Once you choose and fit a final deep learning model in Keras, you can use it to make predictions on new data instances.
There is some confusion amongst beginners about how exactly to do this. I often see questions such as:
How do I make predictions with my model in Keras?
In this tutorial, you will discover exactly how you can make classification and regression predictions with a finalized deep learning model with the Keras Python library.
After completing this tutorial, you will know:
- How to finalize a model in order to make it ready for making predictions.
- How to make class and probability predictions for classification problems in Keras.
- How to make regression predictions in in Keras.
Kick-start your project with my new book Deep Learning With Python, including step-by-step tutorials and the Python source code files for all examples.
Let’s get started.
- Apr/2018: First publish
- Updated Jan/2020: Updated for changes in scikit-learn v0.22 API.
- Updated Aug/2022: Updated for TensorFlow 2.x syntax
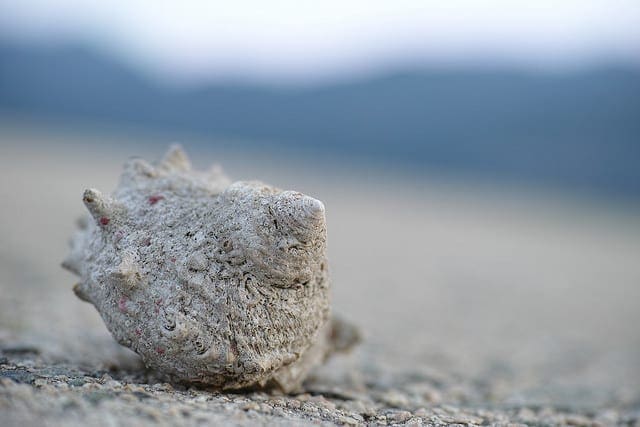
How to Make Classification and Regression Predictions for Deep Learning Models in Keras
Photo by mstk east, some rights reserved.
Tutorial Overview
This tutorial is divided into 3 parts; they are:
- Finalize Model
- Classification Predictions
- Regression Predictions
1. Finalize Model
Before you can make predictions, you must train a final model.
You may have trained models using k-fold cross validation or train/test splits of your data. This was done in order to give you an estimate of the skill of the model on out of sample data, e.g. new data.
These models have served their purpose and can now be discarded.
You now must train a final model on all of your available data. You can learn more about how to train a final model here:
2. Classification Predictions
Classification problems are those where the model learns a mapping between input features and an output feature that is a label, such as “spam” and “not spam“.
Below is an example of a finalized neural network model in Keras developed for a simple two-class (binary) classification problem.
If developing a neural network model in Keras is new to you, see this Keras tutorial.
1 2 3 4 5 6 7 8 9 10 11 12 13 14 15 16 17 |
# example of training a final classification model from keras.models import Sequential from keras.layers import Dense from sklearn.datasets import make_blobs from sklearn.preprocessing import MinMaxScaler # generate 2d classification dataset X, y = make_blobs(n_samples=100, centers=2, n_features=2, random_state=1) scalar = MinMaxScaler() scalar.fit(X) X = scalar.transform(X) # define and fit the final model model = Sequential() model.add(Dense(4, input_shape=(2,), activation='relu')) model.add(Dense(4, activation='relu')) model.add(Dense(1, activation='sigmoid')) model.compile(loss='binary_crossentropy', optimizer='adam') model.fit(X, y, epochs=200, verbose=0) |
After finalizing, you may want to save the model to file, e.g. via the Keras API. Once saved, you can load the model any time and use it to make predictions. For an example of this, see the post:
For simplicity, we will skip this step for the examples in this tutorial.
There are two types of classification predictions we may wish to make with our finalized model; they are class predictions and probability predictions.
Class Predictions
A class prediction is given the finalized model and one or more data instances, predict the class for the data instances.
We do not know the outcome classes for the new data. That is why we need the model in the first place.
We can predict the class for new data instances using our finalized classification model in Keras using the predict_classes() function. Note that this function is only available on Sequential models, not those models developed using the functional API.
For example, we have one or more data instances in an array called Xnew. This can be passed to the predict_classes() function on our model in order to predict the class values for each instance in the array.
1 2 |
Xnew = [[...], [...]] ynew = model.predict_classes(Xnew) |
Let’s make this concrete with an example:
1 2 3 4 5 6 7 8 9 10 11 12 13 14 15 16 17 18 19 20 21 22 23 24 25 |
# example making new class predictions for a classification problem from keras.models import Sequential from keras.layers import Dense from sklearn.datasets import make_blobs from sklearn.preprocessing import MinMaxScaler # generate 2d classification dataset X, y = make_blobs(n_samples=100, centers=2, n_features=2, random_state=1) scalar = MinMaxScaler() scalar.fit(X) X = scalar.transform(X) # define and fit the final model model = Sequential() model.add(Dense(4, input_shape=(2,), activation='relu')) model.add(Dense(4, activation='relu')) model.add(Dense(1, activation='sigmoid')) model.compile(loss='binary_crossentropy', optimizer='adam') model.fit(X, y, epochs=500, verbose=0) # new instances where we do not know the answer Xnew, _ = make_blobs(n_samples=3, centers=2, n_features=2, random_state=1) Xnew = scalar.transform(Xnew) # make a prediction ynew = model.predict_classes(Xnew) # show the inputs and predicted outputs for i in range(len(Xnew)): print("X=%s, Predicted=%s" % (Xnew[i], ynew[i])) |
Running the example predicts the class for the three new data instances, then prints the data and the predictions together.
Note: Your results may vary given the stochastic nature of the algorithm or evaluation procedure, or differences in numerical precision. Consider running the example a few times and compare the average outcome.
1 2 3 |
X=[0.89337759 0.65864154], Predicted=[0] X=[0.29097707 0.12978982], Predicted=[1] X=[0.78082614 0.75391697], Predicted=[0] |
If you had just one new data instance, you could provide this as an instance wrapped in an array to the predict_classes() function; for example:
1 2 3 4 5 6 7 8 9 10 11 12 13 14 15 16 17 18 19 20 21 22 23 24 |
# example making new class prediction for a classification problem from keras.models import Sequential from keras.layers import Dense from sklearn.datasets import make_blobs from sklearn.preprocessing import MinMaxScaler from numpy import array # generate 2d classification dataset X, y = make_blobs(n_samples=100, centers=2, n_features=2, random_state=1) scalar = MinMaxScaler() scalar.fit(X) X = scalar.transform(X) # define and fit the final model model = Sequential() model.add(Dense(4, input_shape=(2,), activation='relu')) model.add(Dense(4, activation='relu')) model.add(Dense(1, activation='sigmoid')) model.compile(loss='binary_crossentropy', optimizer='adam') model.fit(X, y, epochs=500, verbose=0) # new instance where we do not know the answer Xnew = array([[0.89337759, 0.65864154]]) # make a prediction ynew = model.predict_classes(Xnew) # show the inputs and predicted outputs print("X=%s, Predicted=%s" % (Xnew[0], ynew[0])) |
Running the example prints the single instance and the predicted class.
Note: Your results may vary given the stochastic nature of the algorithm or evaluation procedure, or differences in numerical precision. Consider running the example a few times and compare the average outcome.
1 |
X=[0.89337759 0.65864154], Predicted=[0] |
A Note on Class Labels
Note that when you prepared your data, you will have mapped the class values from your domain (such as strings) to integer values. You may have used a LabelEncoder.
This LabelEncoder can be used to convert the integers back into string values via the inverse_transform() function.
For this reason, you may want to save (pickle) the LabelEncoder used to encode your y values when fitting your final model.
Probability Predictions
Another type of prediction you may wish to make is the probability of the data instance belonging to each class.
This is called a probability prediction where, given a new instance, the model returns the probability for each outcome class as a value between 0 and 1.
