Keras is a deep learning library that wraps the efficient numerical libraries Theano and TensorFlow.
In this post, you will discover how to develop and evaluate neural network models using Keras for a regression problem.
After completing this step-by-step tutorial, you will know:
- How to load a CSV dataset and make it available to Keras
- How to create a neural network model with Keras for a regression problem
- How to use scikit-learn with Keras to evaluate models using cross-validation
- How to perform data preparation in order to improve skill with Keras models
- How to tune the network topology of models with Keras
Kick-start your project with my new book Deep Learning With Python, including step-by-step tutorials and the Python source code files for all examples.
Let’s get started.
- Jun/2016: First published
- Update Mar/2017: Updated for Keras 2.0.2, TensorFlow 1.0.1 and Theano 0.9.0
- Update Mar/2018: Added alternate link to download the dataset as the original appears to have been taken down
- Update Apr/2018: Changed nb_epoch argument to epochs
- Update Sep/2019: Updated for Keras 2.2.5 API
- Update Jul/2022: Update for TensorFlow 2.x syntax with SciKeras
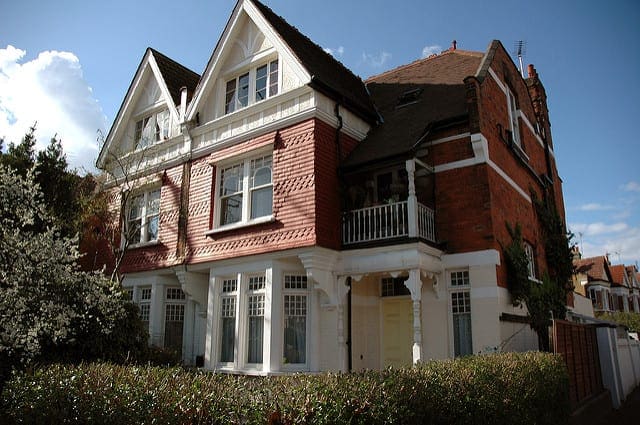
Regression tutorial with Keras deep learning library in Python
Photo by Salim Fadhley, some rights reserved.
1. Problem Description
The problem that we will look at in this tutorial is the Boston house price dataset.
You can download this dataset and save it to your current working directly with the file name housing.csv (update: download data from here).
The dataset describes 13 numerical properties of houses in Boston suburbs and is concerned with modeling the price of houses in those suburbs in thousands of dollars. As such, this is a regression predictive modeling problem. Input attributes include crime rate, the proportion of nonretail business acres, chemical concentrations, and more.
This is a well-studied problem in machine learning. It is convenient to work with because all the input and output attributes are numerical, and there are 506 instances to work with.
Reasonable performance for models evaluated using Mean Squared Error (MSE) is around 20 in thousands of dollars squared (or $4,500 if you take the square root). This is a nice target to aim for with our neural network model.
Need help with Deep Learning in Python?
Take my free 2-week email course and discover MLPs, CNNs and LSTMs (with code).
Click to sign-up now and also get a free PDF Ebook version of the course.
2. Develop a Baseline Neural Network Model
In this section, you will create a baseline neural network model for the regression problem.
Let’s start by including all the functions and objects you will need for this tutorial.
1 2 3 4 5 6 7 8 9 |
import pandas as pd from tensorflow.keras.models import Sequential from tensorflow.keras.layers import Dense from scikeras.wrappers import KerasRegressor from sklearn.model_selection import cross_val_score from sklearn.model_selection import KFold from sklearn.preprocessing import StandardScaler from sklearn.pipeline import Pipeline ... |
You can now load your dataset from a file in the local directory.
The dataset is, in fact, not in CSV format in the UCI Machine Learning Repository. The attributes are instead separated by whitespace. You can load this easily using the pandas library. Then split the input (X) and output (Y) attributes, making them easier to model with Keras and scikit-learn.
1 2 3 4 5 6 7 |
... # load dataset dataframe = pd.read_csv("housing.csv", delim_whitespace=True, header=None) dataset = dataframe.values # split into input (X) and output (Y) variables X = dataset[:,0:13] Y = dataset[:,13] |
You can create Keras models and evaluate them with scikit-learn using handy wrapper objects provided by the Keras library. This is desirable, because scikit-learn excels at evaluating models and will allow you to use powerful data preparation and model evaluation schemes with very few lines of code.
The Keras wrappers require a function as an argument. This function you must define is responsible for creating the neural network model to be evaluated.
Below, you will define the function to create the baseline model to be evaluated. It is a simple model with a single, fully connected hidden layer with the same number of neurons as input attributes (13). The network uses good practices such as the rectifier activation function for the hidden layer. No activation function is used for the output layer because it is a regression problem, and you are interested in predicting numerical values directly without transformation.
The efficient ADAM optimization algorithm is used, and a mean squared error loss function is optimized. This will be the same metric you will use to evaluate the performance of the model. It is a desirable metric because taking the square root gives an error value you can directly understand in the context of the problem (thousands of dollars).
If you are new to Keras or deep learning, see this Keras tutorial.
1 2 3 4 5 6 7 8 9 10 |
... # define base model def baseline_model(): # create model model = Sequential() model.add(Dense(13, input_shape=(13,), kernel_initializer='normal', activation='relu')) model.add(Dense(1, kernel_initializer='normal')) # Compile model model.compile(loss='mean_squared_error', optimizer='adam') return model |
The Keras wrapper object used in scikit-learn as a regression estimator is called KerasRegressor. You create an instance and pass it both the name of the function to create the neural network model and some parameters to pass along to the fit() function of the model later, such as the number of epochs and batch size. Both of these are set to sensible defaults.
The final step is to evaluate this baseline model. You will use 10-fold cross validation to evaluate the model.
1 2 3 4 |
... kfold = KFold(n_splits=10) results = cross_val_score(estimator, X, Y, cv=kfold, scoring='neg_mean_squared_error') print("Results: %.2f (%.2f) MSE" % (results.mean(), results.std())) |
After tying this all together, the complete example is listed below.
1 2 3 4 5 6 7 8 9 10 11 12 13 14 15 16 17 18 19 20 21 22 23 24 25 26 27 |
# Regression Example With Boston Dataset: Baseline from pandas import read_csv from tensorflow.keras.models import Sequential from tensorflow.keras.layers import Dense from scikeras.wrappers import KerasRegressor from sklearn.model_selection import cross_val_score from sklearn.model_selection import KFold # load dataset dataframe = read_csv("housing.csv", delim_whitespace=True, header=None) dataset = dataframe.values # split into input (X) and output (Y) variables X = dataset[:,0:13] Y = dataset[:,13] # define base model def baseline_model(): # create model model = Sequential() model.add(Dense(13, input_shape=(13,), kernel_initializer='normal', activation='relu')) model.add(Dense(1, kernel_initializer='normal')) # Compile model model.compile(loss='mean_squared_error', optimizer='adam') return model # evaluate model estimator = KerasRegressor(model=baseline_model, epochs=100, batch_size=5, verbose=0) kfold = KFold(n_splits=10) results = cross_val_score(estimator, X, Y, cv=kfold, scoring='neg_mean_squared_error') print("Baseline: %.2f (%.2f) MSE" % (results.mean(), results.std())) |
Running this code gives you an estimate of the model’s performance on the problem for unseen data.
