What neural network is appropriate for your predictive modeling problem?
It can be difficult for a beginner to the field of deep learning to know what type of network to use. There are so many types of networks to choose from and new methods being published and discussed every day.
To make things worse, most neural networks are flexible enough that they work (make a prediction) even when used with the wrong type of data or prediction problem.
In this post, you will discover the suggested use for the three main classes of artificial neural networks.
After reading this post, you will know:
- Which types of neural networks to focus on when working on a predictive modeling problem.
- When to use, not use, and possible try using an MLP, CNN, and RNN on a project.
- To consider the use of hybrid models and to have a clear idea of your project goals before selecting a model.
Kick-start your project with my new book Deep Learning With Python, including step-by-step tutorials and the Python source code files for all examples.
Let’s get started.
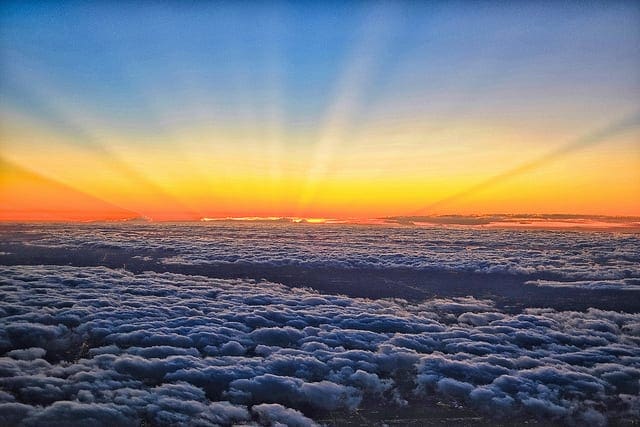
When to Use MLP, CNN, and RNN Neural Networks
Photo by PRODAVID S. FERRY III,DDS, some rights reserved.
Overview
This post is divided into five sections; they are:
- What Neural Networks to Focus on?
- When to Use Multilayer Perceptrons?
- When to Use Convolutional Neural Networks?
- When to Use Recurrent Neural Networks?
- Hybrid Network Models
What Neural Networks to Focus on?
Deep learning is the application of artificial neural networks using modern hardware.
It allows the development, training, and use of neural networks that are much larger (more layers) than was previously thought possible.
There are thousands of types of specific neural networks proposed by researchers as modifications or tweaks to existing models. Sometimes wholly new approaches.
As a practitioner, I recommend waiting until a model emerges as generally applicable. It is hard to tease out the signal of what works well generally from the noise of the vast number of publications released daily or weekly.
There are three classes of artificial neural networks that I recommend that you focus on in general. They are:
- Multilayer Perceptrons (MLPs)
- Convolutional Neural Networks (CNNs)
- Recurrent Neural Networks (RNNs)
These three classes of networks provide a lot of flexibility and have proven themselves over decades to be useful and reliable in a wide range of problems. They also have many subtypes to help specialize them to the quirks of different framings of prediction problems and different datasets.
Now that we know what networks to focus on, let’s look at when we can use each class of neural network.
When to Use Multilayer Perceptrons?
Multilayer Perceptrons, or MLPs for short, are the classical type of neural network.
They are comprised of one or more layers of neurons. Data is fed to the input layer, there may be one or more hidden layers providing levels of abstraction, and predictions are made on the output layer, also called the visible layer.
For more details on the MLP, see the post:
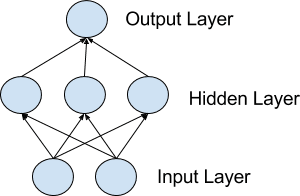
Model of a Simple Network
MLPs are suitable for classification prediction problems where inputs are assigned a class or label.
They are also suitable for regression prediction problems where a real-valued quantity is predicted given a set of inputs. Data is often provided in a tabular format, such as you would see in a CSV file or a spreadsheet.
Use MLPs For:
- Tabular datasets
- Classification prediction problems
- Regression prediction problems
They are very flexible and can be used generally to learn a mapping from inputs to outputs.
This flexibility allows them to be applied to other types of data. For example, the pixels of an image can be reduced down to one long row of data and fed into a MLP. The words of a document can also be reduced to one long row of data and fed to a MLP. Even the lag observations for a time series prediction problem can be reduced to a long row of data and fed to a MLP.
