Time series forecasting can be framed as a supervised learning problem.
This re-framing of your time series data allows you access to the suite of standard linear and nonlinear machine learning algorithms on your problem.
In this post, you will discover how you can re-frame your time series problem as a supervised learning problem for machine learning. After reading this post, you will know:
- What supervised learning is and how it is the foundation for all predictive modeling machine learning algorithms.
- The sliding window method for framing a time series dataset and how to use it.
- How to use the sliding window for multivariate data and multi-step forecasting.
Kick-start your project with my new book Time Series Forecasting With Python, including step-by-step tutorials and the Python source code files for all examples.
Let’s get started.
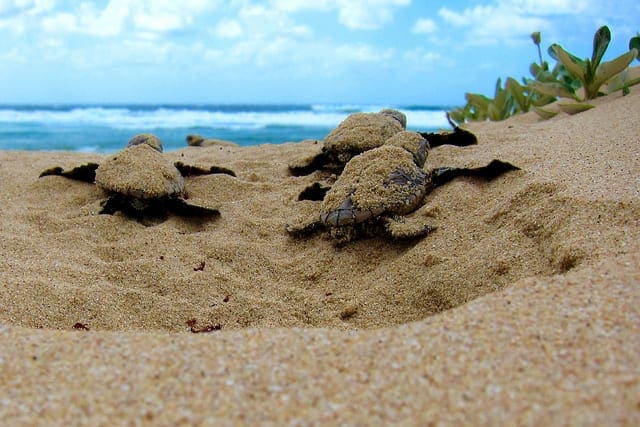
Time Series Forecasting as Supervised Learning
Photo by Jeroen Looyé, some rights reserved.
Supervised Machine Learning
The majority of practical machine learning uses supervised learning.
Supervised learning is where you have input variables (X) and an output variable (y) and you use an algorithm to learn the mapping function from the input to the output.
1 |
Y = f(X) |
The goal is to approximate the real underlying mapping so well that when you have new input data (X), you can predict the output variables (y) for that data.
Below is a contrived example of a supervised learning dataset where each row is an observation comprised of one input variable (X) and one output variable to be predicted (y).
1 2 3 4 5 6 |
X, y 5, 0.9 4, 0.8 5, 1.0 3, 0.7 4, 0.9 |
It is called supervised learning because the process of an algorithm learning from the training dataset can be thought of as a teacher supervising the learning process.
We know the correct answers; the algorithm iteratively makes predictions on the training data and is corrected by making updates. Learning stops when the algorithm achieves an acceptable level of performance.
Supervised learning problems can be further grouped into regression and classification problems.
- Classification: A classification problem is when the output variable is a category, such as “red” and “blue” or “disease” and “no disease.”
- Regression: A regression problem is when the output variable is a real value, such as “dollars” or “weight.” The contrived example above is a regression problem.
Stop learning Time Series Forecasting the slow way!
Take my free 7-day email course and discover how to get started (with sample code).
Click to sign-up and also get a free PDF Ebook version of the course.
Sliding Window For Time Series Data
Time series data can be phrased as supervised learning.
Given a sequence of numbers for a time series dataset, we can restructure the data to look like a supervised learning problem. We can do this by using previous time steps as input variables and use the next time step as the output variable.
Let’s make this concrete with an example. Imagine we have a time series as follows:
1 2 3 4 5 6 |
time, measure 1, 100 2, 110 3, 108 4, 115 5, 120 |
We can restructure this time series dataset as a supervised learning problem by using the value at the previous time step to predict the value at the next time-step. Re-organizing the time series dataset this way, the data would look as follows:
1 2 3 4 5 6 7 |
X, y ?, 100 100, 110 110, 108 108, 115 115, 120 120, ? |
Take a look at the above transformed dataset and compare it to the original time series. Here are some observations:
- We can see that the previous time step is the input (X) and the next time step is the output (y) in our supervised learning problem.
- We can see that the order between the observations is preserved, and must continue to be preserved when using this dataset to train a supervised model.
- We can see that we have no previous value that we can use to predict the first value in the sequence. We will delete this row as we cannot use it.
- We can also see that we do not have a known next value to predict for the last value in the sequence. We may want to delete this value while training our supervised model also.
The use of prior time steps to predict the next time step is called the sliding window method. For short, it may be called the window method in some literature. In statistics and time series analysis, this is called a lag or lag method.
The number of previous time steps is called the window width or size of the lag.
This sliding window is the basis for how we can turn any time series dataset into a supervised learning problem. From this simple example, we can notice a few things:
- We can see how this can work to turn a time series into either a regression or a classification supervised learning problem for real-valued or labeled time series values.
- We can see how once a time series dataset is prepared this way that any of the standard linear and nonlinear machine learning algorithms may be applied, as long as the order of the rows is preserved.
- We can see how the width sliding window can be increased to include more previous time steps.
- We can see how the sliding window approach can be used on a time series that has more than one value, or so-called multivariate time series.
We will explore some of these uses of the sliding window, starting next with using it to handle time series with more than one observation at each time step, called multivariate time series.
Sliding Window With Multivariate Time Series Data
The number of observations recorded for a given time in a time series dataset matters.
Traditionally, different names are used:
- Univariate Time Series: These are datasets where only a single variable is observed at each time, such as temperature each hour. The example in the previous section is a univariate time series dataset.
- Multivariate Time Series: These are datasets where two or more variables are observed at each time.
Most time series analysis methods, and even books on the topic, focus on univariate data. This is because it is the simplest to understand and work with. Multivariate data is often more difficult to work with. It is harder to model and often many of the classical methods do not perform well.
Multivariate time series analysis considers simultaneously multiple time series. … It is, in general, much more complicated than univariate time series analysis
— Page 1, Multivariate Time Series Analysis: With R and Financial Applications.
The sweet spot for using machine learning for time series is where classical methods fall down. This may be with complex univariate time series, and is more likely with multivariate time series given the additional complexity.
Below is another worked example to make the sliding window method concrete for multivariate time series.
Assume we have the contrived multivariate time series dataset below with two observations at each time step. Let’s also assume that we are only concerned with predicting measure2.
1 2 3 4 5 6 |
time, measure1, measure2 1, 0.2, 88 2, 0.5, 89 3, 0.7, 87 4, 0.4, 88 5, 1.0, 90 |
We can re-frame this time series dataset as a supervised learning problem with a window width of one.
This means that we will use the previous time step values of measure1 and measure2. We will also have available the next time step value for measure1. We will then predict the next time step value of measure2.
This will give us 3 input features and one output value to predict for each training pattern.
1 2 3 4 5 6 7 |
X1, X2, X3, y ?, ?, 0.2 , 88 0.2, 88, 0.5, 89 0.5, 89, 0.7, 87 0.7, 87, 0.4, 88 0.4, 88, 1.0, 90 1.0, 90, ?, ? |
We can see that as in the univariate time series example above, we may need to remove the first and last rows in order to train our supervised learning model.
This example raises the question of what if we wanted to predict both measure1 and measure2 for the next time step?
The sliding window approach can also be used in this case.
Using the same time series dataset above, we can phrase it as a supervised learning problem where we predict both measure1 and measure2 with the same window width of one, as follows.
1 2 3 4 5 6 7 |
X1, X2, y1, y2 ?, ?, 0.2, 88 0.2, 88, 0.5, 89 0.5, 89, 0.7, 87 0.7, 87, 0.4, 88 0.4, 88, 1.0, 90 1.0, 90, ?, ? |
Not many supervised learning methods can handle the prediction of multiple output values without modification, but some methods, like artificial neural networks, have little trouble.
We can think of predicting more than one value as predicting a sequence. In this case, we were predicting two different output variables, but we may want to predict multiple time-steps ahead of one output variable.
This is called multi-step forecasting and is covered in the next section.
Sliding Window With Multi-Step Forecasting
The number of time steps ahead to be forecasted is important.
Again, it is traditional to use different names for the problem depending on the number of time-steps to forecast:
- One-Step Forecast: This is where the next time step (t+1) is predicted.
- Multi-Step Forecast: This is where two or more future time steps are to be predicted.
All of the examples we have looked at so far have been one-step forecasts.
There are are a number of ways to model multi-step forecasting as a supervised learning problem. We will cover some of these alternate ways in a future post.
For now, we are focusing on framing multi-step forecast using the sliding window method.
Consider the same univariate time series dataset from the first sliding window example above:
1 2 3 4 5 6 |
time, measure 1, 100 2, 110 3, 108 4, 115 5, 120 |
We can frame this time series as a two-step forecasting dataset for supervised learning with a window width of one, as follows:
1 2 3 4 5 6 7 |
X1, y1, y2 ? 100, 110 100, 110, 108 110, 108, 115 108, 115, 120 115, 120, ? 120, ?, ? |
We can see that the first row and the last two rows cannot be used to train a supervised model.
It is also a good example to show the burden on the input variables. Specifically, that a supervised model only has X1 to work with in order to predict both y1 and y2.
Careful thought and experimentation are needed on your problem to find a window width that results in acceptable model performance.
Further Reading
If you are looking for more resources on how to work with time series data as a machine learning problem, see the following two papers:
- Machine Learning for Sequential Data: A Review (2002) [PDF]
- Machine Learning Strategies for Time Series Forecasting (2013) (also slides PDF)
For Python code for how to do this, see the post:
Summary
In this post, you discovered how you can re-frame your time series prediction problem as a supervised learning problem for use with machine learning methods.
Specifically, you learned:
- Supervised learning is the most popular way of framing problems for machine learning as a collection of observations with inputs and outputs.
- Sliding window is the way to restructure a time series dataset as a supervised learning problem.
- Multivariate and multi-step forecasting time series can also be framed as supervised learning using the sliding window method.
Do you have any questions about the sliding window method or about this post?
Ask your questions in the comments below and I will do my best to answer.
Thanks for the article. I understand the transformation. Now how do you separate the data into training and testing sets? Also, will the next article be working a simple example through to building a predictive model?
Great question Robert, I will have a post on this soon.
Can you please post a link to the article (if you created one) which you mentioned in this comment?
Sure, see this post:
https://machinelearningmastery.com/backtest-machine-learning-models-time-series-forecasting/
Can you share the link
Due to sliding windows, is it possible that windows from the training and testing datasets overlap?
Hi Aini…The following resource may add clarity:
https://programmathically.com/what-is-the-sliding-window-algorithm/
What is the problem of directly do a regression model on the data and use the model to predict future?
You may overfit your model.
Hi Frank, thanks a lot for your helpful posts. I hope you can help me out with a more precise explanation or recommendation on my case. I have aggregated weekly sales for suit cases and need to predict future sales for a couple of weeks and I have been asked to use ML for the task. It’s a univariate ts but I need to transform it to a ML problem. So far I have extracted some predictors(e.g month,day,weeknumber etc) from my time variable as I can always recreate them for future dates as well. My issue is on lags and how to handle it as a predictor. Using your illustration above on univariate and assuming that your Y corresponds to my weekly sales. Now I lag Y and obtain a new predictor with NA which I later drop the related row for every predictor. As a result the number of weeks also drops.I understand that by doing so I be using past values to predict the current value but how do I recreate that lag for a future or today’s date? Like how do you generate line 7 given that you initially have 6 lines or 6 time stamps? I be glad to get your feedback.
Good question, this code will help:
https://machinelearningmastery.com/convert-time-series-supervised-learning-problem-python/
Hey Jason.
Thank you for providing so many useful articles. Can I get your help with time series prediction? Now. I have two variables, X and Y. X is timestamp, but its form is 1631500000, 1631500050, 1631500100… similar 500 points. The corresponding Y is ‘status_score’, and the content is 1.000625, -1.040353, -0.742401 and other scores.
Now I am asked to build a model and predict a data set with the only timestamp. I think the prediction result should be the’status_score’ corresponding to the timestamp.
what should I do? I am very grateful for your feedback.
I don’t see anything interesting with timestamp alone. I agree with you.
Machine learning methods are not suitable for time series analysis. They do not take into account the relationship that exists between data values.
