Keras is a simple and powerful Python library for deep learning.
Since deep learning models can take hours, days, and even weeks to train, it is important to know how to save and load them from a disk.
In this post, you will discover how to save your Keras models to files and load them up again to make predictions.
After reading this tutorial, you will know:
- How to save model weights and model architecture in separate files
- How to save model architecture in both YAML and JSON format
- How to save model weights and architecture into a single file for later use
Kick-start your project with my new book Deep Learning With Python, including step-by-step tutorials and the Python source code files for all examples.
Let’s get started.
- Update Mar 2017: Added instructions to install h5py first
- Update Mar/2017: Updated examples for changes to the Keras API
- Update Mar/2018: Added alternate link to download the dataset
- Update May/2019: Added section on saving and loading the model to a single file
- Update Sep/2019: Added note about using PyYAML version 5
- Update Jun/2022: Added note about deprecated YAML format and added section about protocol buffer
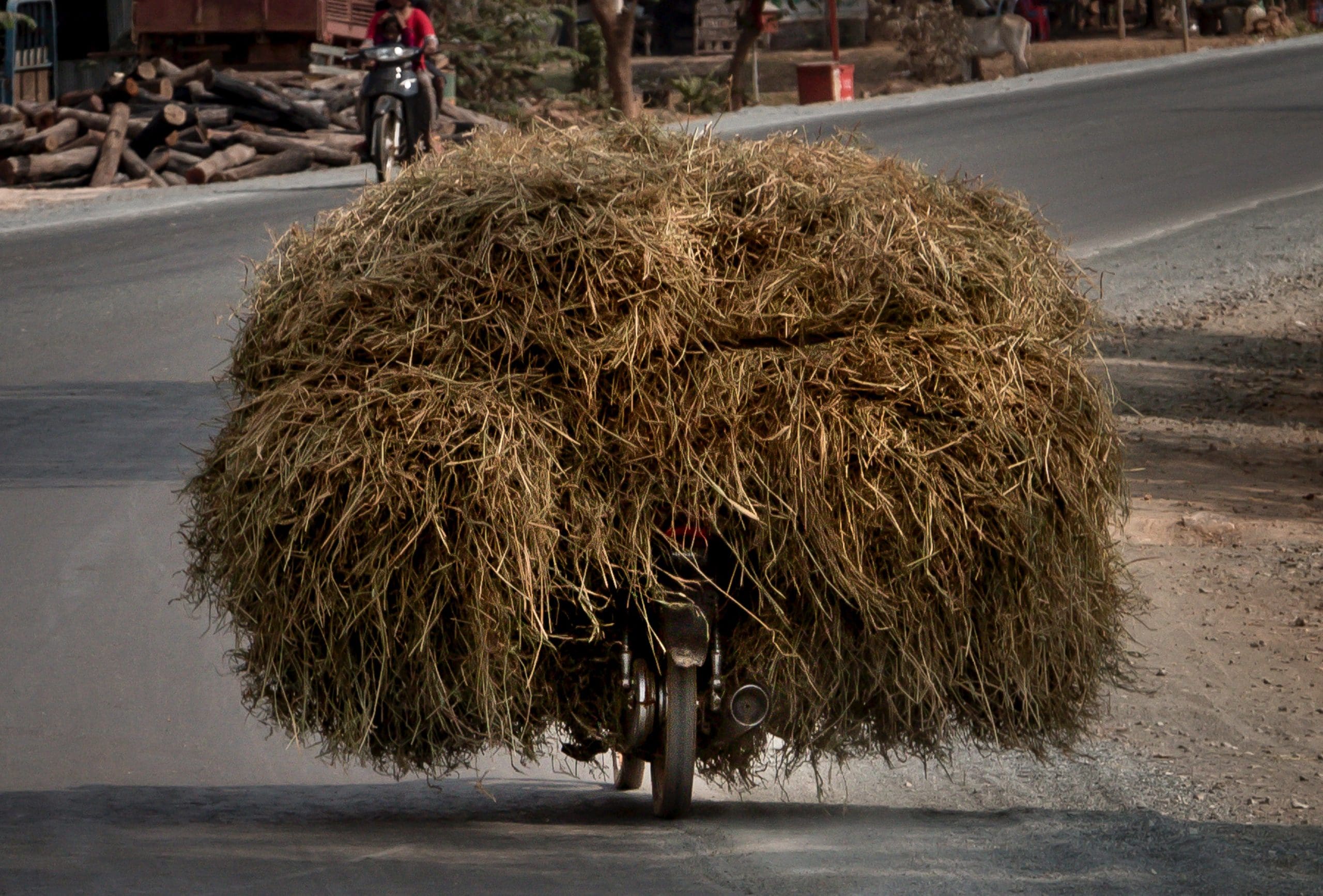
How to save and load your Keras deep learning models
Photo by Ray Harrington, some rights reserved.
Tutorial Overview
If you are new to Keras or deep learning, see this step-by-step Keras tutorial.
Keras separates the concerns of saving your model architecture and saving your model weights.
Model weights are saved to an HDF5 format. This grid format is ideal for storing multi-dimensional arrays of numbers.
The model structure can be described and saved using two different formats: JSON and YAML.
In this post, you will look at three examples of saving and loading your model to a file:
- Save Model to JSON
- Save Model to YAML
- Save Model to HDF5
The first two examples save the model architecture and weights separately. The model weights are saved into an HDF5 format file in all cases.
The examples will use the same simple network trained on the Pima Indians onset of diabetes binary classification dataset. This is a small dataset that contains all numerical data and is easy to work with. You can download this dataset and place it in your working directory with the filename “pima-indians-diabetes.csv” (update: download from here).
Confirm that you have TensorFlow v2.x installed (e.g., v2.9 as of June 2022).
Note: Saving models requires that you have the h5py library installed. It is usually installed as a dependency with TensorFlow. You can also install it easily as follows:
1 |
sudo pip install h5py |
Need help with Deep Learning in Python?
Take my free 2-week email course and discover MLPs, CNNs and LSTMs (with code).
Click to sign-up now and also get a free PDF Ebook version of the course.
Save Your Neural Network Model to JSON
JSON is a simple file format for describing data hierarchically.
Keras provides the ability to describe any model using JSON format with a to_json() function. This can be saved to a file and later loaded via the model_from_json() function that will create a new model from the JSON specification.
The weights are saved directly from the model using the save_weights() function and later loaded using the symmetrical load_weights() function.
The example below trains and evaluates a simple model on the Pima Indians dataset. The model is then converted to JSON format and written to model.json in the local directory. The network weights are written to model.h5 in the local directory.
The model and weight data is loaded from the saved files, and a new model is created. It is important to compile the loaded model before it is used. This is so that predictions made using the model can use the appropriate efficient computation from the Keras backend.
The model is evaluated in the same way, printing the same evaluation score.
1 2 3 4 5 6 7 8 9 10 11 12 13 14 15 16 17 18 19 20 21 22 23 24 25 26 27 28 29 30 31 32 33 34 35 36 37 38 39 40 41 42 43 44 45 46 47 48 |
# MLP for Pima Indians Dataset Serialize to JSON and HDF5 from tensorflow.keras.models import Sequential, model_from_json from tensorflow.keras.layers import Dense import numpy import os # fix random seed for reproducibility numpy.random.seed(7) # load pima indians dataset dataset = numpy.loadtxt("pima-indians-diabetes.csv", delimiter=",") # split into input (X) and output (Y) variables X = dataset[:,0:8] Y = dataset[:,8] # create model model = Sequential() model.add(Dense(12, input_dim=8, activation='relu')) model.add(Dense(8, activation='relu')) model.add(Dense(1, activation='sigmoid')) # Compile model model.compile(loss='binary_crossentropy', optimizer='adam', metrics=['accuracy']) # Fit the model model.fit(X, Y, epochs=150, batch_size=10, verbose=0) # evaluate the model scores = model.evaluate(X, Y, verbose=0) print("%s: %.2f%%" % (model.metrics_names[1], scores[1]*100)) # serialize model to JSON model_json = model.to_json() with open("model.json", "w") as json_file: json_file.write(model_json) # serialize weights to HDF5 model.save_weights("model.h5") print("Saved model to disk") # later... # load json and create model json_file = open('model.json', 'r') loaded_model_json = json_file.read() json_file.close() loaded_model = model_from_json(loaded_model_json) # load weights into new model loaded_model.load_weights("model.h5") print("Loaded model from disk") # evaluate loaded model on test data loaded_model.compile(loss='binary_crossentropy', optimizer='rmsprop', metrics=['accuracy']) score = loaded_model.evaluate(X, Y, verbose=0) print("%s: %.2f%%" % (loaded_model.metrics_names[1], score[1]*100)) |
Note: Your results may vary given the stochastic nature of the algorithm or evaluation procedure, or differences in numerical precision. Consider running the example a few times and compare the average outcome.
Running this example provides the output below.
1 2 3 4 |
acc: 78.78% Saved model to disk Loaded model from disk acc: 78.78% |
The JSON format of the model looks like the following:
1 2 3 4 5 6 7 8 9 10 11 12 13 14 15 16 17 18 19 20 21 22 23 24 25 26 27 28 29 30 31 32 33 34 35 36 37 38 39 40 41 42 43 44 45 46 47 48 49 50 51 52 53 54 55 56 57 58 59 60 61 62 63 64 65 66 67 68 69 70 71 72 73 74 75 76 77 78 79 80 81 82 83 84 85 86 87 88 89 90 91 92 93 94 95 96 97 98 99 100 101 102 103 104 105 106 107 |
{ "class_name":"Sequential", "config":{ "name":"sequential_1", "layers":[ { "class_name":"Dense", "config":{ "name":"dense_1", "trainable":true, "batch_input_shape":[ null, 8 ], "dtype":"float32", "units":12, "activation":"relu", "use_bias":true, "kernel_initializer":{ "class_name":"VarianceScaling", "config":{ "scale":1.0, "mode":"fan_avg", "distribution":"uniform", "seed":null } }, "bias_initializer":{ "class_name":"Zeros", "config":{ } }, "kernel_regularizer":null, "bias_regularizer":null, "activity_regularizer":null, "kernel_constraint":null, "bias_constraint":null } }, { "class_name":"Dense", "config":{ "name":"dense_2", "trainable":true, "dtype":"float32", "units":8, "activation":"relu", "use_bias":true, "kernel_initializer":{ "class_name":"VarianceScaling", "config":{ "scale":1.0, "mode":"fan_avg", "distribution":"uniform", "seed":null } }, "bias_initializer":{ "class_name":"Zeros", "config":{ } }, "kernel_regularizer":null, "bias_regularizer":null, "activity_regularizer":null, "kernel_constraint":null, "bias_constraint":null } }, { "class_name":"Dense", "config":{ "name":"dense_3", "trainable":true, "dtype":"float32", "units":1, "activation":"sigmoid", "use_bias":true, "kernel_initializer":{ "class_name":"VarianceScaling", "config":{ "scale":1.0, "mode":"fan_avg", "distribution":"uniform", "seed":null } }, "bias_initializer":{ "class_name":"Zeros", "config":{ } }, "kernel_regularizer":null, "bias_regularizer":null, "activity_regularizer":null, "kernel_constraint":null, "bias_constraint":null } } ] }, "keras_version":"2.2.5", "backend":"tensorflow" } |
Save Your Neural Network Model to YAML
Note: This method only applies to TensorFlow 2.5 or earlier. If you run it in later versions of TensorFlow, you will see a RuntimeError with the message “Method model.to_yaml()
has been removed due to security risk of arbitrary code execution. Please use model.to_json()
instead.”
This example is much the same as the above JSON example, except the YAML format is used for the model specification.
Note, this example assumes that you have PyYAML 5 installed:
1 |
sudo pip install PyYAML |
In this example, the model is described using YAML, saved to file model.yaml, and later loaded into a new model via the model_from_yaml() function.
Weights are handled the same way as above in the HDF5 format as model.h5.
1 2 3 4 5 6 7 8 9 10 11 12 13 14 15 16 17 18 19 20 21 22 23 24 25 26 27 28 29 30 31 32 33 34 35 36 37 38 39 40 41 42 43 44 45 46 47 48 49 |
# MLP for Pima Indians Dataset serialize to YAML and HDF5 from tensorflow.keras.models import Sequential, model_from_yaml from tensorflow.keras.layers import Dense import numpy import os # fix random seed for reproducibility seed = 7 numpy.random.seed(seed) # load pima indians dataset dataset = numpy.loadtxt("pima-indians-diabetes.csv", delimiter=",") # split into input (X) and output (Y) variables X = dataset[:,0:8] Y = dataset[:,8] # create model model = Sequential() model.add(Dense(12, input_dim=8, activation='relu')) model.add(Dense(8, activation='relu')) model.add(Dense(1, activation='sigmoid')) # Compile model model.compile(loss='binary_crossentropy', optimizer='adam', metrics=['accuracy']) # Fit the model model.fit(X, Y, epochs=150, batch_size=10, verbose=0) # evaluate the model scores = model.evaluate(X, Y, verbose=0) print("%s: %.2f%%" % (model.metrics_names[1], scores[1]*100)) # serialize model to YAML model_yaml = model.to_yaml() with open("model.yaml", "w") as yaml_file: yaml_file.write(model_yaml) # serialize weights to HDF5 model.save_weights("model.h5") print("Saved model to disk") # later... # load YAML and create model yaml_file = open('model.yaml', 'r') loaded_model_yaml = yaml_file.read() yaml_file.close() loaded_model = model_from_yaml(loaded_model_yaml) # load weights into new model loaded_model.load_weights("model.h5") print("Loaded model from disk") # evaluate loaded model on test data loaded_model.compile(loss='binary_crossentropy', optimizer='rmsprop', metrics=['accuracy']) score = loaded_model.evaluate(X, Y, verbose=0) print("%s: %.2f%%" % (loaded_model.metrics_names[1], score[1]*100)) |
Note: Your results may vary given the stochastic nature of the algorithm or evaluation procedure, or differences in numerical precision. Consider running the example a few times and compare the average outcome.
Running the example displays the following output.
1 2 3 4 |
acc: 78.78% Saved model to disk Loaded model from disk acc: 78.78% |
The model described in YAML format looks like the following:
1 2 3 4 5 6 7 8 9 10 11 12 13 14 15 16 17 18 19 20 21 22 23 24 25 26 27 28 29 30 31 32 33 34 35 36 37 38 39 40 41 42 43 44 45 46 47 48 49 50 51 52 53 54 55 56 57 58 59 60 61 62 63 64 65 66 67 68 69 70 71 72 73 74 75 76 77 78 |
backend: tensorflow class_name: Sequential config: layers: - class_name: Dense config: activation: relu activity_regularizer: null batch_input_shape: !!python/tuple - null - 8 bias_constraint: null bias_initializer: class_name: Zeros config: {} bias_regularizer: null dtype: float32 kernel_constraint: null kernel_initializer: class_name: VarianceScaling config: distribution: uniform mode: fan_avg scale: 1.0 seed: null kernel_regularizer: null name: dense_1 trainable: true units: 12 use_bias: true - class_name: Dense config: activation: relu activity_regularizer: null bias_constraint: null bias_initializer: class_name: Zeros config: {} bias_regularizer: null dtype: float32 kernel_constraint: null kernel_initializer: class_name: VarianceScaling config: distribution: uniform mode: fan_avg scale: 1.0 seed: null kernel_regularizer: null name: dense_2 trainable: true units: 8 use_bias: true - class_name: Dense config: activation: sigmoid activity_regularizer: null bias_constraint: null bias_initializer: class_name: Zeros config: {} bias_regularizer: null dtype: float32 kernel_constraint: null kernel_initializer: class_name: VarianceScaling config: distribution: uniform mode: fan_avg scale: 1.0 seed: null kernel_regularizer: null name: dense_3 trainable: true units: 1 use_bias: true name: sequential_1 keras_version: 2.2.5 |
Save Model Weights and Architecture Together
Keras also supports a simpler interface to save both the model weights and model architecture together into a single H5 file.
Saving the model in this way includes everything you need to know about the model, including:
- Model weights
- Model architecture
- Model compilation details (loss and metrics)
- Model optimizer state
This means that you can load and use the model directly without having to re-compile it as you had to in the examples above.
Note: This is the preferred way for saving and loading your Keras model.
How to Save a Keras Model
You can save your model by calling the save() function on the model and specifying the filename.
The example below demonstrates this by first fitting a model, evaluating it, and saving it to the file model.h5.
1 2 3 4 5 6 7 8 9 10 11 12 13 14 15 16 17 18 19 20 21 22 23 24 |
# MLP for Pima Indians Dataset saved to single file from numpy import loadtxt from tensorflow.keras.models import Sequential from tensorflow.keras.layers import Dense # load pima indians dataset dataset = loadtxt("pima-indians-diabetes.csv", delimiter=",") # split into input (X) and output (Y) variables X = dataset[:,0:8] Y = dataset[:,8] # define model model = Sequential() model.add(Dense(12, input_dim=8, activation='relu')) model.add(Dense(8, activation='relu')) model.add(Dense(1, activation='sigmoid')) # compile model model.compile(loss='binary_crossentropy', optimizer='adam', metrics=['accuracy']) # Fit the model model.fit(X, Y, epochs=150, batch_size=10, verbose=0) # evaluate the model scores = model.evaluate(X, Y, verbose=0) print("%s: %.2f%%" % (model.metrics_names[1], scores[1]*100)) # save model and architecture to single file model.save("model.h5") print("Saved model to disk") |
Note: Your results may vary given the stochastic nature of the algorithm or evaluation procedure, or differences in numerical precision. Consider running the example a few times and compare the average outcome.
Running the example fits the model, summarizes the model’s performance on the training dataset, and saves the model to file.
1 2 |
acc: 77.73% Saved model to disk |
You can later load this model from the file and use it.
Note that in the Keras library, there is another function doing the same, as follows:
1 2 3 4 |
... # equivalent to: model.save("model.h5") from tensorflow.keras.models import save_model save_model(model, "model.h5") |
How to Load a Keras Model
Your saved model can then be loaded later by calling the load_model()
function and passing the filename. The function returns the model with the same architecture and weights.
In this case, you load the model, summarize the architecture, and evaluate it on the same dataset to confirm the weights and architecture are the same.