As such, if your data is in a form other than a tabular dataset, such as an image, document, or time series, I would recommend at least testing an MLP on your problem. The results can be used as a baseline point of comparison to confirm that other models that may appear better suited add value.
Try MLPs On:
- Image data
- Text Data
- Time series data
- Other types of data
When to Use Convolutional Neural Networks?
Convolutional Neural Networks, or CNNs, were designed to map image data to an output variable.
They have proven so effective that they are the go-to method for any type of prediction problem involving image data as an input.
For more details on CNNs, see the post:
The benefit of using CNNs is their ability to develop an internal representation of a two-dimensional image. This allows the model to learn position and scale in variant structures in the data, which is important when working with images.
Use CNNs For:
- Image data
- Classification prediction problems
- Regression prediction problems
More generally, CNNs work well with data that has a spatial relationship.
The CNN input is traditionally two-dimensional, a field or matrix, but can also be changed to be one-dimensional, allowing it to develop an internal representation of a one-dimensional sequence.
This allows the CNN to be used more generally on other types of data that has a spatial relationship. For example, there is an order relationship between words in a document of text. There is an ordered relationship in the time steps of a time series.
Although not specifically developed for non-image data, CNNs achieve state-of-the-art results on problems such as document classification used in sentiment analysis and related problems.
Try CNNs On:
- Text data
- Time series data
- Sequence input data
When to Use Recurrent Neural Networks?
Recurrent Neural Networks, or RNNs, were designed to work with sequence prediction problems.
Sequence prediction problems come in many forms and are best described by the types of inputs and outputs supported.
Some examples of sequence prediction problems include:
- One-to-Many: An observation as input mapped to a sequence with multiple steps as an output.
- Many-to-One: A sequence of multiple steps as input mapped to class or quantity prediction.
- Many-to-Many: A sequence of multiple steps as input mapped to a sequence with multiple steps as output.
The Many-to-Many problem is often referred to as sequence-to-sequence, or seq2seq for short.
For more details on the types of sequence prediction problems, see the post:
Recurrent neural networks were traditionally difficult to train.
The Long Short-Term Memory, or LSTM, network is perhaps the most successful RNN because it overcomes the problems of training a recurrent network and in turn has been used on a wide range of applications.
For more details on RNNs, see the post:
RNNs in general and LSTMs in particular have received the most success when working with sequences of words and paragraphs, generally called natural language processing.
This includes both sequences of text and sequences of spoken language represented as a time series. They are also used as generative models that require a sequence output, not only with text, but on applications such as generating handwriting.
Use RNNs For:
- Text data
- Speech data
- Classification prediction problems
- Regression prediction problems
- Generative models
Recurrent neural networks are not appropriate for tabular datasets as you would see in a CSV file or spreadsheet. They are also not appropriate for image data input.
Don’t Use RNNs For:
- Tabular data
- Image data
RNNs and LSTMs have been tested on time series forecasting problems, but the results have been poor, to say the least. Autoregression methods, even linear methods often perform much better. LSTMs are often outperformed by simple MLPs applied on the same data.
For more on this topic, see the post:
Nevertheless, it remains an active area.
Perhaps Try RNNs on:
- Time series data
Hybrid Network Models
A CNN or RNN model is rarely used alone.
These types of networks are used as layers in a broader model that also has one or more MLP layers. Technically, these are a hybrid type of neural network architecture.
Perhaps the most interesting work comes from the mixing of the different types of networks together into hybrid models.
For example, consider a model that uses a stack of layers with a CNN on the input, LSTM in the middle, and MLP at the output. A model like this can read a sequence of image inputs, such as a video, and generate a prediction. This is called a CNN LSTM architecture.
The network types can also be stacked in specific architectures to unlock new capabilities, such as the reusable image recognition models that use very deep CNN and MLP networks that can be added to a new LSTM model and used for captioning photos. Also, the encoder-decoder LSTM networks that can be used to have input and output sequences of differing lengths.
It is important to think clearly about what you and your stakeholders require from the project first, then seek out a network architecture (or develop one) that meets your specific project needs.
For a good framework to help you think about your data and prediction problems, see the post:
Further Reading
This section provides more resources on the topic if you are looking to go deeper.
- What Is Deep Learning?
- Crash Course On Multi-Layer Perceptron Neural Networks
- Crash Course in Convolutional Neural Networks for Machine Learning
- Crash Course in Recurrent Neural Networks for Deep Learning
- Gentle Introduction to Models for Sequence Prediction with Recurrent Neural Networks
- How to Define Your Machine Learning Problem
Summary
In this post, you discovered the suggested use for the three main classes of artificial neural networks.
