Long Short-Term Networks or LSTMs are a popular and powerful type of Recurrent Neural Network, or RNN.
They can be quite difficult to configure and apply to arbitrary sequence prediction problems, even with well defined and “easy to use” interfaces like those provided in the Keras deep learning library in Python.
One reason for this difficulty in Keras is the use of the TimeDistributed wrapper layer and the need for some LSTM layers to return sequences rather than single values.
In this tutorial, you will discover different ways to configure LSTM networks for sequence prediction, the role that the TimeDistributed layer plays, and exactly how to use it.
After completing this tutorial, you will know:
- How to design a one-to-one LSTM for sequence prediction.
- How to design a many-to-one LSTM for sequence prediction without the TimeDistributed Layer.
- How to design a many-to-many LSTM for sequence prediction with the TimeDistributed Layer.
Kick-start your project with my new book Long Short-Term Memory Networks With Python, including step-by-step tutorials and the Python source code files for all examples.
Let’s get started.
- Update Jun/2019: It seems that the Dense layer can now directly support 3D input, perhaps negating the need for the TimeDistributed layer in this example (thanks Nick).
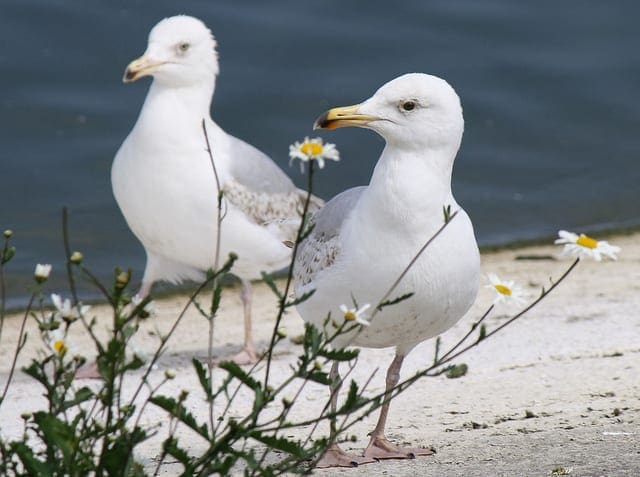
How to Use the TimeDistributed Layer for Long Short-Term Memory Networks in Python
Photo by jans canon, some rights reserved.
Tutorial Overview
This tutorial is divided into 5 parts; they are:
- TimeDistributed Layer
- Sequence Learning Problem
- One-to-One LSTM for Sequence Prediction
- Many-to-One LSTM for Sequence Prediction (without TimeDistributed)
- Many-to-Many LSTM for Sequence Prediction (with TimeDistributed)
Environment
This tutorial assumes a Python 2 or Python 3 development environment with SciPy, NumPy, and Pandas installed.
The tutorial also assumes scikit-learn and Keras v2.0+ are installed with either the Theano or TensorFlow backend.
For help setting up your Python environment, see the post:
Need help with LSTMs for Sequence Prediction?
Take my free 7-day email course and discover 6 different LSTM architectures (with code).
Click to sign-up and also get a free PDF Ebook version of the course.
TimeDistributed Layer
LSTMs are powerful, but hard to use and hard to configure, especially for beginners.
An added complication is the TimeDistributed Layer (and the former TimeDistributedDense layer) that is cryptically described as a layer wrapper:
This wrapper allows us to apply a layer to every temporal slice of an input.
How and when are you supposed to use this wrapper with LSTMs?
The confusion is compounded when you search through discussions about the wrapper layer on the Keras GitHub issues and StackOverflow.
For example, in the issue “When and How to use TimeDistributedDense,” fchollet (Keras’ author) explains:
TimeDistributedDense applies a same Dense (fully-connected) operation to every timestep of a 3D tensor.
This makes perfect sense if you already understand what the TimeDistributed layer is for and when to use it, but is no help at all to a beginner.
This tutorial aims to clear up confusion around using the TimeDistributed wrapper with LSTMs with worked examples that you can inspect, run, and play with to help your concrete understanding.
Sequence Learning Problem
We will use a simple sequence learning problem to demonstrate the TimeDistributed layer.
In this problem, the sequence [0.0, 0.2, 0.4, 0.6, 0.8] will be given as input one item at a time and must be in turn returned as output, one item at a time.
Think of it as learning a simple echo program. We give 0.0 as input, we expect to see 0.0 as output, repeated for each item in the sequence.
We can generate this sequence directly as follows:
1 2 3 4 |
from numpy import array length = 5 seq = array([i/float(length) for i in range(length)]) print(seq) |
Running this example prints the generated sequence:
1 |
[ 0. 0.2 0.4 0.6 0.8] |
The example is configurable and you can play with longer/shorter sequences yourself later if you like. Let me know about your results in the comments.
One-to-One LSTM for Sequence Prediction
Before we dive in, it is important to show that this sequence learning problem can be learned piecewise.
That is, we can reframe the problem into a dataset of input-output pairs for each item in the sequence. Given 0, the network should output 0, given 0.2, the network must output 0.2, and so on.
This is the simplest formulation of the problem and requires the sequence to be split into input-output pairs and for the sequence to be predicted one step at a time and gathered outside of the network.
The input-output pairs are as follows:
1 2 3 4 5 6 |
X, y 0.0, 0.0 0.2, 0.2 0.4, 0.4 0.6, 0.6 0.8, 0.8 |
The input for LSTMs must be three dimensional. We can reshape the 2D sequence into a 3D sequence with 5 samples, 1 time step, and 1 feature. We will define the output as 5 samples with 1 feature.
1 2 |
X = seq.reshape(5, 1, 1) y = seq.reshape(5, 1) |
We will define the network model as having 1 input with 1 time step. The first hidden layer will be an LSTM with 5 units. The output layer with be a fully-connected layer with 1 output.
The model will be fit with efficient ADAM optimization algorithm and the mean squared error loss function.
The batch size was set to the number of samples in the epoch to avoid having to make the LSTM stateful and manage state resets manually, although this could just as easily be done in order to update weights after each sample is shown to the network.
The complete code listing is provided below:
1 2 3 4 5 6 7 8 9 10 11 12 13 14 15 16 17 18 19 20 21 22 23 24 25 |
from numpy import array from keras.models import Sequential from keras.layers import Dense from keras.layers import LSTM # prepare sequence length = 5 seq = array([i/float(length) for i in range(length)]) X = seq.reshape(len(seq), 1, 1) y = seq.reshape(len(seq), 1) # define LSTM configuration n_neurons = length n_batch = length n_epoch = 1000 # create LSTM model = Sequential() model.add(LSTM(n_neurons, input_shape=(1, 1))) model.add(Dense(1)) model.compile(loss='mean_squared_error', optimizer='adam') print(model.summary()) # train LSTM model.fit(X, y, epochs=n_epoch, batch_size=n_batch, verbose=2) # evaluate result = model.predict(X, batch_size=n_batch, verbose=0) for value in result: print('%.1f' % value) |
Running the example first prints the structure of the configured network.
We can see that the LSTM layer has 140 parameters. This is calculated based on the number of inputs (1) and the number of outputs (5 for the 5 units in the hidden layer), as follows:
1 2 3 4 |
n = 4 * ((inputs + 1) * outputs + outputs^2) n = 4 * ((1 + 1) * 5 + 5^2) n = 4 * 35 n = 140 |
We can also see that the fully connected layer only has 6 parameters for the number of inputs (5 for the 5 inputs from the previous layer), number of outputs (1 for the 1 neuron in the layer), and the bias.
1 2 3 |
n = inputs * outputs + outputs n = 5 * 1 + 1 n = 6 |
1 2 3 4 5 6 7 8 9 10 11 |
_________________________________________________________________ Layer (type) Output Shape Param # ================================================================= lstm_1 (LSTM) (None, 1, 5) 140 _________________________________________________________________ dense_1 (Dense) (None, 1, 1) 6 ================================================================= Total params: 146.0 Trainable params: 146 Non-trainable params: 0.0 _________________________________________________________________ |
The network correctly learns the prediction problem.
1 2 3 4 5 |
0.0 0.2 0.4 0.6 0.8 |
Many-to-One LSTM for Sequence Prediction (without TimeDistributed)
In this section, we develop an LSTM to output the sequence all at once, although without the TimeDistributed wrapper layer.
The input for LSTMs must be three dimensional. We can reshape the 2D sequence into a 3D sequence with 1 sample, 5 time steps, and 1 feature. We will define the output as 1 sample with 5 features.
1 2 |
X = seq.reshape(1, 5, 1) y = seq.reshape(1, 5) |
Immediately, you can see that the problem definition must be slightly adjusted to support a network for sequence prediction without a TimeDistributed wrapper. Specifically, output one vector rather build out an output sequence one step at a time. The difference may sound subtle, but it is important to understanding the role of the TimeDistributed wrapper.
We will define the model as having one input with 5 time steps. The first hidden layer will be an LSTM with 5 units. The output layer is a fully-connected layer with 5 neurons.
1 2 3 4 5 6 |
# create LSTM model = Sequential() model.add(LSTM(5, input_shape=(5, 1))) model.add(Dense(length)) model.compile(loss='mean_squared_error', optimizer='adam') print(model.summary()) |
Next, we fit the model for only 500 epochs and a batch size of 1 for the single sample in the training dataset.
1 2 |
# train LSTM model.fit(X, y, epochs=500, batch_size=1, verbose=2) |
Putting this all together, the complete code listing is provided below.
1 2 3 4 5 6 7 8 9 10 11 12 13 14 15 16 17 18 19 20 21 22 23 24 25 |
from numpy import array from keras.models import Sequential from keras.layers import Dense from keras.layers import LSTM # prepare sequence length = 5 seq = array([i/float(length) for i in range(length)]) X = seq.reshape(1, length, 1) y = seq.reshape(1, length) # define LSTM configuration n_neurons = length n_batch = 1 n_epoch = 500 # create LSTM model = Sequential() model.add(LSTM(n_neurons, input_shape=(length, 1))) model.add(Dense(length)) model.compile(loss='mean_squared_error', optimizer='adam') print(model.summary()) # train LSTM model.fit(X, y, epochs=n_epoch, batch_size=n_batch, verbose=2) # evaluate result = model.predict(X, batch_size=n_batch, verbose=0) for value in result[0,:]: print('%.1f' % value) |
Running the example first prints a summary of the configured network.
We can see that the LSTM layer has 140 parameters as in the previous section.
The LSTM units have been crippled and will each output a single value, providing a vector of 5 values as inputs to the fully connected layer. The time dimension or sequence information has been thrown away and collapsed into a vector of 5 values.
We can see that the fully connected output layer has 5 inputs and is expected to output 5 values. We can account for the 30 weights to be learned as follows:
1 2 3 |
n = inputs * outputs + outputs n = 5 * 5 + 5 n = 30 |
The summary of the network is reported as follows:
1 2 3 4 5 6 7 8 9 10 11 |
_________________________________________________________________ Layer (type) Output Shape Param # ================================================================= lstm_1 (LSTM) (None, 5) 140 _________________________________________________________________ dense_1 (Dense) (None, 5) 30 ================================================================= Total params: 170.0 Trainable params: 170 Non-trainable params: 0.0 _________________________________________________________________ |
The model is fit, printing loss information before finalizing and printing the predicted sequence.
The sequence is reproduced correctly, but as a single piece rather than stepwise through the input data. We may have used a Dense layer as the first hidden layer instead of LSTMs as this usage of LSTMs does not take much advantage of their full capability for sequence learning and processing.
1 2 3 4 5 |
0.0 0.2 0.4 0.6 0.8 |
Many-to-Many LSTM for Sequence Prediction (with TimeDistributed)
In this section, we will use the TimeDistributed layer to process the output from the LSTM hidden layer.
There are two key points to remember when using the TimeDistributed wrapper layer:
- The input must be (at least) 3D. This often means that you will need to configure your last LSTM layer prior to your TimeDistributed wrapped Dense layer to return sequences (e.g. set the “return_sequences” argument to “True”).
- The output will be 3D. This means that if your TimeDistributed wrapped Dense layer is your output layer and you are predicting a sequence, you will need to resize your y array into a 3D vector.
We can define the shape of the output as having 1 sample, 5 time steps, and 1 feature, just like the input sequence, as follows:
1 |
y = seq.reshape(1, length, 1) |
We can define the LSTM hidden layer to return sequences rather than single values by setting the “return_sequences” argument to true.
1 |
model.add(LSTM(n_neurons, input_shape=(length, 1), return_sequences=True)) |
This has the effect of each LSTM unit returning a sequence of 5 outputs, one for each time step in the input data, instead of single output value as in the previous example.
We also can use the TimeDistributed on the output layer to wrap a fully connected Dense layer with a single output.