Interesting perspective Leo.
Machine learning methods require this relationship is exposed to them explicitly in the form of a moving average, lag obs, seasonality indicators, etc. Just like linear regression does in ARIMA. No really a big leap here.
Classical methods (like MA/AR/ARMA/ARIMA/and friends) breakdown when relationships are non-linear, obs are not iid, residuals are not gaussian, etc. Sometimes the complexity of the problem requires we try alternate methods.
Finally, there are newer methods that can learn sequence, like LSTM recurrent neural networks. These methods have the potential to redefine an industry, just like has been done in speech recognition and computer vision.
Machine learning methods require that there is no correlation between variables. This breaks down for time series where the lagged values are correlated.
Moreover, there are many nonlinear time series methods like GARCH and its variants.
Great point, thanks Leo.
The point about correlated inputs is true for many statistical methods, less true for others like trees, instance-based methods and even some neural nets (cnn and rnn).
I think you’re spot on – most small univariate time series datasets will be satisfied with a classical statistical method. Perhaps LSTMs or decision trees on lagged vars can add something, perhaps not.
When things get hairy in data with a time component (like movement prediction, gesture classification, …) perhaps ML is the way to go. I need to do a better job of fleshing out this detail.
I tried it half a month ago, but it didn’t work well
Is that not a bit Bombastic.
There are several quant hedge funds that have made and continue to make mind blowing returns through the use of ML methods and correlated variables in multivariate TS data.
Maybe I’m missing something ?
ML does NOT require that there is no correlation between variables… nor does any regression model.
Regression models prefer uncorrelated input variables for model stability.
Not a requirement (we can still do it…), more of a strong preference.
See this article on Multicollinearity
https://en.wikipedia.org/wiki/Multicollinearity
Good point Jason. I guess I need to study LSTM.
When do you public something about the Multi-Step Forecasting? 🙂
They are scheduled for later this month or early next month.
The data generated from sensors of IoT or industrial machines are also typical time siries, and usually of huge amout, aka industrial big data.
For this type of TS, many digital signal processing methods are used when being analysised, such as FFT, wavelet transform, euclidean distance.
It seems that books discussing ML on TS usually don’t cover this DSP area. What do you think?
I agree Dehai.
We can view these methods as data preparation/data transforms in the project process.
Use of more advanced methods like FFT and wavelets requires knowledge of DSP which might be a step too far for devs looking to get into machine learning with little math background.
Thanks!
I had a project where I had to predict the likelihood of equipment failure from an event log. What worked pretty well was creating a training set from the event log with temporal target features that included whether or not a piece of equipment failed in the next 30, 60 days, etc. I also added temporal features for a piece of equipments past history, e.g., frequency of maintenance over different veriods, variance in measurements, etc. Could then apply any machine learning technique. Test set was created from last 20% of samples.
— Jay Urbain
Very nice, thanks for sharing Jay!
Hi Jay,
I am interested in finding out more about the predictive task you were involved with. Any chance you ahve a blog or can share more by email?
Hi Jason and Jay
We are also trying to predict device failure based on temporal signals like temperatures, humidity, power consumption, events\alarms etc..
How does one relate 5 temporal data signals into one single fail\pass result at the end of the period?
Most examples seem to be about predicting the signal itself where as in our case we probably need to find patterns in the relation between the signals. For example, if it is using a lot of power, the ambient temperature is low but the temperature is not decreasing, something something is wrong with the compressor.
Any tips would be highly appreciated.
Perhaps this example of multivariate forecasting will help as a starting point:
https://machinelearningmastery.com/multivariate-time-series-forecasting-lstms-keras/
Hi Sune,
How did your project turn out? I am working on a similar case and wanted to see how you ended up formulating the problem. How long of a time period did your input values end up spanning? Did you find any valuable resources along the way? One formulation I thought of was forecasting selected metric values and then classifying the forecasts as failure/ no failure. However this would heavily rely on accurate forecasting of the former model.
Jason, is using multi steps time lags with multivariate KNN or Random Forrest equivalent to transforming the feature space in similar way to kernel functions?
I will also be curious to see how SVM can be used on multivariate problems.
Thanks for the post.
I don’t think so Ziad, do you have a specific idea in mind?
In activity prediction application, the activity can be predicted only after multiple sequence of steps (multivariate time series data). Kindly suggest how to handle this problem for predicting the activity
Nice problem Kavitha. Sorry, I don’t have any examples of activity prediction. I don’t want to give you uninformed advice.
How would the time series restructuring be affected if we have 2 level or n level categorization within a time series. For example in case of sensor data we get it on each day and with-in the day say at every 5 seconds. The correlation may exist at the outer level i.e at day level but may not at internal level i.e at next sample (in seconds).
Day1 Measure
5PM 20
5PM5Sc 22
Day2 Measure
5pm 25
5pm5sc 27
so on.
Great question pankaj.
I would suggest resampling the data to a few different time scales and building a model on lag signals of each, then ensemble the predictions. Alsom build bigger models on lagged signals at each scale You want to give your models every opportunity to exploit the temporal structure in the problem.
It was a great article. My question is not really on this topic.
how can use capture the errors in a neural network for each instance of a data and print it out in java and now to interpolate on the captured errors so predict the errors.
This is great. Though the multi-step forecast is somewhat border me. If we make a data model with features, for example, 3 continuous lag, then it show that somehow, the next step would be build upon the value of these 3 data, like X(t) = a1.X(t-1) + a2.X(t-2) + a3.X(t-3). And what’s more, to predict further into the future, have we extended the width of the window ? In that case, as the number of features also extended, the size of training data also must be extended right ?
That is correct.
There are two general approaches for a multi-step forecast: direct (one model for each future time step to be predicted) and recursive (use the one-step model again and again with predictions as inputs).
Nice article. You are proposing supervised learning for complex time series, instead of classical forecasting methods. Do you have any particular supervised learning method in mind? If so, what makes you think it will work better than NN based LSTM.
You also mentioned, in response to a comment, that some ML techniques are not adversely impacted by correlated input. Can you please shed some light on your comment.
Hi Pranab,
No specific method in mind, more of a methodology of framing time series forecasting as supervised learning, making it available to the suite of linear and nonlinear machine learning algorithms and ensemble methods. Not a new idea for sure.
Sure, often decision trees are unflappable when it comes to irrelevant features and correlated features. In fact, often when there are unknown nonlinear interactions across features, accepting pairwise multicollinearity in input features results in better performing models.
Hello Jason,
This is a cery interesting. topic Have you considred forecasting one-step-ahead as a function of multi steps before. This will represent an output which is a function of several variables. The question of interest, by analogy to the traditionale mult-variate function, is how many variables (back step) to use and which ones are most significant to use through a variable selecion process.Variable selection could identify which time periods influence the analysis and forecat.
This approach can greatly benefit the forecasting and anallysis of time series using all of machine learning algorithms.
A colleague and I applied this approach. Four published papers on this work can be “googled using my name (Hassine Saidane)
Happy continuation and thanks for sharing the article.
Thanks for sharing Hassine.
Hi Jason,
I am trying to predict customer attrition based on revenue trend as time series
Month1 –> $ ; month2 –> $ as training data set.
How can i use predictive algorithm to predict customer attrition based on the above training data ?
Thanks
Sam
I would encourage you to re-read this post, it sells out exactly how to frame your problem Sam.
Thanks for your response Jason.I understood the above example.The above example seems to be predicting Y as regression value.But i am trying to predict Y as classification value (attrition = 1 or non attrition = 0)
Example : Below is the time series of revenue where 1,2,3.. are the months and Y tell us if the customer attrited or not. Y will have only 2 values 1 or 0.
So can i use the below format for my test data ?
revenue1 revenue2 revenue3 …Y
100 50 -25 1
200 100 300 ….. 0
Appreciate your help.
Thanks
Sam
Looks good Sam.
Thanks a ton Jason for your quick response.You made my day 🙂
I’m here to help Sam.
Dear Dr Jason,
Two topics please
(1) On cropping data and applying the model ‘to the real world’. I understand that cropping is done on the 0th and kth data points to get a 1:1 correspondence between data values at t and t-1. I assume from previous posts that you crop say the (k-10)th to kth data points, perform the successive 1 step ahead predictions and select the model based on the min(set of mse of all selected models) of the difference between the test and predicted models.
(a)Is the idea to use the that model to predict the (k+1)th unknown.
(b)Can we assume that the model you ‘trained’ will be acceptable when more data is acquired. In other words, what happens if you collect another x data points, and you want to predict the (k + x + 1) data point, can we assume that the model trained at k data points will work for the model at k + x data points? Or in other words, when do you ‘retrain’ the model.
(2) On windowing the data: based on this blog, is the purpose of windowing the data to find the differences and train the differenced data to find the model. How can we make the assumption that the (k+1)th differenced observation can be predicted from the kth differenced observation.
Thank you,
Anthony from Sydney Australia
Hi Anthony,
Sorry, I don’t understand what you mean by cropping. Perhaps you could give an example?
Generally, we use all available historical data to make a one-step prediction (t+1) or a multi-step prediction (t+1, t+2, …, t+n). This applies when evaluating a model and when new data becomes available.
Windowing is about framing a univariate time series into a supervised learning problem with lag obs as input features. This allows us to use traditional supervised learning algorithms to model the problem and make predictions.
I hope that helps.
Dear Dr Jason,
I will rephrase both (1) and (2) into one.
Perhaps I wasn’t very clear at all.
Cropping. by cropping I mean remove the earliest, the 0th and the latest kth data points because there are no corresponding lagged values by virtue of lagging.
eg
data point value lagged data point array reference
1 ? – this is cropped/pruned 0
2 1 1
3 2 2
44 3 3
5 4 4
. .
560 1234 k-1.
? X – this is cropped/pruned. k
dataset available for processing
datapoint lagged data point (array ref based on original data)
2 1 1
3 2 2
44 3 3
5 44 4
. .
560 1234 k-1
This is the above dataset with the 0th and kth elements cropped/pruned from the original.
I should have been clearer. I apologise.
My questions
(a) Based on the ‘new’ lagged dataset, how can you make a prediction for the (k + 1)th dataset given the kth data point is not available.In other words, are making a prediction for the (k+1)th data point based on the (k-1)th datapoint.
(b) Perhaps I’m missing something, having read the other posts on ARIMA. How can we make the assumption that predicting the next data point is based on the previous data point when there may well be MA or AR or other kinds processes on the data? Or in other words how can we assume that differencingor windowing as in this tutorial/blog will be the basis of our training model?
(c) Suppose you trained your model based on the original dataset. Suppose that as your system acquires more datapoints, won’t the original model that you trained become invalid. Say you got an extra 10 or 1000 datapoints, do you have to retrain your data because the coefficients of the original model may not be an adequate predictor for a larger dataset.
Thank you again and I hope I have been clearer,
Anthony of Sydney Australia
Hi Anthony,
What is k? Is that a time step t? I think it is given context.
If you want to forecast a new data point that is out of sample (t+1) beyond the training dataset, your model will use t-1, … t-n as inputs to make the forecast.
This applied regardless of the type of model used. E.g. if you are using an AR, the inputs will be lagged obs. If MA, the inputs will be an autoregression of the lagged error series.
If differencing is performed in the preparation of the model, it will have to be performed on any new data. The decision to difference or seasonally adjust is based on the data itself and your analysis of temporal structure like trends and seasonality.
Yes, as new data comes in the model will need to be refit. This is not a requirement for all problems, but a good idea. To mimic this real world expectation, we evaluate models in the same way using walk-forward validation that does exactly this – refits a model each time a new ob is available and predicts the next out of sample ob.
I hope this helps. I do cover all of this in my book, lesson by lesson.
Dear Dr Jason, apologies again, my original spaced data set example did not appear neat.
In both the original and the cropped/pruned/windowed datasets, there are meant to be three columns consisting of the data, data lagged by 1, and the array index based on the original dataset.