Specifically, you learned:
- Which types of neural networks to focus on when working on a predictive modeling problem.
- When to use, not use, and possible try using an MLP, CNN, and RNN on a project.
- To consider the use of hybrid models and to have a clear idea of your project goals before selecting a model.
Do you have any questions?
Ask your questions in the comments below and I will do my best to answer.
Very nice article on neural networks.
I love to work on data using neural networks. The human brain is clearly the baseline for many computer programs and artificial intelligence approaches. Artificial neural networks algorithm are focused on replicating the thought and reasoning patterns of the human brain which makes it an intriguing algorithm to use.
Thanks.
Yes Jason, but I have found RFBNs to be more explainable than MLPs. Ant thoughts?
Doc vK
Fair enough. Use what works best for you.
Hello Sir,
You said that rnns and lstms work badly on time series forecasting problem. But in your book, with the use of lstms, you produced fabulous results for forecasting problem. Is no machine learning model suitable for time series forecasting and hence should we opt for statistical models like ARIMA?
I show how to use them carefully, but the results are not fabulous.
For univariate time series, linear models always beat RNNs in my tests.
Hi Dr. Brownlee,
Firstly, thanks for all your posts, they’ve been a useful reference for me since I began getting involved with ML problems about a year ago.
Right now I’m working with the problem of audio classification using conventional and neural network approaches. I’m actually using the three NNs you mention above. The idea of a hybrid model fascinates me but 1. I don’t know if it can work properly for my audio problem and 2. I don’t have any experience designing these hybrid models.
I appreciate any advice on this!
Try it and see how it goes.
What hybrid do you want to try? I have many on the blog, a good start might be here:
https://machinelearningmastery.com/keras-functional-api-deep-learning/
Thanks jason it is useful
I’m glad to hear that.
Thank you Dr. Jason.
Your tutorials have given me an inroad to ML and Data Mining.
Thank you
I’m glad to hear that.
As my first application of DL I was given 480 sequences of ssDNA and an indication if it cystalizes or not. My goal was to predict cystalization given a sequences.\
First I embedded each sequence (generally 5 – 28 in length) into a blank sequence 40 characters in length … \this allowed me to
1. make the lengths uniform and
2. repeat ssDNA sequences into different positions in the 40 charcter blank … to increase my dataset size. from 480 to 6400
Next I translated A = 10001 T = 010000 C = 00100 G = 00011 Blank = -1, -1, -1, -1, -1
After much testing and reading I created 3 models
ï‚· Model 01: Multi Layered 1D Convolutional Networks + Multilayer Perceptron
ï‚· Model 02: Time Distributed 1D Convolutional Layers + LSTM + Multilayer Perceptron
 Model 03: Stacked LSTM – 1 Perceptron
The final prediction is arrived as follows – if 2 of the 3 models predict crystalization – predict crystalization.
The accuracy will be tested in the lab over the next couple of months.
I have two questions:
Why not use the combined scores of several models.?
Do you have any suggestions or pointers on my first real project ?
Thanks again for being a guiding light for the ML sommunity
You can combine the models, this is called an ensemble prediction. I would recommend it.
I have some general suggestions for improving performance here that might spark some ideas:
https://machinelearningmastery.com/improve-deep-learning-performance/
I have a tabular data set in csv format. How can I use LSTM and CNN on it for customer churn prediction in order compare the accuracy…. I have used MLP and it gave me 97% accuracy and roc 0.87
I recommend starting here:
https://machinelearningmastery.com/start-here/#deep_learning_time_series
Thank you Dr for this explanation,
How can i develop both CNN and RNN to classify voice emotion recognition ,after using
feature extraction.
I don’t have examples of working with voice data, but perhaps you can adapt one of the models here as a starting point:
https://machinelearningmastery.com/start-here/#deep_learning_time_series
Thank you Dr.Jason
These all tutorials related to neural networks is very good and are useful to learn basics of neural network in easy way.
sir can you please give explanation on back propagation algorithm,
how the filter weights are randomly selected. is there any possibility to change that weights of filter.