Note: Your results may vary given the stochastic nature of the algorithm or evaluation procedure, or differences in numerical precision. Consider running the example a few times and compare the average outcome.
Note: The mean squared error is negative because scikit-learn inverts so that the metric is maximized instead of minimized. You can ignore the sign of the result.
The result reports the mean squared error, including the average and standard deviation (average variance) across all ten folds of the cross validation evaluation.
1 |
Baseline: -32.65 (23.33) MSE |
3. Modeling the Standardized Dataset
An important concern with the Boston house price dataset is that the input attributes all vary in their scales because they measure different quantities.
It is almost always good practice to prepare your data before modeling it using a neural network model.
Continuing from the above baseline model, you can re-evaluate the same model using a standardized version of the input dataset.
You can use scikit-learn’s Pipeline framework to perform the standardization during the model evaluation process within each fold of the cross validation. This ensures that there is no data leakage from each test set cross validation fold into the training data.
The code below creates a scikit-learn pipeline that first standardizes the dataset and then creates and evaluates the baseline neural network model.
1 2 3 4 5 6 7 8 9 |
... # evaluate model with standardized dataset estimators = [] estimators.append(('standardize', StandardScaler())) estimators.append(('mlp', KerasRegressor(model=baseline_model, epochs=50, batch_size=5, verbose=0))) pipeline = Pipeline(estimators) kfold = KFold(n_splits=10) results = cross_val_score(pipeline, X, Y, cv=kfold, scoring='neg_mean_squared_error') print("Standardized: %.2f (%.2f) MSE" % (results.mean(), results.std())) |
After tying this together, the complete example is listed below.
1 2 3 4 5 6 7 8 9 10 11 12 13 14 15 16 17 18 19 20 21 22 23 24 25 26 27 28 29 30 31 32 |
# Regression Example With Boston Dataset: Standardized from pandas import read_csv from tensorflow.keras.models import Sequential from tensorflow.keras.layers import Dense from scikeras.wrappers import KerasRegressor from sklearn.model_selection import cross_val_score from sklearn.model_selection import KFold from sklearn.preprocessing import StandardScaler from sklearn.pipeline import Pipeline # load dataset dataframe = read_csv("housing.csv", delim_whitespace=True, header=None) dataset = dataframe.values # split into input (X) and output (Y) variables X = dataset[:,0:13] Y = dataset[:,13] # define base model def baseline_model(): # create model model = Sequential() model.add(Dense(13, input_shape=(13,), kernel_initializer='normal', activation='relu')) model.add(Dense(1, kernel_initializer='normal')) # Compile model model.compile(loss='mean_squared_error', optimizer='adam') return model # evaluate model with standardized dataset estimators = [] estimators.append(('standardize', StandardScaler())) estimators.append(('mlp', KerasRegressor(model=baseline_model, epochs=50, batch_size=5, verbose=0))) pipeline = Pipeline(estimators) kfold = KFold(n_splits=10) results = cross_val_score(pipeline, X, Y, cv=kfold, scoring='neg_mean_squared_error') print("Standardized: %.2f (%.2f) MSE" % (results.mean(), results.std())) |
Note: Your results may vary given the stochastic nature of the algorithm or evaluation procedure, or differences in numerical precision. Consider running the example a few times and compare the average outcome.
Running the example provides an improved performance over the baseline model without standardized data, dropping the error.
1 |
Standardized: -29.54 (27.87) MSE |
A further extension of this section would be to similarly apply a rescaling to the output variable, such as normalizing it to the range of 0-1 and using a Sigmoid or similar activation function on the output layer to narrow output predictions to the same range.
4. Tune the Neural Network Topology
Many concerns can be optimized for a neural network model.
Perhaps the point of biggest leverage is the structure of the network itself, including the number of layers and the number of neurons in each layer.
In this section, you will evaluate two additional network topologies in an effort to further improve the performance of the model. You will look at both a deeper and a wider network topology.
4.1. Evaluate a Deeper Network Topology
One way to improve the performance of a neural network is to add more layers. This might allow the model to extract and recombine higher-order features embedded in the data.
In this section, you will evaluate the effect of adding one more hidden layer to the model. This is as easy as defining a new function to create this deeper model, copied from your baseline model above. You can then insert a new line after the first hidden layer—in this case, with about half the number of neurons.
1 2 3 4 5 6 7 8 9 10 11 |
... # define the model def larger_model(): # create model model = Sequential() model.add(Dense(13, input_shape=(13,), kernel_initializer='normal', activation='relu')) model.add(Dense(6, kernel_initializer='normal', activation='relu')) model.add(Dense(1, kernel_initializer='normal')) # Compile model model.compile(loss='mean_squared_error', optimizer='adam') return model |
Your network topology now looks like this:
1 |
13 inputs -> [13 -> 6] -> 1 output |
You can evaluate this network topology in the same way as above, while also using the standardization of the dataset shown above to improve performance.
1 2 3 4 5 6 7 8 |
... estimators = [] estimators.append(('standardize', StandardScaler())) estimators.append(('mlp', KerasRegressor(model=larger_model, epochs=50, batch_size=5, verbose=0))) pipeline = Pipeline(estimators) kfold = KFold(n_splits=10) results = cross_val_score(pipeline, X, Y, cv=kfold, scoring='neg_mean_squared_error') print("Larger: %.2f (%.2f) MSE" % (results.mean(), results.std())) |
After tying this together, the complete example is listed below.
1 2 3 4 5 6 7 8 9 10 11 12 13 14 15 16 17 18 19 20 21 22 23 24 25 26 27 28 29 30 31 32 33 |
# Regression Example With Boston Dataset: Standardized and Larger from pandas import read_csv from tensorflow.keras.models import Sequential from tensorflow.keras.layers import Dense from scikeras.wrappers import KerasRegressor from sklearn.model_selection import cross_val_score from sklearn.model_selection import KFold from sklearn.preprocessing import StandardScaler from sklearn.pipeline import Pipeline # load dataset dataframe = read_csv("housing.csv", delim_whitespace=True, header=None) dataset = dataframe.values # split into input (X) and output (Y) variables X = dataset[:,0:13] Y = dataset[:,13] # define the model def larger_model(): # create model model = Sequential() model.add(Dense(13, input_shape=(13,), kernel_initializer='normal', activation='relu')) model.add(Dense(6, kernel_initializer='normal', activation='relu')) model.add(Dense(1, kernel_initializer='normal')) # Compile model model.compile(loss='mean_squared_error', optimizer='adam') return model # evaluate model with standardized dataset estimators = [] estimators.append(('standardize', StandardScaler())) estimators.append(('mlp', KerasRegressor(model=larger_model, epochs=50, batch_size=5, verbose=0))) pipeline = Pipeline(estimators) kfold = KFold(n_splits=10) results = cross_val_score(pipeline, X, Y, cv=kfold, scoring='neg_mean_squared_error') print("Larger: %.2f (%.2f) MSE" % (results.mean(), results.std())) |
Note: Your results may vary given the stochastic nature of the algorithm or evaluation procedure, or differences in numerical precision. Consider running the example a few times and compare the average outcome.