1 2 3 4 5 6 7 8 9 10 11 12 13 14 15 16 |
# load and evaluate a saved model from numpy import loadtxt from tensorflow.keras.models import load_model # load model model = load_model('model.h5') # summarize model. model.summary() # load dataset dataset = loadtxt("pima-indians-diabetes.csv", delimiter=",") # split into input (X) and output (Y) variables X = dataset[:,0:8] Y = dataset[:,8] # evaluate the model score = model.evaluate(X, Y, verbose=0) print("%s: %.2f%%" % (model.metrics_names[1], score[1]*100)) |
Running the example first loads the model, prints a summary of the model architecture, and then evaluates the loaded model on the same dataset.
Note: Your results may vary given the stochastic nature of the algorithm or evaluation procedure, or differences in numerical precision. Consider running the example a few times and compare the average outcome.
The model achieves the same accuracy score, which in this case is 77%.
1 2 3 4 5 6 7 8 9 10 11 12 13 14 15 |
_________________________________________________________________ Layer (type) Output Shape Param # ================================================================= dense_1 (Dense) (None, 12) 108 _________________________________________________________________ dense_2 (Dense) (None, 8) 104 _________________________________________________________________ dense_3 (Dense) (None, 1) 9 ================================================================= Total params: 221 Trainable params: 221 Non-trainable params: 0 _________________________________________________________________ acc: 77.73% |
Protocol Buffer Format
While saving and loading a Keras model using HDF5 format is the recommended way, TensorFlow supports yet another format, the protocol buffer. It is considered faster to save and load a protocol buffer format, but doing so will produce multiple files. The syntax is the same, except that you do not need to provide the .h5
extension to the filename:
1 2 3 4 5 6 7 8 9 |
# save model and architecture to single file model.save("model") # ... later # load model model = load_model('model') # print summary model.summary() |
These will create a directory “model” with the following files:
1 2 3 4 5 6 7 |
model/ |-- assets/ |-- keras_metadata.pb |-- saved_model.pb `-- variables/ |-- variables.data-00000-of-00001 `-- variables.index |
This is also the format used to save a model in TensorFlow v1.x. You may encounter this when you download a pre-trained model from TensorFlow Hub.
Further Reading
- How can I save a Keras model? in the Keras documentation
- About Keras models in the Keras documentation
Summary
In this post, you discovered how to serialize your Keras deep learning models.
You learned how to save your trained models to files, later load them up, and use them to make predictions.
You also learned that model weights are easily stored using HDF5 format and that the network structure can be saved in either JSON or YAML format.
Do you have any questions about saving your deep learning models or this post?
Ask your questions in the comments, and I will do my best to answer them.
Hi Jason,
I am grateful you for sharing knowledge through this blog. It has been very helpful for me.
Thank you for the effort.
I have one question. When I am executing keras code to load YAML / JSON data i am seeing following error.
Traceback (most recent call last):
File “simple_rnn.py”, line 158, in
loaded_model = model_from_yaml(loaded_model_yaml)
File “/usr/local/lib/python2.7/dist-packages/Keras-1.0.4-py2.7.egg/keras/models.py”, line 26, in model_from_yaml
return layer_from_config(config, custom_objects=custom_objects)
File “/usr/local/lib/python2.7/dist-packages/Keras-1.0.4-py2.7.egg/keras/utils/layer_utils.py”, line 35, in layer_from_config
return layer_class.from_config(config[‘config’])
File “/usr/local/lib/python2.7/dist-packages/Keras-1.0.4-py2.7.egg/keras/models.py”, line 781, in from_config
layer = get_or_create_layer(first_layer)
File “/usr/local/lib/python2.7/dist-packages/Keras-1.0.4-py2.7.egg/keras/models.py”, line 765, in get_or_create_layer
layer = layer_from_config(layer_data)
File “/usr/local/lib/python2.7/dist-packages/Keras-1.0.4-py2.7.egg/keras/utils/layer_utils.py”, line 35, in layer_from_config
return layer_class.from_config(config[‘config’])
File “/usr/local/lib/python2.7/dist-packages/Keras-1.0.4-py2.7.egg/keras/engine/topology.py”, line 896, in from_config
return cls(**config)
File “/usr/local/lib/python2.7/dist-packages/Keras-1.0.4-py2.7.egg/keras/layers/recurrent.py”, line 290, in __init__
self.init = initializations.get(init)
File “/usr/local/lib/python2.7/dist-packages/Keras-1.0.4-py2.7.egg/keras/initializations.py”, line 109, in get
‘initialization’, kwargs=kwargs)
File “/usr/local/lib/python2.7/dist-packages/Keras-1.0.4-py2.7.egg/keras/utils/generic_utils.py”, line 14, in get_from_module
str(identifier))
Exception: Invalid initialization:
What could be the reason ? File is getting saved properly but at the time of loading model I am facing this issue.
Can you please give me any pointers ?
Thanks,
Onkar
Sorry Onkar, the fault is not clear.
Are you able to execute the example in the tutorial OK?
Hi Jason,
Nice post with helpful steps to save and evaluate model. How do I run the saved model on NEW data without having to re-train it on new data? lets say I have linear regression y=mx+c trained on set of x, once I obtain m and c for given y and x, only thing I need to do is input NEW x and get predicted y with same m and c. I am unable to use LSTM model on these lines.
Thanks you in advance for your help and comments.
Load the model and make predictions:
model.predict(X)
Perhaps I don’t understand the problem?
your code worked fine,
I tried to add saving model to my code but the files were not actually created alyhough I got no error messages
please advice
walid
I expect the files were created. Check your current working directory / the dir where the source code files are located.
Hi Jason,
Thanks for creating this valuable content.
On my Mac (OSX10.11), the script ran fine until the last line, in which it gave a syntax error below:
>>> print “%s: %.2f%%” % (loaded_model.metrics_names[1], score[1]*100)
File “”, line 1
print “%s: %.2f%%” % (loaded_model.metrics_names[1], score[1]*100)
^
SyntaxError: invalid syntax
What could be the issue here?
Thanks,
Peter
Hi Peter, you may be on Python3, try adding brackets around the argument to the print functions.
Hi,
Your blog and books were great, and thanks much to you I finally got my project working in Keras.
I can’t seem to find how to translate a Keras model in to a standalone code that can run without Keras installed.
The best I could find was to learn TensorFlow, build an equivalent model in TF, then use TF to create standalone code.
Does Keras not have such functionality?
Thanks
Hi, my understanding is that Keras is required to use the model in prediction.
You could try to save the network weights and use them in your own code, but you are creating a lot of work for yourself.
Thanks Jason for this incredible blog.
Is saving this model and reload only possible with keras or even in other skilearn models like kmeans Etc?
When I have a few classifies used which are also onehotencoded like salary grade or country etc, Lets say u saved model but how can I apply same encoding and featurescaling on input data for which am expected to give output?
E. G. I may have trained a cancer outcome based model based on country, gender, smoking and drinking status like often, occasional, rare etc. Now when I get new record how to ensure my encoding and featurescaling is aligned with my training set and convert this to get Prediction?
Thanks in advance for your help.
You can save sklearn models:
https://machinelearningmastery.com/save-load-machine-learning-models-python-scikit-learn/
Do you know if it’s possible to load a saved sklearn model with keras? How would that work?
No, sorry.
Hello Jason,
Thanks for your great and very helpful website.
Since in here you talked about how to save a model, I wanted to know how we can save an embedding layer in the way that can be seen in a regular word embeddings file (i.e. text file or txt format). Let’s assume we either learn these word embeddings in the model from scratch or we update those pre-trained ones which are fed in the first layer of the model.
I truly appreciate your response in advance.
Regards,
Davood
I’m not sure we need to save embedding layers Davood.
I believe they are deterministic and can just be re-created.
I guess we should be able to save word embeddings at one point (not needed always though!). To visualize/map them in a (2D) space or to test algebraic word analogies on them can be some examples of this need.
I found the answer for this and I’m sharing this here:
If we train an embedding layer emb (e.g. emb = Embedding(some_parameters_here) ), we can get the resulting word-by-dimension matrix by my_embeddings = emb.get_weights(). Then, we can do normal numpy things like np.save(“my_embeddings.npy”, my_matrix) to save this matrix; or use other built-in write_to_a_file functions in Python to store each line of this matrix along with its associated word. These words and their indices are typically stored in a word_index dictionary somewhere in the code.
Very nice, thanks for sharing the specifics Davood.
You are very welcome Jason.
However I have another question here!
Let’s assume we two columns of networks in keras and these two columns are exactly the same. These two are going to merge on the top and then feed into a dense layer which is the output layer in our model. My question is, while the first layer of each column here is an embedding layer, how can we share the the weights of the similar layers in the columns? No need to say that we set our embedding layers (first layers) in a way that we only have one embedding matrix. What I mean is shared embeddings, something like this:
emb1 = Embedding(some_parameters_here)
emb2 = emb1 # instead of emb2 = Embedding(some_other_parameters_here)).
How about the other layers on top of these two embedding layers?! How to share their weights?
Thanks for your answer in advance.
Hmm, interesting Davood.
I think, and could be wrong, that embedding layers are deterministic. They do not have state, only the weights in or out have state. Create two and use them side by side. Try it and see.
I’d love to know how you go?
Hi Jason, thanks for your share, it helps me a lot. I’d like to ask a question, why the optimizer while compiling the model is adam, but uses rmsprop instead while compiling the loaded_model?
I would suggest trying many different optimizers and see what you like best / works best for your problem.
I find ADAM is fast and gives good results.
I have difficulties finding an answer to this question:
when are weights initialized in keras?
at compile time ? (probably not)
on first epoch ?
This is important when resuming learning
Interesting question.
I don’t know.
If I had to guess, I would say at the model.compile() time when the data structures are created.
It might be worth asking on the keras email list – I’d love to know the answer.
Thank you for creating such great blog.
I saved a model with mentioned code. But when I wanted to load it again, I faced following error. It seems the network architecture was not save correctly?
—————————————————————————
Exception Traceback (most recent call last)
in ()
1 # load weights into new model
—-> 2 modelN.load_weights(“model41.h5”)
3 print(“Loaded model from disk”)
C:\Anaconda2\envs\py35\lib\site-packages\keras\engine\topology.py in load_weights(self, filepath, by_name)
2518 self.load_weights_from_hdf5_group_by_name(f)
2519 else:
-> 2520 self.load_weights_from_hdf5_group(f)
2521
2522 if hasattr(f, ‘close’):
C:\Anaconda2\envs\py35\lib\site-packages\keras\engine\topology.py in load_weights_from_hdf5_group(self, f)
2570 ‘containing ‘ + str(len(layer_names)) +
2571 ‘ layers into a model with ‘ +
-> 2572 str(len(flattened_layers)) + ‘ layers.’)
2573
2574 # We batch weight value assignments in a single backend call
Exception: You are trying to load a weight file containing 4 layers into a model with 5 layers.
Hi Soheil,
It looks like the network structure that you are loading the weights into does not match the structure of the weights.
Double check that the network structure matches exactly the structure that you used when you saved the weights. You can even save this structure as a json or yaml file as well.
Hi Jason!, how can i see the structure of the saved weights in jupyter. I have the same problem of Soheil, but i have a network with 17 layers.
Thanks for read me!
Weights are an array and you can summarize their shape via the .shape property.
Hi Jason,
I have a question. Now that I have saved the model and the weights, is it possible for me to come back after a few days and train the model again with initial weights equal to the one I saved?
Great question prajnya.
You can load the saved weights and continue training/update with new data or start making predictions.
Hi Jason,
I tried to save and load a model trained for 5000 epochs and checked the performance of the model in the same session in comparison with the model performance just before saving after 5000 epochs. I any case using the above code I ended up with random results. But then I only saved the weights and instantiated the model again and loaded the weights with the argument “by_name” model.load_weights(‘model.h5’, by_name=True) then the accuracy as same as the model starting performance at 1st epoch/iteration. But in any case I am not able to replicate/reproduce!!! Request you if you could clarify this with a post, There is a post on github too but it is not yet resolved to satisfaction!!!
Sorry to hear that, I don’t have any good ideas. Perhaps post to stackoverflow?
the github post is at https://github.com/fchollet/keras/issues/4875
Hi Jason,
It is an amazing blog you have here. Thanks for the well documented works.
I have a question regarding loading the model weights. Is there a way to save the weights into a variable rather than loading and assigning the weights to a different model?
I wanted to do some operations on the weights associated with the intermediate hidden layer.
I was anticipating on using ModelCheckpoint but I am a bit lost on reading weights from the hdf5 format and saving it to a variable. Could you please help me figure it out.
Thanks
Great question, sorry I have not done this.
I expect you will be able to extract them using the Keras API, it might be worth looking at the source code on github.
Hi Jason
thanks a lot for your excellent tutorials! Very much appreciated…
Regarding the saving and loading: It seems that Keras as of now saves model and weights in HD5 rather than only the weights.
https://keras.io/getting-started/faq/#how-can-i-save-a-keras-model
This results in a much simpler snippet for import / export:
——————————————————-
from keras.models import load_model
model.save(‘my_model.h5’) # creates a HDF5 file ‘my_model.h5’
del model # deletes the existing model
# returns a compiled model
# identical to the previous one
model = load_model(‘my_model.h5’)
——————————————————-
Thanks Patrick, I’ll investigate and look at updating the post soon.
Getting this error:
NameError: name ‘model_from_json’ is not defined
Thanks in advance for any help on this.
Confirm that you have Keras 1.2.2 or higher installed.
Make sure you run “from keras.models import model_from_json” first
I have saved my weights already in a txt file. Can I use it and load weights?
You may be able, I don’t have an example off-hand, sorry.
Hi Jason,
Thankyou for this great tutorial.
I want to convert this keras model(model.h5) to tensorflow model(filename.pb) because I want to use it in android. I have used the github code that is:
=========================================
import keras
import tensorflow
from keras import backend as K
from tensorflow.contrib.session_bundle import exporter
from keras.models import model_from_config, Sequential
print(“Loading model for exporting to Protocol Buffer format…”)
model_path = “C:/Users/User/buildingrecog/model.h5”
model = keras.models.load_model(model_path)
K.set_learning_phase(0) # all new operations will be in test mode from now on
sess = K.get_session()
# serialize the model and get its weights, for quick re-building
config = model.get_config()
weights = model.get_weights()
# re-build a model where the learning phase is now hard-coded to 0
new_model = Sequential.model_from_config(config)
new_model.set_weights(weights)
export_path = “C:/Users/User/buildingrecog/khi_buildings.pb” # where to save the exported graph
export_version = 1 # version number (integer)
saver = tensorflow.train.Saver(sharded=True)
model_exporter = exporter.Exporter(saver)
signature = exporter.classification_signature(input_tensor=model.input, scores_tensor=model.output)
model_exporter.init(sess.graph.as_graph_def(), default_graph_signature=signature)
model_exporter.export(export_path, tensorflow.constant(export_version), sess)
———————————————————————————–
but has the following error…
=====================
Loading model for exporting to Protocol Buffer format…
—————————————————————————
ValueError Traceback (most recent call last)
in ()
7 print(“Loading model for exporting to Protocol Buffer format…”)
8 model_path = “C:/Users/User/buildingrecog/model.h5”
—-> 9 model = keras.models.load_model(model_path)
10
11 K.set_learning_phase(0) # all new operations will be in test mode from now on
C:\Users\User\Anaconda3\lib\site-packages\keras\models.py in load_model(filepath, custom_objects)
228 model_config = f.attrs.get(‘model_config’)
229 if model_config is None:
–> 230 raise ValueError(‘No model found in config file.’)
231 model_config = json.loads(model_config.decode(‘utf-8’))
232 model = model_from_config(model_config, custom_objects=custom_objects)
ValueError: No model found in config file.
—————————————————————-
Please help me to solve this…!!
Sorry, I don’t know how to load keras models in tensorflow off-hand.
M Amer,
I ma trying to do exactly the same thing. Please let us know if you figure it out.
Hi Jason,
I have created the keras model file (.h5) unfortunately it can’t be loaded. But I want to load it and convert it into tensor flow (.pb) model.Any Solution? Waiting for your response….
Sorry, I don’t have an example of how to load a Keras model in TensorFlow.
Hi Jason,
I am having issues with loading the model which has been saved with normalising (StandardScaler) the columns. Do you have to apply normalising (StandarScaler) when you load the models too?
Here is the snippet of code: 1) Save and 2)Load
Save:
import numpy as np
import matplotlib.pyplot as plt
import pandas as pd
# Importing the dataset
dataset = pd.read_csv(‘Churn_Modelling.csv’)
X = dataset.iloc[:, 3:13].values
y = dataset.iloc[:, 13].values
# Encoding categorical data
from sklearn.preprocessing import LabelEncoder, OneHotEncoder
labelencoder_X_1 = LabelEncoder()
X[:, 1] = labelencoder_X_1.fit_transform(X[:, 1])
labelencoder_X_2 = LabelEncoder()
X[:, 2] = labelencoder_X_2.fit_transform(X[:, 2])
onehotencoder = OneHotEncoder(categorical_features = [1])
X = onehotencoder.fit_transform(X).toarray()
X = X[:, 1:]
# Splitting the dataset into the Training set and Test set
from sklearn.model_selection import train_test_split
X_train, X_test, y_train, y_test = train_test_split(X, y, test_size = 0.2, random_state = 0)
# Feature Scaling
from sklearn.preprocessing import StandardScaler
sc = StandardScaler()
X_train = sc.fit_transform(X_train)
X_test = sc.transform(X_test)
# Importing the Keras libraries and packages
import keras
from keras.models import Sequential
from keras.layers import Dense
# Initialising the ANN
classifier = Sequential()
classifier.add(Dense(units = 6, kernel_initializer = ‘uniform’, activation = ‘relu’, input_dim = 11))
classifier.add(Dense(units = 6, kernel_initializer = ‘uniform’, activation = ‘relu’))
classifier.add(Dense(units = 1, kernel_initializer = ‘uniform’, activation = ‘sigmoid’))
classifier.compile(optimizer = ‘adam’, loss = ‘binary_crossentropy’, metrics = [‘accuracy’])
# Fitting the ANN to the Training set
classifier.fit(X_train, y_train, batch_size = 10, epochs = 1)
# Predicting the Test set results
y_pred = classifier.predict(X_test)
y_pred = (y_pred > 0.5)
# Saving your model
classifier.save(“ann_churn_model_v1.h5”)
Load:
import numpy as np
import matplotlib.pyplot as plt
import pandas as pd
# Reuse churn_model_v1.h5
import keras
from keras.models import load_model
classifier = load_model(“ann_churn_model_v1.h5”)
# Feature Scaling – Here I have a question whether to apply StandarScaler after loading the model?
from sklearn.preprocessing import StandardScaler
#sc = StandardScaler()
new_prediction = classifier.predict(sc.transform(np.array([[0.0, 0.0, 600, 1, 40, 3, 60000, 2, 1, 1, 50000]])))
new_prediction = (new_prediction > 0.5)
Thanks,
Sanj
You will also need to save your scaler.