Yes, see this post:
https://machinelearningmastery.com/implement-backpropagation-algorithm-scratch-python/
sir, which is the best neural network to predict a lottery number/ ( not really a random number because the some numbers are repeating many times within a month)
None. See this:
https://machinelearningmastery.com/faq/single-faq/can-i-use-machine-learning-to-predict-the-lottery
Hi Jason, I’m one of your online fans and students. I have been very constant in following your blogs, and they are pretty great. Please, I was wondering if you could help me with the idea of how to training two models that have the same network structure with the weights of one model initialized by the learned weights of the other. However, during the training of the second model, the layers of the first model are made fixed. I really need your help as it’s part of my final year project. Thank you.
You can save the weights to file, then load the weights into the new model.
Hi,
I have one use where I need to do log mining and classify logs and also predict if the classified logs can produce some undesirable behavior to the system. Given the experience with system I know what logs can produce what kind of cascading effects
Since it is a combination of classification and prediction together , I am not able to get what algorithms to be applied on this.
Could you please help .
Thanks,
Geeta
I would recommend this framework as a first step:
https://machinelearningmastery.com/how-to-define-your-machine-learning-problem/
Thank you Jason, I just started to learn ML and there are so many concepts and which confusing me is really hard to figure out when should I use the different ML algorithms to handle my problems. I’m going to try to make a prediction system for PM2.5 and PM10 in several cities, and I want to know what kind of ML algorithms probably situs for the system to make the prediction when I choose observed PM concentration and other weather info like wind speed, wind direction, temperature, and humidity. so could you give me some advice? thank you very much
I recommend following this process:
https://machinelearningmastery.com/how-to-develop-a-skilful-time-series-forecasting-model/
Hi! Jason. Appreciate your work.
Could I translate and post it into Korean on scimonitors.com with source? It’ll be grateful for us to understand the subject.
Please do not translate and repost my material.
Hello Jason,
Just a message to thank you for your site, I really appreciate all the materials as a total beginner and in my opinion that’s really well written.
Cheers from France.
Thanks, I’m happy it helped.
Hey, hope you’re doing great. Is there anyone here using neural networks as function estimator in Reinforcement learning? if yes, Some questions to which I’d like to know the answer have occupied my mind, my questions are as follows:
1) Do you use an ANN for each action to estimate the value of state S, i.e., one ANN is used to calculate Q(s,a1) , another ANN for Q(s,a2) and so forth? Or a single ANN has been exploited to calculate the Q-value of current state with respect to all actions?
2) Are there any other useful resources that may assist me to fully understand this?
thanks in advance,
Maryam
I hope to cover deep RL in the future.
hi , can i use RNN for symbol by symbol detection ?
Perhaps start with a strong definition of your problem:
https://machinelearningmastery.com/how-to-define-your-machine-learning-problem/
Then perhaps identify the sequence problem type:
https://machinelearningmastery.com/sequence-prediction/
Hi, I’m working on my ME research I need your help. Topic for my thesis is “Short-term Prediction of Exchange Currency Rate using Neural Network”, can you help me in deciding which model is best for the prediction? As many research papers I’ve read have been working on these models, so my approach is to use hybrid model i.e MLP and RNN. Do you think that the results will be more efficient using these two models? Waiting for your response.
Yes, I recommend this process:
https://machinelearningmastery.com/how-to-develop-a-skilful-time-series-forecasting-model/
Hi Jason
Thanks for the lovely explanation that you applied. I am working on time series data (binary data with time stamp) for each action represented human activities. I am working with Fuzzy Finite State Machine (FFSM) in a combination with a standard NNs to generate the fuzzy rules of the FFSM system. I have obtained good results right now. I am just asking if I want to replace the standard NNs in my system with either RNNs or CNNs, which one you suggest and based on your experience will work much better than the standard NNs.
Is it recommended to use RNN or CNN for the purpose of learning the system and generating the fuzzy rules?
Thanks in advance for your advice.
Gad
In this case, I recommend testing a suite of methods in order to discover what works best for your specific dataset.
Hi Jason, very nice article.. Do you know of any good references to Geo Spatial based ML problems or papers etc? Thanks!
Yes, I read some intersting work on CNN-LSTMs and ConvLSTMs for these types of problems.
Perhaps search on scholar.google.com
Hi Jason, very nice article!
I am new to ML, I try to build a chatbot and found many examples. What model should be used for chatbot currently? RNN, LSTM, IndRNN, CNN… or combine them?
Thanks
Sorry, I don’t have experience with chatbots.
Hi
First of all thank you so much for this useful post.