You can make these types of predictions in Keras by calling the predict_proba() function; for example:
1 2 |
Xnew = [[...], [...]] ynew = model.predict_proba(Xnew) |
In the case of a two-class (binary) classification problem, the sigmoid activation function is often used in the output layer. The predicted probability is taken as the likelihood of the observation belonging to class 1, or inverted (1 – probability) to give the probability for class 0.
In the case of a multi-class classification problem, the softmax activation function is often used on the output layer and the likelihood of the observation for each class is returned as a vector.
The example below makes a probability prediction for each example in the Xnew array of data instance.
1 2 3 4 5 6 7 8 9 10 11 12 13 14 15 16 17 18 19 20 21 22 23 24 25 |
# example making new probability predictions for a classification problem from keras.models import Sequential from keras.layers import Dense from sklearn.datasets import make_blobs from sklearn.preprocessing import MinMaxScaler # generate 2d classification dataset X, y = make_blobs(n_samples=100, centers=2, n_features=2, random_state=1) scalar = MinMaxScaler() scalar.fit(X) X = scalar.transform(X) # define and fit the final model model = Sequential() model.add(Dense(4, input_shape=(2,), activation='relu')) model.add(Dense(4, activation='relu')) model.add(Dense(1, activation='sigmoid')) model.compile(loss='binary_crossentropy', optimizer='adam') model.fit(X, y, epochs=500, verbose=0) # new instances where we do not know the answer Xnew, _ = make_blobs(n_samples=3, centers=2, n_features=2, random_state=1) Xnew = scalar.transform(Xnew) # make a prediction ynew = model.predict_proba(Xnew) # show the inputs and predicted outputs for i in range(len(Xnew)): print("X=%s, Predicted=%s" % (Xnew[i], ynew[i])) |
Running the instance makes the probability predictions and then prints the input data instance and the probability of each instance belonging to class 1.
Note: Your results may vary given the stochastic nature of the algorithm or evaluation procedure, or differences in numerical precision. Consider running the example a few times and compare the average outcome.
1 2 3 |
X=[0.89337759 0.65864154], Predicted=[0.0087348] X=[0.29097707 0.12978982], Predicted=[0.82020265] X=[0.78082614 0.75391697], Predicted=[0.00693122] |
This can be helpful in your application if you want to present the probabilities to the user for expert interpretation.
3. Regression Predictions
Regression is a supervised learning problem where given input examples, the model learns a mapping to suitable output quantities, such as “0.1” and “0.2”, etc.
Below is an example of a finalized Keras model for regression.
1 2 3 4 5 6 7 8 9 10 11 12 13 14 15 16 17 18 19 |
# example of training a final regression model from keras.models import Sequential from keras.layers import Dense from sklearn.datasets import make_regression from sklearn.preprocessing import MinMaxScaler # generate regression dataset X, y = make_regression(n_samples=100, n_features=2, noise=0.1, random_state=1) scalarX, scalarY = MinMaxScaler(), MinMaxScaler() scalarX.fit(X) scalarY.fit(y.reshape(100,1)) X = scalarX.transform(X) y = scalarY.transform(y.reshape(100,1)) # define and fit the final model model = Sequential() model.add(Dense(4, input_shape=(2,), activation='relu')) model.add(Dense(4, activation='relu')) model.add(Dense(1, activation='linear')) model.compile(loss='mse', optimizer='adam') model.fit(X, y, epochs=1000, verbose=0) |
We can predict quantities with the finalized regression model by calling the predict() function on the finalized model.
The predict() function takes an array of one or more data instances.
The example below demonstrates how to make regression predictions on multiple data instances with an unknown expected outcome.
1 2 3 4 5 6 7 8 9 10 11 12 13 14 15 16 17 18 19 20 21 22 23 24 25 26 27 |
# example of making predictions for a regression problem from keras.models import Sequential from keras.layers import Dense from sklearn.datasets import make_regression from sklearn.preprocessing import MinMaxScaler # generate regression dataset X, y = make_regression(n_samples=100, n_features=2, noise=0.1, random_state=1) scalarX, scalarY = MinMaxScaler(), MinMaxScaler() scalarX.fit(X) scalarY.fit(y.reshape(100,1)) X = scalarX.transform(X) y = scalarY.transform(y.reshape(100,1)) # define and fit the final model model = Sequential() model.add(Dense(4, input_shape=(2,), activation='relu')) model.add(Dense(4, activation='relu')) model.add(Dense(1, activation='linear')) model.compile(loss='mse', optimizer='adam') model.fit(X, y, epochs=1000, verbose=0) # new instances where we do not know the answer Xnew, a = make_regression(n_samples=3, n_features=2, noise=0.1, random_state=1) Xnew = scalarX.transform(Xnew) # make a prediction ynew = model.predict(Xnew) # show the inputs and predicted outputs for i in range(len(Xnew)): print("X=%s, Predicted=%s" % (Xnew[i], ynew[i])) |
Running the example makes multiple predictions, then prints the inputs and predictions side by side for review.
Note: Your results may vary given the stochastic nature of the algorithm or evaluation procedure, or differences in numerical precision. Consider running the example a few times and compare the average outcome.
1 2 3 |
X=[0.29466096 0.30317302], Predicted=[0.17097184] X=[0.39445118 0.79390858], Predicted=[0.7475489] X=[0.02884127 0.6208843 ], Predicted=[0.43370453] |
The same function can be used to make a prediction for a single data instance, as long as it is suitably wrapped in a surrounding list or array.
For example:
1 2 3 4 5 6 7 8 9 10 11 12 13 14 15 16 17 18 19 20 21 22 23 24 25 26 |
# example of making predictions for a regression problem from keras.models import Sequential from keras.layers import Dense from sklearn.datasets import make_regression from sklearn.preprocessing import MinMaxScaler from numpy import array # generate regression dataset X, y = make_regression(n_samples=100, n_features=2, noise=0.1, random_state=1) scalarX, scalarY = MinMaxScaler(), MinMaxScaler() scalarX.fit(X) scalarY.fit(y.reshape(100,1)) X = scalarX.transform(X) y = scalarY.transform(y.reshape(100,1)) # define and fit the final model model = Sequential() model.add(Dense(4, input_shape=(2,), activation='relu')) model.add(Dense(4, activation='relu')) model.add(Dense(1, activation='linear')) model.compile(loss='mse', optimizer='adam') model.fit(X, y, epochs=1000, verbose=0) # new instance where we do not know the answer Xnew = array([[0.29466096, 0.30317302]]) # make a prediction ynew = model.predict(Xnew) # show the inputs and predicted outputs print("X=%s, Predicted=%s" % (Xnew[0], ynew[0])) |
Running the example makes a single prediction and prints the data instance and prediction for review.
Note: Your results may vary given the stochastic nature of the algorithm or evaluation procedure, or differences in numerical precision. Consider running the example a few times and compare the average outcome.
1 |
X=[0.29466096 0.30317302], Predicted=[0.17333156] |
Further Reading
This section provides more resources on the topic if you are looking to go deeper.
- How to Train a Final Machine Learning Model
- Save and Load Your Keras Deep Learning Models
- Develop Your First Neural Network in Python With Keras Step-By-Step
- The 5 Step Life-Cycle for Long Short-Term Memory Models in Keras
- How to Make Predictions with Long Short-Term Memory Models in Keras
Summary
In this tutorial, you discovered how you can make classification and regression predictions with a finalized deep learning model with the Keras Python library.
Specifically, you learned:
- How to finalize a model in order to make it ready for making predictions.
- How to make class and probability predictions for classification problems in Keras.
- How to make regression predictions in in Keras.
Do you have any questions?
Ask your questions in the comments below and I will do my best to answer.
Great article Jason. Do you recommend any articles for hyperparameter tuning to further improve accuracies? Also any articles for common problems and solutions during model tuning?