I don’t know how to get nicely spaced tabbed data when posting replies on this blog
Regards
Anthony of Sydney
You can use the pre HTML tag, e.g.:
[src]https://en.wikipedia.org/wiki/BBCode[/src]
On how to insert BBCode in forum replies
[list]
* 1 ?
* 2 1
* 3 2
* 4 3
* ? 4
[/list]
This is an experiment in inserting HTML code on a forum reply.
I hope this works,
[b] Anthony [/b] [i] from Sydney [/i]
Testing using the ‘pre’ enclosed in ”, inserting “this is a test message”, then ”
Hope it works
Dear Jason,
have you planned any blog on forecasting Multivariate Time Series? I went through your ARIMA post and it was good start point for me.
Thanks,
#student #aspring data analyst
Thnaks Nirikshith.
Yes, I hope to cover multivariate time series forecasting in depth soon.
Jason,
I am new to machine learning. I have a problem type and I was wondering if you could point me to the right area to study so I can learn and apply the appropriate model/technique. I have a set of time series data(rows), composed of a number of different measurements from a process(columns). Think hundreds of sensors, measured each second. I have a hunch that there is a relationship between the columns that is offset in time. Say something happens at time t1 in column 1 and 10 seconds later there is a change in column 2. My desire is to find the columns that have this time relationship and the time between when a change in one column is reflected in the related column(s). My goal would be to then train a model to indicate predictions based on changes in the earlier in time variable prior to the later in time variable changing. Your article is helpful to understand how I might try to train a model to forecast within a single column, but how do I train or dig out the relationships between columns?
If you could point me to what parts of machine learning I should focus my learning efforts I would appreciate it.
Thanks
Bruce
Hi Bruce, time series analysis is a big field. I’d recommend picking up a good practical book.
Generally, consider looking for correlations between specific lags and your output variable. (e.g. correlation plots).
I hope that helps as a start.
Jason,
Thank you, do you have a suggestion for a good book to start with?
Bruce
Yes, my book:
https://machinelearningmastery.com/introduction-to-time-series-forecasting-with-python/
If you are interested in R, here are more books:
https://machinelearningmastery.com/books-on-time-series-forecasting-with-r/
Hi Jason,
Very Nice Article, Just had a question whether there is a forecasting technique for Region/Branch based forecasting.
What do you mean exactly?
Hi Jason,
Thanks for this article. I have 2 questions:
1. Is there a way to avoid removing the rows altogether? If we are creating lag (t-2), (t-3) etc then we will have to remove more rows. I have seen kaggle masters use XGB with missing = NA option so that it handles missing data but not sure what can be done with other models.
2. Can you please shed some light on the fact that data may not be i.i.d. P(Y|X) (may be identical but y|x may not be independent for rows). I think most ML models should fail in this scenario. Am i thinking in the right direction? Also is there a way to check the iid hypothesis?
Regards,
Rishi
Thanks Rishi.
Yes, you can mark the values as NaN values, some algorithms can support this, or set them to 0.0 and mask them. Like xgboost or neural nets.
Great point. Classical methods would not fail, but may fair worse than methods that are adjusted for the dependence. I’d still recommend spot checking a suit of methods on a problem as a baseline. ARIMA is corrected for the dependence (as far as I remember).
Thanks for the reply Jason. I was reading up on auto correlation correction in regression ( detected using Durbin Watson) but that was applicable for continuous data – Cochrane orcutt. Is there in general any way to correct for it? I think most of the problems that we work on in real world are time series such as customer churn etc. And I feel time series regression is what we (unknowingly) do as well, as in use X such as performance in last month etc. Please suggest some material.
Regards,
Rishabh
Sorry, I’m not sure what you’re asking, can you restate your question Rishi?
Let’s say we pick a real life case study, predict customer’s retail spend this month. In this case a person spending amount this month might depend on whether he had a big spend large month or not. Obviously we can have lagged y as X in the model to capture the info but do you think that data will be iid. Residual analysis should give some insight into it for sure (Durbin Watson should also help detect that).
Also problems like customer churn, I always use this approach: fix a timeline lets say 1 Jan, Target is customer who churned in Jan – Feb and X are information from past (spend in last 2 months Dec and Nov for all customers). Variables used are like spend in last x months etc. Does this approach seem right for time series kind of classification?
Sorry for a long post, just wanted to clarify my thoughts.
Yes, I would encourage you to test it empirically rather than getting too bogged down in analysis.
You cannot pick the best algorithm for a specific prediction problem analytically.
Hi Jason,
Superb post!
I have a query. I am working on a real life problem of forecasting 3 days sales for a Retail store.
I am thinking of applying a hybrid model(ARIMAX+Neural network) i.e Dynamic regression with regressors using auto.arima,then fitting Neural network model on the residuals.The final forecast will be y= L+N where L=forecast from ARIMAX and N= forecast of residuals from NNETAR. What do you think of this approach?
Also, I need your input on applying the cross validation techniques. I have daily sales data from Jan14-June17. Would it be worth to tune the parameters using cross validation techniques(Adding months/quarters) or should I go ahead training the model only once (Let’s say from Jan14-Dec16) and measure the accuracy on the rest? (Test & Validation)?What could be the best approach as I need only 3 days forecasts?
It does not matter what I think, use data to make decisions – e.g. the model or combination of models that get the best skill on a robust test harness.
Use walk forward validation on time series, more here:
https://machinelearningmastery.com/backtest-machine-learning-models-time-series-forecasting/
Jason
Excellent article about time series forecast. I have a fair understanding of statistical traditional ML techniques and its application. I have couple of questions on applying NN/LSTM to time series forecast
1. To what an extent we need to worry about over fitting?
2 are there ensemble techniques that apply different models for different time horizons?.
Overfitting is always a problem in applied machine learning.
Not sure I follow. If you have different time horizons, then you will need different models to make those predictions. Perhaps you can use outputs from one model as inputs to another, but I have not seen a structured way to do this – I’d encourage you to experiment.
You can do encode decoder or multi task learning
Hi Jason,
Thanks for the nice and helpful article you have shared. There is this research paper I am trying to implement, based on predicting cloud resource usage. Sliding window technique is required for preprocessing of data and the data is fed to the LSTM as input. For eg. while predicting CPU usage of a particular VM, I have the time series data at an interval of 1min. in the following format:
Timestamp CPU usage
1. t value1
2. t+1 value2
3. t+2 value3
.
.
.
.
and so on, similarly for other parameters as well, such as RAM, DISK, etc.
Could you please guide me with what should be the format of my training and testing sets, if I use LSTM.
Thanks in advance.
This post on backtesting models for time series data might give you some ideas:
https://machinelearningmastery.com/backtest-machine-learning-models-time-series-forecasting/
Hi Jason,
I was wondering is common/good practice to have two windows/lags in a multivariate analysis? Suppose y is correlated with t-1 on x1, but t-5 on x2. Is this possible?
Thanks,
Jeff
Yes, often a fixed window of lag obs are provided across all features. Zero coefficients can be used to zero out features that do not add value.
Hi Jason i am working on multivariate time series data for anomaly detection could you please suggest some algorithms i have tried isolation forest, and ARIMA but ARIMA works only for single variable.
Please help
Perhaps this process will help you work through your problem systematically:
https://machinelearningmastery.com/start-here/#process
Hello Jason,
I have read your article, I would assume as you have said that forecasting a time series as it is shown might work with certain algorithms, as you said LSTM, however, I am analyzing a multivariate regression with random forests predicting a final output as a value based on an attribute vectors, but the nature of RF is that it is not time dependent so, this time window is not required I believe?
Nevertheless, the ML lag obs can be framed as input variables and sometimes stateless (time-unaware) methods can achieve impressive results. Try it and see on your problem.
Hi,
Thank you for a great post! I enjoyed reading it 🙂
This is my firs time trying to solve a time series problem, so you explanations really ease the “where to start” issue.
I have a problem in which i’m trying to find correlation between:
1. 9 facial expressions scores (given: joy 0.9, happy 0.77, angry 0.5 etc) every 3 mil-seconds
2. participates action – move, spin, play music, stop music (close list of options with time stamps)
3. Curiosity score – as measured by various means (questionnaires, behavioral measures) – one score per participant.
The study question: Is there a correlation between the user’s facial expressions and his behavior and his curiosity?
Can you suggest a way to work on this kind of data?
Can you refer me to a post about it? or an article?
Any idea / suggestion / solution will help 🙂
Thank you very much,
Shani
Sounds like you need to load the data as a DataFrame and calculate the correlation between columns.
This post might help:
https://machinelearningmastery.com/understand-machine-learning-data-descriptive-statistics-python/
You can even calculate the correlation directly on the DataFrame:
https://pandas.pydata.org/pandas-docs/stable/generated/pandas.DataFrame.corr.html
Too much usefull !
I used your technic (Multivariate Time Series) to prepare datas.
After running a regression model from these ones, I get awsome prediction precision about daily industry electrical consumption. And I swear the energy demands was really not stable !
Thanks Jason !
Glad to hear it!
please I need your help in the same problem I need to predict patient future vial signs, and I have multi-values in different dates
A good place to get started is here:
https://machinelearningmastery.com/start-here/#deep_learning_time_series
Hello, I don’t understand the following statements:
“We can see that the order between the observations is preserved, and must continue to be preserved when using this dataset to train a supervised model.”
“We can see how once a time series dataset is prepared this way that any of the standard linear and nonlinear machine learning algorithms may be applied, as long as the order of the rows is preserved.”
Why does the order of the rows have to be preserved when training the data? Haven’t you essentially converted the time series data to cross-sectional data once you have included the relevant lags in a given row?
Thank you,
Andrea
No, we are exposing temporal structure as inputs.
I don’t understand the same statement of Andrea and I have one more question.
1) Why does the order of the instances (rows) have to be preserved when training the data?
2) Does this mean that we can not perform k-fold cross validation on the prepared dataset?
Thanks.
In time series the order between observations is important, we want to harness this in the model. It is also a constraint, e.g. we cannot use obs from the future to predict the future.
Correct, we cannot perform k-fold cross validation. We can use walk forward validation instead:
https://machinelearningmastery.com/backtest-machine-learning-models-time-series-forecasting/
Thanks for the patience but i have this specific problem. I have a univariate time series and i want to train a SVM (regression) in order to predict one step ahead. Suppose we have the sequence: 1, 2, 3, 4, 5, 6, 7, 8, 9.
As you suggest, I create the following representation in order to perform supervised learning:
1 2 3 | 4
2 3 4 | 5
3 4 5 | 6
4 5 6 | 7
5 6 7 | 8
6 7 8 | 9
Where the last column is the target. Now I want to train a SVM and I have to choose hyperparameter such as C and best number of input feature so I need k-fold cross validation. I don’t understand the point when you say that the order of the instances (single row of the dataset above) must be preserved during training so we can’t create random samples as folds of k-fold cross validation. In general, if we pick the dataset and train SVM using instances in reversed or random order (first istance is vector 6, 7, 8 with target 9, second vector is 5 6 7 with target 8 and so on) we must obtain the same model.
I found an article in which authors use SVM and ANN for time series forecasting problem and in order to achieve supervised learning they transform time series according to your idea but also they perform k-fold cross validation (random samples) in order to choose best hyperparameters. What do you think about this article (PAGE 7)? http://docsdrive.com/pdfs/ansinet/jas/2010/950-958.pdf
I understand we can’t perform k-fold cross validation of raw time series if we use statistical models (ARIMA, Exponential Smoothing, ecc) so we use walk forward validation and I accept it. But in case of general purpose algorithms such as SVM and ANN if we transform time series data into a data frame for supervised learning with input variables (features) and output variables (target) we can use it as a “normal” dataset for a regression problem where the order is not important in training so which we can random split for train and test.
Thanks for your support!
Yes, excellent point.
If the model has no state (e.g. not an LSTM), then it is just working with input/output pairs. In which case, using k-fold cross-validation may be defendable. It might even be preferred.
This is true as long as the train/test sets were prepared in such a way as to preserve the order of obs through time. E.g. that the model is not learning about the test set during training.