Actually I wanna solve a problem of unrolling loop factor. the input of my ANN is loop’s characteristics and the output is the predicted unrolling factor. We use our ANN as continuous function (regression pb). After looking how to present our features as input that are variable input length we come across to many type of ANN: RNN, recursive NN.
After reading more about RNN I do not see the sequential concept in our problem, we will give all features at once and predict the output. The only problem is that the number of inputs is diff from a loop to the other, I mean we may have 50 inputs for the first ex as we may have 100 inputs for the second programs and so forth.
I’m very confused what to use in this case. Please is their any suggestions?
And i have a question what is the diff between MLP and DNN, I’m confused :!.
Thank you so much.
Perhaps you can zero-pad the variable length inputs and use a masking layer to ignore them?
Thank you so much to answer me :).
But i was thinking zero-padding may affect negatively the accuracy if we have the diff between the min number of input and max number of inputs bigger more then 20 inputs set to “0” for ex it will affect the learning wont it ?
If you use a masking layer, the padded values are ignored.
Thank you so much I’m reading about it and look like a good trick. thank you so much again :).
@Jason Brownlee i did like your suggestion about using Zero_padding and masking layer to ignore them. Now once it comes to practice I’m confused.
like for ex if we have this input : (the max variable length is 10 for ex)
and we have something like this :
x= [4, 0, 0, 512, 1.0, 0.0, 1.0, 0.0, 128.0 , NaN]
with padding it will be like this :
x_pad= [4, 0, 0, 512, 1.0, 0.0, 1.0, 0.0, 128.0 , 0.0] (last 0 is padded value).
the mask should be :
x_mask= [1, 1, 1, 1, 1, 1, 1, 1, 1, 0]
then after that how should i use them should I multiplu x_pad with x_mask or what ? I still very confused.
No, the Masking layer is a type of layer in the neural network. I show how to use it here:
https://machinelearningmastery.com/handle-missing-timesteps-sequence-prediction-problems-python/
@Jason Brownlee
Yeah we can add masking layer but this is not available only in case of RNNs?
I received an answer here : https://stackoverflow.com/questions/55270074/tensor-flow-how-to-use-padding-and-masking-layer-in-case-of-mlps. saying that:
The only dimensions you can “ignore” are time dimensions in recurrent layers since the number of weights does not scale with the dimension of time and so a single layer can take different sizes in the time dimension.
If you are only using Dense layers you cannot skip anything because your only dimension (besides the batch dimensions) scales directly with the number of weights.
what do you think ?
if it’s still possible what is the equivalent code in TensoFlow?
You can ignore all time steps for a given feature if you choose.
It is supported in Keras with LSTMs, but not Dense layers.
Alternately, you can specify a value to use, e.g. -1 and not use a masking layer and see how it impacts the model performance.
Yes this is true. Mask layer can not be used in case of dense layers. I will try this alternative.
thank you :).
can i use RNNs or LSTMs for sentiment analysis and text summarization of email dataset
I believe so.
do you have a mathemmatical explination for MLP and CNN
No sorry, I don’t focus on teaching the math. Perhaps get a good textbook like this one:
https://amzn.to/2Y67S6n
I’ve seen in some papers that RNN are good for time series data. I am considering to use this kind of Neural Network in my undergraduate paper in which I am doing flood forecasting. However your post makes me confuse right now, since you don’t mention times series in the RNN use as well you say that it is bad to use tabular data. The data that I have is tabular.
Generally, I have found RNNs to be terrible at time series forecasting.
I’ve had better success with CNNs and hybrid models.
Nevertheless, you can get started with LSTMs, as well as CNNs and MLPs for time series forecasting here:
https://machinelearningmastery.com/start-here/#deep_learning_time_series
i think you have not experimented well enough. Please have a look on this link, i was shocked to read that RNN does not perform well on time series data.(on your blog)
https://www.tensorflow.org/tutorials/structured_data/time_series#recurrent_neural_network
See this:
https://machinelearningmastery.com/suitability-long-short-term-memory-networks-time-series-forecasting/
Dr. Brownlee,
Your posts are great – succinct and yet great content.
I am thinking of an ML problem and asking for your advice. If a classification problem into multiple outputs has both spatial and temporal dimensions (e.g. from video clip) would CNN – LSTM – Multi-layer Perception hybrid model be the right approach? From your post, LSTM seems to be for predicting next time step instead of classification ( I may have misunderstood). So I am wondering if LSTM / RNN is required or not.