Running this model shows a further improvement in performance from 28 down to 24 thousand squared dollars.
1 |
Larger: -22.83 (25.33) MSE |
4.2. Evaluate a Wider Network Topology
Another approach to increasing the representational capability of the model is to create a wider network.
In this section, you will evaluate the effect of keeping a shallow network architecture and nearly doubling the number of neurons in the one hidden layer.
Again, all you need to do is define a new function that creates your neural network model. Here, you will increase the number of neurons in the hidden layer compared to the baseline model from 13 to 20.
1 2 3 4 5 6 7 8 9 10 |
... # define wider model def wider_model(): # create model model = Sequential() model.add(Dense(20, input_shape=(13,), kernel_initializer='normal', activation='relu')) model.add(Dense(1, kernel_initializer='normal')) # Compile model model.compile(loss='mean_squared_error', optimizer='adam') return model |
Your network topology now looks like this:
1 |
13 inputs -> [20] -> 1 output |
You can evaluate the wider network topology using the same scheme as above:
1 2 3 4 5 6 7 8 |
... estimators = [] estimators.append(('standardize', StandardScaler())) estimators.append(('mlp', KerasRegressor(model=wider_model, epochs=100, batch_size=5, verbose=0))) pipeline = Pipeline(estimators) kfold = KFold(n_splits=10) results = cross_val_score(pipeline, X, Y, cv=kfold, scoring='neg_mean_squared_error') print("Wider: %.2f (%.2f) MSE" % (results.mean(), results.std())) |
After tying this together, the complete example is listed below.
1 2 3 4 5 6 7 8 9 10 11 12 13 14 15 16 17 18 19 20 21 22 23 24 25 26 27 28 29 30 31 32 |
# Regression Example With Boston Dataset: Standardized and Wider from pandas import read_csv from tensorflow.keras.models import Sequential from tensorflow.keras.layers import Dense from scikeras.wrappers import KerasRegressor from sklearn.model_selection import cross_val_score from sklearn.model_selection import KFold from sklearn.preprocessing import StandardScaler from sklearn.pipeline import Pipeline # load dataset dataframe = read_csv("housing.csv", delim_whitespace=True, header=None) dataset = dataframe.values # split into input (X) and output (Y) variables X = dataset[:,0:13] Y = dataset[:,13] # define wider model def wider_model(): # create model model = Sequential() model.add(Dense(20, input_shape=(13,), kernel_initializer='normal', activation='relu')) model.add(Dense(1, kernel_initializer='normal')) # Compile model model.compile(loss='mean_squared_error', optimizer='adam') return model # evaluate model with standardized dataset estimators = [] estimators.append(('standardize', StandardScaler())) estimators.append(('mlp', KerasRegressor(model=wider_model, epochs=100, batch_size=5, verbose=0))) pipeline = Pipeline(estimators) kfold = KFold(n_splits=10) results = cross_val_score(pipeline, X, Y, cv=kfold, scoring='neg_mean_squared_error') print("Wider: %.2f (%.2f) MSE" % (results.mean(), results.std())) |
Note: Your results may vary given the stochastic nature of the algorithm or evaluation procedure, or differences in numerical precision. Consider running the example a few times and compare the average outcome.
Building the model reveals a further drop in error to about 21 thousand squared dollars. This is not a bad result for this problem.
1 |
Wider: -21.71 (24.39) MSE |
It might have been hard to guess that a wider network would outperform a deeper network on this problem. The results demonstrate the importance of empirical testing in developing neural network models.
Summary
In this post, you discovered the Keras deep learning library for modeling regression problems.
Through this tutorial, you learned how to develop and evaluate neural network models, including:
- How to load data and develop a baseline model
- How to lift performance using data preparation techniques like standardization
- How to design and evaluate networks with different varying topologies on a problem
Do you have any questions about the Keras deep learning library or this post? Ask your questions in the comments, and I will do my best to answer.
Hi did you handle string variables in cross_val_score module?
The dataset is numeric, no string values.
How do we handle string values
Great question, I have a whole section on the topic:
https://machinelearningmastery.com/start-here/#nlp
For some reason my MSE is negative. why?
sklearn will invert mse so that it can be maximized.
One hot encoder is an option.
Hi,
I have
2 input set (that means 2 columns) instead of 13 of this problem
8 output( 8 columns)instead of 1 of this problem
192 training set instead of 506 of this problem
so multi-input multi-output prediction modeling
will this code sufficient or do I have to change anything?
is this deep learning because I heard for deep learning it requires thousand of the training set
forgive me I don’t know anything about deep learning and with this code I am gonna start
I am waiting for your reply
If you are predicting 8 real-valued variables (not 8 classes), you can change the number of nodes in the output layer to 8.
Thank you for your quick response
so, i have to change only the output layer no
Now, i have few more question
If i am able to get the results using this code i have to know some details
1)I suppose it is the latest deep neural network.What is the name of this neural network? (e.g. recurrent, multilayer perceptron, Boltzmann etc)
2)In deep learning parameters are needed to be tuned by varying them
what are the parameters here which i have to vary?
3)Can you send me the image which will show the complete architecture of neural network showing input layer hidden layer output layer transfer function etc.
4)since i will be using this code. I have to refer it in the Journal which i am going to write
should i simply refer this website or any paper of your you suggest me to cite?
For help in tuning your model, I recommend starting here:
https://machinelearningmastery.com/start-here/#better
You can summarize the architecture of your model, learn more here:
https://machinelearningmastery.com/visualize-deep-learning-neural-network-model-keras/
I show how to cite a post or book here:
https://machinelearningmastery.com/faq/single-faq/how-do-i-reference-or-cite-a-book-or-blog-post
Mr. Brownlee,
If I have a multi input and a multi output regression problem, e.g 4 input and 4 output then how do we deal with that.
The model can be defined to expect 4 inputs, and then you can have 4 nodes in the output layer.
Thanks a lot for your kind and prompt reply Mr. Jason.
You’re welcome.
Also in case of a multiple output, do we do the prediction and accuracy the same way we do for on out put case in keras. i am new to deep learning so I am sorry of my question is a bit naive.
You can calculate a score for all outputs and/or for each separate output.
Training, keras will use a single loss, but your project stakeholders may have more requirements when evaluating the final model.
Mr. Jason if I run your code in my system I am getting an error
TypeError: (‘Keyword argument not understood:’, ‘acitivation’)
could you please explain why.