Perhaps you can pickle it or just the coefficients (min/max for each feature) needed to scale data.
Thanks for the useful information.
Is it possible to load this model and weights to any other platform, for example Android or iOS. I believe that model and weights are language independent.
Are there any free / open source solutions for this purpose?
I don’t see why not. Sorry, I not across the android or ios platforms.
Hi Jason,
How can I create a model out of face recognition encodings to save using Saver.save() method?
What is Saver.save()?
Hey Jason, have you tried saving a model, closing the python session, then opening a new python session and then loading a model?
Using python 3.5, if I save a trained model in one session and load it in another, my accuracy drops dramatically and the predictions become random (as if the model wasn’t trained).
This is what I’m trying to do:
”’
embedding_size = 64
hidden_size = 64
input_length = 100
learning_rate = 0.1
patience = 3
num_labels = 6
batch_size= 50
epochs = 100
seq_len = 100′
model = Sequential()
model.add(Embedding(vocab_size, embedding_size, input_length=input_length))
model.add(Bidirectional(GRU(hidden_size, return_sequences=True, activation=”tanh”)))
model.add(TimeDistributed(Dense(num_labels, activation=’softmax’)))
optimizer = Adagrad(lr=learning_rate)
model.compile(loss=’categorical_crossentropy’, optimizer=optimizer, metrics=[‘categorical_accuracy’])
callbacks = [EarlyStopping(monitor=’val_loss’, patience=patience, verbose=0)]
model.fit(x_train, y_train, batch_size=batch_size, epochs = epochs, callbacks=callbacks, validation_data=[x_dev, y_dev])
model.save(“model.h5″)
”’
Evaluating the model in this point gives me accuracy of ~70.
Then I exit python, open a new python session, and try:
”’
model2 = load_mode(‘model_full.h5′)
”’
Evaluating the model in this point gives me accuracy of ~20.
Any ideas?
I have. I don’t believe it is related to the Python session.
Off the cuff, my gut tells me something is different in the saved model.
If you save and load in the same session is the result the same as prior to the save? What if you repeat the load+test process a few times?
Confirm that you are saving the Embedding as well (I think it may need to be saved).
Confirm that you are evaluating it on exactly the same data in the same order and in the same way.
Neural nets are stochastic and a deviation could affect the internal state of your RNN and result in different results, perhaps not as dramatic as you are reporting through.
Am facing somewhat a similar problem,
If I save and load in the same session, the result from loaded model is different to the model already in the session (prior to saving)
Ideally shouldn’t a trained model (in session/memory) and loaded model (post save and load) be identical ?
I searched but have not found any leads yet. Any help/leads would be appreciated. Thanks in advance
Hi Hardik…The following resource may add clarity:
https://machinelearningmastery.com/stochastic-in-machine-learning/
@James – Thanks for your quick reply.
So I understand why the two predictions, one from the session model (ANN) and the other from the loaded model (saved session model) are different.
But in my case, the results from the session model (ANN) are very bad (very very high MAE) and the results from the loaded model (saved session model) are satisfactory (Fairly good MAE). Am not able to understand, why such a huge difference ?
I seem to get an error message
RuntimeError: Unable to create attribute (Object header message is too large)
Github issues states that the error could be due to too large network, which is the case here.. but
How should i then save the weights… Keras doesn’t seem to have any alternative methods..
Sorry, I have not seen this error.
See if you can save the weights with a smaller network on your system to try and narrow down the cause of the fault.
attr = h5a.create(self._id, self._e(tempname), htype, space)
File “h5py\_objects.pyx”, line 54, in h5py._objects.with_phil.wrapper
File “h5py\_objects.pyx”, line 55, in h5py._objects.with_phil.wrapper
File “h5py\h5a.pyx”, line 47, in h5py.h5a.create
RuntimeError: Unable to create attribute (object header message is too large)
I’m trying to save weights of SeRexNext-101,during which im facing the error.
https://github.com/qubvel/classification_models/blob/master/tests/test_models.py
@Jason Brownlee u can try saving weights from this github, Please can u help me out.
Perhaps try posting your error and code on stackoverflow.
Hey guys,
I want to know how can i update value of model, like i have better model, version 2 and not need to stop service, with use of version 1 before in Keras. I want to say a module manager model, can update new version model, and not need to break service, or something like this. Thank you.
You have a few options.
You can replace the weights and continue to use the topology. You can replace the topology and weights.
You can also continue learning from the existing set of weights, see this post:
https://machinelearningmastery.com/update-lstm-networks-training-time-series-forecasting/
thanks you so much jason.
You’re welcome.
Hi Jason,
do you know if it’s possible saving the model only and every time it’s accuracy over the validation set has improved (after each epoch)?
and is it possible checking the validation in a higher frequency than every epoch?
thanks!
I would expect so George, the callback is quite configurable:
https://keras.io/callbacks/#modelcheckpoint
Hi Dr. Jason,
I am using keras with Tensorflow back-end. I have saved my model as you mentioned here. But the problem was it takes some longer time than expected to load the weights. I am only using a CPU (not a GPU) since my model is kind of a small model. Can you please let me know how to improve the loading time of the model? Compared to sci-kit learn pickled model’s loading time, this is very high (nearly about 1 minute).
That is a long time.
Confirm that it is Keras causing the problem.
Perhaps it is something else in your code?
Perhaps you have a very slow HDD?
Perhaps you are running out of RAM for some reason?
Hi Jason,
how can I save a model after gridsearch ? I keep getting errors: “AttributeError: ‘KerasClassifier’ object has no attribute ‘save'”
Hi Jason,
I have two questions,
1) why you compile() the model a second time after load_weights() to reload the model from file?
2) in both examples, you use one optimizer to compile() before the fit(), but pass a different optimizer to compile() after load_weights() , isn’t that problematic? if not, then why should we use different optimizer?
It used ti be required to compile a loaded model.
I saved the model and weights. Then I uploaded the model from other python script, its not working.Why?
Its working if saving and loading the model is within same python script.
I am puzzled with the behaviour. Any help, please.
What is the problem exactly? Is there an error?
Thanks for the article! Helped a lot..
I had the following doubt though –
The following from the Keras docs itself:
“””
You can use model.save(filepath) to save a Keras model into a single HDF5 file which will contain:
– the architecture of the model, allowing to re-create the model
– the weights of the model
– the training configuration (loss, optimizer)
– the state of the optimizer, allowing to resume training exactly where you left off.
“””
As it says, it also saves the training configuration (loss, optimizer) why are we again compiling after loading the model and weights? Why don’t we just directly evaluate on test data?
The API has changed since I wrote this tutorial. You can now save the model in one file and you no longer need to compile after loading.
Hi Jason,
I’m keras novice and I really enjoyed your short tutorials – I have learned a lot.
Perhaps you can advice me how to push the concept of saving/loading net config for a more complex case, when 2 Sequential nets are merged to the new sequential net, sth like this:
model1 = Sequential()
…
model2 = Sequential()
…
model3 = Sequential()
model3.add(Merge([model1, model2], mode=’concat’))
…
I can save/load each model[1-3] separately. But after I load the 3 pieces back I do not know how to glue them together? Can you help how to execute equivalence of
model3.add(Merge([model1, model2], mode=’concat’)) after 3 sub-nets were read in from Yaml?
Below is a simple toy code which is missing just this last step.
Thanks in advance
Jan
– – –
cat toy-2LSTM-save-model.py
#!/usr/bin/env python
“””
definse multi branch Sequential net with Merge()
save net to yaml,
reads it back (and looses some pieces)
“””
import os, time
import warnings
os.environ[‘TF_CPP_MIN_LOG_LEVEL’] = ‘3’ #Hide messy TensorFlow warnings
warnings.filterwarnings(“ignore”) #Hide messy Numpy warnings
from keras.datasets import mnist
from keras import utils as np_utils
from keras.models import Sequential, load_model, model_from_yaml
from keras.callbacks import EarlyStopping, ModelCheckpoint
from keras.layers import Dense, Dropout, Merge, LSTM
import yaml
print(‘build_model:’)
inp_sh1=(10, 20)
inp_sh2=(11, 22)
print(‘build_model inp1:’,inp_sh1,’ inp2:’,inp_sh2)
lstm_na=30
lstm_nb=40
dens_nc=30
model1 = Sequential()
model1.add(LSTM(lstm_na, input_shape=inp_sh1))
model2 = Sequential()
model2.add(LSTM(lstm_nb, input_shape=inp_sh2 ))
model3 = Sequential()
model3.add(Merge([model1, model2], mode=’concat’))
model3.add(Dense(1, activation=’sigmoid’)) # predicts only 0/1
# Compile model
model3.compile(loss=’binary_crossentropy’, optimizer=’adam’, metrics=[‘accuracy’])
print(‘input1:’,inp_sh1, ‘input2:’,inp_sh2)
print(‘1st LSTM branch:’)
model1.summary() # will print
print(‘2nd LSTM branch:’)
model2.summary()
print(‘final Dense branch:’)
model3.summary()
print(“———– Save model as YAML ———–“)
yamlRec1 = model1.to_yaml()
yamlRec2 = model2.to_yaml()
yamlRec3 = model3.to_yaml()
with open(‘jan.model1.yaml’, ‘w’) as outfile:
yaml.dump(yamlRec1, outfile)
with open(‘jan.model2.yaml’, ‘w’) as outfile:
yaml.dump(yamlRec2, outfile)
with open(‘jan.model3.yaml’, ‘w’) as outfile:
yaml.dump(yamlRec3, outfile)
print(“———– Read model from YAML ———–“)
with open(‘jan.model1.yaml’, ‘r’) as inpfile:
yamlRec1b=yaml.load(inpfile)
model1b = model_from_yaml(yamlRec1b)
model1b.summary() # will print
with open(‘jan.model2.yaml’, ‘r’) as inpfile:
yamlRec2b=yaml.load(inpfile)
model2b = model_from_yaml(yamlRec2b)
model2b.summary() # will print
with open(‘jan.model3.yaml’, ‘r’) as inpfile:
yamlRec3b=yaml.load(inpfile)
model3b = model_from_yaml(yamlRec3b)
model3b.summary() # will print
Perhaps you can define your model using the function API and save it as one single model.
Alternatively, perhaps you can load the individual models and use the function API to piece it back together.
I have a post on the functional API scheduled, but until then, you can read about it here:
https://keras.io/getting-started/functional-api-guide/
Thanks a lot Jason !
After I switched to net = concatenate([net1,net2]) it works like a charm.
I’m attaching working toy-example. Fell free to erase my previous not-working code.
Thanks again
Jan
– – – – –
import yaml
from keras.layers import Dense, LSTM, Input, concatenate
from keras.models import Model, load_model, model_from_yaml
input1 = Input(shape=(10,11), name=’inp1′)
input2 = Input(shape=(20,22), name=’inp2′)
print(‘build_model inp1:’,input1.get_shape(),’ inp2:’,input2.get_shape())
net1= LSTM(60) (input1)
net2= LSTM(40) (input2)
net = concatenate([net1,net2],name=’concat-jan’)
net=Dense(30, activation=’relu’)(net)
outputs=Dense(1, activation=’sigmoid’)(net) # predicts only 0/1
model = Model(inputs=[input1,input2], outputs=outputs)
# Compile model
model.compile(loss=’binary_crossentropy’, optimizer=’adam’, metrics=[‘accuracy’])
model.summary() # will print
print(“———– Save model as YAML ———–“)
yamlRec = model.to_yaml()
with open(‘jan.model.yaml’, ‘w’) as outfile:
yaml.dump(yamlRec, outfile)
print(“———– Read model from YAML ———–“)
with open(‘jan.model.yaml’, ‘r’) as inpfile:
yamlRec4=yaml.load(inpfile)
model4 = model_from_yaml(yamlRec4)
model4.summary() # will print
Nice work Jan!
Hi, I have a five layer model. I have save the model later I want to load only the first four layers. would you plese tell me is it possible?
I would recommend loading the whole model and then re-defining it with the layer you do not want removed.
I have trained a CNN containing 3 convolution layers and 3 maxpooling layers for text classification. First top n words are picked from the dataset containing 1000 documents and embedding matrix is constructed for them by looking for these words in Glove embeddings and appending the corresponding word vector to if the word is found in Glove embeddings.
I tested the validation accuracy. I saved the model in h5 format.
Now, I want to load the model in another python file and use to predict the class label of unseen document. I used the following code
from keras.models import load_model
import keras.preprocessing.text
from keras.preprocessing.text import Tokenizer
from keras.preprocessing.sequence import pad_sequences
import numpy as np
import json
MAX_NUMBER_OF_WORDS= 20000
MAX_SEQUENCE_LENGTH = 1000
model1 = load_model(‘my_model.h5’)
f = open(‘/home/siri/Japan_Project/preprocessing/complete_data_stop_words/technology/X.txt’, encoding=’latin-1′)
text = f.read()
#print(text.shape)
tokenizer = Tokenizer(num_words=MAX_NUMBER_OF_WORDS)
tokenizer.fit_on_texts(text)
print(‘\n text = ‘)
print(text)
sequence_list = tokenizer.texts_to_sequences(text)
print(‘\n text to sequences= ‘)
print(sequence_list)
data = pad_sequences(sequence_list, maxlen=MAX_SEQUENCE_LENGTH)
print(data)
print(‘\n np.array(data)’)
print(np.array(data))
prediction = model1.predict(np.array(data))
print(prediction)
y_classes = prediction.argmax(axis=-1)
with open(‘data.json’, ‘r’) as fp:
labels_index = json.load(fp)
print(y_classes)
for k, v in labels_index.items():
print(“\n key= “,k,”val= “,v)
print(‘\n printing class label=’)
for k, v in labels_index.items():
if y_classes[0]==v:
print(“\n key= “,k,”val= “,v)
My doubt is I did not use word embeddings as input to model now, Instead I used numpy.array(data). Is it correct? Can we give word embeddings as input to predict function of keras.
I also saved the class label index (dictionary of class labels) in data.json file after training. and loaded it back in this file. to know the class label of the prediction. Is it correct?
I’m not sure I follow completely.
Generally, word embeddings are we weights and must be saved and loaded as part of the model in the Embedding layer.
Does that help?
How to check if embedding layer is saved or not? If it is saved, how to give unseen text document as input to predict function?
If the Embedding layer is part of the model and you save the model, then the embedding layer will be saved with the model.
Thanks for this valuable answer Jason!
It took me some time to get that. i.e. in production, I just can call my model (h5) and get the word embeddings as well.
Production code is hard :-/
Sir . the way you have saved all the items weights in yolov3.weigths how to save for those items which I want.
I did not save them, I simply show how to load them.
Hi Jason:
I am able to load weights and the model as well as the label encoder and have verified that the test set gives the same predictions with the loaded model.
My problem – to which I am not able to find a definitive answer even after searching – is that when a new input comes in how do I one-hot encode the categorical variables associated with this new input so that the order of the columns exactly matches the training data?
Without being able to do this my accuracy on a set of new inputs is approaching 10% while the validation accuracy is 89%.
Simple question is how to encode categorical variables so that the input data for the set of new-inputs matches the training set? Probably not a real deep learning question but without doing this my sophisticated LSTM model is just not working.
Help will be greatly appreciated!
You must use the same encoding as was used during training.
Perhaps you use your own transform code.
Perhaps you save the transform object.
Perhaps you re-create the transform when needed from training data and confirm that it is consistent.
Does that help?
Hi Jason,
I am using pd.get_dummies to transform to a one hot encoded matrix. How do I reuse that as this is not a Label Encoder or a One Hot Encoder? My training set is 24000 rows and 5255 columns
When I use pd.get_dummies on 3 new items I will get a 3 rows by 6 columns matrix and this cannot be fed into the model as the model expects 5255 rows. Padding with zeros to make up the shortfall is only ruining the case and the output accuracy is ranging in 10% range while validation accuracy is 89%; during validation there is no issue as the train test split is being done AFTER the pd.get dummies has executed and turned the input X into a one hot encoded matrix. This seems to be strange problem as ALL who use the trained model for prediction will have exactly the same problem with any one hot encoded model and thus a simple solution should have been found on the net.
Is there a way to transform the pd.get_dummies to an encoder type object and reload and re-use the same on the real time data. That would make life very simple….
Do let me know.
Thanks
Arnab
I would recommend using the sklearn encoding over the pandas method so that you can either save the object and/or easily reverse the operation.
Hi,
I am getting following error when I applied this knowledge to my code
##########
raceback (most recent call last):
File “DNNrect_VC_VC_Challenge.py”, line 198, in
model.save(path + SRC[sr] + ‘-‘ + TGT[tg] + ‘/DNNerect_25/’+str(NUTTS[nutt])+’/model.hdf5’)
File “/usr/local/lib/python2.7/dist-packages/keras/engine/topology.py”, line 2429, in save
save_model(self, filepath, overwrite)
File “/usr/local/lib/python2.7/dist-packages/keras/models.py”, line 109, in save_model
topology.save_weights_to_hdf5_group(model_weights_group, model_layers)
File “/usr/local/lib/python2.7/dist-packages/keras/engine/topology.py”, line 2708, in save_weights_to_hdf5_group
g = f.create_group(layer.name)
File “/usr/lib/python2.7/dist-packages/h5py/_hl/group.py”, line 41, in create_group
gid = h5g.create(self.id, name, lcpl=lcpl)
File “h5g.pyx”, line 145, in h5py.h5g.create (h5py/h5g.c:2536)
ValueError: unable to create group (Symbol table: Unable to initialize object)
#######################
Can you please comment what can be possible reason ?
Sorry, the cause of the fault is not obvious.
Perhaps post to stackoverflow?