Appreciate your guidance and pointers.
For video data, a CNN-LSTM would be a good starting point:
https://machinelearningmastery.com/cnn-long-short-term-memory-networks/
Hi Jason,
Thank you very much for this useful article. My question is why CNNs are recently being adopted for graphical data (CNNs are being used to learn node representations in a graph), although, based on my understanding, spatial relationships, where order matters, don’t exist? Thanks!
CNNs were developed for image data, and they are very effective, that is why you are seeing their wide use with image data.
Hi Jason,
Article is really great to read and learn. I want to ask someone to get idea. I am trying to predict election results by using data of economical, social welfare and developmental data of 120 countries with 1400 election results from 2000 to 2016. But I am not sure which type of neural network to use and which programming language or package. My pre decision is to use MLP with the technology of pytorch. Do you have any advice?
MLP sounds like a good start.
I’d also encourage you to try xgboost:
https://machinelearningmastery.com/start-here/#xgboost
Thanks Jason for the insight on the various NN algorithms to use for various Machine learning problems. My dilemma is am trying out an idea of predicting pneumonia among infants using various data sets including hmis data,climate data, population data, pharmacy data, to come up up with a predictive model which can give a good accuracy, my question is am grappling with the best machine learning approach i would consider or better still which NN algorithm would be suitable for this problem? Any appropriate suggestion would be appreciated.
This is a common question that I answer here:
https://machinelearningmastery.com/faq/single-faq/what-algorithm-config-should-i-use
Dear Jason,
I am just dropping by to express my sincerest gratitude for your contribution to novices like me. I had to do a short time-series forecasting exercise and I had almost no clue of where to start. Over two days, I have gotten a better understanding and was able to conjure something worthy of a discussion with the help of your website. I really can’t thank you enough for this.
Many thanks,
Niru
Thanks, well done on your project!
Dear Jason,
Your article was very good .
I wanted to ask , can the input of RNNs be the data of the dynamic response of a structure ?!
Or is it better to ask what can be the input of the RNNs in the structures ?!
I want to work on RNNs as my thesis.
Thank you .
There are many ways to frame a sequence prediction problem, perhaps this will help:
https://machinelearningmastery.com/sequence-prediction/
Dear Jason,
Kindly inbox me immediately let’s have a discussion on some cogent matters ASAP.
I need your help.
Okechukwudavid@gmail.com
You can contact me any time via this page:
https://machinelearningmastery.com/contact/
Hi Jason,
First of all, many thanks for your great tutorials for ML practitioners. I am also a big fan of your method where you reference relevant papers/books for further read.
I agree and find it useful that you also discourage the use of RNN for tabular data/forecasting problems. I also understand that many observations are empirical and come with your experience. But, would you happen to know any literature that I can read to get more details on the problem. Thanks again.
What problem exactly?
Applying RNNs to tabular data? There are no references because it is inappropriate.
Really helpful article. Thanks a lot!
You’re very welcome.
Hi Jason,
thanks for the help! I have a question about which NN I should use for my research.
I have two signal (ECG and PPG) and I want to predict a third signal (ABP) with the help of ECG and PPG. I have long series of signals, but i want to use ECG and PPG for the prediction of ABP value. I have ABP signal but for me is important the only value of ABP maximum (systolic Pressure) and ABP Minimum (dyastolic pressure), as output.
What do you think about usable architecture?
I recommend this framework:
https://machinelearningmastery.com/how-to-develop-a-skilful-time-series-forecasting-model/
Can you explain the advantage of Conv1D+MLP architecture over MLP architecture with respect to time series forecasting problem
I could tell a story about how the methods work, but all that matters is: If it gives a better result, use it.
But if the reviewer asks why have you used Conv1D+MLP rather than using any other hybrid then what should be my explanation.
Test other methods and confirm that your chosen approach achieves the best result.
Work must be defendable.
apart from this any mathematical explanation????
Yes, perhaps check this textbook:
https://amzn.to/2C0tBml
Can you please explain the reason for the following:
I am doing a regression problem that is trying to predict multistep rainfall with some set of input variables on a daily scale.
Please explain the reason for the following:
What ever model (viz. MLP, Conv 1D, LSTM, GRU and hybrid model also) I am trying with my dataset loss function (MSE and other loss functions also tried) of training and testing starts decreasing at initial epochs but after epochs say 8or 10 testing loss does not decrease where as training loss keeps on decreasing. Why??