Hi Jason,
Thank you very much for this contribution. Your contribution helped me a lot to understand how to use two powerful tools together. But I have a question. I have a series of data which show seasonality. Would not there be a problem in using this technique or should I first apply a SARIMA model to apply your advice?
Thank you!
I would recommend removing the seasonality first.
Hi Jason! I have three questions regarding the way I’m modeling my problem.
INTRO
I’m trying to predict the demand of different products for a company. I have the day at which the order was registered, the price of the product, size of the order, client id, etc, etc, etc for each order in the past 5 years or more.
EXAMPLE
Here is an oversimplified example I wrote to make it clear:
day | price | size
1 | 80 | 3
2 | 85 | 10
3 | 90 | 5
4 | 100 | 8
5 | 110 | 10
6 | 100 | 12
7 | 90 | 1 <– small size in t=7, maybe this caused the increase in t=10
8 | 100 | 21
9 | 95 | 18
10 | 90 | 50 <– increase
11 | 100 | 25
12 | 100 | 20
13 | 110 | 1 <– small size in t=13, maybe this caused the increase in t=14
14 | 110 | 60
15 | 110 | 27
QUESTION 1
I first tried regression but it's hard to know how well it performs, the model can easily be predicting that the value in t+1 is equal to the value in t plus/minus a random number and the chart would look pretty good anyway, in fact I can approximate the value in t+1 as a simple moving average and that would do it in most cases except during rapid increases which is what I'm trying to detect. How do you evaluate the performance of regression model in this problem?
QUESTION 2
I also tried to model it as a classification problem and here is where I'm stuck. I decided to have two labels: increase and decrease. How do you decide what window size you use? In other words, if I see a rapid increase in t, should I label the sample in t-1 as "increase"? I don't think so, maybe the clue for such a rapid increase is in t-2, or t-10.
I'm afraid that whatever window size I choose, I will be forcing the network to look for a correlation between my inputs and the label at points in which maybe there isn't any correlation to look at. Maybe sometime the label should be in t-1, other times in t-10, t-9, t-8, …, t-1, who knows.
QUESTION 3
The price may change due to inflation and other factors, so the same product may have a price of $30 1 year ago, and $200 next year and that's fine. If I train a model as I described above, shouldn't I do something so all prices are comparable to one another?
Ideally after I train the model I want to to be able to give good predictions regardless of the price level at that time, specially because the test dataset has samples from different periods! This is even worse if I train the model using data of different products where for the same period I would have two products at $100 and $1000, or demands that looks completely different. I have the feeling I should be relativizing those values somehow.
Thank you a lot!
You must choose a way to evaluate a forecast for your problem. It must be meaningful technically and to the stakeholders.
Find out what matters to the stakeholders about a forecast. They might say minimum error. In that case, you could use RMSE or MAE of a forecast to estimate and present the skill of a model.
Start with simple methods such as persistence and moving averages. If a ML method cannot do better than these, it is not skilful and you can move on. More on that here:
https://machinelearningmastery.com/backtest-machine-learning-models-time-series-forecasting/
I would encourage you to explore as many different framings of the problem as you can think up. Framing as a classification problem is a clever idea. See how far you can push it. How to best frame the data or set window size in your case? No one knows, design experiments and discover the answers.
Perhaps look at ACF and PACF plots to get an idea of significant correlations that you can use to help design window sizes. More on that here:
https://machinelearningmastery.com/gentle-introduction-autocorrelation-partial-autocorrelation/
Inflation is a small effect. Nevertheless, you might need to correct data prior to modeling. E.g. transform all dollars to 2018 dollars or similar.
Also, consider modeling by product, by product groups, by all products, etc. Get creative, see what sticks. There are no right answers, only the best results you can discover on your problem given the time and resources you have available.
Does that help as a start?
Thank you for your answers and your prompt reply. I’m not sure about some things you mention, let me ask you some details.
> Find out what matters to the stakeholders about a forecast.
They want to predict spikes in the demand before they occur but the spikes only appear sporadically so in general if you use a moving average the error (RMSE or MAE) is pretty low, but such a simple model also miss all spikes of course.
I’m guessing that’s what the network do for regression. Maybe there’s a loss function I can use in order to penalize very hard differences in the trend (it predicts the demand will go up while it goes down, whatever the value).
> No one knows, design experiments and discover the answers.
I’m arguing that for this problem there should be a more reliable approach that I’m not aware of. In my example no window size will make the labeling correct. This is how I -as a human- would label it assuming a small demand size implies a big demand size in the near future.
day | price | size | label
1 | 80 | 3 | normal
2 | 85 | 10 | normal
3 | 90 | 5 | normal
4 | 100 | 8 | normal
5 | 110 | 10 | normal
6 | 100 | 12 | normal
7 | 90 | 1 | increase (window size 3)
8 | 100 | 20 | normal
9 | 95 | 18 | normal
10 | 90 | 50 | decrease (window size 2)
11 | 100 | 25 | normal
12 | 110 | 1 | increase (window size 2)
13 | 100 | 20 | normal
14 | 110 | 60 | decrease (window size 1)
15 | 110 | 27 | –
As you can see I had to use different window sizes. The problem is in this silly example the labeling is pretty obvious but in reality it’s not, so I thought there was something I can do.
One idea would be to mark the previous n samples before a rapid increase as “increase”, but then the network will look at t=8 and t=9 for instance, and it will try to get some kind of pattern where there’s none. The score will be random and the performance (as in precision/recall) difficult to read!
Makes sense? No idea how to tackle this.
> you might need to correct data prior to modeling
Regarding adding multiple products in the same dataset (or one product in different periods). Not sure it’s used in the industry but I thought about substracting a given value the moving average of the previous N values, so if the price or demand tend to increase over time as a natural process I’ll only see the difference of it against previous values.
I can’t think of any other way to put together products of different price ranges in the same dataset.
Interesting.
Matt, it’s supposed to be a slog/hard work, this is the job: figuring out how to frame the problem and what works best. Running code is the easy part.
Based on this info, I would recommend looking into framing the problem as anomaly detection, perhaps a classification problem where you predict whether a spike is expected in the next interval of time. This might allow you to capture the precursors to the spike and simplify the spike such that you are not predicting the magnitude only the occurrence (simpler). If this works to any degree, you can then later see if the magnitude can be predicted also.
Also, some problems are not predictable. Or not predictable with the data/resources available. Keep this in mind.
Let me know how you go.
On a second thought I think this problem is analogous to predicting movements in the stock market.
Labeling my samples would be equivalent to labeling bars before a spike in the price of a stock. In that case I guess the correct place to put the “spike” label is right before it occurs and not an arbitrary amount of time before it (let’s say 15 minutes).
LSTM should be able to learn the correct dependency even if the catalyst for the spike is not the bar I labeled as “spike”. Right?
LSTMs are poor at autoregression and I am not knee deep in your data. I cannot say anything will work for sure. You’re the expert on your problem and you must discover these answers.
LSTMs __may__ be useful at classifying a sequence of obs and indicating whether an event is imminent. A ton of prior examples would be required though.
Hello Jason,
Is there a way to predict the state variance using LSTMs?
Thank you in advance.
What do you mean by state variance?
Hi Jason,
Great post.
i want to predict the turnover ( in percentage) for candidates for HR analytics for next 6 months. The factors are joining date, age, gender, overtime, commute time, rewards in last year, years in current service etc. Now i want to ask that :
1) Is this a time series problem or a classification problem.
If i do classification then how can i proceed for turnover predictions for upcoming months and if i proceed with time series than how will i take the other factors into consideration.Please advise.
You could frame this as sequence prediction or not. I would recommend exploring both approaches and see what works best for your specific data.
Jason thanks for the reply but the main question is how can we predict for lets say future 1st ,2nd and 3rd months consecutively as i need to predict the percentage turnover for next 3 months. Could you please guide me. I have different independent variables like date of joining, date of leaving, gender, salary, overtime etc
Is this goes like if i have the data for past 3 months then the prediction is for the 4th month. Now to consider the 5th months do i need to merge the past 3 + future 1 month data so as to predict for the 5th month ?
I have gone through a lot of blogs but nowhere it is clearly mentioned. I think it will also help others.
I think you are describing multi-step forecasting. You can learn more here:
https://machinelearningmastery.com/multi-step-time-series-forecasting/
Does that help?
Hello Jason,
I am enjoying your blogs and the two ebooks on time-series. I have been attempting to train an LSTM with a look_back value assigned. After training and testing, the plotted results have a gap equal to the look_back interval between the final training result and the first test result. Is there a way to use the train/test size split instruction to force overlap between the training dataset and the test dataset? I would like to see the first few results from the test data, even though it would be exposing the network to data previously trained on (at least part of the look_back range). I could prepare separate .csv files for training and test, but was wondering if there was a simpler way to accomplish this. Thanks
Great question.
I wrote a function to prepare data for this, you can see it here:
https://machinelearningmastery.com/convert-time-series-supervised-learning-problem-python/
Let me know how you go.
The time series data samples generated by the sliding window method could not be expected to be i.i.d. (independent, identically distributed random variables) in general, so that strategy for turning time series data into training data for a standard supervised learning classifier seems questionable. At least one other seems to have brought this up in another comment above (but stated it somewhat differently).
They will not be IID, and many supervised learning methods do not make this assumption directly.
Further the approach can prove very effective for some problems.
But don’t you think these assumptions must be respected. Additionally, here we are dealing with numerical algorithms which will give us some numbers at the end,but the question is, are those number correct?Also, can you shed some light on the nature of problems where these approaches were effective..Thanks
Nope. I will respect the rules but break them all if it means I get better predictive performance.
In predictive modeling model performance is more important than “correctness”. We are not trying to understand the domain, we are trying to predict it. Understanding is a different problem called “analysis”.
Hello Jason,
I find your articles in https://machinelearningmastery.com/time-series-forecasting-supervised-learning/ and https://machinelearningmastery.com/convert-time-series-supervised-learning-problem-python/ superb! It’s my first time encountering articles talking about lagged values as detailed and concise as yours.
However, after reading your article in here -> https://machinelearningmastery.com/backtest-machine-learning-models-time-series-forecasting/, I became a bit confused.
I hope you won’t be too bothered by my question since I’m a newbie in this area. Trivial as it may seems, I’ve been stuck with this problem for the longest time.
You see, I’m using a sliding window method on my univariate time series dataset, which will be fed to feed-forward ANN for forecasting.
The problem is that, when using ANN, we’re required to split the data into Train-Test set. So, I was wondering if I should first restructure the data into a supervised learning problem and then split the data into train and test sets, or should I split the data first and then use sliding windows on the train and test data separately? You mentioned about respecting the “temporal order of observations” in your other article, but I couldn’t quite catch the meaning behind word.
I hope you can shed some light on this problem for me. Your help is very much appreciated. Thank you in advance!
Thanks.
Yes, structure the data as a supervised learning problem then split it into train/test.
Does that help? Any further confusions?
Hi Dr. Jason,
Thanks for the wonderful article.
I was wondering if there is an algorithm which will forecast based on independent variables.
I have 12 month of data with 30 features, I want to predict for the next 3-6 months ( dependent variable) but I don’t have independent variables for the future so I can’t use conventional forecasting techniques like multivariate forecasting model.
I recommend framing the data as a supervised learning problem then test a suite of machine learning algorithms.
This post will help you to get started:
https://machinelearningmastery.com/convert-time-series-supervised-learning-problem-python/
Uahuuuh, Thanks Jason and all the community. I am learning from both the post and all the questions/answers ! I really appreciate it.
I am completely a newbie and I am tackling a capacity plan problem. Basically I have to create a ML/AI system that can forecast how many Compute instances need to run during the day based on previous data to cope with all the incoming requests. Because the instance will take some time to be ready I cannot rely on real-time autoscaling.
The problem is surely a multi-variate because in the game I have multiple regions ( 3 ) and the capacity plan should consider that one region can completely fail while the others would manage the increased traffic.
In mine idea the features will be:
– QPS ( query per seconds ) x Region
– Total QPS worldwide
– Day of the week
– Day of the year
The forecast would be how many QPS I should have to manage all the incoming traffic. After I have the QPS I can say how many instances I need.