Yes, see this post:
https://machinelearningmastery.com/grid-search-hyperparameters-deep-learning-models-python-keras/
Thanks for tutorial.
I have a problem with my data because there aren’t of the same dimensions, so the software don’t work. If I fill in the blanks with random numbers to have the dimensions, it’s work but I haven’t the good answers. Can you explain me how I can do to solve this problem.
Thanks
Perhaps this post will help:
https://machinelearningmastery.com/handle-missing-timesteps-sequence-prediction-problems-python/
Thanks for the tutorial! If I want to build a CNN which has both classification and regression heads, I suppose I cannot use a sequential model. Do you know an example for such a multi-head CNN? Thank you
Here are some examples of multiple output models:
https://machinelearningmastery.com/keras-functional-api-deep-learning/
hey Jason! your tutorial is very helpful. it would be great of you if you could share how to predict a keras model in which pre-trained model is a pkl file and we need to predict a single image.
Thanks!
Sure, see this:
https://machinelearningmastery.com/how-to-use-transfer-learning-when-developing-convolutional-neural-network-models/
And this:
https://machinelearningmastery.com/save-load-keras-deep-learning-models/
Thanks for your the explication,
Could you please put photos of network architectures aside the code ?
I think it will help us to understand best the architecture
Thanks for the suggestion.
hello Jason,
thanks for your articles. you really help us a lot.
may i ask one question ?
Can Keras be used to build clustering models?
keras.wrappers.scikit_learn can be used to build KerasClassifier model, Keras be used to build clustering models? If it can be, are there any examples for that?
you know i want to use some features like age, city, education, company, job title and so on to cluster people into some groups and to get the key features of each group.
Perhaps, I have not seen this.
Hi Jason and thanks for this post. I have a quick question about regression with bounded target values.
If my target values are always restricted between [0,1] with most of the values close to 0.5 (i.e., values are rarely close to 0 or 1), is it useful to use sigmoid output activation instead of linear? Would it help in convergence or stability when training a complex model? It seems like a waste not to take any advantage of the fact that target values belong into bounded interval.
So in your code, one would simply make a replacement
model.add(Dense(1, activation=’linear’)) –> model.add(Dense(1, activation=’sigmoid’))
Good question.
Yes, interesting idea. It might change the loss function used to fit the model, which may result in optimizing the wrong problem (e.g. logloss instead of mse). Nevertheless, try it and compare error results between the two approaches.
Yes, that is the code change.
What are the considerations in using scikit-learn over Keras for classification problems (or vis versa) in R or Python?
scikit-learn offers a suite of general ML algorithms, Keras is focused on neural network algorithms only.
Regarding Python vs R, I answer that here:
https://machinelearningmastery.com/faq/single-faq/what-programming-language-should-i-use-for-machine-learning
Does that help?
I am trying to predict a new image on a model that I trained with emnist letters. Here is the code snippet that tries to do so.
import matplotlib
# Force matplotlib to not use any Xwindows backend.
matplotlib.use(‘Agg’)
import keras
import matplotlib.pyplot as plt
from keras.models import load_model
from keras.preprocessing import image
from keras import backend as K
from scipy.misc import imread
from PIL import Image
import skimage.io as io
import skimage.transform as tr
import numpy as np
from keras.utils import plot_model
from keras.datasets import mnist
# Returns a compiled model identical to the previous one
model = load_model(‘matLabbed.h5’)
print(“Testing the model on our own input data”)
imgA = imread(‘A.png’)
imgA = tr.resize(imgA, (28, 28, 1)).astype(‘float32’)
imgA = imgA[np.newaxis, …]
y_classes = model.predict_classes(imgA)
When I try to print y_classes, it gives me numerical outputs e.g 4, 10. I am trying to figure out the labels that my data uses and compare that with the y_classes output. Any suggestion? Thanks.
The integer will relate to a class in your training data.
how to predict classes with model developed using functional API ?
You can call model.predict() then use argmax() on the resulting vector to get the class index.
Hi Jason, this code editor is really irritating, please consider substituting it with another or just with some simple text.
Here is more information on how to copy code from the snippets:
https://machinelearningmastery.com/faq/single-faq/how-do-i-copy-code-from-a-tutorial
Does that help?
The networks for classification and regression differ only a little (activation function of the output neuron and the the loss function) yet in the case of classification it is so easy to estimate the probability of the prediction (via predict_proba) while in the case of regression the analog is the prediction interval which is difficult to calculate for non-linear models like neural networks.
Why is there such a difference? Shouldn’t the probability estimation for regression be done as easily as for classification? A straightforward (but maybe naive) solution that I see is to bin the target variable, make a classification, then use predict_proba to get the probability of the predicted value to be in a certain interval, and then to construct prediction interval from that.
Can it be done this way? Or changing the loss function will make two problems (regression and classification for the binned target) so much different that the result of one problem cannot be transferred to another?
The binning approach would be my first/naive thought too. Try it and see.
OK, I tried it for a toy 1-dim regression task.
A few days ago, I asked this question on stackexchange. Didn’t get many replies, so I now answered my own question by implementing in Keras the idea that I outlined in my comment above.
It looks like it works. I am still not sure if I do all this correctly, and if it will work for real multi-dimensional problems. If you like, you can see it here: https://datascience.stackexchange.com/questions/31773/how-to-estimate-the-variance-of-regressors-in-scikit-learn/32486#32486
The approach makes me nervous.
Hi Jason,
I built a model to classify a financial time series for stock trading having 10 inputs and one output (‘0’ for stock going down, ‘1’ going up). Now I have 1400 such inputs for training the model and managed to get an accuracy of 97%! …
but when presenting new inputs to the model I don’t get a classification accuracy more than 30%! ..in my opinion the problem would be:
1. Overfitting
2, time series not predictable?
etc…
do you have any idea what the problem might be or how to improve the accuracy for new data presented to the model?
Thanks
Low skill might mean underfitting, the wrong model, an intractable problem, etc.
how to get the RMSE for the regression model?
You can calculate the MSE and then calculate the square root of the MSE.
sklearn has an implementation here:
http://scikit-learn.org/stable/modules/generated/sklearn.metrics.mean_squared_error.html
Does that help?
very helpful. thanks
I’m glad it helped.
Hi, I am working on a multilabel problem. My X_train has this shape (for instance) [[0 1 0 0 1]…]. I want to predict the next, like [1 0 0 1 0]. How can I do that? Each label is independent from the others. How train that? Where can I find some information to learn about it?
Thanks!:)
Sorry, I don’t have examples of multilabel forecasting, only multiclass forecasting.
Very nice! could you plz also make a similar tutorial with PyToorch.
Thanks for the suggestion.
Thanks for your post, Jason.
When we are predicting, we are required to revert back to the original scaling. as both the input and output seem to be scaled for the model to predict correctly.
Correct, we should return yhat to the original scale before evaluating error in the case of regression.
Hi, thanks for a great post.
I have question related to regression problem.
I am trying to develop a model that predcits 3 outputs from 36 input variables. To be clear, I have 3 output parameters and 36 input parameters. Which means that in the output layer I have 3 neurons. The model can sucessively predict whe there is only one output, but when it comes to 3 outputs it gives somethig useless. What do you suggest? By the way I use keras mlp regressor.
Perhaps try tuning the model to see if you can find a configuration that best suits your specific problem, here are some ideas:
https://machinelearningmastery.com/improve-deep-learning-performance/
Hi Jason, Thanks for the explanation of classification and regression architecture.