Perhaps all models are learning the same underlying function?
Sorry. I didn’t got your point.
Perhaps all models are achieving the same result because they are all learning the same underlying mapping function from inputs to outputs – e.g. they are achieving the best that is possible on your dataset. It’s just an idea.
dear Jason,
I am working on Sarcasm Detection ,one of the major challenge in sentiment analysis.
iam searching for methodology in deep learning that can be best for sarcasm detection in any data set.
is RNN best for it….or a hybrid model……???
please suggest me any link also for better understanding of sarcasm detection using deep learning with RNN.
thanks.
Yes, you can see examples of sentiment analysis with CNNs here under “text classification”:
https://machinelearningmastery.com/start-here/#nlp
Hello Jason,
Thank you for this article. It cleared most of my doubts about Neural Networks. But still I need your one suggestion.
I have a dataset of League football match statistics of last few seasons in the form of CSV. To predict the football match results, which is the best model, MLP or RNN ?
Perhaps test a suite of methods and discover what works best?
Okay… Thank you Jason.
But according to your experience, which model will be suitable for this problem?
It is unknowable. See this:
https://machinelearningmastery.com/faq/single-faq/what-algorithm-config-should-i-use
I just want to thank you Dr. Brownlee, about all the great and exceptional efforts you are offering here.
Thanks, I appreciate your support!
Hi Jason,
As you explained RNN and CNN are used with data that have special relationship( time or space) but they are commonly used in intrusion detection where the data is tabular (the input is a csv file that contains many record with 41 features and one label(one of the five class that we look for)). It’s just a classification problem. But i can’t find the justfication of their use. I even found combination CNN-LSTM
Sounds like a classification problem, e.g. not appropriate for RNN or CNN.
Hi Jason, can you explain how data can be fed into a LSTM net? For example, I have a time a 1D time series of 3 elements [1, 2, 3] and a LSTM net with 2 cells. Thank you very much in advance.
Yes, see this:
https://machinelearningmastery.com/faq/single-faq/what-is-the-difference-between-samples-timesteps-and-features-for-lstm-input
Dear Dr. Brownlee,
Thank you for your post. It is really helpful.
I would like to have your valuable suggestion.
I am working on speech processing problem for classification task. If i extract features from speech/voice .wav file and want to apply on a deep neural network, Which model do you recommend for that? Both for single and multi class problems.
Thank you in advance.
Sorry, I don’t have tutorials on deep learning for audio, but I hope to cover it in the future.
Thank you for your concern Sir.
Hello Jason,
Thanks for your articles, they helped me a lot to step into the field of ML but still my experience is limited to take into considerartion all the factors in order to choose the best suitable framework for implementation in my project. My problem is dealing with the identification of input parameters for a pendulum (initial speed, angle) based on the system behaviour (displacement in X, Y directions). I have a dataset that has 1000 of cases for training a NN, each case has 5000 dispalcement measurements for period of 5 sec, based on these dispalcements I’m trying to predict which input parameters were used. What model would you suggest for this problem? Thanks in advance!
You’re welcome.
This might help choose inputs:
https://machinelearningmastery.com/how-to-define-your-machine-learning-problem/
This will help choose a model:
https://machinelearningmastery.com/how-to-develop-a-skilful-time-series-forecasting-model/
Thanks for your articles, they helped me very much.
My dataset is sequences of network packets I observated. Each observated event has many variables such as IP, port…etc. I try that dataset with LSTM that I follow your articles, it is ok. Now, I want to implement a model on a hardware that support a framework (only CNN is support), so I want to try that dataset with CNN. How can I shape my data for fedding to CNN (many examples only use images for input). Can I shape data like LSTM’s input (sample_num, features, timestep) – every subsequence will not overlap.
Thanks in advance!
This will help you shape your data:
https://machinelearningmastery.com/faq/single-faq/what-is-the-difference-between-samples-timesteps-and-features-for-lstm-input
Hello Mr Jason, I am currently working on predicting soil properties from a hyperspectral image. However, a research has been done using CNN. I am thinking of hybridization of CNN and another deep learning model. Do you think the LSTM architecture mentioned above can improve the accuracy of the CNN . I am a newbie to research in AI
I recommend testing a suite of algorithms on your problem and discover what works best.
Hi Jason,
I am working on DNA sequencing problem, in which I have a large sequence of letters A,G,C,T ad I have to predict whether it belongs to class 0,1,2,3.
ex. AGGGGGCTTTAACTGGG can either belong to class 0,1,2 or 3
like this, I have 44k training examples.