I think that a time-series forecast would help me. Can you give me any hints or suggestion on how to tackle the problem?
Another concern I have is how to transfer the knowledge from the previous data analysis to the next analysis without crunching all the data from the beginning. Imaging that I will run the model every hour and I need to do a multistep ( 2-3 ) forecast and I have already years of back data I would avoid, if possible, to crunch all the data from the beginning at every run.
Thanks
Alessandro
Sounds like a great problem. I recommend starting with a simple ml method, e.g. frame as supervised learning and test a ton of methods from sklearn.
I am currently writing a ton of tutorials on this topic. They should be up soon.
HI Jason,
can you share the tutorial’s title you have in mind. So I will check them out.
Thanks
Thanks for the offer.
Hi Jason,
I have a question for you. Assume there is a correlation between attributes in time series data, then is there any restriction on the choice of algorithms to apply,
What solutions would you recommend if there are missing values in time series data? why?
Here’s help with missing data in time series:
https://machinelearningmastery.com/handle-missing-timesteps-sequence-prediction-problems-python/
hi Jason,
Suppose we have multivariate time series data but the quantity of data is small,could you suggest any semi supervised deep learning model for the following problem
1 ) Regression problem
2 ) Classification problem
Perhaps try transfer learning with a model fit on a lot more time series data?
Hi Jason
Any regression model needs the sample points to be independent of one another. But due to autocorrelation, this does not seem possible here.Because the value at time period t is dependent on the previous values. How do you account for this dependence. There were questions asked around this, but I didnt really understand. Could you please explain this again
A slightly different version of linear regression is fit to account for this.
Perhaps pick up a good book on the math, e.g.:
https://amzn.to/2xbgFYS
Hii Jason,
Fantastic article ,I have some questions:
I still not understand how to predict Multivariate Time Series by SVM.
In your example :
X1, X2, y1, y2
?, ?, 0.2, 88
0.2, 88, 0.5, 89
0.5, 89, 0.7, 87
0.7, 87, 0.4, 88
0.4, 88, 1.0, 90
How to predict y1 and y2?, SVM can predict only one value.
2. I the following example , I think the number of input features need to be 4, because you have 2 origin features and each of them you predict one step back , so 2*2=4
X1, X2, X3, y
?, ?, 0.2 , 88
0.2, 88, 0.5, 89
0.5, 89, 0.7, 87
0.7, 87, 0.4, 88
0.4, 88, 1.0, 90
1.0, 90, ?, ?
3.In this method,the model have only the ability to create connection only for N sample as sequence ?
Dear Mr.Jason,
Thank you because of your useful sharing.
If I want to use the sliding window method to change the time series data to regression data.
x1 x2 … xm
x2 x3 … xm+1
.
.
.
xN-m xN-m+1… xN-1
So, I only use one window and the window size is xN-m right?
This method is call sliding window or only window method?
The tutorial above does describe a sliding window method with overlap.
This is the best explanation of why to use lags I’ve seen.
Where do you draw the line though with how many previous values to include? Would the inclusion of many lags help to model seasonality? Seasonality sets an objective envelope on forecasting values but it’s not clear to me how a supervised model can apply or even discover seasonality as it cannot be derived from a single observation.
One approach is to use correlation (e.g. an ACF/PACF plot) to choose the number of significant lags:
https://machinelearningmastery.com/gentle-introduction-autocorrelation-partial-autocorrelation/
Another approach is to grid search different lags to see what works best.
Hi Jason,
Great article to move towards ML.
We have a volume forecast problem for a toy company. Could you please help me point out any specific inputs on how to start using ML to forecast volume or sales in retail setup.
Perhaps this process will help:
https://machinelearningmastery.com/start-here/#process
Hi Jason,
Thanks for the great tutorials.
I have the following timeseries forecasting problem. I want to predict the value of var1 in t+1 given 3 timesteps in the past (t,t-1,t-2) and I have the data as shown below:
var1 var2 vark
sensor 1 (8:00am) …
sensor 1 (9:00am) …
sensor 1 (10:00am) …
sensor 2 (8:00am) …
sensor 2 (9:00am) …
sensor 2 (10:00am) …
sensor k (8:00am) …
sensor k (9:00am) …
sensor k (10:00am) … ….
If I reframe this problem as a supervised learning problem by creating lagged features for (t,t-1,t-2) the resulting dataframe would be something like this:
var1-t var2-t vark-t var1-t-1 var2-t-1 varkt-1
var1-t-2 var2-t-2 vark-t-2 ->> var1-t+1 var2-t+1 vark-t+1
sensor 1
sensor 1
sensor 1
sensor 2
sensor 2 …
sensor 2 …
sensor k …
sensor k …
sensor k … ….
I am clear how to solve the problem for data coming from one sensor (using the info shown in your tutorials). However if more than one sensor is involved:
– Would you recommend one model per sensor or one model trained on data coming from all the sensors assuming they behave similar?
– In case of using one model for all the sensors how can I put the data from all the
sensors together to train the model.? Should I create lagged features of each sensors and
concatenate them as rows (as shown before) or instead as a new set of features (columns) ?
PD: I think this problem is similar to the one described here:
https://machinelearningmastery.com/how-to-develop-machine-learning-models-for-multivariate-multi-step-air-pollution-time-series-forecasting/
Thanks in advance!
Try a suite of framings of the problem and discover what works best.
I have some ideas here that might also be worth exploring:
https://machinelearningmastery.com/faq/single-faq/how-to-develop-forecast-models-for-multiple-sites
Hi Jason,
Thanks for prompt your reply.
I will have a look!!!
Related to my previous post the other alternative is each row in a dataset could be the complete sequence:
var 1(t) var2(t) var3(t) var 1(t-1) var2(t-1) var3(t-1)
var 1(t-2) var2(t-2) var3(t-2)…..var 1(t-n) var2(t-n) var3(t-n) ->>
var 1(t+1) var2(t+1) var3(t+1)
sensor 1
sensor 2
Which one is a better approach?? Is there any tutorial in the website where you have implemented a similar case?. It would be nice having the seeing the series_to_supervised function modified for this kind of scenario where multiple sites, products, etc are required..
Thanks in advance!
Use prototypes and real results to _discover_ what is better for your specific problem.
Hello Jason,
After using the sliding window method, can we use the classical (Pearson) coorelational matrix on the data? Also should we use Walk Forward Validation instead of Cross Validation even though we converted sequential problem to a supervised learning problem?
Yes, this is called an ACF plot:
https://machinelearningmastery.com/gentle-introduction-autocorrelation-partial-autocorrelation/
Hello Jason, Thank you for the reply.
However, I read in multiple posts ( eg: https://stats.stackexchange.com/questions/133155/how-to-use-pearson-correlation-correctly-with-time-series) that Pearson Coefficient does not make sense with time series data. Consider me a novice in this field, but after using a sliding window method to convert the problem into time series problem, does it make sense to use the Pearson Correlation coefficient to find relationships? Please note that I am using multivariate time series data.
I want to find relationships not only between lags but also among other time series columns in the data.
As far as I understand, the ACF and PACF plots are a standard approach and correlation (serial correlation) does make sense.
I cannot not familiar with the link you have posted, perhaps if you have questions about it you can contact the author.
Thanks for the post.
Suppose I have a uni-variate time series data, what is the best way to do multi step forecasting like for example 30 steps.
Most models will let you forecast multiple steps via the forecast() function, just specify the number of steps.
Try a range of models, find one that does the best.
Perhaps start here:
https://machinelearningmastery.com/time-series-forecasting-methods-in-python-cheat-sheet/
I want predict stock values for next five days using svr in python. Please can you recommend me a way to do this.
This is a common question that I answer here:
https://machinelearningmastery.com/faq/single-faq/can-you-help-me-with-machine-learning-for-finance-or-the-stock-market
Hi Jason ,
Nice Tutorial !
I am actually working on converting time series dataset to multi-classification supervised machine learning problem .
Each class is represented by different time series . the different classes have different time series lengths.
I have problem to select the best or the right lag observation or sliding window that works for the different classes.
As for the first class the lag observation is between 10 – 30 years and for the second class window sliding is around 100 years and for the third class is less than 10 hours
I do not know how I should deal with this problem , Shall I train each class separate or should I choose an unique window sliding working for the three classes or
what is the best approach to deal with this problem ? or should I try other time series multi classification approach such as Dynamic warping time or shapelet transform or Hidden Markov approach …?
Many thanks for your advice and your help !
I recommend testing different sized windows and history input in order to discover what works best for your data and model.
Hello Jason,
Great posts. Please help me with your inputs for a query. I have to predict No. of Building Fire alarms per day based on the data – Date and No. of Fire alarms received on that day. I have data for around 6 months from June to November 2018. I used ARIMA time series forecasting method (following your posts) to predict the no. of alarms per day in future like dec 2018, jan 2019 next etc.
But I was thinking, whether it makes sense to predict no. of fire alarms in future based on the no. of fire alarms in past? Fire alarms are not seasonal etc. They depend on faults which might be coming from various IOT sensors. I think I should try to get more related data(more no. of features).
Please suggest how can I frame this problem and go about solving this.
Ragards.
What do you think. What makes most sense to solve this type of problem.
Perhaps try framing it a few different ways, prototype each and go with the approach that results in the most skillful predictions.
Hi Jason,
Suppose a time series like
1 2 1 3 2 1 1 1
Converting it to supervised learning using lag of 4, it will be
1 2 1 3 2
2 1 3 2 1
1 3 2 1 1
3 2 1 1 1
where the last column is the output to predict at time t
Now using this only the model has high error. So I find the diff of successive time steps.
The original time series now converted like this
1 -1 2 -1 -1
-1 2 -1 -1 0
2 -1 -1 0 0
where positive number shows the trend increases, zero no change and negative means decreases.
Now I apply machine learning algorithm and suppose predict the output for the last column as
-1.5
0.2
0.3
Now my question is about going back to the original values. Is it like below
2 + (-1.5) = 0.5
1 + (0.2) = 1.2
1 + (0.3) = 1.3
Or I need to have cumulative sum like
2 + (-1.5) = 0.5
0.5 + (0.2) = 0.7
0.7 + (0.3) = 1.0
The series would need to be diff’ed before being shifted.
A prediction can invert the diff operation by adding the value prior, perhaps from the original time series?
I give an example here I believe:
https://machinelearningmastery.com/machine-learning-data-transforms-for-time-series-forecasting/
Thanks.
After reading that and 2 other posts I know now that it is difference transform. In my above example I think I’m doing the same by taking difference first and then shifting. Do you want to point something else here which I didn’t get?
I saw in one of your answer we can use either the actual or predicted value for inversion. Did I get it correct? Actually I’ll be rounding the values after this to make it like classification problem. The result will change maybe little but with some effect on accuracy. I’ve read your regression vs classification posts as well and it seems to me ok but your answer will give me more confidence.
If it is a time series classification problem, then there is no need to invert differencing of the predicted value as there would not be a linear relationship between the values.
So what you are saying is that after difference transform I run the algorithm and then compare the predicted output with the transformed output. What if I want to report in terms of original classes? My actual values are integers but my model gives me real/double numbers. So I use MSE and also want to see the accuracy after rounding the double numbers. Do you suggest any better idea other than rounding to calculate accuracy as rounding error sometimes can show misclassification or vice versa.
Also do you have any example for predicting the probabilities in classification problem?
If you want to calculate an error, then both original values and predictions must have the same scale.
It does not make sense to calculate error for a classification problem via MSE.
One might calculate accuracy, or loss between two sets of probabilities via log loss or similar. Probabilities would not be integer values.
For more on the difference between regression and classification, see this:
https://machinelearningmastery.com/faq/single-faq/what-is-the-difference-between-classification-and-regression
Thanks and I’ve read that post earlier which makes clear about the difference between regression and classification.