1 |
model.add(TimeDistributed(Dense(1))) |
The single output value in the output layer is key. It highlights that we intend to output one time step from the sequence for each time step in the input. It just so happens that we will process 5 time steps of the input sequence at a time.
The TimeDistributed achieves this trick by applying the same Dense layer (same weights) to the LSTMs outputs for one time step at a time. In this way, the output layer only needs one connection to each LSTM unit (plus one bias).
For this reason, the number of training epochs needs to be increased to account for the smaller network capacity. I doubled it from 500 to 1000 to match the first one-to-one example.
Putting this together, the full code listing is provided below.
1 2 3 4 5 6 7 8 9 10 11 12 13 14 15 16 17 18 19 20 21 22 23 24 25 26 |
from numpy import array from keras.models import Sequential from keras.layers import Dense from keras.layers import TimeDistributed from keras.layers import LSTM # prepare sequence length = 5 seq = array([i/float(length) for i in range(length)]) X = seq.reshape(1, length, 1) y = seq.reshape(1, length, 1) # define LSTM configuration n_neurons = length n_batch = 1 n_epoch = 1000 # create LSTM model = Sequential() model.add(LSTM(n_neurons, input_shape=(length, 1), return_sequences=True)) model.add(TimeDistributed(Dense(1))) model.compile(loss='mean_squared_error', optimizer='adam') print(model.summary()) # train LSTM model.fit(X, y, epochs=n_epoch, batch_size=n_batch, verbose=2) # evaluate result = model.predict(X, batch_size=n_batch, verbose=0) for value in result[0,:,0]: print('%.1f' % value) |
Running the example, we can see the structure of the configured network.
We can see that as in the previous example, we have 140 parameters in the LSTM hidden layer.
The fully connected output layer is a very different story. In fact, it matches the one-to-one example exactly. One neuron that has one weight for each LSTM unit in the previous layer, plus one for the bias input.
This does two important things:
- Allows the problem to be framed and learned as it was defined, that is one input to one output, keeping the internal process for each time step separate.
- Simplifies the network by requiring far fewer weights such that only one time step is processed at a time.
The one simpler fully connected layer is applied to each time step in the sequence provided from the previous layer to build up the output sequence.
1 2 3 4 5 6 7 8 9 10 11 |
_________________________________________________________________ Layer (type) Output Shape Param # ================================================================= lstm_1 (LSTM) (None, 5, 5) 140 _________________________________________________________________ time_distributed_1 (TimeDist (None, 5, 1) 6 ================================================================= Total params: 146.0 Trainable params: 146 Non-trainable params: 0.0 _________________________________________________________________ |
Again, the network learns the sequence.
1 2 3 4 5 |
0.0 0.2 0.4 0.6 0.8 |
We can think of the framing of the problem with time steps and a TimeDistributed layer as a more compact way of implementing the one-to-one network in the first example. It may even be more efficient (space or time wise) at a larger scale.
Further Reading
Below are some resources and discussions on the TimeDistributed layer you may like to dive in into.
- TimeDistributed Layer in the Keras API
- TimeDistributed code on GitHub
- The difference between ‘Dense’ and ‘TimeDistributedDense’ of ‘Keras’ on StackExchange
- When and How to use TimeDistributedDense on GitHub
Summary
In this tutorial, you discovered how to develop LSTM networks for sequence prediction and the role of the TimeDistributed layer.
Specifically, you learned:
- How to design a one-to-one LSTM for sequence prediction.
- How to design a many-to-one LSTM for sequence prediction without the TimeDistributed Layer.
- How to design a many-to-many LSTM for sequence prediction with the TimeDistributed Layer.
Do you have any questions?
Ask your questions in the comments below and I will do my best to answer them.
Hi, Jason, nice article on TimeDistributed layer!
Basically, there’re three configurations for X (and thus y):
1. (5,1,1) – 5 batchs, 1 time step, 1 feature/step – result shape (5,1)
2. (1,5,1) – 1 batch, 5 time steps, 1 feature/step – result shape (1,5)
3. (1,1,5) – 1 batch, 1 time step, 5 features/step
in article, you discussed previous 2 configures.
I did experiment of config 3, result same shape (1, 5) as 2 does, ’cause X input only 1 batch (which contains 1 sample, which has 5 features.) this config surely lost time information.
3 differ from 2 in two ways:
1) how we/model frame the problem: sequence should be framed as multi time steps as 2
2) different number of LSTM params: config 2 has 140, while config 3 has 220! (big input vector)
Q:
in section ‘many to one without TimeDistributed’, with config 2, you said “The time dimension or sequence information has been thrown away and collapsed into a vector of 5 values.” — that surprise me a little bit.
– does that mean, for seq-to-seq problem, we should always use TimeDistributed?
– what situation suites config 2 (samples, multi-time-steps, features)?
I guess for sequence-to-vector problem (predict one target one time step), config 2 is fine. But for sequence-to-sequence problem discussed here, config 2 is not the right choice, go TimeDistributed.
Very nice, yes I agree.
Generally, we must model sequences as time steps. BPTT will use the sequence data to estimate the gradient. LSTMs have memory, but we cannot rely on them to remember everything (e.g. sequence length of 1).
We can configure an MLP or LSTM to output a vector. For an LSTM, if we output a vector of n values for one time step, each output is considered by the LSTM as a feature, not a time step. Thus it is a many-to-one architecture. The vector may contain timesteps, but the LSTM is not outputting time steps, it is outputting features.
This is no more or less valid, it may require more weights and may give better or worse performance.
Does that make sense?
Any recommendations when facing a one-to-many problem?
They often need more training than you think and consider using bidirectional inputs and regularization on input connections.
This post is great! Thanks for being about the only person to actually explain simply what the TimeDistributed wrapper is doing.
I tried it out with audio vocal data to attempt generation of new speech. I’d previously got basic results with a plain Dense layer on the output.
With the TimeDistributed the network of lstms learned fast. But the result was just to return a rough version of the seed data inputted during generation. This appears to be modelling the equality function, when what I expected was something resembling the sequence following the seed.
My X input is an array of batches, timesteps, and vocal properties. Just a longer version of your example. My y output for measuring error is effectively the same data, just one timestamp later for each batch (time sequence).
Since your examples are for equality modelling, it’s hard to tell if I’ve missed a concept. Any thoughts on why this seems to generate equality rather than next timesteps, from my basic description?
By the way, my original project without TimeDistributed is found at http://babble-rnn.consected.com in case you’re interested in extra context.
Perhaps you need to fit for longer or require more training data?
I wondered about that. I think my mistake may be simple…
Imagine the sequence I was trying to learn was 1,2,3,4,5,6,7,8 (which I’d normalise in the range 0:1). In the standard Keras LSTM example without TimeDistributed I’d have:
input X[0] = [0,1,2]
output y[0] = [3]
X[1] = [1,2,3]
y[1] = [4]
…
So in the TimeDistributed setup I reported above, I tried:
X[0] = [0,1,2]
y[0] = [1,2,3]
X[1] = [1,2,3]
y[1] = [2,3,4]
…
In other words, I was offsetting the intended output by just a single timestep for each batch to be learned.
But I’m guessing that I should really offset the output to be learned by the full number of timesteps in each batch:
X[0] = [0,1,2]
y[0] = [3,4,5]
X[1] = [1,2,3]
y[1] = [4,5,6]
Is the latter example what I should be doing? Intuitively, this would explain why I was learning something close to equality in my first run. But from multiple readings of your code in the post it is not clear to me that this is the case.
I’m not sure I follow. There are indeed many ways to frame a sequence prediction problem.
The simplest framing is sequence in => sequence out where either in or out could be one or more time steps.
Keep one sequence as one sample if possible.
Hmm, I think I have missed something big here. Please humour and let me try once more.
In the standard LSTM examples on Keras, if I was to learn a long time sequence (for example integers incrementing in the range 1..100000), I would pick a shorter segment of the total sequence to pass to the LSTM (I split my corpus into sub-batches that represent the number of LSTM timesteps), then the output to learn would be just the next item in the sequence. There is no TimeDistributed output, so I get one result to calculate error against.
input set: 1,2,3
desired output: 4
then repeat with other sub-batches in the same way (and Keras scrambles the order), so the next one may be…
input set: 473, 474, 475
desired output: 476
If that makes sense, then allow me to ask simply what the input and output should be for the TimeDistributed setup. Would it be
(option A)
input set: 1, 2, 3
desired output: 2, 3, 4
(option B)
input set: 1, 2, 3
desired output: 4, 5, 6
(option C)
something else entirely.
Am I making more sense now?
Your example shows input set and desired output being the same, which says to me that the net will just learn the equality function. Again, am I missing something?
Thanks again for your help.
Yes, good question.
Option B.
In your first example you have a many-to-one time step predictive model. In option B you have a many-to-many time step predictive model. The TimeDistributed wrapper would allow you to use the same Dense layer to output each time step in the output sequence, in this case one output time step per input time step.
I hope that helps.
Agreed. Jason’s practically the only person to explain all kinds of things, especially with regard to the tricky subject of data dimensions in the various permutations of different types of NN layers. It has gotten to point where I approach any new Keras subject by including his name in the Google search.
Haha, thanks John!
If you ever need help on these types of topics, post or email a question to me. I’ll whip up a post. Sounds like I’ve found a valuable niche 🙂
Hi Jason, thanks for the great article!
Having looked through this article and forums online, is it correct to say that if we were to do many-to-one prediction (an input vector with an output value), it will be straightforward and faster to just use the Dense layer?
In the case where we want to do many-to-many predictions (multiple input vectors/matrices with an output vector/matrix), TimeDistributed layer should be used instead?
Yes, but in the latter case the dense is wrapped in the timedistributed.
Thank you. I thought the TimeDistributed layer was going to be used to “pass sequentially” the inputs fot the LSTM layer.
Now I understood that the TimeDistributed layer simply “freezes” the Dense output layer weights in order for them to seem like the Dense part is just another “recurrent” tip of the LSTM layer.
One question:
Why do we always have a Dense layer after the last LSTM? Is that a requirement? If yes, why?
Thank you Dr. Jason!
There’s no requirement to wrap a Dense layer, wrap anything you wish.
That does, thank you! For some reason I couldn’t get that from your post, so thanks for taking the time to explain in more detail.
Thanks, that cleared up return_sequences for me, but I still don’t fully understand what TimeDistributed does.
In the last example (Many-to-Many): If I change TimeDistributed(Dense(1)) to just Dense(1), neither the output shape nor the number of parameters changes and it works just as well. What is the difference between these two options in this case?
Note the number of weights in the network.
Without the TimeDistributed wrapper, the Dense is connected to the output from each time step. With the wrapper, the same Dense is applied to each time step.
It’s a question of how you want to model the problem. Let the Dense combine the time steps and output a vector or process each time step one at a time.
Does that help?
Thanks for the article. I have the same question though… number of weights are same regardless of Dense is wrapped by TimeDistributed or not. So, what is the difference, and where can I see that?
But we have the same number of weights in a many-to-many model as we did no the one-to-one model.
A better model design for increased model complexity/capability with the same resources.
Does that help?
Jason, are you saying that in the wrapped case you’re updating the same weights through each time step while in the non-wrapped case, you can think of having a separate dense network for each time-step, so therefore, the number of weights would be the # of outputs for each time-step * # of time-steps?
Hi Jason, and thank you so much for the article! It is fantastic as always.
Alexandr compares last model (with time distrubuted layer) not with the previous one (second model), but with another model: like the third one, with return_sequences=True but without time distributed.
For example I tried and compared the following models
from numpy import array
from keras.models import Sequential
from keras.layers import Dense
from keras.layers import TimeDistributed
from keras.layers import LSTM
# prepare sequence
length = 5
seq = array([i/float(length) for i in range(length)])
X = seq.reshape(1, length, 1)
y = seq.reshape(1, length, 1)
# define LSTM configuration
n_neurons = length
n_batch = 1
n_epoch = 1000
# create LSTM
model = Sequential()
model.add(LSTM(n_neurons, input_shape=(length, 1), return_sequences=True))
model.add(TimeDistributed(Dense(1)))
model.compile(loss=’mean_squared_error’, optimizer=’adam’)
print(model.summary())
with :
from numpy import array
from keras.models import Sequential
from keras.layers import Dense
from keras.layers import TimeDistributed
from keras.layers import LSTM
# prepare sequence
length = 5
seq = array([i/float(length) for i in range(length)])
X = seq.reshape(1, length, 1)
y = seq.reshape(1, length, 1)
# define LSTM configuration
n_neurons = length
n_batch = 1
n_epoch = 1000
# create LSTM
model = Sequential()
model.add(LSTM(n_neurons, input_shape=(length, 1), return_sequences=True))
model.add(Dense(1))
model.compile(loss=’mean_squared_error’, optimizer=’adam’)
print(model.summary())
In both cases the number of parameters is the same:
Total params: 146
Interesting, if true, perhaps the API changed to perform the role of TimeDistributed automatically?