I am trying to use the regression model on 300 dimensions vectors. So the problem statement is to Know which two vectors are similar or non-similar. So for this, I have taken the difference between two similar vectors and non-similar vectors. We labeled similar diff vectors as 0 and non-similar diff vectors as 1. And feeding it to the network of which looks like this:
model = Sequential()
model.add(Dense(300, input_dim=301, activation=’relu’))
model.add(Dense(1, activation=’sigmoid’))
model.compile(loss=’binary_crossentropy’, optimizer=’adam’, metrics=[‘acc’])
Training_Loss: 0.0171
Training_Acc: 0.9988
Testing_Loss = 0.6456110666
Testing_Acc = 0.821831868464
I am not sure, why the model is overfitting, Can you please give some insight into what could be wrong?
Perhaps try some different model configurations and see if they over fit? E.g. less nodes.
Hi,
Thanks for this explanation.
i am trying to use a end to end nvidia model for self driving car in keras. its a regression problem to predict the angle of steering by providing image of camera installed front side of car.
here the problem i am facing is when i predicting the angle using model.predict() , i get a constant value for all input. even my model gives very less error at the time of training like–
Epoch 00001: val_loss improved from inf to 21.63187, saving model to drive/abc/weights.best6.hdf5
Epoch 2/30
283/283 [==============================] – 199s 702ms/step – loss: 18.0307 – val_loss: 16.9002
Epoch 00002: val_loss improved from 21.63187 to 16.90022, saving model to drive/abc/weights.best6.hdf5
Epoch 3/30
5/283 […………………………] – ETA: 25s – loss: 16.0846283/283 [==============================] – 199s 704ms/step – loss: 14.2439 – val_loss: 13.9543
Epoch 00003: val_loss improved from 16.90022 to 13.95434, saving model to drive/abc/weights.best6.hdf5
Epoch 4/30
283/283 [==============================] – 200s 708ms/step – loss: 11.9354 – val_loss: 12.1763
Epoch 00004: val_loss improved from 13.95434 to 12.17632, saving model to drive/abc/weights.best6.hdf5
Epoch 5/30
4/283 […………………………] – ETA: 25s – loss: 11.4636283/283 [==============================] – 201s 711ms/step – loss: 10.5595 – val_loss: 11.1414
Epoch 00005: val_loss improved from 12.17632 to 11.14141, saving model to drive/abc/weights.best6.hdf5
Epoch 6/30
283/283 [==============================] – 201s 711ms/step – loss: 8.3687 – val_loss: 0.7611
Epoch 00006: val_loss improved from 11.14141 to 0.76112, saving model to drive/abc/weights.best6.hdf5
Epoch 7/30
3/283 […………………………] – ETA: 26s – loss: 1.0417283/283 [==============================] – 199s 704ms/step – loss: 0.7403 – val_loss: 0.4893
Epoch 00007: val_loss improved from 0.76112 to 0.48934, saving model to drive/abc/weights.best6.hdf5
Epoch 8/30
283/283 [==============================] – 203s 716ms/step – loss: 0.5372 – val_loss: 0.3477
Epoch 00008: val_loss improved from 0.48934 to 0.34773, saving model to drive/abc/weights.best6.hdf5
Epoch 9/30
6/283 […………………………] – ETA: 26s – loss: 0.4749283/283 [==============================] – 216s 763ms/step – loss: 0.4332 – val_loss: 0.2760
Epoch 00009: val_loss improved from 0.34773 to 0.27596, saving model to drive/abc/weights.best6.hdf5
Epoch 10/30
283/283 [==============================] – 211s 744ms/step – loss: 0.3821 – val_loss: 0.2406
Epoch 00010: val_loss improved from 0.27596 to 0.24057, saving model to drive/abc/weights.best6.hdf5
Epoch 11/30
6/283 […………………………] – ETA: 25s – loss: 0.3903283/283 [==============================] – 207s 733ms/step – loss: 0.3565 – val_loss: 0.2229
Epoch 00011: val_loss improved from 0.24057 to 0.22293, saving model to drive/abc/weights.best6.hdf5
Epoch 12/30
283/283 [==============================] – 222s 784ms/step – loss: 0.3438 – val_loss: 0.2134
Epoch 00012: val_loss improved from 0.22293 to 0.21340, saving model to drive/abc/weights.best6.hdf5
Epoch 13/30
7/283 […………………………] – ETA: 25s – loss: 0.3331283/283 [==============================] – 205s 724ms/step – loss: 0.3331 – val_loss: 0.2076
Epoch 00013: val_loss improved from 0.21340 to 0.20755, saving model to drive/abc/weights.best6.hdf5
Epoch 14/30
283/283 [==============================] – 218s 771ms/step – loss: 0.3305 – val_loss: 0.2036
Epoch 00014: val_loss improved from 0.20755 to 0.20359, saving model to drive/abc/weights.best6.hdf5
Epoch 15/30
7/283 […………………………] – ETA: 26s – loss: 0.5313283/283 [==============================] – 211s 745ms/step – loss: 0.3295 – val_loss: 0.2006
Epoch 00015: val_loss improved from 0.20359 to 0.20061, saving model to drive/abc/weights.best6.hdf5
Epoch 16/30
283/283 [==============================] – 210s 743ms/step – loss: 0.3272 – val_loss: 0.1982
Epoch 00016: val_loss improved from 0.20061 to 0.19824, saving model to drive/abc/weights.best6.hdf5
Epoch 17/30
6/283 […………………………] – ETA: 27s – loss: 0.3350283/283 [==============================] – 220s 778ms/step – loss: 0.3236 – val_loss: 0.1963
Epoch 00017: val_loss improved from 0.19824 to 0.19628, saving model to drive/abc/weights.best6.hdf5
Epoch 18/30
283/283 [==============================] – 205s 726ms/step – loss: 0.3219 – val_loss: 0.1946
Epoch 00018: val_loss improved from 0.19628 to 0.19460, saving model to drive/abc/weights.best6.hdf5
Epoch 19/30
6/283 […………………………] – ETA: 28s – loss: 0.4911283/283 [==============================] – 215s 761ms/step – loss: 0.3180 – val_loss: 0.1932
Epoch 00019: val_loss improved from 0.19460 to 0.19320, saving model to drive/abc/weights.best6.hdf5
Epoch 20/30
283/283 [==============================] – 215s 761ms/step – loss: 0.3197 – val_loss: 0.1920
Epoch 00020: val_loss improved from 0.19320 to 0.19198, saving model to drive/abc/weights.best6.hdf5
Epoch 21/30
6/283 […………………………] – ETA: 31s – loss: 0.7507283/283 [==============================] – 207s 731ms/step – loss: 0.3188 – val_loss: 0.1910
Epoch 00021: val_loss improved from 0.19198 to 0.19101, saving model to drive/abc/weights.best6.hdf5
Epoch 22/30
283/283 [==============================] – 221s 781ms/step – loss: 0.3201 – val_loss: 0.1901
Epoch 00022: val_loss improved from 0.19101 to 0.19008, saving model to drive/abc/weights.best6.hdf5
Epoch 23/30
7/283 […………………………] – ETA: 27s – loss: 0.6471283/283 [==============================] – 207s 730ms/step – loss: 0.3198 – val_loss: 0.1893
Epoch 00023: val_loss improved from 0.19008 to 0.18925, saving model to drive/abc/weights.best6.hdf5
Epoch 24/30
283/283 [==============================] – 215s 758ms/step – loss: 0.3199 – val_loss: 0.1886
Epoch 00024: val_loss improved from 0.18925 to 0.18856, saving model to drive/abc/weights.best6.hdf5
Epoch 25/30
6/283 […………………………] – ETA: 26s – loss: 0.6787283/283 [==============================] – 218s 769ms/step – loss: 0.3197 – val_loss: 0.1879
Epoch 00025: val_loss improved from 0.18856 to 0.18787, saving model to drive/abc/weights.best6.hdf5
Epoch 26/30
283/283 [==============================] – 206s 727ms/step – loss: 0.3191 – val_loss: 0.1873
Epoch 00026: val_loss improved from 0.18787 to 0.18725, saving model to drive/abc/weights.best6.hdf5
Epoch 27/30
5/283 […………………………] – ETA: 26s – loss: 0.2300283/283 [==============================] – 221s 779ms/step – loss: 0.3175 – val_loss: 0.1868
Epoch 00027: val_loss improved from 0.18725 to 0.18680, saving model to drive/abc/weights.best6.hdf5
Epoch 28/30
283/283 [==============================] – 209s 740ms/step – loss: 0.3186 – val_loss: 0.1865
Epoch 00028: val_loss improved from 0.18680 to 0.18653, saving model to drive/abc/weights.best6.hdf5
Epoch 29/30
5/283 […………………………] – ETA: 28s – loss: 0.1778283/283 [==============================] – 213s 752ms/step – loss: 0.3165 – val_loss: 0.1864
Epoch 00029: val_loss improved from 0.18653 to 0.18639, saving model to drive/abc/weights.best6.hdf5
Epoch 30/30
283/283 [==============================] – 218s 771ms/step – loss: 0.3167 – val_loss: 0.1864
Epoch 00030: val_loss did not improve from 0.18639
so my question is why model.predict() gives constant value always?
