The behavior and performance of many machine learning algorithms are referred to as stochastic.
Stochastic refers to a variable process where the outcome involves some randomness and has some uncertainty. It is a mathematical term and is closely related to “randomness” and “probabilistic” and can be contrasted to the idea of “deterministic.”
The stochastic nature of machine learning algorithms is an important foundational concept in machine learning and is required to be understand in order to effectively interpret the behavior of many predictive models.
In this post, you will discover a gentle introduction to stochasticity in machine learning.
After reading this post, you will know:
- A variable or process is stochastic if there is uncertainty or randomness involved in the outcomes.
- Stochastic is a synonym for random and probabilistic, although is different from non-deterministic.
- Many machine learning algorithms are stochastic because they explicitly use randomness during optimization or learning.
Kick-start your project with my new book Probability for Machine Learning, including step-by-step tutorials and the Python source code files for all examples.
Let’s get started.
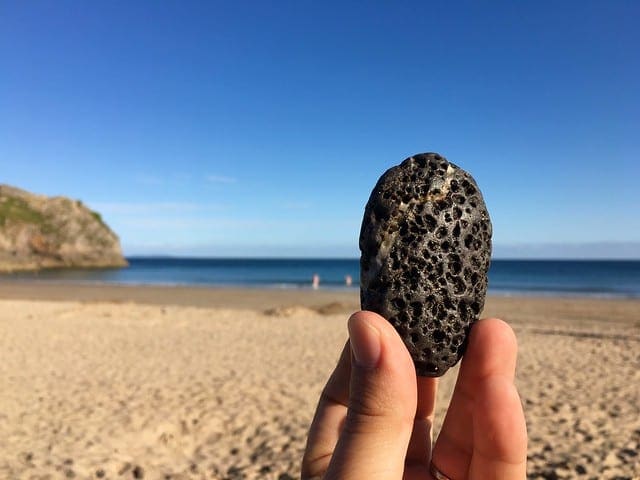
A Gentle Introduction to Stochastic in Machine Learning
Photo by Giles Turnbull, some rights reserved.
Overview
This tutorial is divided into three parts; they are:
- What Does “Stochastic” Mean?
- Stochastic vs. Random, Probabilistic, and Nondeterministic
- Stochastic in Machine Learning
What Does “Stochastic” Mean?
A variable is stochastic if the occurrence of events or outcomes involves randomness or uncertainty.
… “stochastic” means that the model has some kind of randomness in it
— Page 66, Think Bayes.
A process is stochastic if it governs one or more stochastic variables.
Games are stochastic because they include an element of randomness, such as shuffling or rolling of a dice in card games and board games.
In real life, many unpredictable external events can put us into unforeseen situations. Many games mirror this unpredictability by including a random element, such as the throwing of dice. We call these stochastic games.
— Page 177, Artificial Intelligence: A Modern Approach, 3rd edition, 2009.
Stochastic is commonly used to describe mathematical processes that use or harness randomness. Common examples include Brownian motion, Markov Processes, Monte Carlo Sampling, and more.
Now that we have some definitions, let’s try and add some more context by comparing stochastic with other notions of uncertainty.
Want to Learn Probability for Machine Learning
Take my free 7-day email crash course now (with sample code).
Click to sign-up and also get a free PDF Ebook version of the course.
Stochastic vs. Random, Probabilistic, and Non-deterministic
In this section, we’ll try to better understand the idea of a variable or process being stochastic by comparing it to the related terms of “random,” “probabilistic,” and “non-deterministic.”
Stochastic vs. Random
In statistics and probability, a variable is called a “random variable” and can take on one or more outcomes or events.
It is the common name used for a thing that can be measured.
In general, stochastic is a synonym for random.
For example, a stochastic variable is a random variable. A stochastic process is a random process.
Typically, random is used to refer to a lack of dependence between observations in a sequence. For example, the rolls of a fair die are random, so are the flips of a fair coin.
Strictly speaking, a random variable or a random sequence can still be summarized using a probability distribution; it just may be a uniform distribution.
We may choose to describe something as stochastic over random if we are interested in focusing on the probabilistic nature of the variable, such as a partial dependence of the next event on the current event. We may choose random over stochastic if we wish to focus attention on the independence of the events.
Stochastic vs. Probabilistic
In general, stochastic is a synonym for probabilistic.
For example, a stochastic variable or process is probabilistic. It can be summarized and analyzed using the tools of probability.
Most notably, the distribution of events or the next event in a sequence can be described in terms of a probability distribution.
We may choose to describe a variable or process as probabilistic over stochastic if we wish to emphasize the dependence, such as if we are using a parametric model or known probability distribution to summarize the variable or sequence.
Stochastic vs. Non-deterministic
A variable or process is deterministic if the next event in the sequence can be determined exactly from the current event.
For example, a deterministic algorithm will always give the same outcome given the same input. Conversely, a non-deterministic algorithm may give different outcomes for the same input.
A stochastic variable or process is not deterministic because there is uncertainty associated with the outcome.
Nevertheless, a stochastic variable or process is also not non-deterministic because non-determinism only describes the possibility of outcomes, rather than probability.