I also tried two type of model.
First one is:
model = Sequential()
model.add(LSTM(32, input_shape=(5, 1), return_sequences=True))
model.add(Dense(1))
And second one is:
model = Sequential()
model.add(LSTM(32, input_shape=(5, 1), return_sequences=True))
model.add(TimeDistributed(Dense(1)))
—————————————————————————
Both have same number of parameter and similar architecture. After training, I am getting same result.
I am not able to understand what is the difference between above two type of implementations. Please help.
Are you able to confirm they have the same number of weights/parameters by reviewing model.summary()?
I suspect that this is the main difference.
I cross checked the number of parameters.
Please check here:
https://ibb.co/jMMmkPJ
https://ibb.co/QM8xkvK
Nice! Perhaps the API has changed in the many years since the post was written.
Hey,
Thanks for amazing tutorial.
This shows simple echo program implementation right ?
I want something like –
Input(For time period 2012-2013) – 1,2,5,3,6,4,7,8,9,5
output(For Time Period 2014) – 1,3,4
The output sequence should be generated based on the input sequence, kindly guide me on that.
Sounds great.
What is the issue exactly? You can use code from the blog directly and adapt it for your problem. Where are you having trouble?
How to create a pyramid of LSTMs. i.e. the input to the first node of 2nd layer LSTM will be output at t1 and t2 of first layer LSTM, similrly 2nd node of the 2nd layer will use t3 and t4 from first layer, and so on..
You mean a stacked LSTM?
Hmm, that’s an interesting layer configuration, I would go with Tensorflow module directly instead of Keras to create such a model, Keras doesn’t have that functionality I guess.
Hello! Is the a way to have DIFFERENT length of input and output-timesteps?
Like, I have series with 100 timesteps in the past and will learn next 10 in the feature?
TimeDistributed requires equal length.
If I output return_sequence=false in the last LSTM and Dense with 10 neurones, would it be the same?
Thanks You!
Sorry, I’m not sure I follow, can you restate your question?
Generally, different numbers of times steps on the input and output are referred to as seq2seq problems and are perhaps best addressed with an encoder-decoder network.
Is the procedure similar when using SimpleRNN?
What do you mean James?
Hello Jason,
Nice article. I was wondering if TimeDistributed layer in Keras is analogous to sequence-to-sequence learning module in Tensorflow. If not, could you point out the distinction between the two?
Thanks.
Sorry, I cannot draw this comparison for you as I am not deeply familiar with the TF code.
Hey Jason. I hope I’m understanding this correctly.
I was trying to model a certain number of days ahead, and found myself frustrated with the fact that I couldn’t just predict one day ahead, then right away use that as part of the sliding input window prior to weights being adjusted – basically I wanted the sliding window to move n days forward using predicted values and only then have gradient descent update weights.
I think this might be the way to do so, but am unsure if I need to wrap every layer in timedistributed or what exactly to do with that.
You can do this, but you will need to create the sliding window yourself and call your model recursively.
Keras will not do this for you with the TimeDistributed layer.
Hi Jason,
Thanks for this post,
I have an Input sequence and output sequence shape as follows:
X_shape: (1, 82600, 1)
Y_shape: (1, 82600, 1)
When I try to use your code for this input and output I get following error:
—————————————————————————
MemoryError Traceback (most recent call last)
in ()
60 # create LSTM
61 model = Sequential()
—> 62 model.add(LSTM(n_neurons, input_shape=(length, 1), return_sequences=True))
63 model.add(TimeDistributed(Dense(1)))
How can I go around this?
Since my length was 82600, according to the code, nb_neurons = 82600
I just reduced the number of neurons to 8260 and the compilation was successful
Since, by default this model is stateless (stateful = True) is not specifically specified, do you think reducing the number of neurons was a right choice or could you suggest some other method.
Note: In my sequence of length 82600, every 10 numbers are dependent on previous 10 numbers.
Yes, that was far too many neurons for the first hidden layer. Nice work.
Perhaps you have too much data to fit into memory.
Perhaps work with a smaller sample?
Perhaps try running on a larger computer like AWS?
Hi Jason, Thanks for responses,
In extension to the question that Phil Ayres asked:
My training data shape is (4096,8) that is 4096 rows and each row has 8 features (8 numeric values).
and the target shape is the same.
Requirement is one entire row is responsible to predict the next row.
example:
input
[1,2,3,4,5,6,7,8]
expected
[100,200,300,400,500,600,700,800]
How can I use time distributed for this kind of data.
Can you please provide an example?
Do I have to call model iteratively, if yes how?
Your problem is seq2seq:
https://machinelearningmastery.com/models-sequence-prediction-recurrent-neural-networks/
You have options:
1. The model can output one output time step for each input time step (e.g. via timedistributed).
2. The model can read the entire input sequence, encode it, then output the entire output sequence (e.g. no timedistributed).
I would recommend trying both approaches and see what works best for your data.
Hello, I am also doing the same problem you did. May I ask what you did then? I feel that the problem we are studying is not the problem of model prediction.Please reply to me as soon as you see it. Thank you!
Thank you sir
For the clarification i have a question that i have a bit of confusion on parameters you have explained above.
For example:
3D sequence with 5 samples, 1 time step, and 1 feature. We will define the output as 5 samples with 1 feature.
X = seq.reshape(5, 1, 1)
y = seq.reshape(5, 1)
What are feature and samples in this example?
Let me have one example assuming we are working on images.
Then i have 2 classes: class-1 is running man (a sequence with 100 images) and class-2 is walking man (a sequence with 100 images).
Then:
– sample = 2 means 1 image from class-1 and 1 image from class-2?
or just 2 images from class-1 or class-2?
If batch is set of samples then why we define sample = 5 and again batch =
5 in this example?
If sample = 1 then we can define batch = 1. What are difference?
– time step = 10 means we are taking images (t1-t10) from 100 images of a
class for prediction? and next time step we are talking images (t2-t11) and
(t3-t12) etc?
– is ‘feature’ image dimension or feature map as an output of Conv layer?
it sounds like a dimension in this example, however cnn says it is output of
conv layer while we are defining ‘feature’ for input image.
if my input image has width = 100 and height = 50 then feature = 5000?
I explain the LSTM input format in more detail here:
https://machinelearningmastery.com/reshape-input-data-long-short-term-memory-networks-keras/
Hi Jason, first of all thanks for your wonderful tutorial. However, I found myself a bizarre issue when testing out on your third example, which is Many-to-Many LSTM for Sequence Prediction (with TimeDistributed). When I remove the TimeDistributed wrapping for the dense layer while keeping the return sequence statement true for the LSTM layer, the model’s summary doesn’t seem to change (same param #). I suppose removing the TimeDistributed wrapping for the dense layer implies a huge fully connected layer connecting to all the outputs of all time stamps, whereas wrapping by the TimeDistributed implies a relatively small fully connected layer connecting to the outputs of one time stamp at a time. Any explanations to this problem? Thanks in advance 🙂
Yes – I have noticed in some of my own experiments that it seems that Dense can now support 3D input without the wrapper.
Support is one thing, behavior is another. Are you saying that a “TimeDistributed(Dense(n)) “layer is no different than a plain “Dense(n)” layer?
It is the same layer, but the wrapper allows the weights required for one time step output from the LSTM to be reused for each time step.
Thanks for this amazing explanation, Jason! I have already put it to the test by creating a “denoiser”, where an image with noise is given as input and a clean version of that image is returned. This is a problem typically solved with the use of autoencoders, which are a complex matter if you ask me. However, I was able to pull this through using this tutorial and that got me thinking: would it be possible to train many-to-many architectures without autoencoders, just by padding input and output sequences to a fixed length? And if yes, would this model work with one-hot encoded vectors? I am not sure how mean squared error calculates results, but would it work with padded, one-hot encoded timestep sequences?
Yes, I don’t see why not.
Hi Jason,
Thank you for this great post.
I’ve read this post three times and the forum discussions, but I still can’t understand how to apply the techniques to the topic I have been working on recently.
Here is the scenario
Input:
[a1,a2,a3,a4,a5,…,aN]
[b1,b2,b3,b4,b5,…,bN]
[c1,c2,c3,c4,c5,….,cN]
Output:
[yN+1,yN+2,yN+3,yN+4,yN+5,….,yM]
( Imagine we have history stock related data (a ,b,c, 3 features of the input). They are all time-series.
And the task is to make predictions ,say, 10 steps ahead ( y of the output ) )
Is this a many to many sequence prediction ?
If it is, then based on the discussions above, I think I would have to use TimeDistributedDense.
However, according to the heavy discussion in the github link below,
https://github.com/fchollet/keras/issues/1029
“For each sample, the input is a sequence (a1,a2,a3,a4…aN) and the output is a sequence (b1,b2,b3,b4…bN) with the same length. bi could be viewed as the label of ai.
Push a1 into a recurrent nn to get output b1. Than push a2 and the hidden output of a1 to get b2…”
It seems that TimeDistributedDense is for sequence labeling, so it is not suitable for my case. Am I right ?
Perhaps this post will help:
https://machinelearningmastery.com/time-series-forecasting-long-short-term-memory-network-python/
Hi Jason
I have my information in the following way:
X Y
========== =============
t1,t2, t3, t4,t5, t6, t7, t8, t9,t10 t11,t12,t13,t14,t15
10,20,20,30,30,40,50,50,50,60 60, 60,70 ,70 ,70
I have 10 most recent time steps to predict the next 5 steps.
In this case from many to many, I could use that method without TimeDistributed for the dense layer ?. Because I understood that in that case the number of dense layer neurons would give me the value for each time step, in this case if I put 5 neurons would have 5 values representing my 5 time steps.
Or maybe I should use the TimeDistributed (DenseLayer) to produce the prediction of the next 5 steps? I’m confused.
I read your post about encoder-decoder. I do not want to use that configuration, I could convert my problem to many to one, and use the only predicted value t11 to predict the next t12 and so on. Does that idea seem right to you?
PS: I also saw an example using repeatvector to be able to do my problem, but I do not know if it is correct.
Perhaps this post will help:
https://machinelearningmastery.com/models-sequence-prediction-recurrent-neural-networks/
Yes, there are multiple ways to formulate the encoder-decoder model, one way with the repeat vector is easy to code and results in good performance.
Sorry, I’m not sure I follow your data.
If you have 5 outputs, you can have a model that outputs a vector of all 5 values or output one at a time using a distributed dense. Why not try both and see which framing of the problem is easier to learn or results in better skill?
Running examples 1 and 2 (just copying your code) returned loss values during training of
nan
and then correspondingly I gotnan
values for the predictions. After some playing around, I found that simply changing the optimizer tosgd
fixed the issue. I had first tried different learning rates within anAdam
class for optimisation, but it always returnednan
s. I can’t from the Keras implementation why this might be the case.I’m glad to hear that.
Hi Jason,
Nice post! Have a question regarding your statement: “The LSTM units have been crippled and will each output a single value, providing a vector of 5 values as inputs to the fully connected layer. The time dimension or sequence information has been thrown away and collapsed into a vector of 5 values.”
What I am understanding from your statement above is that configuration 2 does not give any opportunity for the unrolling. It is almost like an MLP network. Please correct me if I am wrong. Thank you.
Indeed, there is no unrolling when time steps are set to 1.
Dear Jason,
Another amazing tutorial, big love of your articles! However, i am confused that, in configuration 2, many to one without timedistribute example, the input is
X = seq.reshape(1, 5, 1) whose time step is 5, so in this case, per my understanding, the the LSTM will be unrolled 5 times right? And how come in this case LSTM is just like MLP? Sorry, I am still having a hard time understanding “The LSTM units have been crippled and will each output a single value, providing a vector of 5 values as inputs to the fully connected layer. The time dimension or sequence information has been thrown away and collapsed into a vector of 5 values.” Really apprecaite your patentience and looking forward to you response!
In that case, you are not outputting a sequence step by step, you are outputting a vector directly. It may not have the desired effect.
If my understanding is correct, can you please explain why you have used “LSTM units” and not “LSTM unit”. If all I am doing is taking a 5 length sequence as an input and outputting a 5 length sequence, then why do I need multiple LSTM units? Please explain.
The length of input sequences is unrelated to the number of units in the LSTM layer.
Hloo,
I have a silly doubt that how one-to -one model be a sequence prediction problem because there is no any sequence in input neither any timesteps.
Good question.
If we don’t reset state, there can still be memory from prior I/O, just no BPTT going on.
Small typo “afully-connectedd”
Thanks, fixed.