Hi Jason,
Firstly very thanks for your attempt to write this valuable blog.Very great-full to you. I have a question as follows :
1) I am using below code to train the data and target values to RNN using Keras for 1000000 epoch and save the trained model and weights to disk using the JSON and HDF5 as you mentioned in this blog. “This part works well”, and I am able to generate model.h5 and model.json in the same working directory. Now by using another python program in the same directory i want to use the trained model and weights, but for any values I pass to the trained model, I get the same output which I got while training. I tried to compile with new values still it didn’t help. Is there anything I can do? Here are the code that I have:
rnn3.py [First file that trains for 1000000 epoch]
=========================================
import numpy as np
from keras.models import Sequential
from keras.layers import Dense
from keras.layers import LSTM
from keras.models import model_from_json
data =[688,694.5,700.95,693,665.25,658,660.4,656.5,654.8,652.9,660,642.5,
655,684,693.8,676.2,673.7,676,676,679.5,681.75,675,657,654.1,657,647.1,647.65,
651,639.95,636.95,635,635.5,640.15,636,624,629.95,632.9,622.45,630.1,625,607.4,
600,604.8,616,610.25,585,559.4,567,573,569.7,553.25,560.8,566.95,555,548.9,
554.4,558,562.3,564,557.55,562.1,564.9,565]
target = [691.6,682.3,690.8,697.25,691.45,661,659,660.8,652.55,649.7,649.35,654.1,639.75,654,687.1,687.65,676.4,672.9,678.95,
677.7,679.65,682.9,662.6,655.4,652.8,653,652.1,646.55,651.2,638.05,638.65,630.2,635.85,639,634.6,619.6,621.55,625.65,
625.4,631.2,623.75,596.75,604.35,605.05,616.45,600.05,575.85,559.3,569.25,572.4,567.1,551.9,561.25,565.75,552.95,548.5,
553.25,557.2,571.2,563.3,559.8,558.4,563.95]
#data = [688,694.5,700.95,693,665.25,658,660.4,656.5,654.8,652.9]
data = np.array(data, dtype=float)
#target = [691.6,682.3,690.8,697.25,691.45,661,659,660.8,652.55,649.7]
target = np.array(target,dtype=float)
data = data.reshape((1,1,len(data)))
target = target.reshape((1,1,len(target)))
#x_test=[688,694.5,700.95,693,665.25,658,660.4,656.5,654.8,652.9]
x_test =[688,694.5,700.95,693,665.25,658,660.4,656.5,654.8,652.9,660,642.5,
655,684,693.8,676.2,673.7,676,676,679.5,681.75,675,657,654.1,657,647.1,647.65,
651,639.95,636.95,635,635.5,640.15,636,624,629.95,632.9,622.45,630.1,625,607.4,
600,604.8,616,610.25,585,559.4,567,573,569.7,553.25,560.8,566.95,555,548.9,
554.4,558,562.3,564,557.55,562.1,564.9,565]
x_test=np.array(x_test).reshape((1,1,len(x_test)))
#y_test=[660,642.5,655,684,693.8,676.2,673.7,676,676,679.5]
y_test =[700.95,693,665.25,658,660.4,656.5,654.8,652.9,660,642.5,
655,684,693.8,676.2,673.7,676,676,679.5,681.75,675,657,654.1,657,647.1,647.65,
651,639.95,636.95,635,635.5,640.15,636,624,629.95,632.9,622.45,630.1,625,607.4,
600,604.8,616,610.25,585,559.4,567,573,569.7,553.25,560.8,566.95,555,548.9,
554.4,558,562.3,564,557.55,562.1,564.9,565,688,694.5]
y_test=np.array(y_test).reshape((1,1,len(y_test)))
model = Sequential()
model.add(LSTM(len(data),input_shape=(1,63),return_sequences=True))
model.add(Dense(63))
model.compile(loss=’mean_absolute_error’, optimizer=’adam’,metrics=[‘accuracy’])
model.fit(data,target, nb_epoch=1000000, batch_size=1, verbose=2,validation_data=(x_test,y_test))
# serialize model to JSON
model_json = model.to_json()
with open(“model.json”, “w”) as json_file:
json_file.write(model_json)
# serialize weights to HDF5
model.save_weights(“model.h5”)
print(“Saved model to disk”)
# load json and create model
json_file = open(‘model.json’, ‘r’)
loaded_model_json = json_file.read()
json_file.close()
loaded_model = model_from_json(loaded_model_json)
# load weights into new model
loaded_model.load_weights(“model.h5”)
print(“Loaded model from disk”)
# Evaluate loaded model in test data
loaded_model.compile(loss=’binary_crossentropy’,optimizer=’rmsprop’,metrics=[‘accuracy’])
score = loaded_model.evaluate(data,target, verbose=0)
predict = loaded_model.predict(y_test)
print(predict)
predict = loaded_model.predict(x_test)
print(predict)
Output I get for rnn4.py
====================
[[[ 691.59997559 682.30004883 690.80004883 697.24987793 691.45007324
661.00012207 658.99987793 660.80004883 652.55004883 649.70007324
649.34997559 654.09997559 639.75 654. 687.09997559
687.65002441 676.40002441 672.90002441 678.95007324 677.70007324
679.65002441 682.90002441 662.59997559 655.40002441 652.80004883
652.99987793 652.09997559 646.55004883 651.20007324 638.05004883
638.65002441 630.20007324 635.84997559 639. 634.59997559
619.59997559 621.55004883 625.65002441 625.40002441 631.20007324
623.74987793 596.74987793 604.34997559 605.05004883 616.45007324
600.05004883 575.84997559 559.30004883 569.25 572.40002441
567.09997559 551.90002441 561.25012207 565.75012207 552.95007324
548.50012207 553.24987793 557.20007324 571.20007324 563.30004883
559.80004883 558.40002441 563.95007324]]]
[[[ 691.59997559 682.30004883 690.80004883 697.24987793 691.45007324
661.00012207 658.99987793 660.80004883 652.55004883 649.70007324
649.34997559 654.09997559 639.75 654. 687.09997559
687.65002441 676.40002441 672.90002441 678.95007324 677.70007324
679.65002441 682.90002441 662.59997559 655.40002441 652.80004883
652.99987793 652.09997559 646.55004883 651.20007324 638.05004883
638.65002441 630.20007324 635.84997559 639. 634.59997559
619.59997559 621.55004883 625.65002441 625.40002441 631.20007324
623.74987793 596.74987793 604.34997559 605.05004883 616.45007324
600.05004883 575.84997559 559.30004883 569.25 572.40002441
567.09997559 551.90002441 561.25012207 565.75012207 552.95007324
548.50012207 553.24987793 557.20007324 571.20007324 563.30004883
559.80004883 558.40002441 563.95007324]]]
rnn4.py [2nd file in the same directory of rnn3.py that like to reuse model.h5 and model.json]
======================================================================from keras.models import model_from_json
import numpy as np
#x_test =[688,694.5,700.95,693,665.25,658,660.4,656.5,654.8,652.9,660,642.5,
#655,684,693.8,676.2,673.7,676,676,679.5,681.75,675,657,654.1,657,647.1,647.65,
#651,639.95,636.95,635,635.5,640.15,636,624,629.95,632.9,622.45,630.1,625,607.4,
#600,604.8,616,610.25,585,559.4,567,573,569.7,553.25,560.8,566.95,555,548.9,
#554.4,558,562.3,564,557.55,562.1,564.9,565]
x_test =[[i for i in range(63)]]
x_test=np.array(x_test).reshape((1,1,63))
# load json and create model
json_file = open(‘model.json’, ‘r’)
loaded_model_json = json_file.read()
json_file.close()
loaded_model = model_from_json(loaded_model_json)
# load weights into new model
loaded_model.load_weights(“model.h5”)
print(“Loaded model from disk”)
loaded_model.compile(loss=’binary_crossentropy’,optimizer=’rmsprop’,metrics=[‘accuracy’])
predict = loaded_model.predict(x_test)
print(predict)
Output I get for rnn4.py
==================
[[[ 691.59997559 682.30004883 690.80004883 697.24987793 691.45007324
661.00012207 658.99987793 660.80004883 652.55004883 649.70007324
649.34997559 654.09997559 639.75 654. 687.09997559
687.65002441 676.40002441 672.90002441 678.95007324 677.70007324
679.65002441 682.90002441 662.59997559 655.40002441 652.80004883
652.99987793 652.09997559 646.55004883 651.20007324 638.05004883
638.65002441 630.20007324 635.84997559 639. 634.59997559
619.59997559 621.55004883 625.65002441 625.40002441 631.20007324
623.74987793 596.74987793 604.34997559 605.05004883 616.45007324
600.05004883 575.84997559 559.30004883 569.25 572.40002441
567.09997559 551.90002441 561.25012207 565.75012207 552.95007324
548.50012207 553.24987793 557.20007324 571.20007324 563.30004883
559.80004883 558.40002441 563.95007324]]]
Problem
========
For different input values we expect different output values after recompiling. Why do we get same output of any input values?
The output of the network should be specific (contingent) to the input provided when making a prediction.
If this is not the case, then perhaps your model has overfit the training data?
Hi Brownlee,
Thanks for the quick reply. I was not able to understand “output of the network should be specific (contingent) to the input provided ” Could you explain it more..Perhaps i dint get the context correctly
It suggests that there is a problem with your network as different input should give different output.
I don’t know what the problem could be, I don’t have the capacity to debug your code. Perhaps one of these resources will help:
https://machinelearningmastery.com/get-help-with-keras/
Hi Jason,
Thanks for the amazing post. Really helps people who are new to ML.
I am trying to run the below code
model_json = model.to_json()
with open(“model.json”, “w”) as json_file:
json_file.write(model_json)
getting the error as ‘NameError: name ‘model’ is not defined’
Can you help ?
“model” will be the variable for your trained model.
Fantastic post. I could save and retrieve in local. But in AWS lambda facing a problem of loading weights because of HDF5 format. Can you please suggest any resolution or work around for the same.
Sorry to hear that. I would have expected h5 format to be cross-platform. I believe it is.
Perhaps it is a Python 2 vs Python 3 issue.
sir,
plz explain the python code for feature selection using meta heuristic algorithms like firefly algorithm,particle swarm optimization,brain storm optimization etc…
Thanks for the suggestion, I hope to cover the topic in the future.
Should I compile the model for evaluation, after load model and weights
No need to compile after loading any more I believe, the API has changed.
Thanks Jason for your post. I have a question.
Is there any similar method to have an output file indicating that a model is compiled? Each time that I run my file, it takes time to compile the model and then fit and evaluate the data. I want to change parameters/variables/hyperparameters and run the file again, so I want to have speed up as mush as possible.
I believe you don’t need to compile the model any longer.
Hi Jason,
Thank you for the invaluable help this blog provides developers,
I am facing the same problem as @Lotem above.
I have one script which builds a model (accuracy 60%) and saves it in a different directory.
However, when I load the model back into another script the accuracy decreases to 55%, the predicted values are different.
I have checked the weights and they are the same,
I have set:
from numpy.random import seed
seed(1)
from tensorflow import set_random_seed
set_random_seed(2)
for both files, but I still cannot get the loaded model to give the same accuracy.
I should also mention that the dataset contains the same features.
Any help would be much appreciated as I have been going round in circles having a look at:
https://github.com/keras-team/keras/issues/4875
https://github.com/keras-team/keras/issues/7676
If possible, could you post an example where you save a model and load it in different sessions?
Thank you again
Interesting, I have not had this problem myself.
Some ideas to explore:
– Are you able to replicate the same fault on a different machine? e.g. on AWS?
– Are all of your libraries up to date.
– Are you saving weights and topology to a single file or separate files?
– Are you 100% sure the data used to evaluate the model before/after saving is identical?
Hey Jason, the tutorial is very helpful.
However, there is one question that I have. I trained a LSTM model for sequence classification problem and observed the following.
Within the same python session as the one where model is trained, I get the exact results (loss, accuracy, predicted probabilities) from the loaded_model (using json format). But, in a new python session the results are not exactly the same but are very close.
Can you please help me understand what could be the possible reason for slightly different results? Is there any other random_seed that needs to be fixed for exact match of the results?
Looking forward to your response.
Thanks,
Tarun
There must be some randomness related to the internal state.
I don’t know for sure. Interesting finding!
Hi!
I need to deploy my LSTM model as an API, how should I go about it?
Thank you so much
I don’t have material on this, sorry. I do have general material on putting models into production here that may interest you:
https://machinelearningmastery.com/deploy-machine-learning-model-to-production/
I have seen a basic example in https://machinelearningmastery.com/save-load-keras-deep-learning-models/
How to save model in ini or cfg format instead of json
Sorry, other formats are not supported. You may have to write your own code.
Hi Jason,
I trained the model in keras and got validation accuracy around 50 %, but when i save the model and reload it again as per the code you mentioned, the validation accuracy is just 5%……it seems the loaded model behaves like untrained model
That is odd. I have not seen this myself. Perhaps try posting this as a fault to the Keras issue list:
https://machinelearningmastery.com/get-help-with-keras/
Hi Jason
is it possible that after saving existing model, i want to retrain it on new data. Let’s say if my existing model was trained on 100 dataset, but after sometime i want to retrain it on some new 50 dataset so that it could learn some new dataset as well to make good prediction. It means it would have now impact of 150 dataset.
Need your help.
Thanks in advance.
Yes, here is an example of updating a model:
https://machinelearningmastery.com/update-lstm-networks-training-time-series-forecasting/
Hi,
Is there any way to convert a h5 and a json files into one hdf5 file?
I have many pairs of h5 and json files but when I need to convert keras models to tensorflow pb files, hdf5 files are needed.
Thanks,
Yes, Keras has a new API for saving loading a single file for a model:
https://keras.io/getting-started/faq/#how-can-i-save-a-keras-model
Hi,
I am using keras with a tensorflow backend but my .h5 file soes not save because its says not UTF-8 encoded – so saving is disabled.
Do you know how to get around this problem?
Thanks
I have not seen this. Perhaps try posting to the Keras list:
https://machinelearningmastery.com/get-help-with-keras/
Hi jason, thank you very much for this example, it is the most helpful when dealing with issues regarding saving and loading. I am having a fitting issue after I save and load my model in a different file than where it was trained. It keeps saying that it was not fitted.
I tried doing a prediction in the same file where the model was made and fitted and it works perfectly.
file where I saved the fitted model:
file where I am trying to load the fitted model:
This gives me the following error:
sklearn.exceptions.NotFittedError: This StandardScaler instance is not fitted yet. Call ‘fit’ with appropriate arguments before using this method.
-Thank you in advance
Sorry to hear that, I have not seen this fault. I’m eager to help, but I cannot debug your example sorry.
I will keep at it, thank you for looking into it anyways.
Nice Explanation Sir.
Thank you.
Hi Jason,
Thank you so much for this great tutorial, really helpful. Can we load the saved models in C++. I wish to train using python and then use it for prediction in my C++ program. Is it possible?
Perhaps. I have not done it.
Hey, did you find a way to used trained python models in c++!
https://github.com/Dobiasd/frugally-deep is amazing.
Thanks for sharing.
Hi Jason,
Thanks for the nice tutorial.
I have already read this one too [https://machinelearningmastery.com/make-predictions-long-short-term-memory-models-keras/]. But I do not understand which tutorial is proper for creating a model = (finalized a model to reuse it for many unseen data)??
In the above link we should write model.save(‘lstm_model.h5’) to build a finalized model and must applying the “model = load_model(‘lstm_model.h5’)” command to load it but in this tutorial, you used different commands to serializing and load final model such as these commands for serializing models [[model_json = model.to_json()
with open(“model.json”, “w”) as json_file:
json_file.write(model_json)
# serialize weights to HDF5
model.save_weights(“model.h5”)
print(“Saved model to disk”)]]
in this tutorial you used different commands for serializing model and also used different commands for serializing weights
and you also applied different commands for loading finalized model and also different commands for loading weights such as [[ # load json and create model
json_file = open(‘model.json’, ‘r’)
loaded_model_json = json_file.read()
json_file.close()
loaded_model = model_from_json(loaded_model_json)
# load weights into new model
loaded_model.load_weights(“model.h5”)
print(“Loaded model from disk”)]].
I get confused about which link is proper for saving and loading finalized model(to reuse model for predicting unseeen data many times).this link = [https://machinelearningmastery.com/save-load-keras-deep-learning-models/] or this one [https://machinelearningmastery.com/make-predictions-long-short-term-memory-models-keras/]???
in this tutorial you used different commands for serializing model and also used different commands for serializing weights.
when You save a model in the mentioned link, the single file will contain the model architecture and weights but in this tutorial U have to use some differents commands to save model and save weights separately.
what is the difference between serializing model and saving model??
thank you very much for the time you spend for guiding me.
I really appreciate.
This post provides a good summary for how to finalize a model:
https://machinelearningmastery.com/train-final-machine-learning-model/
Generally, train the model on all available data then save it to file. Later load it and use it to start making predictions on new data.
Use any method you prefer to save your model. It does not really matter which you use. Some developers have a preference for the type of file used to save the model.
Does that help?
Hi Jason,
I am realy grateful for replying but I have already read this link [https://machinelearningmastery.com/train-final-machine-learning-model/].
I think U did not understand my purpose. If I want to ask my question clearly I should say in this way:[what is the diffrence between the method described in this link:(https://machinelearningmastery.com/save-load-keras-deep-learning-models/) and the method explained in this link:(https://machinelearningmastery.com/make-predictions-long-short-term-memory-models-keras/)?
Are both approaches suitable for saving and loading deep models?
If I save in this way:(model.save(‘lstm_model.h5’) and then loading model in this way (model = load_model(‘lstm_model.h5’)) will the weights and model architecture save in that file wuth the mentioned command?? will the weights and model architecture load with the given command?
I hope my question is clear to understanding.
Any answering will be appreciated
They are equivalent. Choose the method you prefer, either saving to one file or two.
Hi Jason,
I am having a little issue with loading the model. The issue basically that your methods causes compiler error as follow.
ValueError: Dimension 0 in both shapes must be equal, but are 4096 and 1000. Shapes are [4096,5] and [1000,5]. for ‘Assign_30’ (op: ‘Assign’) with input shapes: [4096,5], [1000,5].