Thanks,
Perhaps the model needs to be tuned to your problem?
Thank you so much for this tutorial, right now I am facing some issues which may not be covered here, the problem listed hete are single data or a set of data , but since my network have both batch normalization and lstm later,which have impact on the output result if the batch size is different, particularly for the time serial prediction problem, normally trained the model with large batch size to increase the training speed. After traing , the usage is in real time input pattern, only one data per minute , the problem is how to set the input pattern with batch size in real time such that the impact of the batch minimized.
When a new data come, should I just input one data? Or append it to a large batch set and input a batch or even many batches to the model?
Btw I did read your article about the statefull lstm model. Thank you
I recommend evaluating the performance of the model with a single input vs batches of different sizes to see if it impacts model skill, then use the configuration that works best.
I have a question on text prediction.
Since we are using different approaches to enumerate the text before any model fitting. I face a logical problem here.
Imaging in my train set I have 20 uniq token with I enumerate them like this: (“i”,1)(“love”,2)(“this”,3)… .
After training the model and fitting the data I destroy everything but the model. So I cann’t keep track of this dictionary anymore.
When I want to predict a new sequence that its tokens are completely new things [“we”, “went”, “to”, “Spain”].
How could this new tokens being related to the old dictionary?
how should I enumerate it?
If I use a new dictionary to enumerate this, like: (“we”, 1)(“went”, 2)… . then how model can differentiate that this “We” is different from the “i” in train model?
Thanks in advance!
You must also keep the method used to encode words to integers.
Hi Jason, I don’t understand how program predicts 0 or 1?
Which part exactly?
Great Article. one thing is confusing though. For regression predictions, you used 3 instances in first example and 1 instance in next example, instance in second example is actually first instance in the first example. so why are their results different?
Sorry, I don’t follow. Perhaps you can provide more context?
Because model doesn’t run in exact the same manner everytime unless u save it that’s why.
How to evaluate the model? This code does not work for evaluation as in previous example.
scores = model.evaluate(X, y)
print(“\n%s: %.2f%%” % (model.metrics_names[1], scores[1]*100))
This tutorial is about making predictions.
I recommend evaluating model performing using using a train/test split or using a resampling method such as k-fold cross-validation. I have many tutorials on each.
Perhaps start here:
https://machinelearningmastery.com/faq/single-faq/how-do-i-evaluate-a-machine-learning-algorithm
I mean not about about train/test split, what if i want to just evaluate my model on the same data i used for training?
I used the command
scores = model.evaluate(X, y, verbose=2)
print(scores)
In diabetes example when i print scores,
output is : [0.5047814001639684, 0.7513020833333334]
score[1] here means accuracy of the model
In case of this regression example, when i print scores. I get 0.021803879588842393. What does this data mean? How can i calculate accuracy from this?
If you evaluate a model for regression, the score will be an error score, e.g. the loss chosen to minimize during training. Such as mse.
Oh ok thanks . I think i got it. Accuracy is used only in classification to check whether the model classifies it in correct class or not. But in prediction is in real values so accuracy does not make any sense. Now if someone how accurate is my model, should i give them answer in error score?
We use cross-validation or train/test splits to answer the question: “how accurate is my model?”
We use the model to make predictions on new data, to answer the question: “what is the likely class for this observation?”
Does that help?
Thanks, it does help. I think I need to study deeper on this topic. By the way, your contents on this site are great, covering almost all the problems about Deep Learning using python.
Thanks!
Thanks for the interesting post! Is it really required to also do scaling of the ground truth and if yes why?
It can help during training to scale the output for regression. It depends on the model and the problem.
hi Jason,
may I ask you why you did not do a prediction from a saved h5 file?
without it, we need to run the model every time.
to make it simpler to explain?
thanks! Franco
You can. I tried to keep this tutorial simple.
This tutorial will show you how to save/load your model:
https://machinelearningmastery.com/save-load-keras-deep-learning-models/
Are model.predict(…) and model.predict_proba(…) equivalent if activation in last layer is softmax or sigmoid?
No. I believe predict() will perform an argmax on the predict_proba result to give an integer.
In tensorflow 2.X, predict() also returns probability
Agreed, I believe the previous comment was regarding sklearn. Keras/TF does not have a predict_proba() function.
Is there any way to get classes with probabilities like:
class 1 : 0.99, class 2 : 0.8 etc something like this. I have a project in which I have to show confidence of every class for an input, how to do that?
Yes, mentioned in the above post:
sir, may i get any help of abstractive document summarization using Keras?
Sure, I have some tutorials here:
https://machinelearningmastery.com/start-here/#nlp
Hi Jason How can I get the predicted probability of each test sample? it is possible for one image given ?
You can use model.predict_proba()
Is there any way so that I can print classes with confidence in this. Like I am working on a project in which for an input I have to print classes with confidence in the output.
Yes, for confidence intervals:
https://machinelearningmastery.com/confidence-intervals-for-machine-learning/
For prediction intervals:
https://machinelearningmastery.com/prediction-intervals-for-machine-learning/
Hi,
Thank you so much for explaining it so well. I have a question. Can you please tell us what changes will be required if we want to do the regression for multiple outputs. For example, if we have features X=[x1,x2,x3] and we want to produce output Y=[y1,y2]. we want to produce multiple outputs against each observation.
[x1,x2,x3]>>>[y1,y2].
Thanks
Sure, for a problem that requires predicting 2 values, you will require 2 nodes in the output layer of your network. That’s it.
hi jason
is there any model for hyperspectral images. or any link which give training model for hyperspectral images..
Perhaps try a google search?
Hi, what is the meaning of X and y?
I assume X being an input, y some output. But what is the meaning of upper/lower case writing?
X is a matrix y is a vector.
Hi Jason
Is there a way to get variable / input importance with keras ?
or How do you find which variables are important while training a neural network.
Not that I’m aware.
Hi Jason,
why did you use scalar = MinMaxScaler()?
To normalize the inputs.
Hi,
I am running a CNN to classify images labeled as high or low. When I run predict_proba(img) after just one epoch and predict the results of a set of images all classified the same, I see a series of values for the images that are all very similar to:
[[ 0.49511209]]
[[ 0.49458334]]
[[ 0.49470016]]
After 50 epochs, the validation accuracy is about 95%, and the output of predict_proba(img) is similar to:
[[ 0.80663812]]
[[ 0.97021145]]
[[ 0.96050763]]
where none of the values are below 0.5
Could you please tell me essentially what I’m seeing? That is, how do I know what class the probability is referring to (“high” or “low”); why is the probability all below 0.5 for minimum training and closer to 1 at 50 epochs; and why does the probability rise almost uniformly with more training?
Thank you for your work and service in freely sharing your knowledge.