Hi,
I purchased entire bundle, great stuffs ! I have question regarding LSTM though. I have a times-series multi-label problem that need to be classified. The problem somewhat the same as the paper “LEARNING TO DIAGNOSE WITH LSTM RECURRENT
NEURAL NETWORKS” at https://arxiv.org/pdf/1511.03677.pdf.
At each end of each sequence (says 3 diagnostic events / sequence ) they they calculate losses differently: calculate log-loss at each time-steps vs multi-label target, and then combing with final output vs multi-label target and then mean them out for entire sequence.
How do I implement this in Keras ?
Thanks you in advanced.
Steve
Sorry, I am not familiar with that paper, perhaps contact the authors and ask what type of sequence prediction problem it is.
Hi Jason,
When I tried
# train LSTM
model.fit(X, y, epochs=n_epoch, batch_size=n_batch, verbose=2)
The system create the error:
“TypeError Traceback (most recent call last)
C:\Users\Nguyen Viet Linh\Anaconda3\lib\site-packages\theano\gof\cc.py in compile_args(self)
965 try:
–> 966 ret += x.c_compile_args(c_compiler)
967 except TypeError:
TypeError: c_compile_args() takes 1 positional argument but 2 were given”
Looks like your backend might not be installed correctly.
This tutorial may help:
https://machinelearningmastery.com/setup-python-environment-machine-learning-deep-learning-anaconda/
Hi Jason
Thanks for your tutorial. That’s amazing!
I have got 2 questions to ask:
* In the last model that uses TimeDistributed layer, the same weights of the dense layer are applied to all the 5 outputs from the LSTM hidden layer. So during training, for the 5 outputs of the dense layer, is the backprop done 5 times from the last output to the first one?
* You said that the number of training epochs needs to be increased to account for the smaller network capacity. Should it be decreased? Because smaller capacity networks need smaller times of training, while bigger capacity networks need bigger times of training?
Thanks a lot!
Yes, the Dense is trained on each output.
Yes. Small is less training, larger is more training.
I have a question for my text generation project!
Can I adopt
y = np_utils.to_categorical(dataY)
to
TimeDistributed model?
TImeDistributed error say that It needs 3-dimensional input and also output.
But np_utils.to_categorial return 2D output (total_words, n_vocabulary) so I can’t use TimeDistributed model.
Your all posts are really helpful for my LSTM projects! I really appreciate with your sharing
No, TimeDistributed is used for sequence output.
Hi Jason
So what if we don’t use TimeDistributed wrapper when a sequence is returned?
model.add(LSTM(n_neurons, input_shape=(length, 1), return_sequences=True))
model.add((Dense(1)))
I just want to know what the connections would be between the two layers.
Thanks!
I believe the Dense will now handle 3D input.
Hi Jason,
Thank you for your great post. I have 2 questions and hope you may address:
1. Are there any specific reasons behind constraining values in an input sequence to be between 0 and 1? I simply replaced the code to generate seq by:
seq = array([float(i) for i in range(length)])
and all models perform poorly, cannot predict the output y correctly for the same setting of n_epochs, or even 2*n_epochs.
2. Why do we need the Dense layer for the Many-to-One model?
I personally thought that, as return_sequences=True is not set, the output of LSTM layer is already in a 5D vector. Thus, it is unclear to me the specific role of the added Dense layer which receives an input in 5D and also outputs a 5D vector? Removing it can save us 30 parameters to be trained. (Please correct me if I miss something important here)
Thank you very much.
Normalizing inputs is a standard practice that improves modeling.
The LSTM returns the final output from the end of the sequence by default. We can return the sequence of outputs and have a dense layer interpret them before outputting a final prediction.
hi jason
i have for example 10 seq of data with the length of 10 char on each one.
like a matric of 10×10
so at first i want o read row by row and in the next layer iwant to read column by column.
is it true if i use time distributed(lstm) at the first layer and simple lstm in the second?
TimeDistributed is really for seq2seq type problems.
in fact i want to know how network deal with data? and how we can determine the way of feeding data to the lstm network.
Ultimately, data is provided one row at a time.
n = 4 * ((inputs + 1) * outputs + outputs^2), where does the 4 come from?
The number of weights in an LSTM unit (I guess, from memory).
How do we know that it should be 4?
LSTM has 4 gates, so that’s the reason. If you work with GRU, that has 3 gates, you multiply by 3.
Can the Timedistributed layer be applied to predict individual class values (like vocabulary items)? This seems to work well with regression problems, where the mean_squared_error is used, but what if we wanted to output a sequence of vectorized words for example. I’ve tried training a translation model using a padded 3d sequence as X and a padded 3d sequence as y using the sparse_categorical_crossentropy loss function, I had the last Dense layer output as many outputs as the maximum vocabulary. It seemed to output rubbish with less than 20 examples and when I went to 250 examples, the model would only output zeros. What am I doing wrong? Also, is it possible to output variable length vectors? Let’s say I have a sequence X of shape(1, 300, 1). Can I train it to a y vector of shape (1, 50, 1)?
Yes. I have a few examples on the blog for NLP with encoder-decoder LSTMs.
Hi Jason,
Thank you for your post!
I have three questions:
1. Regarding to Many-to-One, the output dimension from the last layer is (1, 5), while the input shape to LSTM is (5, 1). To me, it feels like, the input is a one feature with 5 timesteps data while the prediction output has 5 features with 1 time step… I am confused.
2. What is the difference in the performance of forecasting between Many-to-One and Many-to-Many? According to my understanding, Many-to-Many uses all 5 hidden states of the 5 LSTM cells to make prediction, while Many-to-One only uses the final state.
This post will help you better understand the input shape for LSTMs:
https://machinelearningmastery.com/reshape-input-data-long-short-term-memory-networks-keras/
It is the difference between the models is that of outputting a scalar vs a vector.
Hi Jason,
Your tutorials are game-changing.
To use a TimeDistributed output layer for classifying n different classes, would the Y shape be (samples, timesteps, n), with each label as an n-dimensional one-hot array? Or would the binarized label still be considered one feature?
To match that, should the output layer also be of size n?
Thanks for all of your work!
No, for multi-class classification a TimeDistributed layer would not be required.
You would use n neurons in the output layer and a softmax activation function, where n is the number of class values (factors).
What I mean is, still using the TimeDistributed wrapper to get one output per time_step, but instead of binary classification, having n categories. My input and output layers are, respectively,
LSTM(32, input_shape=(time_step, num_features), return_sequences=True)
and
TimeDistributed(Dense(num_labels, activation=”softmax”))
And it seems to be working well with Y.shape == (samples, timesteps, num_labels)
I see, then each time step would be a one hot encoded vector.
Thanks again, Jason.
Is it possible to apply sample_weight or class_weight to a model with this input?
Keras requires a 2D sample_weight array:
“In order to use timestep-wise sample weighting, you should pass a 2D sample_weight array.”
Reshaping it to be 2D obviously does not match Y.shape of (samples,timesteps,num_labels)
I did not forget to set sample_weight_mode=”temporal”.
With the class_weight approach:
“ValueError:
class_weight
not supported for 3+ dimensional targets.”Is it possible to use either of these functions for the timedistributed layer? Do you have to create a custom loss function?
I don’t know sorry, I have not tried.
Thanks Jason, I’ll share what I find!
It works fine.
I am also trying to run a Timeseries to multi-class classifcation model. I am struggling to reshape Y.
I got the expected results in (Samples, num_labels). In order to use them with the TimeDistributed wrapper I did
y_train = expand_dims(y_train, axis=1)
y_train = repeat(y_train, timesteps, axis=1)
because I am expecting that the expected output for every timestep in a row stays the same. This blows up the numpy array considerably. Any idea how this can be done in a more elegant way.
Thanks a lot in advance
Wirtsi
I have a worked example of LSTM for multi-class classification here:
https://machinelearningmastery.com/how-to-develop-rnn-models-for-human-activity-recognition-time-series-classification/
Ah, beautiful … hadn’t seen that post yet, it’s great. That’s exactly what I am trying to do.
So I am assuming that in the post’s LSTM example. you are _not_ using the TimeDistributed wrapper because the expected output is not a time series but a one-hot encoded array. Is that correct?
I played around with the code in the post by reshaping the output like mentioned above and using the TImeDistributed wrapper.
When removing the Dense Relu Layer, training becomes quite fast but the accuracy is at around 0.84. With the Relu Layer (+ TimeDistributed), accuracy is on par with the original one. So I guess it doesn’t make sense to use the wrapper here.
Bests and thanks for your great work!
Wirtsi
I’m glad it helped.
Thank you very much a great post Jason. I have a problem where the input sequence is longer than the output sequence – input data = (samples, 26 time steps, 20 features) and the target data = (samples, 7 time steps, 1 target). Keras throws and error saying it expects the target data to have a shape of (26, 1) if I feed it the shorter (7, 1) shape in the last TimeDistributed(Dense(1)) layer.
One simple solution would of course be to just grab the first 7 of the 26 steps of the fit. However, I would think that it would be an easier and faster job to fit only 7 steps.
Is it possible to tell Keras that the input sequence is of a different length than the output sequence?
When the input and output sequences have a different lengths, you can use an encoder-decoder architecture:
https://machinelearningmastery.com/encoder-decoder-long-short-term-memory-networks/
Many thanks. I will give that a go.
Thanks for this article, this is very helpful
I am trying to implement a simple sequence classifier. I create a set containing 10 random integers between 0-100 and if that set consists of a number that is a multiple of 10, then y is 1 and if not, then y is 0.
For ex: If X is [10,11,34,56,78,99, 21, 24, 25, 77]. Then y is 1
For ex: if X is [1,11,34,56,78,99, 21, 24, 25, 77], then y is 0
I reused, your code for this purpose, but I am unable to get the correct results. Below is the code, Can you please tell what is wrong with this code? Appreciate your help on this. Thank you!
from random import random
from numpy import array
from numpy import cumsum
from keras.models import Sequential
from keras.layers import LSTM
from keras.layers import Dense
from keras.layers import TimeDistributed
import numpy as np
import random as rd
# create a sequence classification instance
def get_sequence(n_timesteps):
# create a sequence of 10 random numbers in the range [0-100]
X = array([rd.randrange(0, 101, 1) for _ in range(n_timesteps)])
#If the sequence has a number that is a multiple of 10, then Y is 1
#If not, Y is 0 by default
y = 0
for i in range(n_timesteps):
if(X[i] % 10 == 0):
y = 1
break;
#Convert y to a numpy array
y = np.asarray(y)
# reshape input and output data to be suitable for LSTMs
X = X.reshape(1, n_timesteps, 1)
y = y.reshape(1)
return X, y
# define problem properties
n_timesteps = 10
# define LSTM
model = Sequential()
model.add(LSTM(200, input_shape=(n_timesteps, 1)))
model.add(Dense(1, activation=’sigmoid’))
model.compile(loss=’mean_squared_error’, optimizer=’adam’, metrics=[‘acc’])
# train LSTM
for epoch in range(100):
# generate new random sequence
X,y = get_sequence(n_timesteps)
# fit model for one epoch on this sequence
model.fit(X, y, epochs=epoch, batch_size=1, verbose=2)
# evaluate LSTM
#Variable to hold correct predictions
cor_pred = 0
for epoch in range(100):
X,y = get_sequence(n_timesteps)
yhat = model.predict_classes(X, verbose=0)
print(‘Expected:’, y, ‘Predicted’, yhat)
#Check if the predicted vs actual match, if so increment the count of correct predictions
if(y == yhat):
cor_pred = cor_pred + 1
print(“Correct Prediction:”, cor_pred)
Perhaps you need to tune the model for the problem?
Perhaps explore other framings of the problem?
I offer a suite of more ideas here:
https://machinelearningmastery.com/improve-deep-learning-performance/
Hello, this article help me a lot.
Now, I only have one question: how to deal with sequence need padding ?
For example:
I want to train a model to detect wrong word using in an ariticle.
I generate trainning data as below:
corrects = [
['How', 'are', 'you', '!'], # [1, 1, 1, 1]
['Fine', ',', 'thank', 'you', '.'], # [1, 1, 1, 1, 1]
['Do', 'you', 'have', 'meal', '?'], # [1, 1, 1, 1, 1]
...
]
wrongs = [
['How', 'were', 'you', '!'], # [1, 0, 1, 1]
['Find', ',', 'thank', 'you', '.'], # [0, 1, 1, 1, 1]
['Did', 'you', 'have', 'meal', '.'], # [0, 1, 1, 1, 0]
...
]
I need handle the wrong word in first, and also want last word of a sentence can affect by first few words of latter sentence.
Some article told me do not backpropagate for the errors of those padded words, by masking their loss.
But I can’t understand what he said, If I pad X, I need change y to same size (so the padding label is a new value, may be
-1
? ).Could you give me some tip?
You can use zero-padding to make sequences the same length and a Masking layer to ignore the zeros.