Ganesh
Sorry to hear that, I have some suggestions here:
https://machinelearningmastery.com/faq/single-faq/why-does-the-code-in-the-tutorial-not-work-for-me
You should type “activation”, without the “i” after “c”
# Regression Example With Boston Dataset: Standardized and Wider
import pandas as pd
from keras.models import Sequential
from keras.layers import Dense
from keras.wrappers.scikit_learn import KerasRegressor
from sklearn.model_selection import cross_val_score
from sklearn.model_selection import KFold
from sklearn.preprocessing import StandardScaler
from sklearn.pipeline import Pipeline
# load dataset
dataset = pd.read_csv(‘train1.csv’)
testthedata = pd.read_csv(‘test1.csv’)
# split into input (X) and output (Y) variables
X = dataset.drop(columns = [“Id”, “SalePrice”, “Alley”, “MasVnrType”, “BsmtQual”, “BsmtCond”, “BsmtExposure”,
“BsmtFinType1”, “BsmtFinType2”, “Electrical”, “FireplaceQu”, “GarageType”,
“GarageFinish”, “GarageQual”, “GarageCond”, “PoolQC”, “Fence”, “MiscFeature”])
y = dataset[‘SalePrice’].values
testthedata = testthedata.drop(columns = [“MSZoning”, “Utilities”, “Id”, “Alley”, “MasVnrType”, “BsmtQual”, “BsmtCond”, “BsmtExposure”,
“Exterior1st”, “Exterior2nd”, “BsmtFinType1”, “BsmtFinType2”, “Electrical”, “FireplaceQu”, “GarageType”,
“KitchenQual”, “SaleType”, “Functional”, “GarageFinish”, “GarageQual”, “GarageCond”, “PoolQC”, “Fence”, “MiscFeature”])
from sklearn.preprocessing import LabelEncoder, OneHotEncoder
le = LabelEncoder()
le1 = LabelEncoder()
X[‘MSZoning’] = le.fit_transform(X[[‘MSZoning’]])
X[‘Street’] = le.fit_transform(X[[‘Street’]])
X[‘LotShape’] = le.fit_transform(X[[‘LotShape’]])
X[‘LandContour’] = le.fit_transform(X[[‘LandContour’]])
X[‘LotConfig’] = le.fit_transform(X[[‘LotConfig’]])
X[‘LandSlope’] = le.fit_transform(X[[‘LandSlope’]])
X[‘Utilities’] = le.fit_transform(X[[‘Utilities’]])
X[‘Neighborhood’] = le.fit_transform(X[[‘Neighborhood’]])
X[‘Condition1’] = le.fit_transform(X[[‘Condition1’]])
X[‘Condition2’] = le.fit_transform(X[[‘Condition2’]])
X[‘BldgType’] = le.fit_transform(X[[‘BldgType’]])
X[‘HouseStyle’] = le.fit_transform(X[[‘HouseStyle’]])
X[‘RoofStyle’] = le.fit_transform(X[[‘RoofStyle’]])
X[‘RoofMatl’] = le.fit_transform(X[[‘RoofMatl’]])
X[‘Exterior1st’] = le.fit_transform(X[[‘Exterior1st’]])
X[‘Exterior2nd’] = le.fit_transform(X[[‘Exterior2nd’]])
X[‘ExterQual’] = le.fit_transform(X[[‘ExterQual’]])
X[‘ExterCond’] = le.fit_transform(X[[‘ExterCond’]])
X[‘Foundation’] = le.fit_transform(X[[‘Foundation’]])
X[‘Heating’] = le.fit_transform(X[[‘Heating’]])
X[‘HeatingQC’] = le.fit_transform(X[[‘HeatingQC’]])
X[‘KitchenQual’] = le.fit_transform(X[[‘KitchenQual’]])
X[‘Functional’] = le.fit_transform(X[[‘Functional’]])
X[‘PavedDrive’] = le.fit_transform(X[[‘PavedDrive’]])
X[‘SaleType’] = le.fit_transform(X[[‘SaleType’]])
X[‘SaleCondition’] = le.fit_transform(X[[‘SaleCondition’]])
#testing[‘MSZoning’] = le1.fit_transform(testing[[‘MSZoning’]])
testthedata[‘Street’] = le1.fit_transform(testthedata[[‘Street’]])
testthedata[‘LotShape’] = le1.fit_transform(testthedata[[‘LotShape’]])
testthedata[‘LandContour’] = le1.fit_transform(testthedata[[‘LandContour’]])
testthedata[‘LotConfig’] = le1.fit_transform(testthedata[[‘LotConfig’]])
#testthedata[‘LandSlope’] = le1.testthedata(testthedata[[‘LandSlope’]])
#testing[‘Utilities’] = le1.fit_transform(testing[[‘Utilities’]])
testthedata[‘Neighborhood’] = le1.fit_transform(testthedata[[‘Neighborhood’]])
testthedata[‘Condition1’] = le1.fit_transform(testthedata[[‘Condition1’]])
#testthedata[‘Condition2’] = le1.fit_transform(testthedata[[‘Condition2’]])
testthedata[‘BldgType’] = le1.fit_transform(testthedata[[‘BldgType’]])
testthedata[‘HouseStyle’] = le1.fit_transform(testthedata[[‘HouseStyle’]])
testthedata[‘RoofStyle’] = le1.fit_transform(testthedata[[‘RoofStyle’]])
#testthedata[‘RoofMatl’] = le1.fit_transform(testthedata[[‘RoofMatl’]])
#testing[‘Exterior1st’] = le1.fit_transform(testing[[‘Exterior1st’]])
#testing[‘Exterior2nd’] = le1.fit_transform(testing[[‘Exterior2nd’]])
testthedata[‘ExterQual’] = le1.fit_transform(testthedata[[‘ExterQual’]])
#testthedata[‘ExterCond’] = le1.fit_transform(testthedata[[‘ExterCond’]])
testthedata[‘Foundation’] = le1.fit_transform(testthedata[[‘Foundation’]])
testthedata[‘Heating’] = le1.fit_transform(testthedata[[‘Heating’]])
#testthedata[‘HeatingQC’] = le1.fit_transform(testthedata[[‘HeatingQC’]])
#testing[‘KitchenQual’] = le1.fit_transform(testing[[‘KitchenQual’]])
#testing[‘Functional’] = le1.fit_transform(testing[[‘Functional’]])
testthedata[‘PavedDrive’] = le1.fit_transform(testthedata[[‘PavedDrive’]])
#testing[‘SaleType’] = le1.fit_transform(testing[[‘SaleType’]])
testthedata[‘SaleCondition’] = le1.fit_transform(testthedata[[‘SaleCondition’]])
X[‘MSZoning’] = pd.to_numeric(X[‘MSZoning’])
ohe = OneHotEncoder(categorical_features = [1])
X = ohe.fit_transform(X).toarray()
for this code, the error was coming how to rectify it, sir,
File “”, line 1, in
X = ohe.fit_transform(X).toarray()
File “/Users/p.venkatesh/opt/anaconda3/lib/python3.7/site-packages/sklearn/preprocessing/_encoders.py”, line 629, in fit_transform
self._categorical_features, copy=True)
File “/Users/p.venkatesh/opt/anaconda3/lib/python3.7/site-packages/sklearn/preprocessing/base.py”, line 45, in _transform_selected
X = check_array(X, accept_sparse=’csc’, copy=copy, dtype=FLOAT_DTYPES)
File “/Users/p.venkatesh/opt/anaconda3/lib/python3.7/site-packages/sklearn/utils/validation.py”, line 496, in check_array
array = np.asarray(array, dtype=dtype, order=order)
File “/Users/p.venkatesh/opt/anaconda3/lib/python3.7/site-packages/numpy/core/_asarray.py”, line 85, in asarray
return array(a, dtype, copy=False, order=order)
ValueError: could not convert string to float: ‘Y’
Perhaps this will help:
https://machinelearningmastery.com/faq/single-faq/can-you-read-review-or-debug-my-code
How do I measure performance of a Neural network that has a continuous variable as output since i obviously can’t use accuracy?