It suggests for that image that the model is unsure of what it is and strongly believes it belongs to all 3 classes.
Hi, thank you for this response. Those values of accuracy are for three separate images as examples for training at 1 epoch and 50 epochs, respectively. Knowing that, could you please respond to my original questions? Thank you!
I see, the “low” and “high” are mapped to integers 0 and 1, then the predictions will be between 0 and 1 for class 1.
The mapping of strings to ints will be controlled by you, e.g. labelencoder in sklearn, and you can inspect it to discover whether low is 0 or 1.
Does that help?
That does help, thank you! To be sure, when the probability is 0.97, for instance, the predicted class is 1, but when the probability is 0.494, the predicted class is 0?
Sincerely,
David
Yes.
OK, thank you!
For text classification of binary sentiments, positive and negative.
Why are words not found on the model’s vocabulary always give the same prediction value?
For example, when i input a single word ‘extravagant’, which is not found on the vocabulary of the model, it will give me a value of 0.165 that is classified as negative. The same probability is resulted when i enter new single terms that are not found on the vocabulary. What causes these words to be predicted with the probability of 0.165 or the same prediction value?
Unknown words are mapped a zero or “unknown” input.
Hi! Thank you so much for the response Jason.
I’d just like to ask another question. If unknown outputs are mapped zero/unknown, why is it that the output is not zero as well?
Is it due to the model itself? that it generates the prediction 0.165 based on the values its layers always generate when receiving 0 as input?
I suspect because it is unusual to have a single unknown word as input to the model – e.g. it would not happen in practice.
Thank you so much again Jason! I really appreciate the help.
Hello Jason,
Thank you for the great article !
I had a query, you have used Sequential() to create the architecture. How can we predict the values when we have used Model() for the architecture ?
You can use the predict() function on the Model instance.
If you need the class value, you can use something like:
yhat = model.predict()
class_id = argmax(yhat[0])
I build an encoder-decoder model for text summarization based on ‘https://machinelearningmastery.com/encoder-decoder-models-text-summarization-keras/’.
I train the model and now i want to predict a summary based on
a test data set(find the summary for a single document), so i used the same structure for the test data as in the train data. I have a problem with the command model.predict(). Which is the input ?
I tried to use an array, list of numphy arrays, to encode the test data, but nothing. Is there any example for prediction in text summarization ?
The input to the predict function is an array of samples with the same structure/preparation as trainX.
i am working on my project for predicting house price from images,model is created 200 epochs are being scanned and an avg price is being displayed ,now what i want to do is predict the house price with the using four images that is kitchen,bathroom,frontal image and zipcode ,
how shall i apply the input? and how shall i call method.predict(),please can u write me down the code?
Very cool project idea.
Perhaps compare an ensemble of separate models vs a model that takes each photo as input in parallel – e.g. a multi-input model.
Thank you for your effort and excellent post! For the single data, Xnew = array([[0.89337759, 0.65864154]]), do we need to use MinMax scaler to preprocess before we feed it to the model for prediction? This is related to Class Prediction part2 for predicting the class for a single instance. Thanks a lot!
Any new input to the model should be prepared in an identical way to the training data.
I want to ask if I want to use CNN for binary simple non-image classification data without the fully connected layer then how can use.
It would not be appropriate unless the input is a sequence.
Here is an example:
https://machinelearningmastery.com/how-to-develop-convolutional-neural-network-models-for-time-series-forecasting/
Thanks for the tutorial !!
How can I predict images in real time through webcam??
What do you mean by “predict images”?
I have my own dataset and images and label file, How could I load them to Keras and feed them to network?
thanks for this tutorial
This is a good tutorial for getting started, that you can adapt for your problem:
https://machinelearningmastery.com/tutorial-first-neural-network-python-keras/
Hi jason..
I saw you are using keras. Above codes are using BP algorithm for optimizing weights or not at the back end that we can’t see in code? Is it possible
Keras uses backpropagation to update model weights.
I have multiple classes how can i make probabilities for each class?
model.predict()
I run the multichannel cnn model on a text similarity problem.
Every time I run the model, I have different results(loss, accuracy).
Does it is normal to have different loss and accuracy results every time I run the code?
This is to be expected, see this:
https://machinelearningmastery.com/faq/single-faq/why-do-i-get-different-results-each-time-i-run-the-code
model.predict() it gives me an array of 0 and 1 and not the probabilities.
Perhaps confirm you are using a sigmoid activation function and a cross entropy loss.
What is the data type of output of model.predict()? My training dataset has float64 data type. But the model.predict gave me float32 output. Do Keras stores learned weights and biases in float32 format?
Thank you.
Typically it is float32 as far as I know.
So is there no way to make the model predict float64 type?
I don’t see why not.
Hi Jason,
Why loss and accuracy values changes very time i run the model. however, I upload the training, validation, and the testing dataset
This is a common question that I answer here:
https://machinelearningmastery.com/faq/single-faq/why-do-i-get-different-results-each-time-i-run-the-code
Hi this might be stupid question but I want to know what is the difference between the Sequential model from keras and creating an autoencoder for the same prediction problem. I am just looking for a simple or clear definition of both of them. keras documentation does not say much about this sequential model. thanks in advance.
A prediction model maps an input to a given output, e.g. numeric or class label.
An antoencoder provides a compressed representation of an input, e.g. a projection.
Hi, Mr Jason.
How are you?
I have one problem.
I have built model for OCR but i can’t get probability result
In building model, i have softmax as a activation function.
I tried to get the probability result to use prediction function but i had not.
Could you explain this?
Call model.predict() to get probability predictions.
What problem are you having exactly?
thank you for answering
by the way my training result is [0.0,0.0,0.0,0.0,1.0,0.0,0.0,0.0,0.0]
but i want probability result like [0.01,0.03, 0.91,0.07,0.10, …]
i use model.predict() function now.
but result is not probability value.
i want how to get probability value
Kind regards.
thank you sir.
Perhaps you are not using an appropriate loss function to train the model or activation function in the output layer of the model?
Perhaps this will help:
https://machinelearningmastery.com/how-to-choose-loss-functions-when-training-deep-learning-neural-networks/
Thank you for your kindness.
You’re welcome.
Hi Jason,
The sklearn classifiers uses target names as strings (Good, Bad) but the keras sklearn modelling requiring to map as (0,1), is there a way to use as string itself, this is to match with the LIME explanation from the keras model.
No, typically you must map the integers to strings yourself with some custom code.
Thanks Jason,
Keras Sequential Model returns probability of one class as below
pred = Keras_model.predict_proba(testData_for_model)
pred
Out[73]: array([[0.6559619]], dtype=float32)
pre.argmax(axis=-1)[0] gives class 0
so I have to code 1-pred as class 1 probability
Is this way it works
One sample is used to make one prediction.
You can convert probabilities to clasess via argmax or call predict_classes() directly.
Thanks Jason, I am bit confused finding which class belongs to from the following,
keras_model.predict_proba(test_for_model)
Out[30]: array([[0.77143025]], dtype=float32)
keras_model.predict_classes(test_for_model)
Out[32]: array([[1]])
keras_model.predict_proba(test_for_model).argmax(axis=-1)
Out[33]: array([0], dtype=int64)
When I call predict_classes it says 1 and with argmax it says 0, may I know which class it belongs to please
An argmax on a single element vector is invalid.
Instead, you must round the result, which be class 1.
hi Jason, as always i find your tutorials best for me to learn and get better understanding! thx! for a regression system, can you please explain how to actually extract the weights and get real (not scaled ) predictions values?
Thanks.
You can fit the model on scaled data, then invert the scaling on the predicted values – this is common.
Hi dear Jason. Thanks for your good site and contents which helps us to start coding machine learning model.