Here’s examples of padding:
https://machinelearningmastery.com/data-preparation-variable-length-input-sequences-sequence-prediction/
Here’s an example of masking:
https://machinelearningmastery.com/handle-missing-timesteps-sequence-prediction-problems-python/
Thank you, I get it.
May I ask one more question?
I’d like to use
Bidirectional
,from https://machinelearningmastery.com/develop-bidirectional-lstm-sequence-classification-python-keras/
You use
Bidirectional
andTimeDistributed
together, is it suit for my wrong word detection task ?You can use them together.
I don’t know about the use on that application, try it and see.
Thank you for the great article Jason. I believe i am dealing with a problem of type that can be solved using “Many-to-Many LSTM for Sequence Prediction (with TimeDistributed)” technique explained above.
In my problem i have more samples and more features compared to the example above but i am trying sequence to sequence with output at every timestep.
One sample in my problem, input(1,5,2)–> output(1,5,1) looks as follows.
[[1,2,3,4,5]
[10,20,30,40,50]] –> [10,40,90,160,250]
Am i correct in trying out the TimeDistributed technique for this problem?
If so, how are batch-size, epochs, number of neurons going to impact the model intuitively?
Is it correct to say that batch-size and epochs together play role in number of iterations which leads to number of times the weights are updated. And number of neurons help the model to remember the sequence?
Do i have to worry about “stateful” in this kind of problem?
You could try with and without it and compare the results.
Model weights are updated after each batch. You can make the model stateful and control when weights are updated instead – if you need that control.
More neurons means more capacity to learn, but harder and slower to train.
Thank you.
Very interesting article!
When you write,
“The LSTM units have been crippled and will each output a single value, providing a vector of 5 values as inputs to the fully connected layer.”,
is this vector is the output of the last LSTM unit?
No, of the network.
I’m struggling to figure out what data property determines what the TimeDistributed(Dense(1)) expects. It never seems to line up for me.
Thanks
What do you mean exactly Jared?
Hi Jason, great tutorials, I was wondering , what is the definition of many to many in this example? Is it many features to many features or many time steps to many time steps? Thanks. Jimmy
Many input time steps to many output time steps, regardless of the number of features.
Thanks. So if I want to have 3 outputs at the same time do I change model.add(TimeDistributed(Dense(1))) to model.add(TimeDistributed(Dense(3))) ? Will this be the same as multi steps forecasting?
That would produce 3 features per output time step.
Perhaps try an encoder-decoder network instead:
https://machinelearningmastery.com/encoder-decoder-long-short-term-memory-networks/
Awesome Thanks. Btw, please see below, both model 1 and 2 compiled and trained but I don’t know what’s their difference?
x_train.shape (4767, 10, 4)
y_train.shape (4767, 5, 1)
What’s the difference?
#— model 1
model = Sequential()
model.add(LSTM(128, input_shape=(data.shape[1],data.shape[2]), return_sequences=True))
model.add(Dropout(d))
model.add(LSTM(64, input_shape=(data.shape[1],data.shape[2]), return_sequences=False))
model.add(Dropout(d))
model.add(RepeatVector(y_train.shape[1]))
model.add(LSTM(64, return_sequences=True))
model.add((Dense(1)))
model.compile(loss=’mse’,optimizer=’adam’)
#— model 2
model = Sequential()
model.add(LSTM(128, input_shape=(data.shape[1],data.shape[2]), return_sequences=True))
model.add(Dropout(d))
model.add(LSTM(64, input_shape=(data.shape[1],data.shape[2]), return_sequences=False))
model.add(Dropout(d))
model.add(RepeatVector(y_train.shape[1]))
model.add(LSTM(64, return_sequences=True))
model.add(TimeDistributed(Dense(1)))
model.compile(loss=’mse’,optimizer=’adam’)
In the first, there will be 1 output, in the second there will be one output per input.
Are they both multi layer seq2seq models ?
Yes and no, it depends on how you define a seq2seq model.
Focus on results rather than model types.
Hi Jason, by the way, I’m trying to use 10 time steps with 4 features to predict 5 time steps but I got some error.
x_train.shape
(4768, 10, 4)
y_train.shape
(4768, 5, 1)
d = 0.2
model = Sequential()
model.add(LSTM(128, input_shape = (x_train.shape[1], x_train.shape[2]), return_sequences=True))
model.add(Dropout(d))
model.add(LSTM(64, input_shape = (x_train.shape[1], x_train.shape[2]), return_sequences=True))
model.add(Dropout(d))
model.add(TimeDistributed(Dense(5)))
model.compile(loss=’mse’,optimizer=’adam’,metrics=[‘accuracy’])
model.fit(x_train, y_train, batch_size=512, nb_epoch=3, validation_split=0.05)
#—— got error
ValueError: Error when checking target: expected time_distributed_14 to have shape (None, 10, 5) but got array with shape (4768, 5, 1)
I recommend using an encoder-decoder model for a seq2seq type problem.
Hi, I am trying to build a hierarchical model using timedistributed and want to access intermediate layers.
Model:
h_words = Bidirectional(GRU(200, return_sequences=True))(words)
sentence = Attention()(h_words)
sent_encoder = Model(sequence_input, sentence)
document_input = Input(shape=(None, MAX_SENT_LENGTH), dtype=’int32′)
document_enc = TimeDistributed(sent_encoder)(document_input)
h_sentences = Bidirectional(GRU(100, return_sequences=True))(document_enc)
preds = Dense(7, activation=’softmax’)(h_sentences)
model = Model(document_input, preds)
So, model.layers only gives me the layers from the second part of the model. How can I access model layers used within timedistributed.
By using the functional API, you can keep reference to any layer you wish directly.
Thank for your explanation.
My question: Is it possible to use TimeDistributed in Many-to-One LSTM? Is there any different in terms of accuracy and sensitivity if we use and don’t use TimeDistributed?
Sure.
It may or may not impact skill directly, it is more a question of how you want the model to interpret your data, e.g. as a vector or as independent time steps.
Hi, I want to make a very long sequence classification. I have a dataset with 11000 samples where each sample is composed of 20 sequences of 8000 points. Therefore, the input shape should be (11000, 8000, 20) isn’t it?
However, you recommended several times not to set a time step bigger than 400-600. I have 2 related questions (truncating the sequences is not an option):
1.- I should add an extra dimension to the input shape so as each sample is divided in multiple chunks? Let’s say I want to divide each sequence into 100 sub-sequences of 80 points. The input shape should look like (11000, 100, 80, 20). I do not know if this is the correct way to do this.
2.- In this way, I have to match multiple multiple sub-sequences to a single output. How can I achieve this with TimeDistributed and LSTM?
Any suggestion?
Thank you in advance
I don’t understand what you mean by sequences and points.
Perhaps this will help:
https://machinelearningmastery.com/faq/single-faq/how-do-i-prepare-my-data-for-an-lstm
Sorry, I did not explain myself correctly. In my case, sequences are time series and the points are the values of the time series.
In this way, I want to make a binary classification of events that are composed of 20 time series of length 8000, that is, for each event, I have multiple large time series and 1 target.
CNNs are excellent a time series classification tasks. I’d recommend starting there.
Hi Jason
Thanks alot for this blog, learned alot and finally found a possible solution as I am on the verge of giving up.
this is my code, is it possible to have a quick browse through if this makes sense:
model = Sequential()
model.add(Bidirectional(LSTM(448, input_shape = (3, 43), activation = ‘relu’, return_sequences=True)))
model.add(Dropout(0.1))
model.add(Bidirectional(LSTM(256, activation = ‘relu’, return_sequences = True)))
model.add(Dropout(0.2))
model.add(TimeDistributed(Dense(64, kernel_initializer = ‘uniform’, activation = ‘relu’)))
model.add(TimeDistributed(Dense(1, kernel_initializer = ‘uniform’, activation = ‘linear’,
kernel_regularizer = regularizers.l2(regu))))
model.compile(optimizer = ‘adam’, loss = ‘mse’, metrics = [‘accuracy’])
net_history = model.fit(X_train, y_train, batch_size = batch_size, epochs = num_epochs, verbose = 0, validation_split = val_split, shuffle = True, callbacks = [best_model, early_stop])
1. I just to seek your opinion if the above model makes sense.
2. I have having trouble with the shape of test. As you mentioned in the article, it needs to be 3 dimensions. This is my current shape:
X_train (3620, 3, 43) y_train (3620, 1)
X_test (905, 3, 43) y_test (905, 1)
I got my X train and test to have a lag of 3 using a moving window method. So when i get an error as follows:
ValueError: Error when checking target: expected time_distributed_4 to have 3 dimensions, but got array with shape (3620, 1), does it mean I need to apply the moving window to y as well?
Extremely confused and appreciate if you can help me out.
Cheers
Sorry, I don’t have the capacity to review and debug your code example.
Regarding the shape of the data, this might help:
https://machinelearningmastery.com/faq/single-faq/how-do-i-prepare-my-data-for-an-lstm
in many to many model, full code line 18,
model.add(TimeDistributed(Dense(1)))
Why should we add this TimeDstributed layer here? I changed this line to following:
model.add(Dense(1))
the code still works.
So what is the difference between these two?
The difference is the number of weights.
Perhaps re-read the tutorial?
Whether I did use TimeDistributed(Dense()) or just Dense(), model description from both cases were exactly the same on Output Shape/Param #.
Is it just a matter of historical design? I had seen through some forum and they said Dense layer won’t compile if an input was not a 2D layer. Now Dense layer supports nD layers. If the parameter numbers and shapes remain the same, and this guy said the Dense connects to all steps when return_sequences=True, does that mean the weights are shared automatically?
https://stackoverflow.com/questions/43265084/keras-timedistributed-are-weights-shared
There is a prove that both are the same, but I don’t know if it is valid:
https://stackoverflow.com/questions/44611006/timedistributeddense-vs-dense-in-keras-same-number-of-parameters
It comes down to how many weights are in your model and what the output part of the model is actually doing.
Yes, it is a matter of design. Try both, go with what performs better or makes more sense to you.
What do you mean by: “The LSTM units have been crippled and will each output a single value, providing a vector of 5 values as inputs to the fully connected layer. The time dimension or sequence information has been thrown away and collapsed into a vector of 5 values.”
Isn’t the (1,5,1) input, the input that is using the sequence information? From the documentation, the dimensions are (batch_size, time_steps, features). Shouldn’t the first version, with dimension (5,1,1) be the case where LSTM is useless, unless you keep the state for the next example?
Thanks
I am suggesting that information over the sequence is lost as we reduce the input sequence down to only the output of the LSTM layer at the end of the sequence, not the output of the layer over the whole sequence.
State is maintained across the batch, the default batch size is 32, no problem with state here.
I don’t see a problem, but perhaps I misunderstand your comment?
Thanks for your quick response!
BUT, I am confused about how the batches work in RNNs. The point of the RNN is to use information from previous observations, right? And the equations of an RNN/LSTM are feeding the hidden state Ht-1 from time step t-1 and combining it with the input Xt at timestep t. Where this is done recursively for every t.
Say that we are doing a sentiment classification, which means we are given an input-text, say of length 100, and we are predicting a binary label, 0,1. And we have 1000 of these texts with corresponding label. This will then be a (1000, 100, “embedding_size”) shaped input. The batches are then taken from index, 0, ie dividing up the 1000 examples, correct? Say we have a batch size of 10. This is then 10 sequences, which is not really related. Are the states shared among these 10 sequences for every timestep?
In that case, is the way to “isolate”, ie model one sequence at a time, to use a batch size of size 1?
Thanks
Previous observations are available within each sample as “time steps”. Words would be time steps within a sample.
The model can also maintain state across samples within a batch, or more if you choose stateless and control when the state is reset.
No, it would be [1000, 100, 1] as the embedding takes integer encoded words which it will then map to vectors.
If you have a batch size of 10, then 10 samples of the 1000 will share state before state was reset and weights updated. If each sample is one document, then sharing state across documents may not make sense.
Thanks a lot for that comprehensive response. It made things much clearer for me I think.
So if I don’t want states to be shared within a batch, because the sequences are not necessarily related within the batch, is the best solution to use a bath size of 1? Or structure my data in a way that related sequences end up in the same batch?
Yes.
Or first confirm that it even matters for your problem. It often doesn’t.
Hi Jason,
thanks for the tutorial, i found if very helpful, however i have a doubt that if for the staetless lstm, if the states of samples within a batch share their state? say if the batch size is 10, according to this link: https://www.oipapio.com/question-81259
the 10 sequences have 10 states, so they don’t share the states within the batch,right?
best,
fan
Yes, state is preserved across samples until the end of the batch.
Thanks for the detailed explanation. Might be my question would be simple but I didn’t understand. While doing the parameter calculations I didn’t get why you have multiplied with 4.