You can use an error metric:
https://machinelearningmastery.com/regression-metrics-for-machine-learning/
Hi Jason,
Great tutorial(s) they have been very helpful as a crash course for me so far.
Is there a way to have the model output the estimated Ys in this example? I would like to evaluate the model a little more directly while I’m still learning Keras.
Thanks!
Hi Paul, you can make predictions by calling model.predict()
Hey Paul,
How are you inserting the function model.predict() in the above code to run in on test data? Please let me know.
Hi,
Is this how you insert predict and then get predictions in the model?
def mymodel():
model = Sequential()
model.add(Dense(13, input_dim=13, kernel_initializer=’normal’, activation=’relu’))
model.add(Dense(6, kernel_initializer=’normal’, activation=’relu’))
model.add(Dense(1, kernel_initializer=’normal’))
model.compile(loss=’mean_squared_error’, optimizer=’adam’)
model.fit(X,y, nb_epoch=50, batch_size=5)
predictions = model.predict(X)
return model
I actually want to write the predictions in a file?
DataScientistPM,
He is using Scikit-Learn’s cross-validation framework, which must be calling fit internally.
Correct.
Hi,
but how can you get the prediction for one X value?
If I try this:
y_predict = mymodel.predict(x)
I get this error:
AttributeError: ‘function’ object has no attribute ‘predict’.
I guess it’s because we are calling Scikit-Learn, but don’t guess how to predict a new value.
Here’s how to predict with a sklearn model:
https://machinelearningmastery.com/make-predictions-scikit-learn/
Here’s how to predict with a Keras model:
https://machinelearningmastery.com/how-to-make-classification-and-regression-predictions-for-deep-learning-models-in-keras/
Hi, Great post thank you, Could you please give a sample on how to use Keras LSTM layer for considering time impact on this dataset ?
Thanks
Thanks Chris.
You can see an example of LSTMs on this dataset here:
https://machinelearningmastery.com/time-series-prediction-lstm-recurrent-neural-networks-python-keras/
That was Awesome, thank you Json.
You’re welcome Chris.
Hi,
Thanks for the tutorial. I have a regression problem with bounded outputs (0-1). Is there an opitmal way to deal with this?
Thanks!
Marc
Hi Marc, I think a linear activation function on the output layer will be just fun.
This is a good example. However, it is not relevant to Neural networks when over-fitting is considered. The validation process should be included inside the fit() function to monitor over-fitting status. Moreover, early stopping can be used based on the internal validation step. This example is only applicable for large data compared to the number of all weights of input and hidden nodes.
Great feedback, thanks James I agree.
It is intended as a good example to show how to develop a net for regression, but the dataset is indeed a bit small.
Thanks Jason and James! A few questions (and also how to implement in python):
1) How can we monitor the over-fitting status in deep learning
2) how can we include the cross-validation process inside the fit() function to monitor the over-fitting status
3) How can we use early stopping based on the internal validation step
4) Why is this example only applicable for a large data set? What should we do if the data set is small?
Great questions Amir!
1. Monitor the performance of the model on the training and a standalone validation dataset. (even plot these learning curves). When skill on the validation set goes down and skill on training goes up or keeps going up, you are overlearning.
2. Cross validation is just a method for estimating the performance of a model on unseen data. It wraps everything you are doing to prepare data and your model, it does not go inside fit.
3. Monitor skill on a validation dataset as in 1, when skill stops improving on the validation set, stop training.
4. Generally, neural nets need a lot more data to train than other methods.
Here’s a tutorial on checkpointing that you can use to save “early stopped” models:
https://machinelearningmastery.com/check-point-deep-learning-models-keras/
Hi,
How once can predict new data point on a model while during building the model the training data has been standardised using sklearn.
You can save the object you used to standardize the data and later reuse it to standardize new data before making a prediction. This might be the MinMaxScaler for example.
Can you give an example of how this would be done?
See this:
https://machinelearningmastery.com/standardscaler-and-minmaxscaler-transforms-in-python/
And this:
https://machinelearningmastery.com/how-to-save-and-load-models-and-data-preparation-in-scikit-learn-for-later-use/
Hi,
I am not using the automatic data normalization as you show, but simply compute the mean and stdev for each feature (data column) in my training data and manually perform zscore ((data – mean) / stdev). By normalization I mean bringing the data to 0-mean, 1-stdev. I know there are several names for this process but let’s call it “normalization” for the sake of this argument.
So I’ve got 2 questions:
1) Should I also normalize the output column? Or just leave it as it is in my train/test?
2) I take the mean, stdev for my training data and use them to normalize the test data. But it seems that doesn’t center my data; no matter how I split the data, and no matter that each mini-batch is balanced (has the same distribution of output values). What am I missing / what can I do?
Hi Guy, yeah this is normally called standardization.
Generally, you can get good results from applying the same transform to the output column. Try and see how it affects your results. If MSE or RMSE is the performance measure, you may need to be careful with the interpretation of the results as the scale of these scores will also change.
Yep, this is a common problem. Ideally, you want a very large training dataset to effectively estimate these values. You could try using bootstrap on the training dataset (or within a fold of cross validation) to create a more robust estimate of these terms. Bootstrap is just the repeated subsampling of your dataset and estimation of the statistical quantities, then take the mean from all the estimates. It works quite well.
I hope that helps.
Hello Jason,
How should i load multiple finger print images into keras.
Can you please advise further.
Best Regards,
Pranith
Hi Jason, great tutorial. The best out there for free.
Can I use R² as my metric? If so, how?
Regards
Thanks Luciano.
You can use R^2, see this list of metrics you can use:
http://scikit-learn.org/stable/modules/model_evaluation.html
shouldn’t results.mean() print accuracy instead of error?
We summarize error for regression problems instead of accuracy (x/y correct). I hope that helps.
Hi,
if I have a new dataset, X_new, and I want to make a prediction, the model.predict(X_new) shows the error ”NameError: name model is not defined’ and estimator.predict(X_test) shows the error message ‘KerasRegressor object has no attribute model’.
Do you have any suggestion? Thanks.
Hi David, this post will get you started with the lifecycle of a Keras model:
https://machinelearningmastery.com/5-step-life-cycle-neural-network-models-keras/
Hi Jason,
That page does not use KerasRegressor. How can we save the model and its weights in the code from this tutorial?
