for one year(2000-2020). one of this bands is land cover of pixel.
I want forecast land cover change for next year of data
which model do you suggest? ConvLSTM?
Hi Arsalan…That would be a great model type to start with! Let us know how it goes!
Hello,
First, I would like to say, that this is an amazing tutorial!
My question is, at the Multiple Parallel Series example where we have three input series and three output series (3 features) in a single LSTM net, how is the loss computed? Is it the average of the losses in each of the three parallel series?
Best!!
Hi Charitini…Multivariate considerations are discused here:
https://machinelearningmastery.com/multivariate-time-series-forecasting-lstms-keras/
ModuleNotFoundError Traceback (most recent call last)
Cell In[32], line 1
—-> 1 from keras.layers.convolutional import Conv1D
2 from keras.layers.convolutional import MaxPooling1D
ModuleNotFoundError: No module named ‘keras.layers.convolutional’
What version is the keras on the above??
from keras.layers import Conv1D
from keras.layers import MaxPooling1D
this seems to resolve the above
Thank you for your feedback Kai!