Describing something as stochastic is a stronger claim than describing it as non-deterministic because we can use the tools of probability in analysis, such as expected outcome and variance.
… “stochastic” generally implies that uncertainty about outcomes is quantified in terms of probabilities; a nondeterministic environment is one in which actions are characterized by their possible outcomes, but no probabilities are attached to them.
— Page 43, Artificial Intelligence: A Modern Approach, 3rd edition, 2009.
Stochastic in Machine Learning
Many machine learning algorithms and models are described in terms of being stochastic.
This is because many optimization and learning algorithms both must operate in stochastic domains and because some algorithms make use of randomness or probabilistic decisions.
Let’s take a closer look at the source of uncertainty and the nature of stochastic algorithms in machine learning.
Stochastic Problem Domains
Stochastic domains are those that involve uncertainty.
… machine learning must always deal with uncertain quantities, and sometimes may also need to deal with stochastic (non-deterministic) quantities. Uncertainty and stochasticity can arise from many sources.
— Page 54, Deep Learning, 2016.
This uncertainty can come from a target or objective function that is subjected to statistical noise or random errors.
It can also come from the fact that the data used to fit a model is an incomplete sample from a broader population.
Finally, the models chosen are rarely able to capture all of the aspects of the domain, and instead must generalize to unseen circumstances and lose some fidelity.
Stochastic Optimization Algorithms
Stochastic optimization refers to a field of optimization algorithms that explicitly use randomness to find the optima of an objective function, or optimize an objective function that itself has randomness (statistical noise).
Most commonly, stochastic optimization algorithms seek a balance between exploring the search space and exploiting what has already been learned about the search space in order to hone in on the optima. The choice of the next locations in the search space are chosen stochastically, that is probabilistically based on what areas have been searched recently.
Stochastic hill climbing chooses at random from among the uphill moves; the probability of selection can vary with the steepness of the uphill move.
— Page 124, Artificial Intelligence: A Modern Approach, 3rd edition, 2009.
Popular examples of stochastic optimization algorithms are:
- Simulated Annealing
- Genetic Algorithm
- Particle Swarm Optimization
Particle swarm optimization (PSO) is a stochastic optimization approach, modeled on the social behavior of bird flocks.
— Page 9, Computational Intelligence: An Introduction.
Stochastic Learning Algorithms
Most machine learning algorithms are stochastic because they make use of randomness during learning.
Using randomness is a feature, not a bug. It allows the algorithms to avoid getting stuck and achieve results that deterministic (non-stochastic) algorithms cannot achieve.
For example, some machine learning algorithms even include “stochastic” in their name such as:
- Stochastic Gradient Descent (optimization algorithm).
- Stochastic Gradient Boosting (ensemble algorithm).
Stochastic gradient descent optimizes the parameters of a model, such as an artificial neural network, that involves randomly shuffling the training dataset before each iteration that causes different orders of updates to the model parameters. In addition, model weights in a neural network are often initialized to a random starting point.
Most deep learning algorithms are based on an optimization algorithm called stochastic gradient descent.
— Page 98, Deep Learning, 2016.
Stochastic gradient boosting is an ensemble of decision trees algorithms. The stochastic aspect refers to the random subset of rows chosen from the training dataset used to construct trees, specifically the split points of trees.
Stochastic Algorithm Behaviour
Because many machine learning algorithms make use of randomness, their nature (e.g. behavior and performance) is also stochastic.
The stochastic nature of machine learning algorithms is most commonly seen on complex and nonlinear methods used for classification and regression predictive modeling problems.
These algorithms make use of randomness during the process of constructing a model from the training data which has the effect of fitting a different model each time same algorithm is run on the same data. In turn, the slightly different models have different performance when evaluated on a hold out test dataset.
This stochastic behavior of nonlinear machine learning algorithms is challenging for beginners who assume that learning algorithms will be deterministic, e.g. fit the same model when the algorithm is run on the same data.
This stochastic behavior requires that the performance of the model must be summarized using summary statistics that describe the mean or expected performance of the model, rather than the performance of the model from any single training run.
For more on this topic, see the post:
Further Reading
This section provides more resources on the topic if you are looking to go deeper.
Posts
- How to Generate Random Numbers in Python
- Introduction to Random Number Generators for Machine Learning in Python
- Embrace Randomness in Machine Learning
- Why Initialize a Neural Network with Random Weights?
- Stochastic Gradient Boosting with XGBoost and scikit-learn in Python
Articles
- Random variable, Wikipedia.
- Statistical randomness, Wikipedia.
- Stochastic, Wikipedia.
- Stochastic process, Wikipedia.
- Stochastic optimization.
Summary
In this post, you discovered a gentle introduction to stochasticity in machine learning.
Specifically, you learned:
- A variable or process is stochastic if there is uncertainty or randomness involved in the outcomes.
- Stochastic is a synonym for random and probabilistic, although is different from non-deterministic.
- Many machine learning algorithms are stochastic because they explicitly use randomness during optimization or learning.
Do you have any questions?