Thanks!
I’m getting more error by standardizing dataset using the same seed.What must be the reason behind it?
also deeper network topology seems not to help .It increases the MSE
deeper network without standardisation gives better results.Somehow standardisation is adding more noise
Hey great tutorial. I tried to use both Theano and Tensorflow backend, but I obtained very different results for the larger_model. With Theano I obtained results very similar to you, but with Tensorflow I have MSE larger than 100.
Do you have any clue?
Michele
Great question Michele,
Off the cuff, I would think it is probably the reproducibility problems we are seeing with Python deep learning stack. It seems near impossible to tie down the random number generators used to get repeatable results.
I would not rule out a bug in one implementation or another, but I would find this very surprising for such a simple network.
hi, i have a question about sklearn interface.
although we sent the NN model to sklearn and evaluate the regression performance, how can we get the exactly predictions of the input data X, like usually when we r using Keras we can call the model.predict(X) function in keras. btw, I mean the model is in sklearn right?
Hi Kenny,
You can use the sklearn model.predict() function in the same way to make predictions on new input data.
Hi Jason
I bought the book “Deep Learning with Python”. Thanks for your great work!
I see the question about “model.predict()” quite often. I have it as well. In the code above “model” is undefined. So what variable contains the trained model? I tried “estimator.predict()” but there I get the following error:
> ‘KerasRegressor’ object has no attribute ‘model’
I think it would help many readers
Thanks for your support Silvan.
With a keras model, you can train the model, assign it to a variable and call model.predict(). See this post:
https://machinelearningmastery.com/5-step-life-cycle-neural-network-models-keras/
In the above example, we use a pipeline, which is also a sklearn Estimator. We can call estimator.predict() directly (same function name, different API), more here:
http://scikit-learn.org/stable/modules/generated/sklearn.pipeline.Pipeline.html#sklearn.pipeline.Pipeline.predict
Does that help?
Hey Jason,
Is there anyway for you to provide a direct example of using the model.predict() for the example shown in this post? I’ve been following your posts for a couple months now and have gotten much more comfortable with Keras. However, I still cannot seem to be able to use .predict() on this example.
Thanks!
Hi Dee,
There info on the predict function here:
https://machinelearningmastery.com/5-step-life-cycle-neural-network-models-keras/
There’s an example of calling predict in this post:
https://machinelearningmastery.com/tutorial-first-neural-network-python-keras/
Does that help?
Hi Dee
Jason, correct me if I am wrong: If I understand correctly the sample above does *not* provide a trained model as output. So you won’t be able to use the .predict() function immediately.
Instead you have to train the pipeline:
pipeline.fit(X,Y)
Then only you can do predictions:
pipeline.predict(numpy.array([[ 0.0273, 0. , 7.07 , 0. , 0.469 , 6.421 ,
78.9 , 4.9671, 2. , 242. , 17.8 , 396.9 ,
9.14 ]]))
# will return array(22.125564575195312, dtype=float32)
Yes, thanks for the correction.
Sorry, for the confusion.
Hey Silvan,
Thanks for the tip! I had a feeling that the crossval from SciKit did not output the fitted model but just the RMSE or MSE of the crossval cost function.
I’ll give it a go with the .fit()!
Thanks!
Hi Jason & Silvan,
Could you pls tell me whether I am given “pipeline.fit(X,Y)” in correct position?
pls correct me if I am wrong.
numpy.random.seed(seed)
estimators = []
estimators.append((‘standardize’, StandardScaler()))
estimators.append((‘mlp’, KerasRegressor(build_fn=larger_model, nb_epoch=50, batch_size=5, verbose=0)))
pipeline = Pipeline(estimators)
pipeline.fit(X,Y)
kfold = KFold(n_splits=10, random_state=seed)
results = cross_val_score(pipeline, X, Y, cv=kfold)
print(“Larger: %.2f (%.2f) MSE” % (results.mean(), results.std()))
Thank you!
pipeline.fit is not needed as you are evaluating the pipeline using kfold cross validation.
Dear Jason,
I have a few questions. I am running the wider neural network on a dataset that corresponds to modelling with better accuracy the number of people walking in and out of a store. I get Wider: 24.73 (7.64) MSE. <– Can you explain exactly what those values mean?
Also can you suggest any other method of improving the neural network? Do I have to keep re-iterating and tuning according to different topological methods?
Also what exact function do you use to predict the new data with no ground truth? Is it the sklearn model.predict(X) where X is the new dataset with one lesser dimension because there is no output? Could you please elaborate and explain in detail. I would be really grateful to you.
Thank you
Hi Rahul,
The model reports on Mean Squared Error (MSE). It reports both the mean and the standard deviation of performance across 10 cross validation folds. This gives an idea of the expected spread in the performance results on new data.
I would suggest trying different network configurations until you find a setup that performs well on your problem. There are no good rules for net configuration.
You can use model.predict() to make new predictions. You are correct.
Hey! Jason.
Great work on machine learning. I have learned everything from here.
One question.
When we say that we have to train the model first and then predict, are we trying to determine what no. of layers and what no. of neurons, along with other Keras attributes, to get the best fit…and then use the same attributes on prediction dataset?
Bottom line: are we trying to determine what keras attributes fits our model while we are training the model?
Generally, we want a model that makes good predictions on new data where we don’t know the answer.
We evaluate different models and model configurations on test data to get an idea of how the models will perform when making predictions on new data, so that we can pick one or a few that we think will work well.
Does that help?
Hi Jason,
Thank you for the great tutorial.
I redo the code on a Ubuntu machine and run them on TITAN X GPU. While I get similar results for experiment in section 4.1, my results in section 4.2 is different from yours:
Larger: 103.31 (236.28) MSE
no_epoch is 50 and batch_size is 5.
This can happen, it is hard to control the random number generators in Keras.
See this post:
https://machinelearningmastery.com/randomness-in-machine-learning/
Hi Jason,
Thanks for sharing these useful tutorials. Two questions:
1) If regression model calculates the error and returns as result (no doubt for this) then what is those ‘accuracy’ values printed for each epoch when ‘verbose=1’?
2) With those predicted values (fit.predict() or cross_val_predict), is it meaningful to find the closest value(s) to predicted result and calculate an accuracy? (This way, more than one accuracy can be calculated: accuracy for closest 2, closest 3, …)
Hi A. Batuhan D.,
1. You cannot print accuracy for a regression problem, it does not make sense. It would be loss or error.
2. Again, accuracy does not make sense for regression. It sounds like you are describing an instance based regression model like kNN?
Hi jason,
1. I know, it doesn’t make any sense to calculate accuracy for a regression problem but when using Keras library and set verbose=1, function prints accuracy values also alongside with loss values. I’d like to ask the reason of this situation. It is confusing. In your example, verbose parameter is set to 0.