I think it is best if I also included my code for creating the model.
import keras
from keras import backend as K
from keras.models import Sequential, Model
from keras.layers import Activation, Dense
from keras.optimizers import Adam
from keras.metrics import categorical_crossentropy
from keras.preprocessing.image import ImageDataGenerator
from keras.preprocessing import image
from keras.applications.vgg16 import VGG16
train_data_dir = ‘./data/train’
validation_data_dir = ‘./data/validation’
test_path = ‘./data/test’
# dimensions of our images.
img_width, img_height = 224, 224
if K.image_data_format() == ‘channels_first’:
input_shape = (3, img_width, img_height)
else:
input_shape = (img_width, img_height, 3)
nb_train_samples = 5400
nb_validation_samples = 2000
top_epochs = 10
fit_epochs = 10
batch_size = 20
train_generator = ImageDataGenerator().flow_from_directory(
train_data_dir,
target_size=(img_height, img_width),
batch_size=batch_size,
classes=[‘backpack’, ‘Baseball’, ‘Coffee-mug’, ‘orange’, ‘running-shoe’])
validation_generator = ImageDataGenerator().flow_from_directory(
validation_data_dir,
target_size=(img_height, img_width),
batch_size=batch_size,
classes=[‘backpack’, ‘Baseball’, ‘Coffee-mug’, ‘orange’, ‘running-shoe’])
vgg16_model = VGG16()
model = Sequential()
for layer in vgg16_model.layers:
model.add(layer)
model.layers.pop()
for layer in model.layers:
layer.trainable = False
model.add(Dense(5, activation=’softmax’))
model.compile(Adam(lr=.0001), loss=’categorical_crossentropy’, metrics=[‘accuracy’])
model.fit_generator(train_generator, steps_per_epoch=270,
validation_data=validation_generator, validation_steps=100, epochs=top_epochs, verbose=2)
test_batches = ImageDataGenerator().flow_from_directory(test_path, target_size=(img_width,img_height), classes=[‘backpack’, ‘Baseball’, ‘Coffee-mug’, ‘orange’, ‘running-shoe’], batch_size=50)
predictions = model.predict_generator(test_batches, steps=1, verbose=0)
predictions
model.save(‘classes.h5’)
model.save_weights(‘classes-weights.h5’)
model_json=model.to_json()
with open(“classes.json”,”w”) as json_file:
json_file.write(model_json)
With output after training and testing as follow:
Training:
Epoch 10/10 – 91s – loss: 0.0775 – acc: 0.9985 – val_loss: 0.2168 – val_acc: 0.9570
Testing:
array([[0.01292046, 0.01129738, 0.9499369 , 0.01299447, 0.01285083],
[0.02066054, 0.9075736 , 0.02441081, 0.02221865, 0.02513652],
[0.01651708, 0.01449703, 0.01844079, 0.93347657, 0.01706842],
[0.01643269, 0.01293082, 0.01643352, 0.01377147, 0.94043154],
[0.02066054, 0.9075736 , 0.02441081, 0.02221865, 0.02513652],
[0.01651708, 0.01449703, 0.01844079, 0.93347657, 0.01706842],
[0.02066054, 0.9075736 , 0.02441081, 0.02221865, 0.02513652],
[0.9319746 , 0.0148032 , 0.02086181, 0.01540569, 0.01695477],
[0.01643269, 0.01293082, 0.01643352, 0.01377147, 0.94043154],
[0.02066054, 0.9075736 , 0.02441081, 0.02221865, 0.02513652],
[0.01643282, 0.01293091, 0.01643365, 0.01377157, 0.940431 ],
[0.01292046, 0.01129738, 0.9499369 , 0.01299447, 0.01285083],
[0.01292046, 0.01129738, 0.9499369 , 0.01299447, 0.01285083],
[0.01651709, 0.01449704, 0.0184408 , 0.93347657, 0.01706843],
[0.01692604, 0.0131789 , 0.01675364, 0.01416142, 0.93898004],
[0.02066054, 0.9075736 , 0.02441081, 0.02221865, 0.02513652],
[0.01292046, 0.01129738, 0.9499369 , 0.01299447, 0.01285083],
[0.02066054, 0.9075736 , 0.02441081, 0.02221865, 0.02513652],
[0.9319746 , 0.0148032 , 0.02086181, 0.01540569, 0.01695477],
[0.01651708, 0.01449703, 0.01844079, 0.93347657, 0.01706842],
[0.01643269, 0.01293082, 0.01643352, 0.01377147, 0.94043154],
[0.01651708, 0.01449703, 0.01844079, 0.93347657, 0.01706842],
[0.01643269, 0.01293082, 0.01643352, 0.01377147, 0.94043154],
[0.9319746 , 0.0148032 , 0.02086181, 0.01540569, 0.01695477],
[0.01651708, 0.01449703, 0.01844079, 0.93347657, 0.01706842],
[0.01643269, 0.01293082, 0.01643352, 0.01377147, 0.94043154],
[0.01292046, 0.01129738, 0.9499369 , 0.01299447, 0.01285083],
[0.9319746 , 0.0148032 , 0.02086181, 0.01540569, 0.01695477],
[0.01292046, 0.01129738, 0.9499369 , 0.01299447, 0.01285083],
[0.01651708, 0.01449703, 0.01844079, 0.93347657, 0.01706842],
[0.9319746 , 0.0148032 , 0.02086181, 0.01540569, 0.01695477],
[0.01292046, 0.01129738, 0.9499369 , 0.01299447, 0.01285083],
[0.01643269, 0.01293082, 0.01643352, 0.01377147, 0.94043154],
[0.01643269, 0.01293082, 0.01643352, 0.01377147, 0.94043154],
[0.02984857, 0.01736826, 0.9126526 , 0.01986017, 0.02027044],
[0.9319746 , 0.0148032 , 0.02086181, 0.01540569, 0.01695477],
[0.02066054, 0.9075736 , 0.02441081, 0.02221865, 0.02513652],
[0.02066054, 0.9075736 , 0.02441081, 0.02221865, 0.02513652],
[0.9319746 , 0.0148032 , 0.02086181, 0.01540569, 0.01695477],
[0.01651708, 0.01449703, 0.01844079, 0.93347657, 0.01706842],
[0.01653831, 0.01302506, 0.01664082, 0.01386674, 0.9399291 ],
[0.0165362 , 0.01452375, 0.01846231, 0.9333804 , 0.01709736],
[0.02066054, 0.9075736 , 0.02441081, 0.02221865, 0.02513652],
[0.9319746 , 0.0148032 , 0.02086181, 0.01540569, 0.01695477],
[0.01643269, 0.01293082, 0.01643352, 0.01377147, 0.94043154],
[0.01651708, 0.01449703, 0.01844079, 0.93347657, 0.01706842],
[0.9319746 , 0.0148032 , 0.02086181, 0.01540569, 0.01695477],
[0.02066054, 0.9075736 , 0.02441081, 0.02221865, 0.02513652],
[0.01292046, 0.01129738, 0.9499369 , 0.01299447, 0.01285083],
[0.9316361 , 0.01485772, 0.02102509, 0.01546398, 0.0170171 ]],
dtype=float32)
Booth being extremely good.
The reason that I included the above is that when I tried the load the model as follow, the code compile but the model work so bad as if it was never trained.
import keras
from keras.models import Sequential, Model
from keras.layers import Activation, Dense
from keras.optimizers import Adam
from keras.metrics import categorical_crossentropy
from keras.applications.vgg16 import VGG16
from keras.models import load_model
from keras.preprocessing import image
from keras.applications.vgg16 import preprocess_input, decode_predictions
import numpy as np
import sys
import os
os.environ[‘TF_CPP_MIN_LOG_LEVEL’]=’1′ # suppress gpu info
vgg16_model = VGG16()
model = Sequential()
for layer in vgg16_model.layers:
model.add(layer)
model.layers.pop()
for layer in model.layers:
layer.trainable = False
model.add(Dense(5, activation=’softmax’))
model.compile(Adam(lr=.0001), loss=’categorical_crossentropy’, metrics=[‘accuracy’])
model.load_weights(‘classes-weights.h5’)
img = image.load_img(‘n07747607_30848.JPEG’, target_size=(224, 224))
x = image.img_to_array(img)
x = np.expand_dims(x, axis=0)
x = preprocess_input(x)
preds = model.predict(x)
print(preds[0])
Your respond will be appreciated
I’m sorry, I cannot debug your code for you.
Perhaps post your code to stackoverflow?
Code works just fine. This issue is faced by many who uses tensorflow-GPU to train the model and save the weights to reload them later. There is an easy fix which consist of retraining the model for one epoch to retrieve the trained weight. I was hoping you might know of a better way. Thanks any how.
Sorry, I have not experienced this issue.
Hi Jason, thanks for wonderful content as always. I came across this article while working on a project for work. Do you know if it would be possible to upload the weights to cloud storage? Such as Azure blob storage? And then when you need to use the weights download them, and use them for further training?
My setup at the moment is saving my weights at a given interval, where I do the same for my loss function. I then cross-check with my loss value to see which weights are best (working with GANs so the last weight is not necessarily the best) and load the best model.
I don’t see why not, although I don’t know the specific details of “Azure blob storage”.
You could upload them to Amazon S3 without any trouble.
I am truly grateful for this wonderful tutorial.. This code helped me a lot
Thanks, I’m glad to hear that.
Hi Jason,
Your blog has helped me a lot both in my work and study. I am currently training keras models in cloud, I run into problems saving the model directly to s3.
Do you have any idea how to workaround?
Sorry, I don’t know about saving directly to S3.
Can i save another models with the same metod?
Models made by LRN, SVM,KNN Or there is another way to do it?
You can use pickle to save/load other models, see how here:
https://machinelearningmastery.com/save-load-machine-learning-models-python-scikit-learn/
Thanks Jason. A much needed blog and very nicely explained.
I’m glad it helped.
Hi Jason,
I’m loading the VGG16 pretrained model, adding a couple of dense layers and fine tuning the last 5 layers of the base VGG16. I’m training my model on mutliple gpus. I saved the model before and after training. The weights are the same inspite of having layers.trainable = True.
Please help!
Heres the code:
from keras import applications
from keras import Model
model = applications.VGG16(weights = “imagenet”, include_top = False, input_shape = (224,224,3))
model.save(‘./before_training’)
for layer in model.layers:
layer.trainable = False
for layer in model.layers[-5:]:
layer.trainable = True
x = model.output
x = Flatten()(x)
x = Dense(1024, activation = “relu”)(x)
x = Dropout(0.5)(x)
x = Dense(1024, activation = “relu”)(x)
predictions = Dense(2, activation = “softmax”)(x)
model_final = Model(input = model.input, output = predictions)
from keras.utils import multi_gpu_model
parallel_model = multi_gpu_model(model_final, gpus = 4)
parallel_model.compile(loss = “categorical_crossentropy” ….. )
datagen = ImageDataGenerator(….)
early = EarlyStopping(…)
train_generator = datagen.flow_from_directory(train_data_dir,…)
validation_generator = datagen.flow_from_directory(test_data_dir,…)
parallel_model.fit_generator(train_generator, validation_data = valiudation_generator,…)
model_final.save(‘./after_training)
That is surprising.
I have some suggestions here that might help:
https://machinelearningmastery.com/faq/single-faq/can-you-read-review-or-debug-my-code
if test_image.size != target_size:
test_image = test_image.resize(target_size)
x = image.img_to_array(test_image)
x = np.expand_dims(x, axis=0)
x = preprocess_input(x)
preds = loaded_model.predict_classes(x)
print(preds)
I have used the above code to predict on a single image and I got the output like this
[[1]]
what does that mean??
what should I do if I want the name of the predicted image by model and also I want the probabilities for each class.
It has predicted class number 1.
This post will show you how to predict probabilities:
https://machinelearningmastery.com/how-to-make-classification-and-regression-predictions-for-deep-learning-models-in-keras/
Nice blog post and nice photo… I recognized a work of the sculptor québécois «Robert Roussil» who died in 2013.
Thanks, nicely noticed!
hi jason,
how can i create a config file such that i can change the epoch size,batch size,no of cnn layers etc from the config file without editing the original cnn python script
Sure, it is all just code. You can implement it any way you want.
Hi Jason, if I have a deep learning script in keras that I created on a Linux OS and my model output is HD5… How would I deploy this on a different computer?
My Linux workstation is in an office location and I want to use my model in the field on a Windows laptop. I do have Keras installed on my Windows laptop but when I attempt to load the model with
keras load_model
I get an error that Tensorflow is not installed.And I didnt even bother trying installing Tensor flow on my Windows laptop as I think Tensorflow cannot run on Windows, correct??? Any suggestions to try?? Thanks!
The model is just an n-dimensional array of numbers (weights).
You can make predictions manually using Python code (or other languages) or you can make predictions using the Keras+TF libraries.
How you deploy the model is really an engineering decision.
Can you give me a tip (or link to one of your write ups) on how to try something different on my end? What would be a different method of saving a deep learning model in Python other than keras load_mode method? Thank you…
Yes, I meant that you can walk through the forward propagation of inputs through the network using the raw weights.
This post might give you ideas if this is new to you:
https://machinelearningmastery.com/implement-backpropagation-algorithm-scratch-python/
Hi Jason,
I am understanding this a little bit… And a model is just trained weights from what I am reading. But is there an option if a deep learning model is trained in Keras is there another way to load/save the model without using Keras? All of this stems from wanting to deploy the model on a Windows OS but the model was trained on a Linux OS, and when I use the Keras load_model method I get an error in Windows because I dont have Tensorflow installed…
Im just trying to wrap my head around what I can do if I cannot install Tensorflow on a Windows OS. Thanks!
Yes, in theory I don’t see why you couldn’t write some python code to use the weights in a saved h5 to make predictions.
This would be very easy for an MLP, and some work for other network types.
I have not done this, so this is just an off-the-cuff opinion.
I have done this for regression models from statsmodels before with great success.
model.save(‘model.h5’)
## then after a while
my_model = load_model(‘model.h5’)
## why this way bringing me error::
NameError: name ‘tf’ is not defined
Sorry, I have not seen that error.
Perhaps check that you have the latest version of the libraries installed?
I am trying to save model on this page: https://www.depends-on-the-definition.com/guide-sequence-tagging-neural-networks-python/
The code yields an accuracy of 98% approx. , But after I save the model as you have described above and re-run code for predictions from the loaded module, predictions are messed up and accuracy is dropped down to 3,4,20% after random executions. I think embeddings that are learned during training aren’t saved . i’ve also tried saving whole model using mode.save(path) and keras.reload_model() but it didn’t work.
I’ve also tried to save word2idx dictionary to preserve vocab index but unfortunately, it didn’t work.
Kindly help me a little. Thanks
I’m sorry to hear that, perhaps there is a bug.
I’d recommend posting to the Keras support:
https://machinelearningmastery.com/get-help-with-keras/
Hey jason ,
I have one question, Can we again train the model after loading it.LIke after executing load_model command can we agai train the same model with new data IF yes the how ??
Yes, load it and call fit() again.
Hi Jason.
Im doing deep learning using keras, and reading your tutotials about keras, I came up asking you a question.
If we build and train a sequential deep network with keras, and in the said model we use CuDNN layers and dropout layers, and the training is done on a gpu equipped machine, and we tend to Deploy this model, are we required to deploy this model on a gpu equipped docker or what ever the container, or there is a way to deploy this on a lightweight cpu machine, or any other trick?
Thanks.
In general, Keras models can run on the CPU or GPU. I don’t know about CuDNN layers.
Hi jason ,
Excellent Post ,
Ran into an error .
Ive trained an Lstm for predicting r,g,b values . I have saved the model as json and weights as .h5 file . But when i load it again m, it gives me same accuracy but the predictions are awfully different . Im not sure where the issue is val accuracy is same before and after .
Interesting, I have not seen this issue.
Perhaps try posting on the Keras user group:
https://machinelearningmastery.com/get-help-with-keras/
Is it possible to combine multiple models? The cluster we have access to has multiple nodes, each with 2 GPUs per node. We’re thinking we could partition the dataset, run each subset on a node, save the models, then combine all saved models into one to make final predictions. Does that sound like the right idea to utilize more GPUs across multiple nodes?
Sounds like an ensemble, perhaps try the ensemble approach and compare to a single model. If it looks good, then double down.
json_file = open(‘DNN061061.json’, ‘r’)
loaded_model_json = json_file.read()
json_file.close()
DNN = model_from_json(loaded_model_json)
# load weights into new model
DNN.load_weights(“DNN061061.h5”)
print(“Loaded model from disk”)
# evaluate loaded model on test data
DNN.compile(loss=’categorical_crossentropy’, optimizer=’adam’, metrics=[‘accuracy’])
score = DNN.evaluate(X, Y, verbose=0)
print(“%s: %.2f%%” % (loaded_model.metrics_names[1], score[1]*100))
I saved model and load it to predict.
I found that the score is not the same. It’s decrease. Why?
That is surprising. Perhaps it is a bug? Try posting to the Keras user group or stackoverflow?
https://machinelearningmastery.com/get-help-with-keras/
hi.
Jason, thanks for your your sharing of post. its really helpul. i have a problem
i have a list in such format.
list=[1,2,3,[[3,4,5]]]
I want to convert it to a ndarray..
waiting for your reply.
Perhaps post programming questions to stackoverflow.
I built a model and performed fit function, then I saved this model as JSON file. when I load the saved model, the trained weights are loaded but if I check the weights in another jupyter notebook cell, I found the weights and biases return to their initial un-trained values?!!!! what is the problem?
The JSON only contains the structure. You must save the weights to an h5 file.
Hi Jason
After training and saving the loading the model how I can predict the output of single data input ? Effectively I need to know how to use the predict function on the trained model.
I show how to make a prediction here:
https://machinelearningmastery.com/how-to-make-classification-and-regression-predictions-for-deep-learning-models-in-keras/
I used the following to do prediction
print(X[5])
AA = np.array(X[5]) ;
AA.reshape(1, 8)
print(AA)
prediction = model.predict(AA)
print(prediction)
But I get this error
ValueError: Error when checking input: expected dense_1_input to have shape (8,) but got array with shape (1,)
The reshape method on an array does not reshape the array in place. You have to assign the returned value to a variable.
So just change:
AA.reshape(1, 8)
to
AA = AA.reshape(1, 8)
and it should work.
I believe your model expects a 1D array, not a 2D array.
Hi Jason, thanks a lot for your post.
May I ask how to store lstm model please? I tried using model.save(), load_model(). I got quite different results between direct forecast by using model after training and forecast by loading model.
It may be because LSTM model contains not only model and weight but also internal states. If there is a way to restore the internal state as well?
Thanks in advance.
Yes, use save() and load_model().
I expect internal state is cleared when the model is saved. If you need this state, you may be able to save it separately.