I use fuzzy logic which provides crisp value as double number and then I round it to see whether it is correctly classified or not. That’s why I need some insight about difference transform. Because when we do this transform the scale becomes small and then when I inverse transform the diff. between actual and predicted one is small and rounding gives good accuracy. Sorry as my explanation might not be good.
Hi Jason,
If I’m developing some patient care prediction system with each patient has time series and for example one patient has this time series
1 2 3 4 5
and another one like
5 6 7 8 9
Now using lag of 2 we get for patient 1
1 2 3
2 3 4
3 4 5
and for patient 2
5 6 7
6 7 8
7 8 9
Now my question is if I combine these and many other patients and apply some ML algorithm does it make sense? Is it we are developing some averaging algorithm for all responses. So when the new patients come as a test case then we apply this model and get some prediction.
Is this same for auto correlation to find out significant lags? Can I combine all and try to find correlation or it must be done patient wise?
Lastly since each patient has very small time steps, which method you suggest if I want to do prediction patient wise as MLP need lot of data. What about ARIMA or other simple algorithm?
I tried LSTM but for plotting it cannot be like one time series and must be done for each patient.
It might make sense if you are looking to model across cases/patients.
It comes down to what you want the model to do/to learn.
Autocorrelation is a great tool to find significant lags.
I recommend testing a range of methods, for example:
https://machinelearningmastery.com/how-to-develop-a-skilful-time-series-forecasting-model/
I use timeseries forecasting in WEKA in the same method that you kindly explain above. I try to predict electron flux in space with the lag values of the flux in advance one day by using Linear regression, Multilayer perceptron, and SMOreg. Unfortunately, the prediction is out of phase of the validate data about 1 day in all the three methods; the predict is faster than the observed data a day. I do not understand this. Is it dataset shift or error? Please explain about this, it is very important .
It may be that the model has learned to perform persistence, this might help:
https://machinelearningmastery.com/faq/single-faq/why-is-my-forecasted-time-series-right-behind-the-actual-time-series
Thank you so much. Because I use neural network, this means that
the model requires further tuning or
the chosen model cannot address specific dataset or
It might also mean that the time series problem is not predictable, right?.
Ia there any simpler way to fix the problem. Please suggest me.
Faithfully,
Correct.
Yes, perhaps some of the methods here:
https://machinelearningmastery.com/start-here/#better
Hi Jason,
I read the article and its very meaningful.
So i have a question about the above example of two observation:
X1, X2, X3, y
?, ?, 0.2 , 88
0.2, 88, 0.5, 89
0.5, 89, 0.7, 87
0.7, 87, 0.4, 88
0.4, 88, 1.0, 90
1.0, 90, ?, ?
I have to feed the values of (X1,X2,X3) as input ‘X’ and values of (y) as output ‘Y’ to the LSTM model like:
model.fit(X ,Y ,….).
Am i right?
Thanks
Yes.
I have another question in mind.
time, measure1, measure2
1, 0.2, 88
2, 0.5, 89
3, 0.7, 87
4, 0.4, 88
5, 1.0, 90
This example have shape1 = (1 input feature , 1 output).
After changed it into supervised learning:
X1, X2, X3, y
?, ?, 0.2 , 88
0.2, 88, 0.5, 89
0.5, 89, 0.7, 87
0.7, 87, 0.4, 88
0.4, 88, 1.0, 90
1.0, 90, ?, ?
Now it shape2 = (3 input feature , 1 timestamp , 1 output).
So my question is that when i train the model with shape2 and save it with 3 input features but later when i load it again for predicting the unseen data which have 1 input feature because unseen data have no timestamp(X1,X2) and not predicted/output variable(y).
And it will be problem after load and making prediction with different input features.
What should we do for it?
Thanks in advance for giving time.
You will have prior data from the train set you can use as inputs for predicting the next value on the test set or on real data.
Sorry i don’t understand about prior data from the train set.
if i want to predict the output (y) for input(X3) = 1.2. (this is my real data).
What should be the value of (X1,X2) from the train set because the train set will contain many rows?
It depends on the framing of your problem.
If the problem takes the two prior time steps and predicts the subsequent time step, then the input will be the two prior time steps.
If the prior time steps are observations in the training dataset, then you will need to retrieve them.
Hi Jason
I am currently working on time series classification of sensor data. I have achieved a good enough accuracy in the classification of the data.
As the second step I am being given system metrics and its values. I understand the sensor data will be affected by the system metrics, but am having a hard time to visualize how I should relate the two while applying any models.
Perhaps provide them both as input features?
I spoke to the guy who made the data sets.
I was trying to figure out anomalies in the data. The two data sets were used to identify different kinds of anomalies and are independent.
Thank you for the reply
Hi Jason
I’m working on machine learning models and I would like to incorporate the time series into my data set as a descriptor, not as a predictor. It’s possible?
I don’t see why not.
only changing the class of the variables with st() of my data set the models know that to do with this type of variables?
Sorry, I don’t understand the question, can you rephrase it please?
Hi, I’m new to time series data
I have a data set of input (18,24,2) which is (number of samples, time_steps, number of features) and output: (18,1), and it is hard to deal with this type of data.
I trained my model in LSTM, but it didn’t give me good performance, I assume it is because of the small data. Is there any other model I can train my data with to get good performance or even to compare it the LSTM performance
Yes, I recommend this process:
https://machinelearningmastery.com/how-to-develop-a-skilful-time-series-forecasting-model/
HI Jason, Thanks for nice post.
I have a question. As you know most of TS in real world are not stationary. You need to make them stationary (Tranformation, diff, …). I haven’t seen this step in your post. Do you mean by using window method and then using ML we can skip this step?
You can use differencing to remove trend and seasonality and a power transform to remove changes in variance.
A good place to start is here:
https://machinelearningmastery.com/machine-learning-data-transforms-for-time-series-forecasting/
So, These 4 methods (Differencing, Transformation, standardization,…) are optional in ML and there is no need to convert to a stationary model before applying ML, like ARIMA. Is it correct?
It depends on the specifics of the data. Try with and without a given transform and compare the skill of the resulting model.
Many models don’t require the data to be stationary, e.g. they learn the trend/seasonality, although many methods perform better if the data is stationary.
Hello Jason, First of all, tons of thanks to you for such an awesome post.
I want to share my problem and want some idea. I have a power plant dataset where I am getting 7 different data from 7 different sensors for each minute. And I have to predict data of the 8th sensor. As the problem is not only dependent on time but also other different variables so that I can say it is a Multivariate time series problem. In my case, I am assuming that I have training data from 8 am to 10 am(120 minutes) and I want to predict data from 10 a to 11 am (every minute of 1 hour, and also every 5 minutes of 1 hour). Unfortunately, I couldn’t get any structured way to get rid of this problem. The problem is I haven’t understood how can I make my train dataset and test dataset.
I have made my DateTime as the index of the dataset. If you could please provide me with any link or idea where I can get some resource about solving multivariate time series(one step forecast, multistep forecast) I will be very grateful to you.
Another thing, If my dataset has 10000 rows(minutes) and I have 8 sensors data(where 7 will act as input feature and the last one is the targetted one) then if I say—
dataset=[sensor 1,2,3,4,5,6,7,8]
train_X=dataset[:8000,:7]
train_y=dataset[:8000,7]
test_X=dataset[8000:,:7]
test_y=dataset[8000:,7] is it correct ?? I am asking it because will I make array like this first and then apply sliding window method OR, is there completely separate idea to make train and test array to train and test the model?
I have read your https://machinelearningmastery.com/multivariate-time-series-forecasting-lstms-keras/ post also.
My best advice is to start here:
https://machinelearningmastery.com/start-here/#deep_learning_time_series
Specifically, the process, and also the tutorials on power prediction will be very useful.
Thanks for your reply
I have a question which is if your window has a continuous value within it, like for example,in ECG wave, brain wave,(there are sharp spikes) to a finite vector? If you use one-to-one mapping,it seems impossible to convert it to a finite vector
You can operate on overlapping windows of input data.
Hello Jason,
I have a question in relation to the way you re-frame the multivariate dataset.
Originally it looks like this:
time, measure1, measure2
1, 0.2, 88
2, 0.5, 89
3, 0.7, 87
4, 0.4, 88
5, 1.0, 90
After you re-frame it, it looks like this:
X1, X2, X3, y
?, ?, 0.2 , 88
0.2, 88, 0.5, 89
0.5, 89, 0.7, 87
0.7, 87, 0.4, 88
0.4, 88, 1.0, 90
1.0, 90, ?, ?
If measure2 is the variable we want to predict and our window width = 1, why is it that the re-framed dataset does not look like this:
X1-1, X2-1, y
?, ?, 88
0.2, 88, 89
0.5, 89, 87
0.7, 87, 88
0.4, 88, 90
1.0, 90, ?
I thought that re-framing a dataset using a window width of one means that you replace you your X variables at time t with X variables that correspond to t-1 (or whatever window width you choose).
What is confusing me is the fact that you kept measure1 (later defined as X3) instead of removing it and having somehing like what I showed in my example.
Thanks for your help.
Good question, it really comes down to how you want to frame the problem.
Both a different and valid framings. You must choose what inputs you want and what outputs, and this applies to lagged observations not just the variables themselves.
Some of the exotic examples in this post may help to make the point:
https://machinelearningmastery.com/how-to-develop-lstm-models-for-time-series-forecasting/
Thank you for your reply.
I have decided to use the approach I suggested above and I have implemented KNN and Gaussian Process regrission with that framing, however, for some reason my predictions seem to be on step ahead of where they should be. My predictions are actually quite good in terms of accuracy, the only problem is that they seem to be shifted ahead and do not correspond to the expected Y. I guess this behaviour is not normal. Do you have any recommendation on how to deal with this problem ?
Thanks!!!
This sounds like the model has learned a persistence forecast:
https://machinelearningmastery.com/faq/single-faq/why-is-my-forecasted-time-series-right-behind-the-actual-time-series
Hi Jason,
It was a helpful article! I just had a little confusion what is the difference between multi-step forecast and multi window width. How would the data look with multi window width.
Thx!
What is “multi window width”?
Hello Jason Sir,
I’m a college student and doing an internship online but i have lack of confidence there because i have no guide to complete that can you help me there that i’m in right way or not.
It would be a great help for me.
my gmail id is cv091998@gmail.com and my linkedin profile is https://www.linkedin.com/in/chirag-verma-205005159/
You can get started with time series and start building your confidence here:
https://machinelearningmastery.com/start-here/#timeseries
Hi Jason,
1. To prepare a time series data to supervised machine learning data for time series forecasting using machine learning algo’s. The new lagged variables should be formed from target variable only and not the predictor variables?
2. Till what lag we should for the new variable for model t-1,t-2……? Should we also use t+1 also ?
Test a range of different lags to see what works best, or use an ACF/PACF plot to get some ideas:
https://machinelearningmastery.com/gentle-introduction-autocorrelation-partial-autocorrelation/
Hi Jason,
I have a demand forecasting problem to solve. I have time series for several products and I should conduct a multi-step forecast for all of them. Ideally, I would like the products to exchange cross-series information. So after reading your blog post, I assume my problem can be classified as a multivariate multi-step forecast, right? I was thinking of using LSTMs to solve that. Can you give me any tips on how to proceed on that problem? I would be very grateful.
Best regards,
Ali
Sounds great.
I recommend this framework:
https://machinelearningmastery.com/how-to-develop-a-skilful-time-series-forecasting-model/
Then these tutorials:
https://machinelearningmastery.com/start-here/#deep_learning_time_series
Thank you very much!
You’re welcome.
Let me know how you go.
Hi Jason,
I’m currently working on a multivariate multi-step regression problem. Basically I want to forecast the electricity price for the day-ahead or the next 24 hours. I have system load information, electricity price as well as other exogenous factors recorded at hourly intervals and I assume was recorded in real-time as well as their time stamps. I did some coding, but I’m getting a bit confused when it comes to the time-shifts. I have several questions related to this:
1) I included lagged system load and electricity prices for my input: specifically these are 24 hour previous, as well as 24 hour previous SMA, and a week lagged. Now do I have to apply a negative shift of 24 steps (shift to the future) for the target electricity price as well? I’m really confused about this.