2. What i do is to calculate some vectors. As input, i’m using vectors (say embedded word vectors of a phrase) and trying to calculate a vector (next word prediction) as an output (may not belong to any known vector in dictionary and probably not). Afterwards, i’m searching the closest vector in dictionary to one calculated by network by cosine distance approach. Counting model predicted vectors who are most similar to the true words vector (say next words vector) than others in dictionary may lead to a reasonable accuracy in my opinion. That’s a brief summary of what i do. I think that it is not related to instance based regression models.
Thanks.
That is very odd that accuracy is printed for a regression problem. I have not seen it, perhaps it’s a new bug in Keras?
Are you able to paste a short code + output example?
Hi,
I tried this tutorial – but it crashes with the following:
Traceback (most recent call last):
File “Riskind_p1.py”, line 132, in
results = cross_val_score(estimator, X, Y, cv=kfold)
File “C:\Python27\lib\site-packages\sklearn\model_selection\_validation.py”, line 140, in cross_val_score
for train, test in cv_iter)
File “C:\Python27\lib\site-packages\sklearn\externals\joblib\parallel.py”, line 758, in __call__
while self.dispatch_one_batch(iterator):
File “C:\Python27\lib\site-packages\sklearn\externals\joblib\parallel.py”, line 603, in dispatch_one_batch
tasks = BatchedCalls(itertools.islice(iterator, batch_size))
File “C:\Python27\lib\site-packages\sklearn\externals\joblib\parallel.py”, line 127, in __init__
self.items = list(iterator_slice)
File “C:\Python27\lib\site-packages\sklearn\model_selection\_validation.py”, line 140, in
for train, test in cv_iter)
File “C:\Python27\lib\site-packages\sklearn\base.py”, line 67, in clone
new_object_params = estimator.get_params(deep=False)
TypeError: get_params() got an unexpected keyword argument ‘deep’
Some one else also got this same error and posted a question on StackOverflow.
Any help is appreciated.
Sorry to hear that.
What versions of sklearn, Keras and tensorflow or theano are you using?
I have the same problem after an update to Keras 1.2.1. In my case: theano is 0.8.2 and sklearn is 0.18.1.
I could be wrong, but this could be a problem with the latest version of Keras…
Ok, I think I have managed to solve the issues. I think the problem are crashess between different version of the packages. What it solves everything is to create an evironment. I have posted in stack overflow a solution, @Partha, here: http://stackoverflow.com/questions/41796618/python-keras-cross-val-score-error/41832675#41832675
My versions are 0.8.2 for theano and 0.18.1 for sklearn and 1.2.1 for keras.
I did a new anaconda installation on another machine and it worked there.
Thanks,
Thanks David, I’ll take a look at the post.
Hi David, I have reproduced the fault and understand the cause.
The error is caused by a bug in Keras 1.2.1 and I have two candidate fixes for the issue.
I have written up the problem and fixes here:
http://stackoverflow.com/a/41841066/78453
Thanks, I will investigate and attempt to reproduce.
Hi,
yes, Jason’s solution is the correct one. My solution works because in the environment the Keras version installed is 1.1.1, not the one with the bug (1.2.1).
Great tutorial, many thanks!
Just wondering how do you train on a standardaised dataset (as per section 3), but produce actual (i.e. NOT standardised) predictions with scikit-learn Pipeline?
Great question Andy,
The standardization occurs within the pipeline which can invert the transforms as needed. This is one of the benefits of using the sklearn Pipeline.
Great tutorial, many thanks!
How do I recover actual predictions (NOT standardized ones) having fit the pipeline in section 3 with pipeline.fit(X,Y)? I believe pipeline.predict(testX) yields a standardised predictedY?
I see there is an inverse_transform method for Pipeline, however appears to be for only reverting a transformed X.
Thanks for you post..
I am currently having some problems with an regression problem, as such you represent here.
you seem to both normal both input and output, but what do you do if if the output should be used by a different component?… unnormalize it? and if so, wouldn’t the error scale up as well?
I am currently working on mapping framed audio to MFCC features.
I tried a lot of different network structures.. cnn, multiple layers..
I just recently tried adding a linear layer at the end… and wauw.. what an effect.. it keeps declining.. how come?.. do you have any idea?
Hi James, yes the output must be denormalized (invert any data prep process) before use.
If the data prep processes are separate, you can keep track of the Python object (or coefficients) and invert the process ad hoc on predictions.
Is there any way to use pipeline but still be able to graph MSE over epochs for kerasregressor?
Not that I have seen Sarick. If you figure a way, let me know.
Can you tell me how to do regression with convolutional neural network?
Great question Aritra.
You can use the standard CNN structure and modify the example to use a linear output function and a suitable regression loss function.
Hello Jason,
I assume if you use CNN it is necessary to reshape the output or not?
A CNN would not be appropriate if your data is tabular, e.g. a table like excel.
If it is sequence data, like a time series, then this tutorial will show you how:
https://machinelearningmastery.com/how-to-develop-convolutional-neural-network-models-for-time-series-forecasting/
Hi Jason,
Could you tell me how to decide batch_size? Is there a rule of thumb for this?
Great question kono.
Generally, I treat it like a parameter to be optimized for the problem, like learning rate.
These posts might help:
How large should the batch size be for stochastic gradient descent?
http://stats.stackexchange.com/questions/140811/how-large-should-the-batch-size-be-for-stochastic-gradient-descent
What is batch size in neural network?
http://stats.stackexchange.com/questions/153531/what-is-batch-size-in-neural-network
Hi Jason,
I see some people use fit_generator to train a MLP. Could you tell me when to use fit_generator() and when to use fit()?
Hi kono, fit_generator() is used when working with a Data Generator, such as is the case with image augmentation:
https://machinelearningmastery.com/image-augmentation-deep-learning-keras/
Hi Jason,
Thank you for the post. I used two of your post this and one on GridSearchCV to get a keras regression workflow with Pipeline.
My question is how to get weight matrices and bias vectors of keras regressor in a fit, that is on the pipeline.
(My posts keep getting rejected/disappear, am I breaking some protocol/rule of the site?)
Comments are moderated, that is why you do not seem the immediately.
To access the weights, I would recommend training a standalone Keras model rather than using the KerasClassifier and sklearn Pipeline.
Hi,
Thank you for the excelent example! as a beginner, it was the best to start with.
But I have some questions:
In the wider topology, what does it mean to have more neurons?
e.g., in my input layer I “receive” 150 dimensions/features (input_dim) and output 250 dimensions (output_dim). What is in those 100 “extra” neurons (that are propagated to the next hidden layers) ?
Best,
Pedro
Hi Pedro,
A neuron is a single learning unit. A layer is comprised of neurons.
The size of the input layer must match the number of input variables. The size of the output layer must match the number of output variables or output classes in the case of classification.
The number of hidden layers can vary and the number of neurons per hidden layer can vary. This is the art of configuring a neural net for a given problem.
Does that help?
Hi,
In your wider example, the input layer does not match/output the number of input variables/features:
model.add(Dense(20, input_dim=13, init=’normal’, activation=’relu’))
so my question is: apart from the 13 input features, what’s in the 7 neurons, output by this (input) layer?
Hi Pedro, I’m not sure I understand, sorry.