Here there is one more catch – A-T and G-C bond together. Also, the structure (spatial orientation)of the molecule plays an important role (therefore I was thinking of graph NN also)
Can you please suggest which architecture will work best
Thank You
Omkar
I recommend modeling it as a sequence prediction problem.
Try an MLP, CNN and LSTM, this will help:
https://machinelearningmastery.com/start-here/#deep_learning_time_series
Thank you so much. will try that.
You’re welcome.
Hi, Jason,
Thank you for your tutorial.
I have a question about NN structures.
If we want to make a sequence prediction (SeqToSeq) we have to use a Recurrent Model this is obvious. But what if we want to create a model for ManyToOne problem?
For example, we know household power consumption for every single customer (Multivariate TimeSequence for every User) and we want to predict each user’s consumption for the next day (individually).
In this scenario, we could use CNN (1D CNN, multi-headed 1D CNN, Dilated CNN, etc.) and change the last Dense layer activation function to linear?
Like this: https://machinelearningmastery.com/cnn-models-for-human-activity-recognition-time-series-classification/
Or we have to use the RNN or TimeDistributed model?
Yes, many different models could be used. It would be a good idea to test a few and see what works well or makes the most sense.
Yes for CNN or Yes for RNN? 🙂
In my head, the picture is put together in such a way that CNN learns the shape of the time series, and that’s why CNN good at the time-series classification problem. Do you think it can also be good for a regression problem? Like forecast stock piece, etc….. Like ManyToOne SeqToSeq problem?
I’m suggesting that either might be appropriate, but we cannot know for a specific dataset until we run experiemnts.
Stock market is not predictable:
https://machinelearningmastery.com/faq/single-faq/can-you-help-me-with-machine-learning-for-finance-or-the-stock-market
Hi Jason
I have a basic question, what is the difference between MLP and deep neural networks?
for example, when I write an article, when I can claim I apply deep neural network on the data?
thanks ahead 🙂
Deep learning refers to any neural net really, or more specifically a neural net with many layers.
Sure.
Hello,
First of all, thank you for your great articles! I’m new to deep learning, and I would like to ask a probably too trivial question for you:
I want to use the deep learning for binary classification of a tabular dataset, according to this article, the MLP should work better than CNN or LSTM. Can you please explain the reasoning in detail? Or can you please refer me to another article to help me understand the reasoning?
I’m looking forward to learning (pun intended) and thank you in advance.
You’re welcome.
1dCNN and LSTM are inappropriate because they are for sequence prediction. A tabular dataset in a CSV is not a sequence prediction problem.
Is that reasoning clear?
Thank you for the response, but there are still unclear things for me (sorry):
“Inappropriate” doesn’t mean both 1D-CNN or LSTM can’t work on a tabular dataset, right? Because when I tried to run them (MLP, 1D-CNN, LSTM, and CNN-LSTM) on my CSV dataset, their accuracy is high and there is not much difference between them. Can you please tell me why? Does their performance depend on the dataset as well? To put more context, my CSV dataset consists of labeled data with “0” and “1” being “benign” and “malicious”, respectively.
Thanks again.
It can be forced but would be deeply wrong.
They are designed for sequence data, a tabular dataset is not sequence data.
If the idea of sequence problems is new for you, perhaps start here:
https://machinelearningmastery.com/start-here/#lstm
Hi Jason,
Thanks a lot for the article. I am working on a music recommendation system n planning to use a hybrid model. can I use content-based filtering using CNN and collaborative filtering using RNN then merge them as a hybrid model or will it be a disaster?
Thanks again.
Perhaps try it and compare results to other methods.
Big fan here. How do I implement this hybrid model for binary image classification?
There are an infinite number of ways, perhaps experiment until you find something that works well for your specific dataset.
Hi Jason,
Great article, thank you for detailed explanation on the process.
i am looking to build a multi- output regression model for 4 target variables using tabular data as input. What kind of neural network model do you recommend for such a problem?
Additionally, apart from NNs, what other models do you recommend for such as scenario? i wanted to use XGB but according to my understanding it doesn’t support multi target regression models.
Thanks again!
what if i want to find what is common between two images, which is the appropriate method to be used?
Hi Skuoon…the following resource may add clarity:
https://arxiv.org/pdf/1709.08761