Ask your questions in the comments below and I will do my best to answer.
Good article! Thank you. stochastic == randomness and uncertainty. Didn’t know that many ML algorithms explicitly make use of randomness. Learned a lot from this article.
Thanks, I’m really happy to hear that!
Great introduction. I always used to wonder about the SGD…and then you explained beautifully about the differences between stochastic /deterministic/non-deterministic. Fantastic explanation.
Thanks, I’m happy it helped!
Thanks for the article Jason, I love your top-down approach books which are really useful to try out things really quickly but also complete in their content.
About stochasticity, maybe we could make a distinction between the training and estimating point to make it clear? I mean, although the training process can be stochastic when fitting a neural network, the estimating process when predicting the output (for an already trained network model) is deterministic (i.e. we hope to get the same output with the same input).
Thanks!
Exactly right. Training is stochastic, inference is deterministic.
Great distinction and suggestion.
Hello Jason!
Thank you for this article that makes many thing clear in terms of terminology! I could imagine one more sub-chapter called: “Stochastic vs. Statistical”.
Just for curiosity: your posts recommended for further reading are inserted manually or maybe you apply some document suggestion model/algorithm (such as TF-IDF)?
Regards!
Thanks.
I write the sections manually as I gather resources for the tutorial. I’m very manual/analog in general 🙂
Hi Jason,
Just to clarify for my own understanding, if we set a random seed (and random_state) for ML model on some data
(say seed/state = 123), the trained model will be the same for each training iteration, right?
Thanks,
Matt
It depends what the seed is for.
If the seed is for the resampling method or train/test split, you will have a different split of the data and training set with different seeds.
I’m in my seventies, very well educated & read but heard a word from my 9 yr old grandson I had never heard before. My daughter, her son and me driving home from skiing and James said something and mom asked are you being “sarcastic”. He said no, ” scoastic”.when asked what he meant he said “out of the blue”. We did not know the word..so later on the next day I googled ” what I thought he said and Google asked ” did I mean scholastic ?..meaning random or “” out of the blue “” .That is what James meant. He had heard this from one of his primary school teachers, remembered the word , the meaning “out of the blue “. He is in grade 4…Just a reminder for us adults NEVER to underestimate others and say “” no you are wrong..that is wrong,no such word,that could never had happened etc.. What damage that could cause in so many ways.
I called James today and told him there was such a word and it did mean out of the blue “” random””.
Too many parents, teachers or others jump TOO QUICKLY that they must be right !
But if we try we can improve !!!
Haha, thanks for sharing!
what to expect under “Stochastic search methods”?
For example, simulated annealing. Because it search over different possible solutions randomly.
Excellent explanation. I understood the idea of random/stochastic/probabilistic are in general synonym but still couldn’t understand the idea of using one term over the other.
Great point, thanks! I’ll think about how to explain when to use each term.
i love your page, always excellent and understandable information!
Thanks!
Great, even I understood the basics (and that’s saying something!)
Thanks, I’m happy to hear it was helpful!
Thank you Jason, always inspiring reading your posts!
In viewing the stochastic nature of ML algorithm, what do you think will be the best protocol to do hyperparameter search during cross validation? Will the optimal hyper parameters change due to random seed?
You mentioned we should show the mean of performance for many trainings rather than a single training, I highly agree with you. Assume we find a set of optimal hyper parameters (with a particular random seed), will it be sufficient if we just change the random seed as a source of randomness to estimate the mean and variance of the performance?
Cheers
You’re welcome.
Nested cv is a good approach:
https://machinelearningmastery.com/nested-cross-validation-for-machine-learning-with-python/
Thank you Jason. I wonder if there is any I should pay attention to if the data set is evolving time series (apart from the need to use tscv instead of k-fold cv)?
Cheers
k-fold cross-validation is not appropriate for time series, you can use walk-forward validation:
https://machinelearningmastery.com/backtest-machine-learning-models-time-series-forecasting/
hi,
I used one-class classification. Because of the stochastic nature of machine learning algorithms, I want to repeat the experiment several times and calculate the average of the metrics. In the code I wrote:
tprs=[]
fprs=[]
for i in rang(2):
clf=OneClassSVM(nu=0.1, kernel=’rbf’).fit(x_train)
score=clf.decision_function(x_test)
fpr, tpr, thresholds=metrics.roc_curve(y_test, score)
tprs.append(tpr)
fprs.append(fpr)
print(np.mean(tprs), np.mean(fprs)) # error in this ligne
This error occurred in np.mean() because the shape of fpr or tpr is changed for both iterations. How can I correct this error and compute the mean of fpr and tpr?
This is a common question that I answer here:
https://machinelearningmastery.com/faq/single-faq/can-you-read-review-or-debug-my-code
I’m going to purchase your Probability for Machine Learning, eBook…Also, I’m interested in Stochastic Processes in Machine Learning and Quantum Mechanics in Machine Learning-Do you any recommendations for Stochastic & QM in Machine Learning!? Please let me know…Thanks, FR
Hi Frank…Thank you for your support! We do not offer content related to QM. Please let us know if we can answer any questions regarding our content.