I’d recommend posting a question about this on the Keras user group:
https://machinelearningmastery.com/get-help-with-keras/
hi sir, thank you very much for you very useful articles and your responses.
after I am built my model,compile, fit it, I am serialized my model to JSON and weights to hdf5 as above. the model saved in json file, but the weights cannot be saved and i gets unreadable file with rabish and question marks and i gets the error message:
Saved model to disk
Exception ignored in: <bound method BaseSession.__del__ of >
Traceback (most recent call last):
File “C:\Users\SONY\PycharmProjects\multiclass_face_recognition\venv\lib\site-packages\tensorflow\python\client\session.py”, line 702, in __del__
File “C:\Users\SONY\PycharmProjects\multiclass_face_recognition\venv\lib\site-packages\tensorflow\python\framework\c_api_util.py”, line 31, in __init__
TypeError: ‘NoneType’ object is not callable
Process finished with exit code 0
can you please help me with this problem and i will be very grateful for that. Thanks a lot.
Perhaps try running the example from the command line and ensure your libraries are up to date?
OK, Thanks for your suggestion.
Hi Jason, I dont really get that line:
loaded_model = model_from_json(loaded_model_json)
Where does that model_from_json part come from? How should I define that?
Thank you.
It is a function that we import from the Keras library.
Hi Jason,
Thanks for amazing tutorial. I have a question why do you use different optimizers for initial compilation and for one after loading the model?
Thanks a lot!
No reason at all, sorry for the confusion.
Hi Jason,
I just wanted to create and load yolo3 model which builted on Keras,such as:
# serialize model to YAML
model_yaml = model.to_yaml()
with open(“model.yaml”, “w”) as yaml_file:
yaml_file.write(model_yaml)
model.save_weights(“model.h5”)
# load YAML and create model
yaml_file = open(‘model.yaml’, ‘r’)
loaded_model_yaml = yaml_file.read()
yaml_file.close()
loaded_model = model_from_yaml(loaded_model_yaml)
# load weights into new model
loaded_model.load_weights(“model.h5”)
but some errors occorred as follows:
Traceback (most recent call last):
File “D:/softwaree/MyPrgrams/VOCdefects/train.py”, line 291, in
yolo.runTrain()
File “D:/softwaree/MyPrgrams/VOCdefects/train.py”, line 270, in runTrain
loaded_model = model_from_yaml(loaded_model_yaml)
File “D:\softwares setup\anaconda3.5\lib\site-packages\keras\models.py”, line 330, in model_from_yaml
return layer_module.deserialize(config, custom_objects=custom_objects)
File “D:\softwares setup\anaconda3.5\lib\site-packages\keras\layers\__init__.py”, line 55, in deserialize
printable_module_name=’layer’)
File “D:\softwares setup\anaconda3.5\lib\site-packages\keras\utils\generic_utils.py”, line 140, in deserialize_keras_object
list(custom_objects.items())))
File “D:\softwares setup\anaconda3.5\lib\site-packages\keras\engine\topology.py”, line 2500, in from_config
process_node(layer, node_data)
File “D:\softwares setup\anaconda3.5\lib\site-packages\keras\engine\topology.py”, line 2459, in process_node
layer(input_tensors, **kwargs)
File “D:\softwares setup\anaconda3.5\lib\site-packages\keras\engine\topology.py”, line 603, in __call__
output = self.call(inputs, **kwargs)
File “D:\softwares setup\anaconda3.5\lib\site-packages\keras\layers\core.py”, line 651, in call
return self.function(inputs, **arguments)
File “D:\softwaree\MyPrgrams\VOCdefects\yolo3\model.py”, line 375, in yolo_loss
grid, raw_pred, pred_xy, pred_wh = yolo_head(yolo_outputs[l],
NameError: name ‘yolo_head’ is not defined.
I don’t know why the errors occorred since I could find ‘yolo_head’ be defined in model.py
Perhaps post your question to stackoverflow?
Hello Sir, I started Machine Learning and now dived into deep-learning
I created my own CNN using Keras and saved and loaded the model, which I’ve created.
Now, I don’t know how to use the loaded model for prediction…
can you explain the code for predicting the image
Perhaps this tutorial will help:
https://machinelearningmastery.com/how-to-make-classification-and-regression-predictions-for-deep-learning-models-in-keras/
great tutorial Sir
Thanks, I’nm glad it helped.
i am able to load the already trained model in keras. but can we increment the model on already trained model.
If get new data to model , its not suppose to start from scratch ,it has to start the training the model along with already trained model.
Please help me on this requirement.
I would to like train the new data on already trained model not suppose to start from scratch .
Yes, call fit() again with new data.
it will work nicely , it won’t start from scratch.
Hi Jason,
Thank you for this tutorial, it really helped me.
However there are a few things i don’t understand.
When first compiling the model you use ‘adam’ as the optimizer.
But when you load the saved model, you instead use RMSprop as the optimizer.
Why is this? Shouldn’t you be using the ‘adam’ optimizer again instead or doesn’t this matter to much?
I also saw you made a tutorial to serialize and save models with Pickle and Joblib.
Is there a ‘best option’ to save your models? When saving the models with Pickle or Joblib you don’t seem to recompile the models? Why is this? Is it because weights are saved seperate with json/yaml in the h5 file, and with Pickle/Joblib the weights are saved with the main model?
Thanks in advance for your time,
Jonas Boecquaert
Ideally the same optimizer would be used, sounds like a typo. Does not matter though, it’s not used.
You cannot use pickle for Keras models as far as I know.
Is it possible to save/load the whole model(weight and architecture) to json file ? or is there any possibility to save the whole model without using h5 file ?
No, weights are saved in h5. You can save the entire model to an h5 via model.save()
Ok. So can I convert this H5 file to other file extensions like .CSV or .mat ?
Thanks
I’m not sure that it is a direct mapping.
You could devise your own mapping, then load the h5 file, enumerate the layers and weights and output in any format you wish.
I don’t have a worked example, but it should be straightforward.
I have trained the rnn(lstm) model classification using keras ,after that i did model.predict_classes(X_test ) on X_test data i am getting accurate results, but it comes to model.loading from h5 file and configuration from json file in another file, i am getting randome predictions .
Please suggest me on this issue,why i am not getting correct predictions after loading from file.
Sorry, I have not seen this, it sounds like a bug. Perhaps use the method that works or report the bug to the Keras users group?
After using the model to predict new csv. file, how could I save the prediction result? Also, my prediction accuracy is so low? How to improve it?
See the documentation here:
http://pandas.pydata.org/pandas-docs/stable/indexing.html#ix-indexer-is-deprecated
(147725, 3, 14) (147725,)
Loaded model from disk
acc: 20.32%
thx.
The prediction will be a numpy array, you can save a numpy array using the save() function:
https://docs.scipy.org/doc/numpy/reference/generated/numpy.save.html
from tempfile import TemporaryFile
aa=[x for x in range(200)]
plt.plot(aa, inv_y[:200], marker=’.’, label=”actual”)
plt.plot(aa, inv_yhat[:200], ‘r’, label=”prediction”)
plt.ylabel(‘Global_active_power’, size=15)
plt.xlabel(‘Time step’, size=15)
plt.legend(fontsize=15)
plt.show()
model_yaml = model.to_yaml()
np.save(outfile, yaml_file)
with open(“/model.yaml”, “w”) as yaml_file:
yaml_file.write(model_yaml)
yaml_file.close()
# serialize weights to HDF5
model.save_weights(“/model.h5”)
print(“Saved model to disk”)
outfile = TemporaryFile()
like this???
what variable I should save??
look like we need to use model.model to call the function “to_json()”, can you please introduce which version you used?
The example works with Keras 2, for more on to_json(), see:
https://keras.io/models/about-keras-models/
Jason, can you give me a tip on how to save a keras model to pickle? I am utilizing the code in your post “Regression Tutorial with the Keras Deep Learning Library in Python” and the models are created thru functions like:
def larger_model():
not themodel.fit
method… Is there a way I can use the Keras API to save a pickle? ThanksI recommend saving models to H5, not pickle, I cannot help you with pickling models sorry.
Hi Jason, I trained a model in Ubuntu, saved the model:
loaded_model.load_weights("demandFinal.h5")
And then hoping to take that saved .h5 file to my windows 10 laptop running IPython anaconda 3.7 distribution to make predictions… Except I am running into some issues…
Do deep learning libraries like Tensorflow need to be installed on my Windows laptop in addition to Keras?
Or is there a different method to saving the model like YML or json for utilizing the model NOT on the machine that I created the model???
Thanks..
Yes, the backend (tenorflow) and the wrapper library (keras) will both have to be installed.
Hi Mr. Jason
I’m currently work on deep learning project for binary classification on tweet sentiment analysis, I had train my deep learning model and save its model and weights… the problem is that when im load model to make prediction why this model always give the same output for each test datasets. for examples : model always give outputs 0 for all test dataset which cause my accuracy is only around 47% – 50%
I had try several change on my datasets and model parameter like make balance dataset, change activation function, learning rate, number of epoch, batch size, simply model, but all of them doesn’t show the improve
So what should i do to solve this issue ?
Thanks..
I have some suggestions and systematic approaches to try here:
https://machinelearningmastery.com/start-here/#better
Wow nice that’s what i need..thanks Mr
Glad to hear it.
Thanks for all the info. I get directed here a lot.
Y_true and y_pred are tensors. How are they different than a list or a DataFrame? I’m working a binary classification problem where all models produce way too many false positives. I want to create a metric to maximize non-false-positives. So far I simply return
K.sum(y_true – y_pred, axis = )
and I get a float. I expect an integer. I get different results using axis 1 or 0 but don’t really know what axis I’m summing on.
Do all metrics try to maximize?
Perhaps start with an existing metric such as precision or recall, you can start here:
https://machinelearningmastery.com/how-to-calculate-precision-recall-f1-and-more-for-deep-learning-models/
You have no idea, how much I learnt from your blog……I am a mechanical engineer, started using machine learning out of passion. Everytime I search for something on machine learning.your blog will be one of the first three results (the other one being stackoverflow). you peel the banana and put it in our mouths.Awesome job boss!!!!
Thanks, I’m glad the tutorials are helpful!
Hi Mr. Jason
I want to ask: Can the model be reloaded and continue to be used for training? Is this based on previous data to continue to increase data training? In other words, you can use a lot of data to predict and increase the time. On the basis of the previous one, is this the case? thank you!
Yes. You can load a model later and continue training.
Hi Jason,
I’m building a Conv1D model to do multi label classification.
First I divide the dataset to 70% training, 20% testing and 10% validation.
As soon as the training is finished I conduct testing, and it gives an accuracy of 76%
But after saving the model using model.save(…) function to a .h5 file. And loading this file in a different session, the predictions are hopeless. Now it gives an accuracy around 25% on a different dataset.
I’m using the same pre-processing steps to clean the reviews.
Except a new tokenizer instance is used to fit_on_texts, texts_to_sequences and pad_sequences with the same maxlen as of the training tokenizer used for training.
If you have any suggestions for this difference in accuracy, please let me know.
Thanks,
Achira.
If you are working with text, you must also prepare the tokenizer in the same way on the same data, or save the tokenizer as well with the model.
Hi, Jason.
I think I found the issue. And yes you are correct.
The model saving and loading works alright as expected.
But the problem is with the tokenizing of the text data and converting the labels to categorical.
1) Yes, using the same tokenizer instance from the training setup works, but I’ve tried using pickle to save the tokenizer instance but that didn’t do any change. Code is as below for saving and loading. Or do you have any suggestions for saving the tokenizer ?
# saving
with open(‘tokenizer.pickle’, ‘wb’) as handle:
pickle.dump(tokenizer_train, handle, protocol=pickle.HIGHEST_PROTOCOL)
# load tokenizer
tokenizer = Tokenizer()
with open(‘tokenizer.pickle’, ‘rb’) as handle:
tokenizer = pickle.load(handle)
2) I’m using to_categorical to convert the labels (0 to 5) to one hot vectors. I’m using the below piece of code in the training session and testing session. But when testing, doing this gives me false accuracy but using the already converted values from the training session gives me the correct accuracy. Do you think we need to save this by any means as well ?
test_revs = pd.read_csv(‘hotel_reviews_test.csv’) # fetching the reviews from csv to a dataframe
test_revs.loc[:, ‘rating’] = test_revs[‘rating’].apply(points_to_class)
Y_test = to_categorical(test_revs[‘rating’], 5) # converting to one hot vectors with 5 as number of classes
I’m kind of stuck in this phase, I’d like if you can help 🙂
Thanks !
OMG you saved my week Steve thank you so much, it should be told that indexators are built randomly
Hi Jason, I have created a keras model cnn-sequential with 2 classes , now want to add 2 more classes to this model without retraining the model from scratch. May I know if you have any blog/code for this?
Great question!
Perhaps you can use all of the weights as a starting point, then add a new output layer. Then train just the output layer and perhaps fine tune the other layers.
sir can you tell me how i predict the data of mine through using .h5 file
Yes, you can follow this process:
https://machinelearningmastery.com/start-here/#process
hi how to save lstm network as numpy array? i need the trained model be in the numpy array format please help me
You cannot do this directly, sorry.
Instead, I would encourage you to save the model as an H5 file.
Which of the two approaches is the preferred way to save and load models?
1. Separate model structure and weights files
2. Single file for both
Single file for both.
Hi Jason,
I create my own keras metric called correlation_coefficient.
I successfully save the model, where a model.h5 file is created.
However, when I try to load the model, the model doesn’t see my custom metric and following error appear:
ValueError: Unknown metric function:correlation_coefficient
Yes, you must specify the custom_objects argument when loading the model with a dict that maps the name of the function to the actual function.
For example:
Hi Jason,
I have a sklearn model and keras sequential model, both the model I saved using base64 encode, (Keras_model.fit(X_train, y_train, batch_size = 100, epochs = 200) and randomForest.fit(X_train, y_train)),
I read Keras_model and randomForest as bytes by saving then encode to base64,
While reading both model for prediction in local script, it is working good,
but in Flask API randomForest working but Keras model saying
from tensorflow.core.framework.graph_pb2 import *
from google.protobuf import descriptor as _descriptor
ImportError: cannot import name ‘descriptor’ from ‘google.protobuf’ (unknown location)
Using python 3.7, Can you please help on this.
Sorry, I cannot help you with this issue, perhaps try posting the code and the fault to stackoverflow?
Hi Jason,
After loading weights and model from saved files, If I need to fine tune the model ,how to do it? Is is just a fit command with new dataset?
Correct, use the fit command with data and perhaps a lower learning rate.
Hi Jason,
my model output the weights in .h5 format after training i want to convert them to .weight format any idea how can i convert them or any idea how can i save my weights in .weight format while training
What is .weight format?
it is a file containing weigth get from darknet framework
Say, total epoch no is 200, but it takes too long, so I first want to train for 50 epochs, then restart another training and so on, but in all training phases, the training data is the whole same data. In this case, will the last method (weight and architecture together) work? Will it be same if I were to train for all 200 epochs together rather than 50 epochs*4 phases?
Yes, 4 runs of 50 epochs on the same model is the same as 1 run of 200 epochs if the learning rate is constant.
How to load the model from json file?
As follows:
Hello Jason,
Thank you a lot for sharing your knowledge and expertise !
I am using an LSTM Keras model with 2 outputs (i.e predictions) for Slot and Intent filling. When I save, then load it (in json, yaml and single file format) , it provides random results. It seems like if the model does not fit well the loaded weights: The model has 84% of accuracy before saving, 20% after loading.
I don’t understand what is wrong.. Thanks in advance for your help.
This is my code:
—— code——
inputs = Input(shape=(20, 300))
x = LSTM(64, return_sequences=True)(inputs)
x = Dropout(0.3)(x)
a = LSTM(64, return_sequences=True)(x)
a = Dropout(0.3)(a)
b = LSTM(64, return_sequences=False)(x)
b = Dropout(0.3)(b)
main_output = Dense(57, activation=’softmax’, name=’main_output’)(a)
auxiliary_output = Dense(12, activation=’softmax’, name=’aux_output’)(b)
model = Model(inputs=inputs, outputs=[main_output, auxiliary_output])
That is surprising.
Are you evaluating the model on the same data before and after?
Are you saving and loading weights as well as structure?
Hi Jason Brownlee
Thank you so much for leaving a good tutorial
I have a question, are you okay?
First of all, here’s the HDF file,
If we think we have collected new data (the classification is the same).
Then when I loaded that model and applied predict to the new data
Is the value we get correct?
I’m struggling with this issue.
Should we retrain when new data comes in?
Thanks!
You can decide to re-train or not-retrain the model when you get new data.
It only makes sense to retrain if you can confirm that re-training will make the model better for the future. Perhaps test the idea for your specific dataset/domain.
Thank you for sharing your great experiences of machine learning.
I have a question with load and use pretrained model by kereas, tensorflow.
It’s a .h5 to load and use, but it takes half hour to get the result in my laptop. But in other desktop, it can get result in 1 second! Do you know what’s wrong with my laptop or what should I modify settings to run it?
Thanks.
That’s odd.
Maybe the laptop does not have enough RAM or sufficient CPU?
That’s also my guess, but my laptop has better RAM and CPU. I also check for different computers. I found that in the virtual machine, conda, and virtualenv these program ran about 30 mins to get result. I tried to install tensorflow by pip3 commands in another computer, it can get result in 1-2 seconds. According to your experience, is it possible that using pip3 to install packages and run it in terminal directly would be faster than in virtual environment or PyCharm or Anaconda Spyder?
Thanks!
I recommend always using the command line. Many readers end up having problems with notebooks and IDEs.
Hi Jason,
I am using python 3 and spyder.
I am trying to use VGG 19 for my application but I am not using Keras
I am using PyTorch.
But the h5py file is not getting downloaded bcoz of connection resetting.
I want to download and save the weights.
Can you tell me where to save these weights and how to run the program.
Thanks in advance.