Could you please clarify?
n = 4 * ((inputs + 1) * outputs + outputs^2)
n = 4 * ((1 + 1) * 5 + 5^2)
n = 4 * 35
n = 140
4 weights per unit.
Thanks Jason, this really helps!
For keras model # params calculation , it took me a while to figure out in your calculation, the multiplier “4” is from the # of LSTM gates (update, forget, )
Yes.
Hi Jason,
I use “series_to_supervised” as you teach in my LSTM. Is Timedistributed wrapper is the same or it is a good way to combine both?
It is very similar.
It may be easier to use for some people.
Hi Jason,
ok the model have learned the sequence,can this type of model predict the next step of the sequence?
Yes. You can get started here:
https://machinelearningmastery.com/start-here/#lstm
yes i have read a lot thank you for the articles but i can’t find more and i am confused about get the prediction from sequence
You can make a prediction with a fit model by calling the predict() function.
Does that help?
yes but hear in this article you enter the sequence [ 0. 0.2 0.4 0.6 0.8]
when you run the predict you get again the sequence–>
0.0
0.2
0.4
0.6
0.8
that means the model learned the sequence ok?Now how to predict the next step of this sequence—>0.10
Pass in [0.2,0.4,0.6,0.8] to the predict() function.
# prepare sequence
length = 5
seq = array([i/float(length) for i in range(length)])
X = seq.reshape(len(seq), 1, 1)
y = seq.reshape(len(seq), 1)
# define LSTM configuration
n_neurons = length
n_batch = length
n_epoch = 1000
# create LSTM
model = Sequential()
model.add(LSTM(n_neurons, input_shape=(1, 1)))
model.add(Dense(1))
model.compile(loss=’mean_squared_error’, optimizer=’adam’)
print(model.summary())
# train LSTM
model.fit(X, y, epochs=n_epoch, batch_size=n_batch, verbose=2)
# evaluate
seq = array([0.2,0.4,0.6,0.8])
s = seq.reshape(len(seq), 1, 1)
result = model.predict(s, batch_size=n_batch, verbose=0)
for value in result:
print(‘%.1f’ % value)
0.2
0.4
0.6
0.8
again the same did i pass wrong the list [0.2,0.4,0.6,0.8] to the predict() function?
Perhaps the example is too advanced for you, a good place to get started with time series prediction is here:
https://machinelearningmastery.com/start-here/#timeseries
the same and the same again and with other inputs what i do wrong?
Perhaps try going back to basics:
https://machinelearningmastery.com/start-here/#lstm
please help more dr my head it’s going to explode from the reading,this is your example with the inputs to predict as you say.
Hi
Can one say the following in general: while training sequence data using RNN, one should wrap any used Dense layer by the hidden or output layer with a corresponding TimeDistributed layer only and only if the number of timesteps by that given Dense layer is bigger than one. Not doing so would cause the Dense layer weights of different timestamps to interfere with each other while training the model?
No, only when the number of input and output time steps differ, or when you want to use the same output layer for each output time step.
Hi! Still dont get what TimeDistributed does after reading the article.
We can run your last many-to-many example
model = Sequential()
model.add(LSTM(n_neurons, input_shape=(length, 1), return_sequences=True))
model.add(TimeDistributed(Dense(1)))
without TimeDistributed wrapping as
model = Sequential()
model.add(LSTM(n_neurons, input_shape=(length, 1), return_sequences=True))
model.add(Dense(1))
and get absolutely same results in terms of loss, params number and training time
It is not about the result, but instead about the number of weights/parameters in the model with and without the wrapper.
It is an architectural concern that could effect performance.
Dear Jason.
Thank you for your super article. But I have this problem: I have sound samples of 2 sec. I used FFT. I created an numpy array of size 42.96. 42 rows represent a time sequence (0.47s, 0.95s …) and 96 columns are single frequencies (27.5Hz, 29.13Hz ..). How should I create a LSTM and TimeDistribution model when no time information is stored anywhere, but the individual rows simply have a fixed time distance? And how to organize it so that the model is dealt with separately by the individual frequencies (given that one column is actually the development of a certain frequency over time)? Thanks for any advice.
I recommend exploring a range of different framings of the problem in order to discover what works best for your specific dataset.
Perhaps start here:
https://machinelearningmastery.com/start-here/#deep_learning_time_series
Hi Jason,
In CNN+LSTM part, why we need to split the timestamp 128 into 32, 4? Does the split process must be done?
Can we modify the model.add(TimeDistributed(Conv1D(….), input_shape=(None, n_timesteps, n_features))) to
model.add(TimeDistributed(Conv1D(….), input_shape=(n_timesteps, n_features))), let the dimenstion of input_shape be 2, not 3
Thanks
The CNN must operate on sub-patterns. The TimeDistributed layer will provide multiple sequences.
Good Content
Thanks.
n = inputs * outputs + outputs –> n = inputs * outputs + bias, I suppose
Yes.
Hi Jason, this is an awesome post.
But there is one thing I confuse about, why the output shapes of lstm_1 (LSTM) and dense_1 (Dense) in one-to-one LSTM are (None, 1, 5) and (None, 1, 1), aren’t they supposed to be (None, 5) and (None, 1) because the return_sequence is not True and there is no TimeDistributed ?
The output model will have one output sample per input sample and each sample will have one or more time steps, e.g. it must be 2d.
Hi, Jason.
I ran the one-to-one LSTM for Sequence Prediction code you shared by myself, the output shapes of lstm_1 and dense_1 are (None, 5) and (None, 1) which are different from the result you showed below the code in this post. Why did that happen?
I have some suggestions here:
https://machinelearningmastery.com/faq/single-faq/why-does-the-code-in-the-tutorial-not-work-for-me
Hi Jason. Thank you for nice article.
I have a question about Time Distributed wrapper.
@Phil Ayres asked you what is input and output data structure for Time Distributed wrapper, and you answered him that option B is correct, not option A.
(option A)
input set: 1, 2, 3
desired output: 2, 3, 4
(option B)
input set: 1, 2, 3
desired output: 4, 5, 6
But I can’t understand why option B is correct for Time Distributed wrapper.
As I understand it, Time Distributed wrapper allows that dense layer uses same weights for each outputs generated from one time steps.
It means, in option B, output 4 is generated from input 1 with random initial states and output 5 is generated from input 2 with conveyed states. Is this right?
If I understand it correctly, I think that this output results are not good and not logical.
In the LSTM model using option B data, first LSTM cell using input 1 can not generate output 4 because their is no time series information between 1 and 4. \
For the same reason, I think that input 2 can not generate 5 and input 3 can not generate 6.
So I thought that it is better to use many-to-one model producing one vector for option B, or to repeat many-to-one model producing one output value and using that result as input again. I thought that Time Distribution wrapper is good for specific data like option A only.
Would you please tell me what are I misunderstood?
The option a vs b dichotomy feels false. It’s probably neither. It is a mapping problem of some inputs to some outputs.
The LSTM will output a representation of the entire input sequence. The dense output layer can then read from it and output a sequence.
There’s no state in the dense, as you say, the same set of weights is applied to each interpreted input step.
Thank you for your kind reply Jason.
But I can’t understand “The LSTM will output a representation of the entire input sequence.”.
I thought that TimeDistribution wrapper is used for many-to-many mapping, like a rightmost example in first figure in http://karpathy.github.io/2015/05/21/rnn-effectiveness, not another many-to-many mapping.
TimeDistribution wrapped Dense layer will be applied to each outputs generated from LSTM of each step.
I think that only last LSTM cell will output a representation of the entire input sequence. The other LSTM cells will output a representation of the part of input sequence because remaining sequence data are not inputted yet.
Am I missing something?
Thanks again for your kind advice.
Correct, the return_sequences=True means that each unit will return an output time step for each input time step. This is representation of the input sequence.
Hi Jason!
First of all thanks for your nice tutorial! They really help a lot.
I think get the idea behing the Time Distributed layer. I was wondering, though, why in this other tutorial https://machinelearningmastery.com/how-to-develop-lstm-models-for-time-series-forecasting/ , when you explain the vector output model you do not use the TD layer.
Thanks again!
Thanks Oscar.
The wrapper is used to use the same dense layer to output each value or time step in the output sequence.
Thanks for replying!
Let me ask another final question:
– Let’s say we want to solve a seq2seq problem. If we have the same number time steps in the input and output sequences, we can use either the TimeDistributed layer or a vector output multi-step LSTM model (which I guess it’s the 2nd case here). Is then the vector output model converting the problem from many-2-many into many-2-one?
– Therefore, is it always better to use the TimeDistributed for such problems?
Yes, vector output is a many to one model.
Always experiment/test and use results to make the decision about what is best for your dataset.
Hi,Jason! I want to take a dense layer as the first layer instead of LSTM layer, but how can I change the shape of training set so as to work well in the model? Do you have examples about this kind of model?
A dense layer can only take a 1D vector per sample as input.
Sorry,I don’t understand. for example, I want to make a LSTM model of 4 layers (the structure of the lstm: the input layer —>activate function 1—->Multi-RNN—->activate function 2—>output layer—>activate function 3—>the prediction of label) ,and this model is a many-to-one model with a input X (shape: (376,5,7), means (376 samples, 5 time_steps, 7 features)), and Y(shape(376,5,1)). how can I programming for it.
A good place to start would be this tutorial, and adapt an example for your specific project:
https://machinelearningmastery.com/how-to-develop-lstm-models-for-time-series-forecasting/
And, I want to use keras to program it . I already have other method like: “””
batch_size = tf.shape(X)[0]
time_step = tf.shape(X)[1]
w_in = weights[‘in’]
b_in = bias[‘in’]
input_data = tf.reshape(X,[input_features,-1])
input_lstm = tf.matmul(w_in,tf.cast(input_data,tf.float32)) + b_in
input_lstm = tf.nn.leaky_relu(input_lstm)
input_lstm = tf.reshape(input_lstm,[-1,time_step,n_features])
“””
activate function for input layer to the lstm layer 1
“””
#input_lstm = tf.nn.leaky_relu(input_lstm)
cell_1 = tf.nn.rnn_cell.BasicLSTMCell(n_features, state_is_tuple=True)
cell_1 = tf.nn.rnn_cell.DropoutWrapper(cell_1,input_keep_prob=forget_rate[‘input_keep_prob’],
output_keep_prob=forget_rate[‘output_keep_prob’])
Multi_lstm_cell = tf.nn.rnn_cell.MultiRNNCell([cell_1] * num_LSTMlayers, state_is_tuple=True)
init_state = Multi_lstm_cell.zero_state(batch_size, tf.float32)
#run the lstm process
with tf.variable_scope(‘sec_lstm’,reuse =tf.AUTO_REUSE):
output_lstm, final_state = tf.nn.dynamic_rnn(Multi_lstm_cell, input_lstm, initial_state = init_state, time_major = False)
“””
activate function for output from lstm layer 2 to the output layer
“””
output_lstm = tf.reshape(output_lstm,[n_features,-1])
output_lstm = tf.nn.leaky_relu(output_lstm)
w_out = weights[‘out’]
b_out = bias[‘out’]
output_data = tf.matmul(w_out,output_lstm) + b_out
“””
activate function for output from output layer to the label
“””
output_data = tf.tanh(output_data)
return output_data,final_state “””
Thank you for some other informative website.
Where else may just I get that type of information written in such an ideal way?
I’ve a undertaking that I am simply now operating on, and I have been on the look out for such info.
Thanks.
Hi Jason,
thank you for the post. It is really helpful. I have a question. Here you can solve the problem by stating it many-to-one and or many-to-many sequence problem. What happens if it was classification instead of regression? Assume that I have inputs and outputs like:
x = [0, 1, 2, 3, 4]
y = [a,b, c, d, e]
x = [4, 4, 3, 0, 1]
y= [a, b, d, d, c]
…
So I have 5 categories for the output. It can be solved as many-to-many like:
model = Sequential()
model.add(LSTM(20, input_shape = (5, 1), return_sequences = True)
model.add(TimeDistributed(Dense(5, activation = ‘softmax’)))
So at each time step, it outputs 5 outputs and the one with the highest one is the output for this timestep.
My question is that is it possible to state this problem as many-to-one?
Yes, your model can output a vector with n nodes, matching the no nodes for input.
I would expect a seq2seq model would perform better, try both.
Hi Jason, great blogpost, it’s very clear!
I have a small question about
model.add(TimeDistributed(Dense(1)))
The layer preceding this line has the summary:
lstm_1 (LSTM) (None, 5, 5) 140
and then the time-distributed dense layer gives
time_distributed_1 (TimeDist (None, 5, 1) 6
but I find that a Dense(1) layer without the TimeDistributed wrapper gives the same thing, as it should. Such a plain Dense(1) layer should and does map the final dimension of the output of the LSTM into a scalar and does not touch the other dimensions of the LSTM.