I have a dataset of some activities. The dataset contains the status of different sensors and the label of activity. T trained a model in Keras with the following architecture which models the activities.
model = Sequential()
model.add(Embedding(max_features+1, embedding_vector_dim))
model.add(LSTM(64, dropout=0.2, recurrent_dropout=0.2, return_sequences = True))
model.add(Dense(1, activation=’sigmoid’))
Now, I need to make a different LSTM-based model for each activity separately. Then, calculate the probability of creating the sequence by each model. Can I do that with Keras? How should I design the architecture of the model? Is it right to remove the dense layer and then create a separate model for each activity label separately?
I believe so.
Sorry, I don’t have the capacity to review/design a custom model for you. Perhaps start with some of the free tutorials here:
https://machinelearningmastery.com/start-here/#deep_learning_time_series
I would like to know when I give a number of test samples for the prediction to model.predict(), does the model takes each sample sequentially? Or does the model takes all samples at a time and give the predicted output? Is there a way to make the prediction in a vectorized manner? Thank you.
Yes, input examples are processed sequentially.
That means the first prediction will be for the first row of input.
Does that help?
hie Jason. thanks for the tutorial. I managed to make the model (model.yaml /model.h5) from the previous tutorial. Now how do i use this file to predict the output when i enter new data?
Load the model and call predict()
thank you.as an example is this correct?
from keras.models import load_model
model = load_model(‘model.h5’)
print(model.predict([1,0,0,1,0,5849,0,200,360,1,0]))
Looks like a good start.
I have a question. I want to make a prediction, but it is not based on numbers, but on text data. Is there an effective way to do it?
Input is date, time, location. Output is hashtag 1 to 35. There are thousands of possible hashtags.
The training file has tens of thousands of dates, times, locations and fields of 1-35 hashtags.
Do I need to convert everything to numbers in order to make the regression? Or can the nltk help me somehow?
I can do regression with numbers, but how do I use text?
Thank you so much for your help.
Sounds like a classification task, where the input data will have to be converted to numbers.
For text data, you can start with something simple like a bag of words representation.
I have tons of tutorials to help you get started:
https://machinelearningmastery.com/start-here/#nlp
Hi Jason,
Your work, books and posts have made great impact on Deep Learning understanding across CS enthusiasts, scientists and researchers. Awesome! Thanks
Currently I work on robot inverse kinematics which solution will be approximate by neural network (NN). Data set comes from forward kinematics. My data set consists of 50000 records (each record consist of 7 values ) – 7 Inputs (position of end effector X,Y,Z and orientation *4 = 7). Outputs from NN are motor/robot angles => 6. I defined many different architectures (flat/deep/broad and narrow) but still there is a issue with quality (the quality of prediction of angles are unfortunately quiet poor MSE = 0.3).
I suppose that the problem is directly connected with architecture of NN. It will be great if you have a chance and can give me some tips how to improve prediction (change the NN architecture or something more).
Details:
INPUT = 7 (x,y,x + orientation*4 )
OUTPUT = 6 robot motor angles
I use sequential NN from Keras. Neurons are configured as follow (deployed NN architecture) : 7 – 32 -128 – 256 – 256 -128 – 32 – 6. I tried also to increase the hidden layers or made the NN totally flat but without positive result.
I used also (for example) 7-256-512-1024-1024-1024-512-256-6 and other variants but without succeed (batch_size = from 32 to 512; epoch from 1000 to 500. The dataset is pre-proceeded – I used the MinMaxScaler (0,1). Neurons have a RELU activation function
I wonder Jason if you can help me, please?
Thanks.
Best regards,
Markus Buchholz
Thanks Markus!
I believe the tutorials here will help in the general sense to diagnose the learning dynamics of a given model and suggest improvements:
https://machinelearningmastery.com/start-here/#better
Awesome. Thanks and good luck!
Thanks.
Hi, Jason.
if there is a way to output prediction values as integers only (not float).
You can call predict_classes() to get the class integers.
Hello, sir. I’d like to make a crop recommendation. There are many kinds of crops, about soil characteristics (pH, N, P, K…). Recommend crops by forecasting the yield of various crops.But I don’t know how to implement it with keras. Can you give me some ideas?
Perhaps this process will help as a first step:
https://machinelearningmastery.com/start-here/#process
Hi, thanks for this, so i have a question, how can i get 2 or more outputs with this example? and then, how can i print this outputs?
You can provide an array with two input samples to make two predictions.
You can print predictions by using the print() function.
Thank you I really appreciate your step-by-step approach. I’ve been taking quite many courses on deeplearning already I know a lot of theories but how to apply them there are not so many articles are as clear as yours.
Keep the good work!
Thanks!
Hi Jason,
Great post! I have been following several of your posts and they are all great!
One question about “predict_proba(..)”: is it available for a VGG model? It seems that it does not exist. If I just use “predict(..)”, it only returns an array like “[[7.090991e-05]]”. Is this the probability for class 1 (and thus the probability for class 0 is 7.090991e-05)?
Calling predict() on a classification model will return probabilities.
Thanks for the tutorial! I built the DNN model to predict the survivability of breast cancer patients (Died(1) or survived (0) ), how to predict the class label (0 or 1) for new cases? “without the training process directly” just I want to insert features value and predict it using DNN model?
You’re welcome!
You can create an array and pass it to the predict() function. The above tutorial shows you how.
Xnew = array([[1,2,3,4,5,6,7,8,9,10,11,12,13,14,15,16,17,18,19,20,21,22,23,
24,25,26,27,28,29,30,31,32,33]])
ynew = model.predict_classes(Xnew)
I have 33 features, and I want to predict new cases (0 or 1) after fitting the model!
But show this error!
Error when checking input: expected input_2 to have shape (33,) but got array with shape (2,)
That is surprising, perhaps double check the expectation of your model.
Hello Mr. Jason,
thanks for your great support.
I have one query. When I calculate confusion matrix for binary classification using sigmoid, I get wrong confusion matrix with 50 percent accuracy. My code is:
y_true = test_it.classes
Y_pred = model.predict(test_it, STEP_SIZE_TEST)
Y_pred = model.predict(test_it, STEP_SIZE_TEST)
y_pred1 = np.argmax(Y_pred, axis=1, out=None)
cm = confusion_matrix(y_true, y_pred1)
One possible solution I found was
y_pred1 = np.where(Y_pred>=0.5, 1, 0)
However, this is also not matching with the test accuracy though its very close to it.
kindly guide on this issue.
Thanks and Regards
NKM
The cause of your issue is not obvious to me, sorry.
Hi Jason,
Thanks for your great support.
I would like to ask for binary classification of Gray-scale images, what should be the activation function in the last layer, sigmoid or softmax?. Theoretically both should give the same results but practically both are not matching. Even test accuracy differs. Kindly guide.
sigmoid.
thanks for quick reply. I observed the same thing.
Further for this case, during prediction I used
predictedClasses = np.where(predictions>0.5, 1, 0) instead of np.argmax(Y_pred, axis=1) since in this case np.argmax will always output 0 as it is a column vector.
np.where(predictions>0.5, 1, 0) returns 1 if prediction > 0.5 else returns 0.
However, the accuracy through this approach (np.where) does not match (variation around 2-3%) to the accuracy from model.evaluate step.
Kindly guide.
argmax is a good default to use:
https://machinelearningmastery.com/argmax-in-machine-learning/
Otherwise you can use threshold moving:
https://machinelearningmastery.com/threshold-moving-for-imbalanced-classification/
Hi Jason,
I have developed a beautiful time series prediction model based on https://machinelearningmastery.com/multivariate-time-series-forecasting-lstms-keras/.
Now my inexperience in machine learning shows: I saved the model and then wanted to make a prediction based on 2 rows of new data.
“yhat = model.predict(X)”. The result is scaled, right? So I wanted to inverse that by “scaler.inverse_transform(yhat)”.
I get a “ValueError: non-broadcastable output operand with shape (2,1) doesn’t match the broadcast shape (2,8)”.