2) Also can shifting 24 hours into the future (negative shift) be a valid way to produce so-called day-ahead forecasts from real-time records to be used as a predictor?
3) Is it valid to use a predictor alongside its lagged equivalents? I used system load with its lagged counterparts.
Thank you for reading and for this blog. I would gladly support you by buying your books but unfortunately I’m currently recuperating from a work-related injury and money has been tight. In the meantime I’m trying to learn how to code, just in case I, and your blog really helps. Keep it up, and thank you again.
This function will help you prepare the data:
https://machinelearningmastery.com/convert-time-series-supervised-learning-problem-python/
You can see many worked examples here that you can use as a starting point and adapt:
https://machinelearningmastery.com/start-here/#deep_learning_time_series
Addendum:
I have been thinking, but I might have some intuition on the first question:
Say, I’m just forecasting for 1 step ahead, and I have a lag input of 1-step alongside other inputs that aren’t lagged. Its like this:
[[ inputs ]] [[ target ]]
t-1 t t+1
x-1 x, a, b y
Present (t) can be thought of as forecast of the Past (t-1). Day-ahead or tomorrow is the Future (t+1) which is predicted by present (and past). Thus, I do have to apply a negative shift or a shift to the future for the target, alongside the shifts for the lag.
Does this make sense?
Thank you for reading.
messed up formatting:
[[ inputs ]] ….[[ target ]]
t-1 ……t ………..t+1
x-1… x, a, b ……y
Both x-1, x, a, and b are part of inputs
This will help when thinking about shifts:
https://machinelearningmastery.com/time-series-forecasting-supervised-learning/
Hello Jason, I like your site very much. I have learned a lot from it. Thanks for all your contributions!
I have one question. So once you’ve done all your feature engineering and created all your lagged values etc. How do you do the future forecasting? as you won’t have future lagged values. I still don’t understand this part. Should I forecast one day ahead t+1 and then use that forecast to create a future lagged value and use them to forecast t + 2? Or is it possible to forecast multiple steps ahead at once?
Thanks a lot Jason!!!
Thanks!
Fit the model on all available data and start forecasting. Perhaps this will help:
https://machinelearningmastery.com/make-sample-forecasts-arima-python/
Yes, you can do a multi-step forecast directly. More here:
https://machinelearningmastery.com/multi-step-time-series-forecasting/
Thank you Jason!
You’re welcome.
I am trying to understand all aspects of “windowing” . Your article is great by emphasizing transforming the data and windows, but can you explain the possibilities when it comes to forecasting(y) from (x) where x or y are vectors wrt to windowing:
I see it as the following:
1) Given a sequence S and a value s of S, we can forecast “n” values past s using “m” values before s.
2) In this case x has “m” values and y has “n” values
This would akin to a multivariate model of predicting n values from m features
As I understand your article, we are generating several x and y’s by windowing across the series S. The window sizes do not need to be same for before or after a value of s of S, and we could even vary the window size as the window traverses the sequence S…is this correct? Are there technical terms already formalized that capture these concepts? Don’t want to rediscover the wheel.
The window sizes are kept constant in size, e.g. 5 inputs or 10 inputs, where each input is a lag ob, e.g. t-1, t-2, etc.
Not sure what you mean technical by technical concepts? There’s not a lot to this.
I guess I am a bit confused on how the forecasting is using past values. I think I am correct on on how using windowing on a single series we can translate that into a multivariate linear model (given the residual patterns work out correctly) where we are forecasting, say 5 outputs for say 8 inputs. Since the windows stay fixed, then we have an instance of this model for every shift(lag)in the window. Is this correct?
But what if we have two series, then we a collection of multivariate models, one for each series?
Yes.
Yes.
You can see many examples on the blog, perhaps try it with your own data and this function:
https://machinelearningmastery.com/convert-time-series-supervised-learning-problem-python/
Hey,
Can you please tell me what is Fixed effect and Random effect model? How to make out that when to use fixed effect and random effect model? What are the examples of fixed effect and Random effect models?
Thanks in Advance
Sorry, I don’t have tutorials on this topic. I cannot give you good off the cuff advice.
Hello,
As ARIMA model uses linear regression modelling.
Hence linear regression has few assumption one of them is that the data should not have autocorrelation.
Now my questions are as follows-
1. Why does ARIMA model use Autocorrelation in modelling, when data should not have autocorrelation in it?
2. How the autocorrelation is avoided in the ARIMA model, by differencing, detrending or deseasoning the data?
3. Why do we detrend, deseason or use differencing in ARIMA model?
The model is an autocorrelation model, e.g. lag obs are correlated with current obs.
We don’t avoid it, it is a base assumption for the approach.
We remove obvious structures like trend and cycles so the model can focus on the signal in the series.
Does non stationary data is hetroscedastic in nature. Thats why we use detrending and deseasonality in data to make it stationary ?
Not quite.
In ARIMA model we take univariate variable as input. Now I want to know, does ARIMA model create three new independent variables of the input univariate and then do the operation like – AR on 1st variable, diffenecing on 2nd variable and MA on the 3rd variable ? Or all the operations i.e AR, differencing and MA is done on the same input univariate only.
Yes, depending on the arguments of the model, e.g. p/q values.
Here I have speciefied two arguments i.e.
1. It creates single variable or
2 Does it create multiple variables.
Kindly be specific.
Yes, p and q define the number of AR and MA inputs to use. d controls the number of difference operations applied to AR and MA inputs.
Hi its really nice and i love your all ML stuff , so in this article how do we forecast using sliding window method is there any use case or example please share links if you have already posted
thanks
Yes, I have hundreds, perhaps start here:
https://machinelearningmastery.com/start-here/#deep_learning_time_series
Hi sorry im really got struck finding link for sliding window method , can you please provide link which is redirect to sliding window method , im working on temperature data so i need to predict next value using sliding window method , so kindly give me the link which contain predictive model (like train test split and future forecast using sliding window)
Thanks Jason
This tutorial will show you how to split the data:
https://machinelearningmastery.com/convert-time-series-supervised-learning-problem-python/
This tutorial describes how to evaluate models using walk-forward validation (cross-validation is invalid):
https://machinelearningmastery.com/backtest-machine-learning-models-time-series-forecasting/
The tutorials here make use of the above two methods using neural networks:
https://machinelearningmastery.com/start-here/#deep_learning_time_series
is there any library or package to use sliding window method in time series forecasting?
Yes, I created one:
https://machinelearningmastery.com/convert-time-series-supervised-learning-problem-python/
from pandas import read_csv
from pandas import DataFrame
from pandas import concat
series = read_csv(r’data.csv’, header=0, index_col=0)
temps = DataFrame(series.values)
dataframe = concat([temps.shift(3), temps.shift(2), temps.shift(1), temps], axis=1)
dataframe.columns = [‘t-3’, ‘t-2’, ‘t-1’, ‘t+1’]
print(dataframe.head(20))
out put:
t-3 t-2 t-1 t+1
0 NaN NaN NaN 41
1 NaN NaN 41 40
2 NaN 41 40 39
3 41 40 39 39
4 40 39 39 40
5 39 39 40 39
6 39 40 39 38
7 40 39 38 42
8 39 38 42 51
9 38 42 51 59
10 42 51 59 62
11 51 59 62 63
12 59 62 63 62
13 62 63 62 61
14 63 62 61 65
15 62 61 65 56
16 61 65 56 64
17 65 56 64 65
18 56 64 65 64
19 64 65 64 61
so this is the way to forecast using sliding window method?
if my approch is correct then t-2 t-3 are my foretasted values ?
im littele confuse kindly suggest me
thanks
jason
pls Give some information for this post
That is the way to prepare data using the sliding window method.
Hi, Jason, I have tried ur approach but got stuck in this step
Date value
1-1-19 5
2-1-19 6
3-1-19 8
4-1-19 10
5-1-19 2
6-1-19 11
This is my data I have reframed it using a sliding window
So, in this case, shall I consider the Date column or i need to remove?
if I need to consider then I need to Date column as an index ?
because I’m using regression model to predict time series data?
Kindly plz suggest how do i pass this Date to regression model?
The date column is discarded.
Ok if I discarded Date Column, then how can I predict the value on a particular date?
Basically, if I pass any date my model should predict the value.
Using that predicted value I need to fill missing values in future
Kindly Help me with detail suggestion ur my great trainer
Call predict() to make a prediction in new cases.
Here are some examples:
https://machinelearningmastery.com/make-sample-forecasts-arima-python/
Hi,
I just want to ask you question following my problem in my research.
I am not so good in the programming, but I am looking for the solution for my problem.
I have half hourly based eddy covariance 4 years measured data.
I am studying CO2 fluxes, but unfortunately we have gap 3.5 months which I cant gap fill with common based technique. So I need to use some maybe RF or SVR, or BiLSTM model to gap fill this long gap. Can you please suggest how to look and where to look for the code (better in Python) following those models?
Thanks in advance
This might give you ideas:
https://machinelearningmastery.com/faq/single-faq/how-do-i-handle-discontiguous-time-series-data
There is a dataset with fields: date, balance, sales amount, quantity (target variable). If we create train and test samples for fitting the model, then how can the predict result put into production, because in real conditions there will be nothing ut a date for the prediction, and the balance, sales amount are sent to the test sample?
You must design the model based on how you intend to use it in production. E.g. select inputs that will be available at prediction time.
Hi Jason,
Thanks for this article, it resolved my few doubts.
I have a question, I am working on a dataset in which I have many time series (Stock price and macroeconomic variables) and there is only one dependent variable. I want to predict stock price on next time step with using all time series( basically want to include effects of macroeconomic variables). Do you think it is advisable to use 12 periods lags of dependent and independent variables in my study? I am a bit worried about using the dependent variable lags as it can cause Bias and may reduce the effect of other variables. Also should I use the lags of all variables to not lose any information and later remove the unimportant ones using feature importance?
Sorry for the long query, your advice would be highly appreciated. Many thanks in advance.
You’re welcome.
I think stocks are not predictable:
https://machinelearningmastery.com/faq/single-faq/can-you-help-me-with-machine-learning-for-finance-or-the-stock-market
Nevertheless, try a range of configurations in order to discover what works best for your specific model and dataset.
Thanks for the reply, Yes I agree that Stocks are unpredictable, that’s why I am trying to check the market movement for the next month using macroeconomic variables whether it will go up or down.
Good luck with your project!
Hello Sir! can you please give an example using window size of greater than 2 or 3. Actually sir I am not able to understand this sliding thing int this sliding window concept means what is sliding here.
Than you sir
This function will do it for you:
https://machinelearningmastery.com/convert-time-series-supervised-learning-problem-python/
Thanks for reply sir!
You’re welcome.
Do you have any article around demand sensing?
I don’t think so, but maybe these tutorials will help to get you started:
https://machinelearningmastery.com/start-here/#timeseries
Jason,
Nice article as always, I understand the explained above about time series forecasting been treated as supervised learning problem. Can be treated otherwise, unsupervised learning, semi-unsupervised, reinforcement learning, etc…?
Thanks!
I don’t see why not.
Do you have any example of this? I don’t know, how the data most be handle and what cain of ANN will be the one to use in case this problem is treated as unsupervised or reinforcement learning.
Sorry I do not. I focus on supervised learning.
Do you know where I can find any?
Perhaps start with a search on scholar.google.com
Hi Jason,
Very interesting article, and thanks for the clear step by step code.
I think I am missing the problem however. Is treating the data this way redundant if we use an LSTM? I am thinking that the y(t-1) can be fed into the next cell as x(t). And any other relevant information of x and y (including from t-2, t-3, etc) is passed in the cell state (t-1) and hidden activation (t-1).
The way the data is constructed here explicitly adds x(t-2), x(t-3), etc, where previously they were implicit in the cell state and hidden activations. This makes it a bit redundant.
However, I’m new to sequence models, and I may be missing something 🙂 Would love to hear your thoughts.
David
Thanks!