Am I missing something else that the TimeDistributed wrapper is doing?
best
Nick
Without the TimeDistributed layer (section titled “Many-to-One LSTM for Sequence Prediction (without TimeDistributed)”), the Dense has 30 weights, with the TimeDistributed layer (section titled “Many-to-Many LSTM for Sequence Prediction (with TimeDistributed)”), the Dense has 6 weights.
That is the main difference.
Does that help?
Hi again, so I think the difference in the size of the weights you quote is due to the reshaping of the input not the TimeDistributed wrapper.
This stackoverflow answer
https://stackoverflow.com/questions/46896807/keras-lstm-timedistributed-stateful
is fairly clear, in particular I quote
“Using the TimeDistributed or not with Dense layers is optional and the result is the same: if your data is 3D, the Dense layer will be repeated for the second dimension. ”
of course that quote alone doesn’t make it correct 🙂 However I explicitly tried replacing
model.add(TimeDistributed(Dense(1)))
with
model.add(Dense(1))
and it gives the same model.summary
Thanks.
I can confirm that replacing the TimeDistributed(Dense) with a Dense in the second example achieve the same number of weights.
I suspect the API, e.g. the support for 3d input to the Dense, has changed since the post was written all those years ago.
I’ve added a note to the top of the post.
There is problem in the models posted.
They don’t actually learn sequence, they learn identity function.
For example, in 3rd model (many-to-many) if you replace 2 lines
model.add(LSTM(n_neurons, input_shape=(length, 1), return_sequences=True))
model.add(TimeDistributed(Dense(1)))
with
# model.add(LSTM(n_neurons, input_shape=(length, 1), return_sequences=True))
model.add(TimeDistributed(Dense(1, use_bias=False), input_shape=(length, 1)))
comment out LSTM at all and even prevent bias in Dense layer, you will get exactly the same result!
That’s why there are so many questions in comments how to apply LSTM to sequences to predict next element.
The post is about demonstrating the architecture, not best modeling the problem.
Perhaps I should have made that clearer.
Hi Jason,
x_train = (31499, 100, 50)
y_train = (31499, 50, 15097)
Which LSTM model should i be using?
If many to many then what would be the architecture
Perhaps an encoder-decoder. You can start here:
https://machinelearningmastery.com/how-to-develop-lstm-models-for-time-series-forecasting/
hi Jason
thank you very much for your useful articles.
I have a question which I will be thankful if you help me. I am trying to Implement a two-layer LSTM based model for document classification. I have pretrained word vectors. now I want to use the first layer of LSTM to produce sentence representation for each sentence in the document and then give it to the next layer for document classification. this https://www.aclweb.org/anthology/D15-1167 is the model that I want to implement. my problem is that I don’t know how to implement the first layer to give a sentence representation for each sentence. the problem is that each document is made of diffrent sentences and each sentence is made of diffrent words, therfore my input for each document is this:
[ [w ,w, w …] , [w ,w ,w …]…., [ w,w,w …] ]
and each w is a vector of size 300. now I need multiple LSTM in the first layer to get a representation for each sentence . how should I implement multiple LSTM in one layer ? can I use timedistributed?
I am not familiar with that paper sorry, perhaps contact the author to see if they have sample code?
If you want to stack LSTM layers, perhaps this will help:
https://machinelearningmastery.com/stacked-long-short-term-memory-networks/
thank you for your answer, I don’t want to stack Lstm on each other, I just need to have an LSTM for each sentence in the first layer. for example, if my document has 4 sentences, I need for LSTM so I can feed each sentence to it and each sentence has for example 10 words. there for each LSTM has 10 time step. therefore my input is (documents, sentences, words, word_feature), and the problem is that LSTM should have (samples.timesteps, feature) and mine is (sample, timesteps, timesteps, feature). any idea, please? I really need it.
Good question.
Perhaps you can use a time distributed wrapper for the second LSTM, that way the first LSTM processes sentences and encodes them, the second takes the encoded sentences and builds up an encoding of the whole document. Much like we would for a CNN-LSTM or a ConvLSTM.
It’s a cool idea. Let me know how you go?
thank you very much. actually I used it and it seems like to work although I am not sure if it does what I want. I used time distributed wrapper over embeding layer and then over the first Lstm. this is the model that I have implemented (very simple one):
model.add(tf.keras.layers.TimeDistributed(embeding_layer))
model.add(tf.keras.layers.TimeDistributed (layers.LSTM(50,activation=’relu’)))
model.add(layers.LSTM(50,activation=’relu’))
model.add(layers.Dense(1,activation=’sigmoid’))
would please chek if my interpretation of the network works is correct?
my interpretation :
my input to the embedding layer is (document, sentences, words). I padded the document to have 30 sentences and I also padded the sentences to have at 200 words. I have 20000 documents so my input shape is (20000,30,200). after feeding it to the network it first go through emeding layer which is 300 length for each word vector. so after applying embeding layer to first docuemnt with shape (1.30,200), then I get (1,30,200,300) which would be the input for the timedistributed LSTM. then time distribut, will make 30 copy of LSTM layer with shared wights where each LSTM will output a sentece vector, and then the next LSTM will be applied to this 30 sentence vectors. am I right ?
I can’t tell from looking at the code, sorry.
by the way this http://ir.hit.edu.cn/~dytang/paper/emnlp2015/codes.zip the link of codes which are in java and I can not understand what he has done.
Sorry, I don’t have the capacity to review your linked java code.
can I somehow use TimeDistributed to implement it? I really appreciate your help.
Exactly!
Also see the CNN-LSTM in this post:
https://machinelearningmastery.com/how-to-develop-lstm-models-for-time-series-forecasting/
I have another question, please! I have to implement a costume LSTM which is like this:
it = sigmoid(Wi · [ht−1; st] + bi)
ft = sigmoid(Wf · [ht−1; st] + bf )
gt = tanh(Wr · [ht−1; st] + br)
ht = tanh(it gt + ft ht−1)
I know that I should make a subclass of LSTM class, but then what ? I looked up at the LSTM class’s code, there is a function named ‘step()’ that you can easily change the units there and make the customized LSTM, but when I inherit from it, I can not find step method so I can override it. would please help me ?
Sorry, Id on’t have a tutorial on writing custom LSTM layers.
Hi Jason,
I appreciate your work and it is the lone tutorial on this topic till date.
But I still don’t get it, do you have some video on the same or can you explain me over some conference platform.
Really looking forward to it.
I will be grateful
Thanks.
Sorry, I don’t make videos.
Hi Jason, after reading all that i also feel like my head is exploding, why isn’t yours :)?
You’re putting so much stuff on, i can’t follow so fast. Thanks for sharing all this!
All the best to you and your family…
Regards from Berlin…
Thanks, I’m happy you found it useful.
Yes, there’s tons of tutorials on the site now. Over 800! It’s a little crazy.
Hi, thank you for the article. I have one question about what model to choose.
Are architectures with RepeatVector and TimeDistributed layers more advanced than “simple” Recurrent encoder-decoder networks? If that’s so, why? What are the technical reasons that make them better?
Using repeatvector and the timedistributed wrapper is a simpler implementation of the encoder-decoder architecture. It is based on the LSTM autoencoder:
https://machinelearningmastery.com/lstm-autoencoders/
Hi
In your example
Many-to-Many LSTM for Sequence Prediction (with TimeDistributed)
I changed one line of the the code from:
model.add(TimeDistributed(Dense(1)))
To
model.add(Dense(1))
And the accuracy is still the same, and the model output shape is also exactly the same with or without TimeDistributed!
How is this possible?
Thanks
What about the number of model parameters?
Yes that’s the difference.
Thanks
Ah the puzzle solved:
https://stackoverflow.com/questions/47305618/what-is-the-role-of-timedistributed-layer-in-keras/47309453#47309453
Thanks
Hey Jason,
question regarding many-to-many with TimeDistributed:
You set return_sequence=True, so the LSTM layer outputs the hidden state of every unit, which is in this case 5(thinking of 1 for each timestep) because the number of units of the LSTM layer is set to 5.
So do I have to set the number of units in the LSTM layer equal to the number of timesteps?
What happens, if I have more units than timesteps and try to do this approach?
Thanks for your help,
Philipp
Yes, you can set the number of units to anything you wish. It only impacts the “features” of the output, not the time steps.
Hey Jason,
Could we have a blend of CNN with RNN or LSTM example.
Lets say we have Images of progressing stages of face over time, or progressing stages of cancer cell. How could we blend Image and Sequence together.
FOr one person we may have 7 images, and another we may have 12. The time diffrence between images may range from one month to 1 year.
Yes, it is a cnn-lstm and you can lern about it here:
https://machinelearningmastery.com/cnn-long-short-term-memory-networks/
Hey Jason:
I have been following your tutorials, and they have been great! Thanks for those great materials.
I am trying to build a CRN network for regression problem, namely speech enhancement. The input is a noisy spectrogram of 161(frequency bins ) by 8 (time stamps) and target output is clean spectrogram of the same shape. I use convolution layers to pull features and reshape them to 8 (time stamps) by 1024 (feature ) and use 2 lstm with input shape (8,1024) and return sequence. I use a final dnn layer trying to map (8,1024) to (8,161) and do a reshape to match output shape. Currently I use flatten and a dnn layer for regression and then map them back to (8,161). Is TimeDistributed(Dense(161)) a better choice in this scenario? Also, i did not use timedistributed cnn like you introduced in another tutorial, is that okay?
Best and stay safe!
Perhaps evaluate both approaches and compare the results?
Thank you Jason for this useful explaination of the use of the TimeDistributed wrapper. I have 2 questions, one tecnical, and the other one more theoretical:
1- I have tryied to using a dense layer after an LSTM layer with the return_sequences=True flag , and the model fit and predict as expected (seq in input, seq in output). Is maybe tensorflow and keras updated to handle this automatically?
2- Assume I have a many_to_one task. Could I have any benefit from training the model as it was a many_to_many task, and then taking the last element only of the prediction?
Thank you very muck for your kindness.
Bests
First point already solved reading more carefully the start of you post. Sorry 🙂
Great!
Yes, I think the API changed and it now handles it automatically.
Maybe, test and discover the answer for your model and data.
What about the second point? Do you have any insights to suggest?
I don’t think there would be benefit, but run the experiment and discover the answer for your specific model and data.
LSTM network in R for time series prediction.
I have an univariate monthly time series of size 64. I’d like to make a multi-step forecast – the last three month values (266, 286 and 230) – using the remaining months as the training set.
data <- c(113, 55, 77, 114, 73, 72, 75, 135, 84, 66, 167, 93, 83,
164, 76, 97, 148, 74, 76, 173, 70, 86, 167, 37, 1, 49,
48,37, 117, 178, 167, 177, 295, 167, 224, 225, 198, 217, 220, 175,
360, 289, 209, 369, 287, 249, 336, 219, 288, 248, 370, 296, 337,
246, 377, 324, 288, 367, 309, 128, 382, 266, 286, 230)
In order to model a LSTM network I am shaping the training/testing data the following way:
X_train = [55,6,1] # 6 timesteps (t-6,t-5,t-4,t-3,t-2,t-1)
Y_train = [55,3,1] # forecast horizon (t+1,t+2,t+3)
X_test = [1,6,1]
Y_test = [1,3,1]
However, when I set up the LSTM as below I get an error
Error in py_call_impl(callable, dots$args, dots$keywords) :
ValueError: Error when checking target: expected time_distributed_16 to have
shape (6, 1) but got array with shape (3, 1)
model %
layer_lstm(
units = 32,
batch_input_shape = c(1, 6, 1),
dropout = 0.2,
recurrent_dropout = 0.2,
return_sequences = TRUE
) %>% time_distributed(layer_dense(units = 1))
model %>%
compile(loss = FLAGS$loss, optimizer = optimizer, metrics =
list(“mean_squared_error”))
history % fit(x = X_train,
y = Y_train,
batch_size = 1,
epochs = 100,
callbacks = callbacks)
I am struggling with this error. Does anybody know the conceptual mistake of this modeling? Thanks in advance.
This is a common question that I answer here:
https://machinelearningmastery.com/faq/single-faq/can-you-read-review-or-debug-my-code
Can you please explain what would happen if you don’t use TimeDistributed in Many-to-many?
I understood what it does technically, but why is this required? What problem/issue does it intend to solve?
The difference is the number of weights and structure of the model.
It would probably output a vector rather than reuse the same output model for each output time step. Try it and see.
Hi Jason,
Tankhs for sharing nice tutorials. I have an issue with timedistributed layer. It has memory leak. I am using tensorflow v2.2.0 and v2.0 and both of them throw exception during training. Have you ever encounter such problem? There is also an issue on github (https://github.com/tensorflow/tensorflow/issues/33178), problem seems fixed but the problem still exists? If you have an expreince with this problem, could you please share it? Thanks.
