Once you fit a deep learning neural network model, you must evaluate its performance on a test dataset. This is critical, as the reported performance allows you to both choose between candidate models and to communicate to stakeholders about how good the model is at solving the problem. The Keras deep learning API model is […]
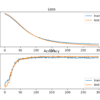