I get the following stacktrace:
runfile(‘C:/Users/CoE10/Anaconda3/Lib/TST/TransferLearning_VGG_UCUM.py’, wdir=’C:/Users/CoE10/Anaconda3/Lib/TST’)
Downloading: “https://download.pytorch.org/models/alexnet-owt-4df8aa71.pth” to C:\Users\CoE10/.cache\torch\checkpoints\alexnet-owt-4df8aa71.pth
15.0%
Traceback (most recent call last):
File “”, line 1, in
runfile(‘C:/Users/CoE10/Anaconda3/Lib/TST/TransferLearning_VGG_UCUM.py’, wdir=’C:/Users/CoE10/Anaconda3/Lib/TST’)
File “C:\Users\CoE10\Anaconda3\envs\tarakeras\lib\site-packages\spyder_kernels\customize\spydercustomize.py”, line 827, in runfile
execfile(filename, namespace)
File “C:\Users\CoE10\Anaconda3\envs\tarakeras\lib\site-packages\spyder_kernels\customize\spydercustomize.py”, line 110, in execfile
exec(compile(f.read(), filename, ‘exec’), namespace)
File “C:/Users/CoE10/Anaconda3/Lib/TST/TransferLearning_VGG_UCUM.py”, line 43, in
model=models.alexnet(pretrained=True)
File “C:\Users\CoE10\Anaconda3\envs\tarakeras\lib\site-packages\torchvision\models\alexnet.py”, line 63, in alexnet
progress=progress)
File “C:\Users\CoE10\Anaconda3\envs\tarakeras\lib\site-packages\torch\hub.py”, line 485, in load_state_dict_from_url
download_url_to_file(url, cached_file, hash_prefix, progress=progress)
File “C:\Users\CoE10\Anaconda3\envs\tarakeras\lib\site-packages\torch\hub.py”, line 406, in download_url_to_file
buffer = u.read(8192)
File “C:\Users\CoE10\Anaconda3\envs\tarakeras\lib\http\client.py”, line 457, in read
n = self.readinto(b)
File “C:\Users\CoE10\Anaconda3\envs\tarakeras\lib\http\client.py”, line 501, in readinto
n = self.fp.readinto(b)
File “C:\Users\CoE10\Anaconda3\envs\tarakeras\lib\socket.py”, line 589, in readinto
return self._sock.recv_into(b)
File “C:\Users\CoE10\Anaconda3\envs\tarakeras\lib\ssl.py”, line 1071, in recv_into
return self.read(nbytes, buffer)
File “C:\Users\CoE10\Anaconda3\envs\tarakeras\lib\ssl.py”, line 929, in read
return self._sslobj.read(len, buffer)
ConnectionResetError: [WinError 10054] An existing connection was forcibly closed by the remote host
Sorry, I don’t have tutorials on Pytoch, perhaps try posting on stackoverflow?
why do i keep getting this exception:
JSONDecodeError: Expecting value: line 1 column 1 (char 0)
when trying to run this
loaded_model=model_from_json(loaded_model_json)
Sorry to hear that, I have some suggestions here:
https://machinelearningmastery.com/faq/single-faq/why-does-the-code-in-the-tutorial-not-work-for-me
Hello,
I believe that you are the most qualified person to ask the following question: I do not want to merely save the model, I actually want to create a function to predict the values, and use it in maybe another language, i.e. I want to use the weights and architecture of the NN to write down a simple function. Is this possible?
Thank you for your excellent work, it was most helpful!
Yes.
You will need to load the model weights in your program and perform the forward pass operation in an identical manner as the Keras code – often trivial for MLP networks, harder for others.
Hi Jason,
actually i have taken 6 classes of images for image classification.but in output it shows me 7 classes.i don’t know why?how can i resolved it.plz help me.
Thank you.
Perhaps double check that your data was loaded as you expected.
i have taken 6 classes but in my output it returns 7 classes.how can i resolved my problem.
my code is
my_model.compile(optimizer=’adam’, loss=’binary_crossentropy’,
metrics=[‘accuracy’])
from tensorflow.python.keras.applications.vgg16 import preprocess_input
from tensorflow.python.keras.preprocessing.image import ImageDataGenerator
# set up data generator
data_generator = ImageDataGenerator(preprocessing_function=preprocess_input)
# get batches of training images from the directory
#train_generator = data_generator.flow_from_directory(
# ‘data/train’,
# target_size=(178, 218),
# batch_size=12,
# class_mode=’categorical’)
# get batches of validation images from the directory
validation_generator = data_generator.flow_from_directory(
‘data/test’,
target_size=(178, 218),
batch_size=12,
class_mode=’categorical’)
history = my_model.fit_generator(
validation_generator,
epochs=10,
steps_per_epoch=500,
validation_data=validation_generator,
validation_steps=667, callbacks=cb_list)
####### Testing ################################
# load a saved model
from keras.models import load_model
import os
os.chdir(‘data\\data4\\’)
saved_model = load_model(‘data’)
# generate data for test set of images
test_generator = data_generator.flow_from_directory(
‘data/test’,
target_size=(178, 218),
batch_size=1,
class_mode=’categorical’,
shuffle=False)
# obtain predicted activation values for the last dense layer
import numpy as np
test_generator.reset()
pred=saved_model.predict_generator(test_generator, verbose=1, steps=1000)
# determine the maximum activation value for each sample
predicted_class_indices=np.argmax(pred,axis=1)
# label each predicted value to correct class
labels = (test_generator.class_indices)
labels = dict((v,k) for k,v in labels.items())
predictions = [labels[k] for k in predicted_class_indices]
# format file names to simply which type
filenames=test_generator.filenames
filenz=[0]
for i in range(0,len(filenames)):
filenz.append(filenames[i].split(‘\\’)[0])
filenz=filenz[1:]
# determine the test set accuracy
match=[]
for i in range(0,len(filenames)):
match.append(filenz[i]==predictions[i])
match.count(True)/1000
output
Found 840 images belonging to 7 classes.
Epoch 1/10
500/500 [==============================] – 499s 997ms/step – loss: 0.4227 – acc: 0.8276 – val_loss: 0.3815 – val_acc: 0.8548
Epoch 00001: val_loss improved from inf to 0.38155, saving model to data/test.h5
Epoch 2/10
500/500 [==============================] – 656s 1s/step – loss: 0.3420 – acc: 0.8580 – val_loss: 10.1089 – val_acc: 0.2443
Epoch 00002: val_loss did not improve from 0.38155
Epoch 3/10
449/500 [=========================>….] – ETA: 4:26 – loss: 0.3308 – acc: 0.8616
please help me how can i get the correct class numbers(6)
Perhaps try posting your code and question to stackoverflow?
hi sir I am gating this Error while importing the file
please help me sir
>>> from keras.models import load_model
Using TensorFlow backend.
>>> model = load_model(‘models/_mini_XCEPTION.106-0.65.hdf5′)
Traceback (most recent call last):
File “”, line 1, in
File “C:\Users\abc\.conda\envs\tensorflow_env\lib\site-packages\keras\engine\saving.py”, line 419, in load_model
model = _deserialize_model(f, custom_objects, compile)
File “C:\Users\abc\.conda\envs\tensorflow_env\lib\site-packages\keras\engine\saving.py”, line 225, in _deserialize_model
model = model_from_config(model_config, custom_objects=custom_objects)
File “C:\Users\abc\.conda\envs\tensorflow_env\lib\site-packages\keras\engine\saving.py”, line 458, in model_from_config
return deserialize(config, custom_objects=custom_objects)
File “C:\Users\abc\.conda\envs\tensorflow_env\lib\site-packages\keras\layers\__init__.py”, line 55, in deserialize
printable_module_name=’layer’)
File “C:\Users\abc\.conda\envs\tensorflow_env\lib\site-packages\keras\utils\generic_utils.py”, line 145, in deserialize_keras_object
list(custom_objects.items())))
File “C:\Users\abc\.conda\envs\tensorflow_env\lib\site-packages\keras\engine\network.py”, line 1022, in from_config
process_layer(layer_data)
File “C:\Users\abc\.conda\envs\tensorflow_env\lib\site-packages\keras\engine\network.py”, line 1008, in process_layer
custom_objects=custom_objects)
File “C:\Users\abc\.conda\envs\tensorflow_env\lib\site-packages\keras\layers\__init__.py”, line 55, in deserialize
printable_module_name=’layer’)
File “C:\Users\abc\.conda\envs\tensorflow_env\lib\site-packages\keras\utils\generic_utils.py”, line 147, in deserialize_keras_object
return cls.from_config(config[‘config’])
File “C:\Users\abc\.conda\envs\tensorflow_env\lib\site-packages\keras\engine\base_layer.py”, line 1109, in from_config
return cls(**config)
File “C:\Users\abc\.conda\envs\tensorflow_env\lib\site-packages\keras\legacy\interfaces.py”, line 91, in wrapper
return func(*args, **kwargs)
File “C:\Users\abc\.conda\envs\tensorflow_env\lib\site-packages\keras\engine\input_layer.py”, line 87, in __init__
name=self.name)
File “C:\Users\abc\.conda\envs\tensorflow_env\lib\site-packages\keras\backend\tensorflow_backend.py”, line 517, in placeholder
x = tf.placeholder(dtype, shape=shape, name=name)
AttributeError: module ‘tensorflow’ has no attribute ‘placeholder’
>>>
Try updating to tensorflow 2 and keras 2.3.
I have a large number of features. I train several keras models by varying the number of features to see the impact of these features on model accuracy. In the end, when I load these models, I no longer know on which features the model was trained on so when I do the predictions, I get the error like:
Error when checking input: expected dense_4_input to have shape (29,) but got array with shape (47,)
Is there a way to know what are the exact feature names on which the model was trained? I use keras.models.load_model to load the model and keras.models.sequence.save(path) to save the model
Yes, you can access the first layer of the model and access it’s shape.
from keras.models import model_from_json add this to use
loaded_model = model_from_json(loaded_model_json)
Thanks.
Hi Jason, love your tutorials (and bought the books)!
I am stuck with loading a model that has prelu as acitvation. I get the ValueError. I tried with
model = load_model(‘model.h4’, custom_objects={‘…’:…}), however I did not manage to correctly define the custom_object. Do you know how to load PReLu?
Thank you so much!
Not off hand, sorry. Perhaps try posting to stackoverflow?
This worked:
-explicitly load this as a layer (from tensorflow.keras.layers import PReLU)
-then load it as custom_objects={‘PReLU’: PReLU}
Nice work!
hi there
I was following an example that in first step made this class as neural net:
{class Net(nn.Module):
def __init__(self):
# Define all the parameters of the net
super(Net, self).__init__()
self.fc1 = nn.Linear(28 * 28 * 1, 200)
self.fc2 = nn.Linear(200,10)
def forward(self, x):
# Do the forward pass
x = F.relu(self.fc1(x))
x = self.fc2(x)
return x
}
and in the next step use it and call it as “model” in this line of code:
{optimizer = optim.Adam(model.parameters(), lr=3e-4)
}
when I run the entire code it fail because this error: model is not defined or parameters() missing 1 required positional argument: ‘self’
whould you help me please?
Your example looks like pytorch, whereas this post is about Keras. Perhaps try posting your question to stackoverflow?
Hi Jason,
I save model in H5 format, my problem is that after loading saved model, using same dataset and same data augmentation choices,batch size etc. In new training, loss value doesn’t continue from saved model last epoch’s value, it starts from beginning like not trained before..
What is wrong with this case?
sample code example is given Let’s say saved model is Model-A.h5:
trained_model=keras.models.load_model(‘Model-A.h5’)
train_datagen = ImageDataGenerator(same things in pretrained model)
validation_datagen = ImageDataGenerator(same things in pretrained model)
train_generator = train_datagen.flow_from_directory(‘datafile’,
target_size=(image_size, image_size),
batch_size=train_batchsize,
class_mode=’binary’,
shuffle=True)
validation_generator = validation_datagen.flow_from_directory(…….)
trained_model.fit_generator(
train_generator,
steps_per_epoch=train_generator.samples/train_generator.batch_size ,
epochs=…,
validation_data=validation_generator,
validation_steps=validation_generator.samples/validation_generator.batch_size)
After you load the model, you must use a smaller learning rate to avoid “washing away” the weights that you started with.
Thank you Jason!
Dear Jason,
when I load model using H5 file, model was compiled before using a learning rate.
After loading, we are not using model.compile() again, right?
so how can I add a smaller learning rate in code?
thank you
You can re-set the learning rate and compile again.
See this for setting the learning rate:
https://machinelearningmastery.com/understand-the-dynamics-of-learning-rate-on-deep-learning-neural-networks/
You’re welcome.
Hi Jason,
Thanks a million for this blog.
I have problem when loading the model again. It does look that there is not problem with saving it, but when I load it again I run into the following error :
—————————————————————————
ValueError Traceback (most recent call last)
in
4 from keras.models import load_model
5
—-> 6 model_24=load_model(“dove_model_24.h5”)
~\Anaconda3\lib\site-packages\keras\engine\saving.py in load_wrapper(*args, **kwargs)
490 os.remove(tmp_filepath)
491 return res
–> 492 return load_function(*args, **kwargs)
493
494 return load_wrapper
~\Anaconda3\lib\site-packages\keras\engine\saving.py in load_model(filepath, custom_objects, compile)
582 if H5Dict.is_supported_type(filepath):
583 with H5Dict(filepath, mode=’r’) as h5dict:
–> 584 model = _deserialize_model(h5dict, custom_objects, compile)
585 elif hasattr(filepath, ‘write’) and callable(filepath.write):
586 def load_function(h5file):
~\Anaconda3\lib\site-packages\keras\engine\saving.py in _deserialize_model(h5dict, custom_objects, compile)
268 return obj
269
–> 270 model_config = h5dict[‘model_config’]
271 if model_config is None:
272 raise ValueError(‘No model found in config.’)
~\Anaconda3\lib\site-packages\keras\utils\io_utils.py in __getitem__(self, attr)
316 else:
317 if self.read_only:
–> 318 raise ValueError(‘Cannot create group in read-only mode.’)
319 val = H5Dict(self.data.create_group(attr))
320 return val
ValueError: Cannot create group in read-only mode.
I wonder what would be the solution or how can I avoid this,
Regards,
Mohammed
Sorry, I don’t know the cause, perhaps try posting your code and error to stackoverflow.
Hey, I hope you are doing well. Did you find a solution? I am having the same issue.
Regards
Thank you – Well explaned
You’re welcome.
hi Jason. I want to ask you why everytime the coding is running, the result will be changed?Really need an explanation about it because the output will be not consistent
This is to be expected, you can learn more here:
https://machinelearningmastery.com/faq/single-faq/why-do-i-get-different-results-each-time-i-run-the-code
Hi, Jason
Awesome Tutorial , however i have one doubt , the weights you are saving in this tutorial are in .h5 format.
However if you want to save them in “.mat” format , how to save and load the weights?
I don’t know about .mat format, sorry.
Hi Jason, Thanks for the tutorial.
I managed to use save and load model to build on my training for my model with different batches of data. previously was not able to leverage on GPU due to the size.
Basically I randomize the indexes of data before I split them into train and validation data.
In every training iteration, I take a slice of training data and go through 15 epoch. In all training iterations, I validated against the same valid data.
The accuracy actually went down instead when I do this. I am wondering if I take this approach, should the validation data be the same throughout?
Not sure I follow.
Generally, test and validation data must not be used in the training of the model to give an unbiased evaluation of the model.
Hi Jason, that’s what I have done. Firstly, I have split the data into train and validation.
Within training data samples, I break them up into chunks and use them to train the model chunk by chunk due to the size.
But for every training, I used the same validation samples. Was wondering since I cut the train data into chunks, does it make sense that the validation set remains 25% of total dataset size?
Perhaps, as long as the validation set is representative of the broader problem.
Looking at the validation set too much (trying to optimize results on it) will lead to overfitting.
Hi,
is there a tutorial to freeze a keras model?
Hmmm, I guess I demonstrate freezing layers in many computer vision and gan tutorials.
Perhaps try a search on the blog.
Hi Mr. Jason,
Thanks for all the materials you have made available free of charge online.
My first deep learning code works perfectly, I was able to tune it up to %80.20 accuracy by setting the ephoc to 200.
It was after the above results that began to think about catching the model and loading it later to make predictions. After making some search on Google I was directed back to this site.
I have tried out the example and it’s working perfectly.
Thanks once again.
You’re welcome.
Well done!
Hi, thank you for the article. I’ve used the following code: keras.models.load_model(“modelS.h5”) but I get this error: ValueError: Unknown layer: KerasLayer. Do you know how to fix this by any chance?
I have not seen this error, perhaps try posting your code and error on stackoverflow.
Hello,Jason,thanks for sharing.
What should I do when I want to train the model with several files?
Thank you
Load the files into memory, combine them into a dataset, then train a model on them.
hello jason . . .
i m working on credit card fraud detection projet. . . i have the model in .h5 format but i dont understand what to do next with that .h5 format model means i dont understand how to deploy that model on web . . basically i want to detect a transaction is fraudulent or not by taking an input on the webpage but i dont know how to deploy that model.h5 model on that webpage. . .can you please just tell me the further steps n the required tools . .
Load the model in your custom code and call it with data from your application.
Using a model in a webpage is more of an engineering question, not a machine learning question.
I have saved by model by model.save() function , while loading i am using keras.models.load_model(cnnmodel_path). It is working fine on my machine but when i tried to execute on Amazon ecs instance, it got an exception “Cannot create group in read only mode.”..
That is an odd error, perhaps try searching/posting on stackoverflow?
Hi, I was working with ner and following the code in the following link-
https://www.depends-on-the-definition.com/named-entity-recognition-with-residual-lstm-and-elmo/
But, I was unable to save the model and then load it. Can you help me with this? I am really new to ML and these topics.
Perhaps contact the author directly.
can we continue/transfer training with new dataset if we have the description of the model in json file and weights?
Sure, load it and continue training.
ValueError Traceback (most recent call last)
in
1
2 from keras.models import load_model
—-> 3 model = load_model(‘mnist_mlp_model.h8’)
4
5 yhat = model_predict(model, history, cfg)
C:\ProgramData\Anaconda3\lib\site-packages\keras\engine\saving.py in load_model(filepath, custom_objects, compile)
262
263 # set weights
–> 264 load_weights_from_hdf5_group(f[‘model_weights’], model.layers)
265
266 if compile:
C:\ProgramData\Anaconda3\lib\site-packages\keras\engine\saving.py in load_weights_from_hdf5_group(f, layers, reshape)
899 ‘containing ‘ + str(len(layer_names)) +
900 ‘ layers into a model with ‘ +
–> 901 str(len(filtered_layers)) + ‘ layers.’)
902
903 # We batch weight value assignments in a single backend call
ValueError: You are trying to load a weight file containing 6 layers into a model with 0 layers.
Hello my name is kimhun
I am having a trouble like this;
when i load the saved model -> model = load_model(‘mnist_mlp_model.h8’)
but it occured this problem
how can i do to solve it?
Perhaps confirm that the model was saved correctly? E.g. try saving again and loading afresh?
Perhaps ensure your libraries are up to date.
Quando tento carregar a base ele dá o seguinte erro:
ValueError: could not convert string to float: ‘\ufeff6,148,72,35,0,33.6,0.627,50,1’
Eu baixei a base do link que você colocou no tutorial. Salvo ela em csv mas dá esse erro.