I have not seen this problem.
Perhaps ensure you are using keras 2.3 on top of tensorflow.
Hi Jason,
I am trying to use a Time Distributed layer with LSTM. But, I want to pass the initial_state of the LSTM as well. I am not able to use ‘initial_state’ when the LSTM is wrapped within the TimeDistributed layer. How can this be fixed?
Thanks
I’m not sure off the cuff, perhaps this will give you ideas:
https://machinelearningmastery.com/return-sequences-and-return-states-for-lstms-in-keras/
Hi Jason.
Thank you for your great articles.
I noticed that you use n_batches for batch_size parameter which can be confusing since n_batches: “number of batches” equals “number of samples” divided by batch_size: “number of samples per batch”.
Thanks!
Great point.
Hi Jason,
I loved the article. I am stuck at a thing and would very much appreciate if you can kindly help me here. I am trying to build a hierarchical attention netwrok model using ALBERT.
def build_model(*, max_senten_num, max_seq_length, n_labels=768, lstm_units=100) -> Model:
REG_PARAM = 1e-13
l2_reg = regularizers.l2(REG_PARAM)
in_id = Input(shape=(max_seq_length,), dtype=tf.int32, name=”input_word_ids”)
in_mask = Input(shape=(max_seq_length,), dtype=tf.int32, name=”input_mask”)
in_segment = Input(shape=(max_seq_length,), dtype=tf.int32, name=”segment_ids”)
albert_layer = hub.KerasLayer(“https://tfhub.dev/tensorflow/albert_en_base/1″,
trainable=True)
pooled_output, sequence_output = albert_layer([in_id, in_mask, in_segment])
albert_inputs = [in_id, in_mask, in_segment]
albert_output = pooled_output
albert_output = RepeatVector(1)(albert_output)
word_lstm = Bidirectional(LSTM(lstm_units, return_sequences=True, kernel_regularizer=l2_reg,
name=”Word-LSTM”))(albert_output)
word_batch_norm = LayerNormalization(name=”Word-LayerNorm”)(word_lstm)
word_dense = TimeDistributed(Dense(200, kernel_regularizer=l2_reg, name=”Word-Dense”))(word_batch_norm)
word_att = AttentionWithContext()(word_dense)
word_encoder = Model(albert_inputs, word_att)
sent_in_id = Input(shape=(max_senten_num, max_seq_length), dtype=tf.int32, name=”input_word_ids”)
sent_in_mask = Input(shape=(max_senten_num, max_seq_length), dtype=tf.int32, name=”input_sent_mask”)
sent_in_segment = Input(shape=(max_senten_num, max_seq_length), dtype=tf.int32, name=”input_sent_segment_ids”)
sent_inputs = [sent_in_id, sent_in_mask, sent_in_segment]
sent_encoder = TimeDistributed(word_encoder)(sent_inputs)
sent_lstm = Bidirectional(LSTM(lstm_units, return_sequences=True, kernel_regularizer=l2_reg,
name=”Sent-LSTM”))(sent_encoder)
sent_dense = TimeDistributed(Dense(200, kernel_regularizer=l2_reg, name=”Sent-Dense”))(sent_lstm)
sent_att = AttentionWithContext()(sent_dense)
sent_dropout = Dropout(0.5, name=”Sent-Dropout”)(sent_att)
sent_batch_norm = LayerNormalization(name=”Sent-LayerNorm”)(sent_dropout)
preds = Dense(n_labels, name=”Prediction-Dense”)(sent_batch_norm)
cosine_loss = tf.keras.losses.CosineSimilarity(axis=1)
model = Model(sent_inputs, preds)
model.compile(optimizer=’adam’, loss=cosine_loss)
print(model.summary())
return model
*************************************************************************************
but the line – “sent_encoder = TimeDistributed(word_encoder)(sent_inputs)” is causing a problem. here I am trying to pass a list of inputs to the time distributed layer. But i am getting the error –
TypeError: Dimension value must be integer or None or have an __index__ method, got value ‘TensorShape([None, 200, 50])’ with type ”
Thanks.
This is a common question that I answer here:
https://machinelearningmastery.com/faq/single-faq/can-you-read-review-or-debug-my-code
Hi Jason, another great work.
Q: Can I use Time distributed when inputs and outputs have different dimensions?
i.e inputs (5400 samples, 46 time steps, 1 feature) outputs (5400 samples, 23 time steps, 1 feature).
You would need a seq2seq model in that case. A timedistributed layer could be used in your seq2seq model.
Thanks, do you have any example of this?
Yes, 100s of tutorials on the blog, use the search.
Perhaps start here:
https://machinelearningmastery.com/how-to-develop-lstm-models-for-time-series-forecasting/
Hi Jason ,
With the time-series forecasting using a univariate model for multiple steps
I evaluated the use of TimeDistributed Layer with CNN-LSTM model, in 2 different situations:
1- using TimeDistributed Layer in the encoder part with Conv1d
2- using TimeDistributed Layer in the decoder part with Dense
with trying different hyperparameters.
I found the first situation to give better predictions.
Do you have any guessing why that happened? So, why using TimeDistributed Layer in the encoder part was better? does that mean it applies the convolution operation for each time step?
I will appreciate if you can help me to understand that in simple English.
Thanks in Advance,
They are very different.
The “why” depends on your data and model. We cannot really answer why questions in applied machine learning, only what, as in what works.
Hi Jason,
Thanks for your reply.
You said “They are very different.”, could you tell different in what exactly, please ?
Best Regards,
Yes, in the first case you are reusing the encoder model for each input time step, in the second case you are reusing the decoder model for each output time step.
Jason, I talked to you in a previous post that I am regenerating many to many seq2seq texts and I used byte level instead of character level and it remarkably regenerates text to a very low error, where each text is distinct from other.
My question if I am adding a two fully connected dense layer to a new model with the weights of the pretrained one I told you about, do I include time distributed wrapper for both fully connected layers I added(one has has lets say 512 units(some activation) the other 1 unit(sigmoid))?
Thanks!
Perhaps try it and compare the results.
Hi Jason, your tutorials are amazing.
I am trying to create a prediction of the next word in a sequence of time stamped words. What are your thoughts about this approach:
Using a word embedding, convert the words to vectors then use the many-to-one LSTM prediction with TimeDistributed wrapper to get the prediction, one at a time.
Thanks!
Perhaps try it and see if it is effective.
Hi Jason.
Great article. I think it’s great that you’re still replying to comments on a 3 years old article.
Question:
I’m trying to build a model where there is a TimeDistributed dense layer before my LSTM layer. Because it’s the first layer, I need to declare the input shape. However, I’m not sure on how to do this. Do you have an idea on how to approach this?
Currently I’m trying something like this:
model = Sequential()
model.add(TimeDistributed(Dense(64), input_shape=(timesteps, features)))
model.add(LSTM(128))
model.add(Dense(3, activation=’softmax’))
model.compile(loss=’categorical_crossentropy’, optimizer=’adam’, metrics=[‘accuracy’])
But I’m afraid that I might be doing this incorrectly.
Hmmm. Good question.
You might need to experiment, but off the cuff, perhaps try the functional API so you can separate the Input layer from the timedistributed layer:
https://machinelearningmastery.com/keras-functional-api-deep-learning/
Hey Jason, Thanks for your helpful content. can you please help me with my question since i couldn’t understand by searching around the web.
I propose to have 5 different inputs to several LSTMs. As, their weights are the shared, I am thinking whether using Timedistruted wrapper can help or not. To be explicit, I have (5, 2, 2) shape. I want to make a convolution among each of 5 lstm’s output to the rest of my network. is it right to use a Timedistributed(lstm(shape=(2,2)) and then finding a way to do the convolution?
You’re welcome.
I think you’re talking about a CNN-LSTM, if so perhaps this will help:
https://machinelearningmastery.com/cnn-long-short-term-memory-networks/
Hi Jason
I still confused, about time distributed. my point is if we set the return_sequence =True
let’ say we have 3 sequence:
inputs1 = Input(shape=(3, 1))
lstm1 = LSTM(1,return_sequences=True)(inputs1)
x = Dense(1)(lstm1)
model = Model(inputs=inputs1, outputs=x)
Dense layer get 3 input sequence from LSTM output, But this 3 input sequence will be multiply to the same weight of Dense layer(share weight)
so what’s the purpose of timedistributed(as you said it’s allowed share weight)?
Thanks
Mike
In your case without the time distributed layer, the dense layer is interpreting the vector output of the LSTM layer directly. With the time distributed layer the dense layer is a sub model that will process each step of the of output separately (I think – totally off the cuff).
Hello Jason
What is the significance of “timestep”? what it actually means ? does it affects how output is produced? what relation it has with samples? When you say input as 1 sample, 5 timesteps,1 feature , does this mean that same input is fed into LSTM 5 times to produce one output?
Good question, see this:
https://machinelearningmastery.com/faq/single-faq/what-is-the-difference-between-samples-timesteps-and-features-for-lstm-input
Thanks Jason
if my input have 1000 samples ,250 timestep and 1 feature, Am I right in assuming that the a state less LSTM will update its weights after 1000/250=4 samples being fed ?
Regardless of the size of your data, the model weights are updated at the end of each batch.
Hi,
Thanks for your great tutorial! I wonder whether n_neurons needs to be equal to length (5) or it just happened to be 5.
Cheers,
Trung
The number of nodes/neurons is unrelated to the number of time steps, you can learn more here:
https://machinelearningmastery.com/faq/single-faq/what-is-the-difference-between-samples-timesteps-and-features-for-lstm-input
Hi,
My objective is to predict if a word is skill/notskill so my output is 1/0. My question is since I am passing a sentence it can have any no. of words and for each word it has to predcit 1/0. So this is many-to-many problem or many-to-one.
Sounds like many to many to me.
Hi, I’ve ran on this tutorial while researching my problem and it’s amazing. I have just one question and hoping you can help me:
I have multiple time series (25 time series, 52 weeks each). In order to predict all of them, I’ve used following arhitecture:
data.shape # (52, 25)
batch_size=128
units = 64
generator = TimeseriesGenerator(data, data, length=25, batch_size=batch_size)
model = Sequential()
model.add(LSTM(units, return_sequences=False, input_shape=(data.shape[0]/2, data.shape[1])))
model.add(Dense(data.shape[1]))
so this way I’m training on all data and returning predictions for all 25 time series.
I hope this is correct formulation and I haven’t missed something important.
Also I’d like to include external regressors here, but I’m struggling how to organize data.
I’ve generated separate dataset with 35 features with shape (52, 35)
all_data = np.dstack((np.expand_dims(data.T, axis=2), np.reshape(np.tile(xreg.values,(data.shape[1], 1)), (data.shape[1],data.shape[0],xreg.shape[1]))))
this way I’ve got data shaped like (25, 52, 35) – num samples, timestaps, features
but I’m not sure how to use TimeseriesGenerator and what should be input size in LSTM layer for this type of data?
TimeseriesGenerator is just a utility function to give you overlapping windows from a time series. See the documentation here: https://www.tensorflow.org/api_docs/python/tf/keras/preprocessing/sequence/TimeseriesGenerator
If you run the example code there (I suggest you to use sampling_rate=1 instead), and check out data_gen[0], data_gen[1], data_gen[2], you should see what I mean.
Hello. I have a question. I couldn’t solve it for very long time. If you respond, I would be glad.
I am using LSTM to predict some graphs. Problem is there are many graphs, not 1. I tried to be clear in below.
In classical prediction problem, for example temperature prediction problem, there are rows for different days and there are columns which represents features that affecting the temperature. Lets say 1000 day and 4 features and looking only for temperature. And we are looking the problem in the 5 time steps. So, shape of x is (1000,5,4) and shape of y is (1000,1). This works in LSTM without problem I know.
BUT, in my problem, there are many of those predictions. I mean only one of the sample composed of 1000 days, 5 time steps and 4 features. There are 126 graphs. So, when I stack them, shape of x=(126,1000,5,4) and shape of y is (126,1000,1).
I guess it is like predicting a temperature of one city with looking to another cities. Relevant code is below:
#Time distributed lstm
inp = Input(shape=(1000, 5, 4))
lstm1 = TimeDistributed(LSTM(64))(inp)
dense1=Dense(8,”relu”)(lstm1)
dense2=TimeDistributed(Dense(1,”linear”))(dense1)
model_td = Model(inp, dense2)
model_td.summary()
When I feed this model with input have shape as (126,1000,5,4) and output shape as (126,1000,1). It works but not adequately. It must seperate 1000 rows in 5 time steps, having 4 features. But I think I have a problem in my code.
Can you help me to prepare data for LSTM and the model?
Thanks.