That operand is ‘yhat’, and the expected shape seems to expect all features (count 8) for the inverse_transform function.
I believe that I am missing something basic, but I don’t know how to fill the gap. Maybe I missed that point in your books and other posts …
Thanks again!
Valentin
Good question.
To use the transform object to invert scaling, the input to the transform object must have the same shape, e.g. same columns in the same order as when the transform was performed.
Good morning,
I wanted to know how to reprocess and have predictions for data outside the range 0-1,
A solution regarding the activation function is to use the “relu” function, but anyway, and I don’t understand why, the prediction values are still equal to 1.
You can use a linear output for your model, then write code to scale the output to any range you require.
Hi Jason,
Great article! I am using your code with my own dataset, but I have about 70 inputs. The predicted probabilities are much lower than they should be, and I am wondering if you have any suggestions on how to fix this. I am new to neural networks, and I think I may need to change the architecture a fair amount.
Thanks!
Yes, here are some suggestions for improving the performance of a neural net:
https://machinelearningmastery.com/start-here/#better
why I am getting one output with predict_proba?
X=[0.98656553]
given that I need both values because I need them as input for a function
If you provide one sample as input, you will get one output.
I have an estimator that returns two values
(predicted_labels, log_probs) = create_model( is_predicting, input_ids, input_mask, segment_ids, label_ids, num_labels)
the log_probs should return the probability of each class why I got this result
array([-6.2299333e+00, -1.9715650e-03], dtype=float32)
They are small values close to zero.
yes but I was expected to retrieve the probability of each class [0.70, 0.30] as examples, in which their sum gives 1…
You will only get the probability for each class if you use a softmax activation in the output layer – e.g. for multiclass classificaton, not binary classificaiton.
Sir, How we can use ELM and F score for lie detection? Can you please explain or help me in this
Perhaps this process will help you work through your project:
https://machinelearningmastery.com/start-here/#process
Hello Jason,
I have a problem. I have trained 1d-CNN for regression purposes. I have used sigmoid activation function for my output dense layer to bound the results between 0 and 1. But when I want to do the prediction, my model gives accurate results only on the first try. When I use it for the second time the accuracy decreases. for each time I run it next the accuracy falls in a pattern (maybe 2 or 3 percent).
I have used seeding when I wanted to train my model.
Any idea what is the source of problem?? Thank you
Sigmoid activation is not appropriate in the output layer for a regression problem, you must use linear.
Also, accuracy is not appropriate metric for regression, you must use error, like RMSE or MAE.
Thanks for your reply. I changed the activation function. but still every time I run the model on the same inputs the results get worst and worst (this time I tested that using R2 and RMSE). only my first run on the model I get good results.
thanks
Perhaps try an alternate data preparation, alternate model configuration or alternate model.
This is so useful. I was wondering if its possible to calculate the F1 score, precision, and recall from the examples you present here. I’m reproducing your example with my data, but I’ve been struggling to figure out how to obtain these values. I wonder If you could provide a suggestion to this.
Kind regards,
Sure, see this:
https://machinelearningmastery.com/how-to-calculate-precision-recall-f1-and-more-for-deep-learning-models/
what kind of neural network that you use? deep neural network or artificial neural network? i dont know the difference from dnn and ann
There is no difference.
I used your code for predictions and every time i run the code i get a different prediction for the same input data, how could i solve this?
Good question, see this:
https://machinelearningmastery.com/faq/single-faq/why-do-i-get-different-results-each-time-i-run-the-code
Hi Jason,
I am using keras and tensorflow versions 2.6.0 and I am getting the following error when doing ‘predict_classes’: AttributeError: ‘Sequential’ object has no attribute ‘predict_classes’. Do you know a way to fix this?
In that case, you need to use argmax with the predict result, such as np.argmax(y_pred, axis=1)
hi, i wanna ask you, can we predict the nan / missing value data? if can, how to predict nan/missing value data, thanks
Technically cannot. But if you mean to collect data from somewhere and you want to predict if you will see nan or missing values, then it is a binary classification problem. In that case, you can build a model to predict it
ok adrian, I mean it’s possible to train neural networks with missing data? because I’ve already tried and the result shows loss and the result is also nan
Neural networks are based on differentiable functions and matrix multiplications. Hence no NaN value should be input to the neural network. You either substitute them with some value, or not to use neural network for that.
Ok, Adrian thanks for your answer, i’ve already run my neural network with ratio of training and testing data is 0.1 (training data) and 0.9 (testing data) and the result showed coefficient correlation is 0.9. Why it can be happen? because all of said if you have a little of amount training data the result must be bad
Not must be bad, but more likely to be bad. In your case, maybe you just being lucky (or the nature of the dataset is beautifully fit).
To understand what happened, it is useful to think in linear regression (the simplest machine learning model) – if all points are on the same line perfectly, you just need two point from the dataset and you get a perfect model.
if we want to predict some NaN values, should we use training data with no NaN values?. Or we can use training data with NaN values?
Make it a binary classification model of whether it is NaN or not.
Thank you for this article Jason.
I am wondering whether it is it possible to print both the prediction that the model makes, as well as the probability that this prediction is achieved.
Say for example the prediction from the model will be an integer between 75 – 251 – how could I then see the probability of this outcome?
To generate this initial prediction (75 – 251) the output layer has 1 neuron and no sigmoid/softmax function.
With there being no sigmoid/softmax function on the last layer it is not normalised to make probability predictions. However, if I do add these, them I’m unable to make the initial prediction.
I would have guessed it would have been a combination of predict_ and predict_proba, but I have confused myself and I’m not even sure if this is possible?
Any advice/guidance is much appreciated.
Thank you
Dummy example below:
model1 = Sequential()
model1.add(Dense(250, input_dim=5))
model1.add(BatchNormalization())
model1.add(Activation(‘relu’))
model1.add(Dense(1))
model1.compile(loss=’mse’, optimizer=’Adam’)
model1.fit(X, y, validation_split=0.25, epochs=10, batch_size=32, verbose=1)
Xnew = array([[118,1,1,0,0]])
ynew = model1(Xnew)
print(ynew)
output: 158.35785
How do you define the probability in this case?
Hi Adrian. The probability I would like to define is the likelihood that the output 158.35785 occurs. Is it possible for a model to make the prediction (of 158.35785 in this case) as well as then predict the probability that this occurs?
I would say the probably is zero. That’s the nature of any continuous variable. But even so, unlike linear regression, neural network does not give you the error margin to its result naturally.
i’ve already tried and the result shows still NaN value, i cannot predict the NaN values
this message is reply for your message “Make it a binary classification model of whether it is NaN or not.” Before your message, i asked “if we want to predict some NaN values, should we use training data with no NaN values?. Or we can use training data with NaN values?”
Hello Adrian Tam,
thank you for such great content,
I am working on a binary classification problem (IDS with normal and malicious packets), I am using sequential
model from keras. My question is how can I get the probability prediction for both classes in each single sample for example, a function like:
predict (x),
that returns–> [0: (probability for class 0), 1: (probability for class 1)]
You are very welcome Fatimah! Please let us know if you have any questions we can help you with!
Thank you,
I am working on a binary classification problem (IDS with normal and malicious packets), I am using sequential
model from keras. My question is how can I get the probability prediction for both classes in each single sample for example, a function like:
predict (x),
that returns–> [0: (probability for class 0), 1: (probability for class 1)]
Hi @Jason!
First of all, thank you very much for all your amazing posts!!
My question is: when your are going to predict a model that has been previosly trained (the final selected model), the input data to predict the output variable has to be preprocessed in the same way that the data used to train (clean outliers, selected important features…)?
Thank you very much!
Hi AmBG…You are very welcome! The following resource may be of interest to you:
https://machinelearningmastery.com/what-is-generalization-in-machine-learning/