I’m sorry to hear that, perhaps try downloading the dataset again?
Perhaps some of the suggestions here will help:
https://machinelearningmastery.com/faq/single-faq/why-does-the-code-in-the-tutorial-not-work-for-me
The following line of code isn’t working.
loaded_model = model_from_yaml(loaded_model_yaml)
ConstructorError: could not determine a constructor for the tag ‘tag:yaml.org,2002:python/object/apply:tensorflow.python.framework.tensor_shape.TensorShape’
in “”, line 4, column 22:
build_input_shape: !!python/object/apply:tensorflow …
^
Please note: I do have PyYAML(version>=5) installed.
I’m sorry to hear that you’re having trouble, here are some suggestions:
https://machinelearningmastery.com/faq/single-faq/why-does-the-code-in-the-tutorial-not-work-for-me
Hi Jason, How to save weights for keras subclassed model as single file, it saves as folder
model.save_weights('./model_weights', save_format='tf')
the subclassed model can’t be stored as .h5 format
Thanks
Why not? I have not tried myself, I’m just curious.
Perhaps you can retrieve the weight matrix and save as a binary file manually?
Hi, great post by the way, but what would you do if you had k-fold validation. I have weights saved for each fold but I do not know how to average them into one .h5 file for the weights. How would I average several weights together?
Discard all models from the cross-validation process. They are only used to estimate model performance.
Then train a final model on all available data. This may help:
https://machinelearningmastery.com/how-to-make-classification-and-regression-predictions-for-deep-learning-models-in-keras/
Nice tutorial .
I have a for loop with 3 repetitions and each time i train a model with a different dataset but same architecture structure . My code is :
# Build model ….
epochs = 80
batch_size = 32
# Configure the model
model.compile(loss=”categorical_crossentropy”, optimizer=”adam”, metrics=[“accuracy”])
# Checkpoint – Create callback_list according min_validation_loss
checkpoint = ModelCheckpoint(‘best_model.h5′, monitor=’val_loss’, verbose=1,
save_best_only=True, save_weights_only=False, mode=’min’)
# TensorBoard for plotting metrics
tensorboard = TensorBoard(log_dir=log_directory)
# Train model
history = model.fit(xtrain, ytrain, batch_size=batch_size, epochs=epochs,
validation_split=0.2, verbose=0, callbacks=[checkpoint, tensorboard])
# load the saved model
saved_model = load_model(‘best_model.h5’)
My codes runs and crashes when it tries to train the third model with error :
InternalError: Failed copying input tensor from /job:localhost/replica:0/task:0/device:CPU:0 to /job:localhost/replica:0/task:0/device:GPU:0 in order to run GatherV2: Dst tensor is not initialized. [Op:GatherV2]
My question is :
Do I keep in memory all the 3 models ? If yes , is there a way to “clear the memory” just before the next iteration starts ?
Perhaps try removing the tensorboard?
hi Jason,
Thank you for this helpful tutorial,
In fact I have an error while saving the h5 file
ErrorCode: a bytes-like object is required, not ‘str’
can you help me with that?
Thank you
Sorry to har that you’re having trouble, perhaps these tips will help:
https://machinelearningmastery.com/faq/single-faq/why-does-the-code-in-the-tutorial-not-work-for-me
I am getting the exact same error, did you figure out a solution ?
I had just done this:
model.save(modelSavePath, save_format=’h5′)
model = tf.keras.models.load_model(modelLoadPath)
The last few error lines are as follows,
File “/usr/local/lib/python3.8/site-packages/tensorflow/python/ops/init_ops_v2.py”, line 545, in __call__
fan_in, fan_out = _compute_fans(scale_shape)
File “/usr/local/lib/python3.8/site-packages/tensorflow/python/ops/init_ops.py”, line 1425, in _compute_fans
return int(fan_in), int(fan_out)
TypeError: int() argument must be a string, a bytes-like object or a number, not ‘NoneType’
Some ideas:
Perhaps use the Keras API directly as demonstrated in the tutorial?
Perhaps use Python 3.6, Id on’t 3.8 is supported?
Hi Jason,
Many thanks for your good tutorials.
I have built a CNN model + Google embeddings, measured the accuracy (i.e. precision:0.7) based on your tutorials but on my own data for binary classes. then saved the model and load it and used the same dataset to be labelled by the model. I compared results manually and gained precision:0.15!
is there any reason for such gap?
Thank you
You’re welcome.
Once a model is trained it should/will give the same performance on the same dataset.
Perhaps check for bugs in your test harness and ensure you are not re-training the model each time it is loaded.
Hi Jason,
Thanks for the amazing contents.
I have trained a siamese network and tried to save and load using mentioned methods in this post even with the presence of the lambda layer I used but I keep on getting errors “SystemError: unknown opcode”.
I have tried training and loading the model with TensorFlow 2.3 but still getting the same error
Sometimes, when saving a model with custom layers, you must import and specify the names of the custom layers when loading the model.
Specifically, via the “custom_objects” argument to the function:
https://www.tensorflow.org/api_docs/python/tf/keras/models/load_model
Dear Jason,
Thanks for a quick response. I have managed to find a workaround for this. Kindly see my GitHub comment here
https://github.com/keras-team/keras/issues/9595#issuecomment-742413448
I’m happy to hear you have solved your problem.
Dear Jason,
I really appreciate your amazing content;
I found some difficulties to load my trained tflearn model.
I saved my model in this way: model.save(‘model.tflearn’) and then, I tried to load it in a new notebook, first I build the model and I define it so that I can assign the model variable to model.load(‘model.tflearn’) but I got a serie of errors which holding me back to load it.
I look forward to your reply,
Thanks!
Sorry to hear that, perhaps try the code without a notebook.
Thanks for these wonderful tutorials Jason!
It really helped me to dive into ML without a hassle!
You’re very welcome!
Hi Jason,
Thank you for your post. How we can improve the load_model time? My model is small. Also, how we can use model weights to predict new image?
You’re welcome.
Perhaps use a smaller model?
Perhaps faster HDD?
Thank you for your reply. I have used a very shallow version of densenet which has only 0.5M parameters and the size of file is only 7 MB.
Nice!
How do I save and load weights for: CNN_LSTM multivariate multi step time series model – run 30 times and boxplot used for forecast?
Good question, this tutorial shows you how to save and load models:
https://machinelearningmastery.com/save-load-keras-deep-learning-models/
@Jason, what is the best way to save all the 30 weights – 30 runs of the model.
(1) run in loop and save in loop.
(2) is there a way to create the model function outside the loop and there by save all the 30 runs and their weights in one go.
Why would you do that?
Yes, calling save() within the loop with a unique filename.
thanks for these tutorials they are really helpful . my issue is if i run the tutorials on using different IDEs i get very different results for example if i run the tutorial on serialization using pycharm i get an accuracy of about 37% And running the same code using spyder -ide i get an accuracy of about 78% what might be causing this. i would expect the values to be close to each other as its exactly the same code.
This is a common question that I answer here:
https://machinelearningmastery.com/faq/single-faq/why-do-i-get-different-results-each-time-i-run-the-code
one question Sir.If i save the weights of my model in a .h5 file, how can find this file in my disk.where is it saved in my pc?I use jupyter notebook of google collab to execute the training simulations
You must specify the path location on system where to save.
If you don’t specify a path, it will save in the same location as your python file.
I don’t recommend using notebooks:
https://machinelearningmastery.com/faq/single-faq/why-dont-use-or-recommend-notebooks
Hi jason,
I have one small query … suppose we train yolo model for class A (e.g pen) .. . we get the weight file and using this weight file we train model again for class B (eg. Pencil) … then the result weight file will able to detect both class A and Class B objects.
Eg. If we use a image for both pen and pencli ..will it able to identify them both ?
Perhaps try it and see.
Hi
I have below installed version
Python –3.8.5
tensorflow — 2.4.1
Keras –2.4.0
I am trying to load YOLO model, but getting error like
D:\Anaconda3\python.exe D:/Python_project/PY_worrks/AI-work/Keras_module/Autonomous_driving_application_Car_detection/Autonomous_driving_application_Car_detection.py
2021-05-03 11:54:11.870644: W tensorflow/stream_executor/platform/default/dso_loader.cc:60] Could not load dynamic library ‘cudart64_110.dll’; dlerror: cudart64_110.dll not found
2021-05-03 11:54:11.871551: I tensorflow/stream_executor/cuda/cudart_stub.cc:29] Ignore above cudart dlerror if you do not have a GPU set up on your machine.
Traceback (most recent call last):
File “D:/Python_project/PY_worrks/AI-work/Keras_module/Autonomous_driving_application_Car_detection/Autonomous_driving_application_Car_detection.py”, line 227, in
yolo_model = load_model(“yolo.h5”)
File “D:\Anaconda3\lib\site-packages\tensorflow\python\keras\saving\save.py”, line 206, in load_model
return hdf5_format.load_model_from_hdf5(filepath, custom_objects,
File “D:\Anaconda3\lib\site-packages\tensorflow\python\keras\saving\hdf5_format.py”, line 183, in load_model_from_hdf5
model = model_config_lib.model_from_config(model_config,
File “D:\Anaconda3\lib\site-packages\tensorflow\python\keras\saving\model_config.py”, line 64, in model_from_config
return deserialize(config, custom_objects=custom_objects)
File “D:\Anaconda3\lib\site-packages\tensorflow\python\keras\layers\serialization.py”, line 173, in deserialize
return generic_utils.deserialize_keras_object(
File “D:\Anaconda3\lib\site-packages\tensorflow\python\keras\utils\generic_utils.py”, line 354, in deserialize_keras_object
return cls.from_config(
File “D:\Anaconda3\lib\site-packages\tensorflow\python\keras\engine\training.py”, line 2261, in from_config
return functional.Functional.from_config(
File “D:\Anaconda3\lib\site-packages\tensorflow\python\keras\engine\functional.py”, line 668, in from_config
input_tensors, output_tensors, created_layers = reconstruct_from_config(
File “D:\Anaconda3\lib\site-packages\tensorflow\python\keras\engine\functional.py”, line 1275, in reconstruct_from_config
process_layer(layer_data)
File “D:\Anaconda3\lib\site-packages\tensorflow\python\keras\engine\functional.py”, line 1257, in process_layer
layer = deserialize_layer(layer_data, custom_objects=custom_objects)
File “D:\Anaconda3\lib\site-packages\tensorflow\python\keras\layers\serialization.py”, line 173, in deserialize
return generic_utils.deserialize_keras_object(
File “D:\Anaconda3\lib\site-packages\tensorflow\python\keras\utils\generic_utils.py”, line 354, in deserialize_keras_object
return cls.from_config(
File “D:\Anaconda3\lib\site-packages\tensorflow\python\keras\layers\core.py”, line 1019, in from_config
function = cls._parse_function_from_config(
File “D:\Anaconda3\lib\site-packages\tensorflow\python\keras\layers\core.py”, line 1071, in _parse_function_from_config
function = generic_utils.func_load(
File “D:\Anaconda3\lib\site-packages\tensorflow\python\keras\utils\generic_utils.py”, line 457, in func_load
code = marshal.loads(raw_code)
ValueError: bad marshal data (unknown type code)
Process finished with exit code 1
Sorry to hear that, perhaps some of these tips will help:
https://machinelearningmastery.com/faq/single-faq/why-does-the-code-in-the-tutorial-not-work-for-me
Hi jason, excellent lecture. I am working on GAN and trained my generator and stored the architecture in JSON file and weights in .h5 file. During testing, I have loaded the json architecture and H5 files. Now you have mentioned the compile step as below:
loaded_model.compile(loss=’binary_crossentropy’, optimizer=’rmsprop’, metrics=[‘accuracy’])
Similarly, to compile my generator model, how to compile?, which loss function to use during compilation, bec the generator is trained with reconstruction loss, etc and doesnt have compile phase in training, please suggest.
Thanks!
No need to compile any longer, just load and start using.
Dear Jason as per your tutorial i made and saved my entire code as follows,
import pandas as pd
import numpy as np
from sklearn.model_selection import train_test_split
df=pd.read_csv(‘Al-Besharah Data.csv’)
df.head()
X=df.iloc[:,:-1]
Y=df.iloc[:,-1].values
from sklearn.preprocessing import MinMaxScaler
scaler = MinMaxScaler()
Xnorm = pd.DataFrame(data = scaler.fit_transform(X))
Yshape = pd.DataFrame(data = Y.reshape(-1,1))
Ynorm = pd.DataFrame(data = scaler.fit_transform(Yshape))
XTrain,XTest,YTrain,YTest=train_test_split(Xnorm,Ynorm,train_size=0.7,random_state=2)
import keras.backend as K
def r2(y_true, y_pred):
SS_res = K.sum(K.square(y_true – y_pred))
SS_tot = K.sum(K.square(y_true – K.mean(y_true)))
return ( 1 – SS_res/(SS_tot + K.epsilon()) )
from keras.models import Sequential
from keras.layers import Dense
from keras.callbacks import EarlyStopping
model1 = Sequential()
model1.add(Dense(100,activation=’relu’,input_dim=4))
model1.add(Dense(100,activation=’relu’))
model1.add(Dense(1))
np.random.seed(50)
model1.compile(optimizer=’adam’,loss=’mean_squared_error’,metrics=[‘mse’,r2])
early_stopping_monitor = EarlyStopping(monitor =”val_loss”,
mode =”min”, patience = 5,
restore_best_weights = True)
history=model1.fit(XTrain,YTrain,epochs=5000,
batch_size=64,validation_data=(XTest,YTest),
callbacks=[early_stopping_monitor]);
import matplotlib.pyplot as plt
plt.plot(history.history[‘r2’])
plt.plot(history.history[‘val_r2’],’r–‘)
plt.title(‘Model accuracy’)
plt.ylabel(‘Accuracy value’)
plt.xlabel(‘# epochs’)
plt.legend([‘train’,’test’],loc =’lower right’)
plt.show()
import matplotlib.pyplot as plt
plt.plot(history.history[‘loss’])
plt.plot(history.history[‘val_loss’],’r–‘)
plt.title(‘Model Loss’)
plt.ylabel(‘Loss value’)
plt.xlabel(‘# epochs’)
plt.legend([‘train’,’test’],loc =’upper right’)
plt.show()
from sklearn.metrics import r2_score
y_train_pred = model1.predict(XTrain)
y_test_pred = model1.predict(XTest)
y_pred = model1.predict(Xnorm)
r2_score(YTrain,y_train_pred),r2_score(YTest,y_test_pred),r2_score(Ynorm,y_pred)
#save the entire model
model1.save(“model1.h5”)
I believe this saved the whole model including the weights and architecture. In addition to this, i tried to load the model and to use it in predicting a new dataset but the error I got is;
ValueError: Unknown metric function: r2. Please ensure this object is passed to the
custom_objects
argument.This is how I tried to load the code;
import pandas as pd
from sklearn.model_selection import train_test_split
from tensorflow import keras
model2=keras.models.load_model(‘model1.h5’)
df=pd.read_csv(‘Al-Besharah Data.csv’)
df.head()
X=df.iloc[:,:-1]
Y=df.iloc[:,-1].values
from sklearn.preprocessing import MinMaxScaler
scaler = MinMaxScaler()
Xnorm = pd.DataFrame(data = scaler.fit_transform(X))
Yshape = pd.DataFrame(data = Y.reshape(-1,1))
Ynorm = pd.DataFrame(data = scaler.fit_transform(Yshape))
XTrain,XTest,YTrain,YTest=train_test_split(Xnorm,Ynorm,train_size=0.7,random_state=2)
Well done.
Question :
I have a file (model.h5) and I would like to open it as a source code (file.ipynb). Is it possible
The model seems to be a keras model saved in HDF5 format. Try to look for keras.models.load_model() function. But if the file does not have the full model, you must get the original model source code before you can use it.
Hi Jason
My question is i have bulit a model that is a hybrid of two cnn models. I have saved and loaded it as described here. But then it gives me error like this :-
AttributeError Traceback (most recent call last)
in ()
—-> 1 predictions = model_1.predict_proba([video_features_arr_norm,audio_features_arr])
AttributeError: ‘Functional’ object has no attribute ‘predict_proba’
What is the problem please tell me. Is saving hybrid models not possible by this method?
I think your model does not support predict_proba(), and not every model does. In this case you need to make your own function as replacement. See this question: https://stackoverflow.com/questions/63649503/attributeerror-functional-object-has-no-attribute-predict-proba
model.to_json() gives me a NotImplementedError. I have tried earlier .save() but when I loaded it back I got a dimension error which led me to look at saving the weights. What do I do if to_json and save are not working for me?
It said it all. The to_json() is not supported for this model. The save() should work but you must load it back into the same model. If anything goes off (e.g., a different size in one of the layer), you get dimension error.
Hello Jason,
As discussed in
https://machinelearningmastery.com/manually-optimize-neural-networks/?unapproved=654896&moderation-hash=fe3b8b1630d977e40be66ecc358cfaf7#comment-654896
How can we save a manual neural network as a model?
model from json fails with Custom layers. Any idea why?
Because Keras would not understand your custom layer by default. You need to tell Keras how you implemented your custom layer. See the example from https://www.tensorflow.org/guide/keras/save_and_serialize:
Excellent Blog on save load keras deep learning models. We are one of the tech news provider offering news related with recent development going on the tech tools. We gather information from various mnc and allocate with respect to categories
@Jason Brownlee
Can you please explain how to retrain/fine-tune the loaded model in keras?
Hi Meenakshi…The following resource may be of interest to you:
https://machinelearningmastery.com/how-to-train-an-object-detection-model-with-keras/
@James Carmichael
After loading the model I want to fine tune this model with my dataset and then want to evaluate it.
Kindly help.
As in the above code:
# serialize model to JSON
model_json = model.to_json()
with open(“model.json”, “w”) as json_file:
json_file.write(model_json)
# serialize weights to HDF5
model.save_weights(“model.h5”)
print(“Saved model to disk”)
# later…
# load json and create model
json_file = open(‘model.json’, ‘r’)
loaded_model_json = json_file.read()
json_file.close()
loaded_model = model_from_json(loaded_model_json)
# load weights into new model
loaded_model.load_weights(“model.h5”)
print(“Loaded model from disk”)
When you save the weights separate to the model, and recombine how does the computer know which weight goes to which neuron? Presumably some positional information ….
Would be great if you could help me with this.