No, such a transform is required to get started with an LSTM for time series. Perhaps start here:
https://machinelearningmastery.com/start-here/#deep_learning_time_series
Jason, thank you for the article. The sliding window will help me to predict many steps ahead but I’d need to to consider exogenous inputs in these models. Is there anyway this can be done?
Yes, exog vars can be prepared in an identical manner.
Hello, thank you for the article, I’ve learned so much from it. Do you have some tips what topics to read or which algorithm/methods to study, if I have timeseries datasets like this:
Time Value
1 2
2 0
3 3
…
and I have a single output variable Pass/Fail for whole dataset like above. So I need to decide for new whole datasets if they are similar to passed datasets or failed datasets. Im thinking if conversion to format:
ID 1 2 3 … Output
Dataset_1 2 0 3 Pass
Dataset_2 1 2 4 Fail
…
is ok.
Thank you for your topics and thanks for answer!
You’re welcome.
Sounds like time series classification. Yes, you’re on the right track.
Also, you can find “activity recognition” time series classification tutorials here that you can adapt for your problem:
https://machinelearningmastery.com/start-here/#deep_learning_time_series
Hello Jason,
thanks for the wonderful tutorial I have read many tutorials where for forecasting we are using regression king of problem. such as price forecasting, weather, and stock but I have a dataset of a production line where the machine is producing multiple error codes as the target variable.
i know using classification it’s a pretty easy job but my goal is to predict that for the next 10 cycles which error code could come.
could you please recommend me which tutorial should I read and also if there is any working example on this topic?
here is how my data look like
TimeStamp Cycle FCode WT_Nr DcPaSpU DcPaSpR Temp__RT
09:02:30 0 0 20 500 20000 24.86
09:02:36 1 41 34 500 20000 24.85
09:02:42 2 0 11 500 20000 24.86
09:02:48 3 0 69 500 20000 24.85
09:02:54 4 0 6 500 20000 24.84
09:02:58 5 90 7 0 0 -999.00
You’re welcome.
Perhaps start here:
https://machinelearningmastery.com/start-here/#deep_learning_time_series
Hi Jason,
I am looking into works and articles online that apply ML models to time series.
I have noticed, aside from the application of a sliding window, quite a number of works make point prediction for all time steps into the future. By that I mean they create date-time features or a simple lag as big as the forecast they want to make or time series other than target as features. That means all their features are available for the future unseen data. Then they feed it in any ML model and predict for all time steps in the future.
My question is, isn’t this also a form of multistep forecast?
Even though it is neither recursive nor direct by definition. What do we call this kind of lazy approach?
If you are predicting multiple steps into the future, it is a multi-step forecast.
Hi Jason,
Can the forecasting problems be framed as a predictive problem?
For eg: Say I have the data of power generated for a month. Can the next month’s power be forecasted or predicted?
Will it be viable to say the power for the next month is predicted not forecasted?
If I am correct prediction is all depends upon the particular data point if we talk about real-time scoring.
On the other hand, forecasting is learning from the previous data points and forecast the values without even depending upon the real-time data. This is what the forecast is.
Yes:
https://machinelearningmastery.com/time-series-forecasting-supervised-learning/
Hi Jason!
I want your opinion on this!
I used a hybrid Random forest and MLP in forecasting port terminal performance
(throughput). inputs were 9 port performance indicators (monthly data) from 2009 – 2020 and I have decent results. it was a multi-step forecast for 12 months of 2021
but the research community says I should rather use the classical methods for the problem!
how can I defend the use of machine learning models on this one?
Good question.
Collect evidence on how well classical methods do on the same data/problem and compare your alternate approach and compare directly. This would be strong support your methods are better suited or more capable on the problem.
Hi Jason
I am solving a problem where I have Daily volume file with dates, Holidays and market indicators
goal is to use the daily volume data to create a function that will predict 1 day ahead on a rolling basis.
will use a hold out set that will be used to measure model accuracy (MAE, MSE and directional accuracy).
do not use any data that would not be available on the day to predict ahead (do not use current data for current day prediction). In real life, we would not have that data.
Jason, do you think converting the given time series to a supervised format will help me do this?
I would really appreciate if you could help me out here
Yes, as long as you preserve the temporal order of observations (e.g. don’t shuffle).
Also, evaluate using walk-forward validation.
I’m solving a multivariate problem. So when I convert the training data into supervised, I lose the first and the last row. Do I need to make a similar transform to the Holdout set?
Yes.
Thank you very much for your explanations, they are very useful to me. I have a variant to these cases, and if what you wanted is to take several rows of the time sequence in the dataframe and that the output (y) was the next value of the timestamp, for example: that the first 10 rows of the time series were the input (X), and the 11th was the output (y) ,; and then from row 2 to 11 were the next input (X) and the output was row number 12 and so on.
And that for a multivariate series, these would be possible with the sliding window method or some other method.
Thank you for your attention, I am awaiting your response.
You’re welcome.
Yes, you can use the sliding window for multivariate time series. There are many examples of this on the blog, perhaps start here:
https://machinelearningmastery.com/start-here/#deep_learning_time_series
Thanks for your answer, I will review the examples, if I do not find something similar, I will comment.
You’re welcome.
Hi Jason,
Thanks for all the time you are putting into this. I have a basic question that for some reason I just can’t figure out. In a supervised learning set-up for for multi-step forecasting, where you have multiple outputs (one for each t+k step), how do you actually get out-of-sample predictions? If I want a prediction for a specific day, I may have one prediction for that day in one output column (say +5 prediction window), and another prediction for that same day in another output column (say +4 prediction window). So how do I get a single prediction out of that? What I am missing here?
Best,
You’re welcome.
You must frame the input to the model based on the data you have at prediction time, or use the model recursively.
Do we need to perform scaling or normalizing the time-series data after converting into it as supervised form or before?
Acc. to me if we perform scaling before converting into supervised form then scaler.inverse_transform gives wrong result?
Please correct me if am wrong.
Please reply. It will really helpful.
Thanks in advance.
Before, per variable. Fit on the training set only to avoid data leakage.
Sir, This topic was addressed beautifully and made concepts clear.
Can you please make some article on Temporal difference and Just-in-time technique? That would be of great help to many, I guess.
Looking forward to hear from you.
Best wishes & regards.
Thanks!
Great suggestions, thanks.
Hi Jason, always get enlightening from your article and books.
I have question, rather dumb question, forgive me.
I compare the performance of ARIMA and other windows-sliding algoritm (such as ANN) for univariate time series data.
Wonder, is it fair to compare the ARIMA with p and q, lets say 5, and compare with ANN with sliding windows 2 observation (t-2,. t-1 to forecast t+1)?
Or should I use same lag for both algorithm? so p and q should be same with the ANN parameter?
Thanks
Joko
Hmmm, generally you must choose a point of comparison and defend it.
Off the cuff, as long as both algorithms have access to the same data and are evaluated under the same conditions, then the evaluation seems reasonable, e.g. yes as long as the ARIMA config is optimal compared to other configs meaning you’re getting the most out of it.
Thanks Jason. it seem my optimal-config ARIMA always outperform my ANN for either stationary and non stationary dataset.
probably I took wrong dataset to play, since the persistence/naive sometime beat ANN as well …sigh….
Modeling is tricky!
Hi Jason,
Great article and thanks so much for answering all the questions in the chat! It has been incredibly useful to read through them.
I have a question as well:
I have a time series dataset with multiple features X_n from which I want to predict an output y. However, both the x and y values are unevenly spaced and were sometimes collected at different frequencies.
For example, let’s say the temperature variable is collected 3 times a day while the oxygen saturation is only collected once a day and then the output (harvest weight) is collected once every two days. In addition sometimes the data was just not collected and so there will be a lot of values missing.
What is the best practice in modelling these kind of datasets? Should I do a very basic linear interpolation between all the data points and then supply the most granular frequency with interpolated values to the model? Or is it best practice to stick to the less granular values and lose all the information from the higher frequency data?
The intention is to create a dataset over which I will use sliding windows to feed into a variety of ML forecasting models (gaussian processes, LSTM, CNNs, symbolic regression etc)
Any help would be much appreciated!
This may give you ideas:
https://machinelearningmastery.com/faq/single-faq/how-do-i-handle-discontiguous-time-series-data
Hi Jason,
Thank you so much for your great article. It’s really helpful for me. In case I want to forecast univariate time series using KNN regression with lags = 4, could you please give me some advice with how can i restructure my data ?
My idea is to create 4 input columns for x-4, x-3, x-2, x-1 and 1 column for output y so i can calculate the distance between y to each of input value. Since i am totally new to ML?KNN, i still don’t know if my direction is correct,
Hope to hear from you soon.
Thanks a lot.
Min
If you use regression (KNN or not), what you need is to make x-1, x-2, x-3, x-4 as the predictors and x (lag 0) as the target.
Thank you very much Adrian.
Min
Thank you very much for this great tutorial.
Hello, thanks for your great articles.
I have a real world time series problem to forecast next days sales of many products .
Do you have any idea how I can deal it? I don’t know what the approach to such a problem is called to google it.
After a lot of searching and reading many articles I found two keywords: grouped and hierarchical approaches. Is that true and if yes, do you have any article about it in your website?
It’s your kindness if you give me any related keywords, hints or maybe links.
Sorry, I don’t think there is any article on these approaches. But I notice that this book covered such topics: https://otexts.com/fpp3/
Maybe you can take a look. I highly recommend this book for those who want a deeper understanding on time series forecasting.
Thank you. I’ll check it.
Hi Jason
Thank you very much for the excellent training.
I have read several your articles about input data and reshape them but I am still a little confused.
If I want to define the shape of the input data for each of the examples in this article, it will look like this:
example input_shape= (samples, time steps, features)
1 input 1 output (4, 1, 1)
3 input 1 output (4, 3, 1)
2 input 2 output (4, 2, 2)
1 input 2 output two-step (3, 2, 2)
Please correct me!
Another question is what is the relationship between the number of nodes in LSTM layer and the number of input data? Should not nodes be a factor of the number of input data?
No, your output should not be counted in the input shape. If you get 3 input features, that would be (N, M, 3) for N the number of samples and M the number of time steps you want to use in the model.
Hi Jason.
I have try to studying predicting covid-19 vulnerability using time series forecasting with XGBoost regression ensemble algorithm and also use two date & cases independent variables.I think you have a clue what I done. but I am not good for programing. could you attach sample link which used as a clear guidance for a beginner. if there is article like my study please attach the link again.
thanks for your cooperation my trainer.
If you need help on starting Python programming, the must-read is the Python’s own tutorial: https://docs.python.org/3/tutorial/index.html
sorry the tool which I have using is python.
Hi Jason,
I am new to time-series analysis, but this brief blog post was incredibly insightful. You mention at the end of the blog post that “[c]areful thought and experimentation are needed on your problem to find a window width that results in acceptable model performance.” Are there are any good rules of thumb or general principles that would be useful in finding that window for a given dataset?
Hi Josh…The following resource is a great starting point:
https://machinelearningmastery.com/introduction-to-time-series-forecasting-with-python/
Hi James,
Thanks for the great article, i have a question on multivariate time series part, when you convert it to time series you use the values of measure1 at time t as a feature, but at time t we do not know the measure1 right?, so is it supposed to be like below, since we do not know x3?
x1 x2 Y
lag1(measure1) lag1(measure2) measure1 (at time t)
Also, i have another question, i want to find independent forecasts which have same dependent features, for example i want to find 100 store sales for next 15 days, and i have daily summary sale datas for each store, to convert this series into supervied, which method do you suggest? time series or ML techniques?
Thank you
Hi Yudum…You are very welcome! I would recommend going through a more focused example such as the following:
https://machinelearningmastery.com/multivariate-time-series-forecasting-lstms-keras/
Hey James,
thanks a lot for all the awesome work.
I am struggling to use your code with a .xlsx file with already separated columns for each variable (so not like your one column .csv test data). Could you help me out how it would be possible to switch the dataset from one column csv to multi-column excel and make your code work again?
Thank you very much and all the best to you!