The example takes as input 13 features. The input layer (input_dim) expects 13 input values. The first hidden layer combines these weighted inputs 20 times or 20 different ways (20 neurons in the layer) and each neuron outputs one value. These are combined into one neuron (poor guy!) which outputs a prediction.
Hi,
Yes, now I understand (I was not confident that the input layer was also an hidden layer). Thank you again
The input layer is separate from the first hidden layer. The Keras API makes this confusing because both are specified on the same line.
Hi Jason,
You’ve said that an activation function is not necessary as we want a numerical value as an output of our network. I’ve been looking at recurrent network and in particular this guide: https://deeplearning4j.org/lstm . It recommended using an identity activation function at the output. I was wondering is there any difference between your approach: using Dense(1) as the output layer, and adding an identity activation function at the output of the network: Activation(‘linear’) ? are there any situations when I should use the identity activation layer? Could you elaborate on this?
In case of this tutorial the network would look like this with the identity function:
model = Sequential()
model.add(Dense(13, input_dim=13, init=’normal’, activation=’relu’))
model.add(Dense(6, init=’normal’, activation=’relu’))
model.add(Dense(1, init=’normal’))
model.add(Activation(‘linear’))
Regards,
Bartosz
Indeed, the example uses a linear activation function by default.
Hi Jason,
my current understanding is that we want to fit + transform the scaling only on our training set and transform without fit on the testset. In case we use the pipeline in the cv like you did. Do we ensure that for each cv the scaling fit only takes place for the 9 training sets and the transform without the fit on the test set?
Thanks very much
Top question.
The Pipeline does this for us. It is fit then applied to the training set each CV fold, then the fit transforms are applied to the test set to evaluate the model on the fold. It’s a great automatic pattern built into sklearn.
Hi! I ran your code with your data and we got a different MSE. Should I be concerned? Thanks for help!
Generally no, machine learning algorithms are stochastic.
More details here:
https://machinelearningmastery.com/randomness-in-machine-learning/
Hi Jason
while running this above code i found the error as
Y = dataset[:,25]
IndexError: index 25 is out of bounds for axis 1 with size 1
i had declared X and Y as
X = dataset[:,0:25]
Y = dataset[:,25]
help me for solving this
Hi Jason, Thanks for your great article !
I am working with same problem [No of samples: 460000 , No of Features:8 ] but my target column output has too big values like in between 20000 to 90000 !
I tried different NN architecture [ larger to small ] with different batch size and epoch but still not getting good accuracy !
should i have to normalize my target column ? Please help me for solving this !
Thanks for your time !
Yes, you must rescale your input and output data.
Hi Jason, Thanks for your reply !
Yes i tried different ways to rescale my data using
https://machinelearningmastery.com/prepare-data-machine-learning-python-scikit-learn/
url but i still i only got 20% accuracy !
I tried different NN topology with different batch size and epoch but not getting good results !
My code :
inputFilePath = “path-to-input-file”
dataframe = pandas.read_csv(inputFilePath, sep=”\t”, header=None)
dataset = dataframe._values
# split into input (X) and output (Y) variables
X = dataset[:,0:8]
Y = dataset[:,8]
scaler = StandardScaler().fit(X)
X = scaler.fit_transform(X)
maxnumber = max(Y) #Max number i got is : 79882.0
Y=Y / maxnumber
# create model
model = Sequential()
model.add(Dense(100, input_dim=8, init=’normal’, activation=’relu’))
model.add(Dense(100, init=’normal’, activation=’relu’))
model.add(Dense(80, init=’normal’, activation=’relu’))
model.add(Dense(40, init=’normal’, activation=’relu’))
model.add(Dense(20, init=’normal’, activation=’relu’))
model.add(Dense(8, init=’normal’, activation=’relu’))
model.add(Dense(6, init=’normal’, activation=’relu’))
model.add(Dense(6, init=’normal’, activation=’relu’))
model.add(Dense(1, init=’normal’,activation=’relu’))
model.compile(loss=’mean_absolute_error’, optimizer=’adam’, metrics=[‘accuracy’])
# checkpoint
model.fit(X, Y,nb_epoch=100, batch_size=400)
# 4. evaluate the network
loss, accuracy = model.evaluate(X, Y)
print(“\nLoss: %.2f, Accuracy: %.2f%%” % (loss, accuracy*100))
I tried MSE and MAE in loss with adam and rmsprop optimizer but still not getting accuracy !
Please help me ! Thanks
100 epochs will not be enough for such a deep network. It might need millions.
Hello Jason, Thanks for your reply !
How can i ensure that i will get output after millions of epoch because after 10000 epoch accuracy is still 0.2378 !
How can i dynamically decide the number of layers and Neurons size in my neural network ? Is there any way ?
I already used neural network checkpoint mechanism to ensure its accuracy on validation spilt !
My code looks like
model.compile(loss=’mean_absolute_error’, optimizer=’adam’, metrics=[‘accuracy’])
checkpoint = ModelCheckpoint(save_file_path, monitor=’val_acc’, verbose=1, save_best_only=True, mode=’max’)
callbacks_list = [checkpoint]
model.fit(X_Feature_Vector, Y_Output_Vector,validation_split=0.33, nb_epoch=1000000, batch_size=1300, callbacks=callbacks_list, verbose=0)
Let me know if i miss something !
Looks good.
There are neural net growing and pruning algorithms but I do not have tutorials sorry.
See the book: Neural Smithing http://amzn.to/2oOfXOz
Hi Jason,
Thanks for this great tutorial.
I do believe that there is a small mistake, when giving as parameters the number of epochs, the documentations shows that it should be given as:
estimator = KerasRegressor(build_fn=baseline_model, epochs=100, batch_size=5, verbose=0).
When giving:
estimator = KerasRegressor(build_fn=baseline_model, nb_epoch=100, batch_size=5, verbose=0)
the function doesn’t recognise the argument and just ignore it.
Can you confirm?
I’m using your ‘How to Grid Search Hyperparameters for Deep Learning Models in Python With Keras’ tutorial and have trouble tuning the number of epochs. If I checked one of the results of the GridSearchCv with a simple cross validation with the same number of folds I don’t obtain the same results at all. There might be a similar mistake there?
Thank your for your time!
You can pass through any parameters you wish:
https://keras.io/scikit-learn-api/
You will get different results on each run because neural network behavior is stochastic. this post will help:
https://machinelearningmastery.com/randomness-in-machine-learning/
https://keras.io/scikit-learn-api/ precises that number of epochs should be given as epochs=n and not nb_epoch=n. When giving the latter, the function will ignore the argument. As an example:
np.random.seed(seed)
estimators = []
estimators.append((‘standardize’, StandardScaler()))
estimators.append((‘mlp’, KerasRegressor(build_fn=baseline_model, nb_epoch=’hi’, batch_size=50, verbose=0)))
pipeline = Pipeline(estimators)
kfold = KFold(n_splits=10, random_state=seed)
results = cross_val_score(pipeline, X1, Y, cv=kfold)
print(“Standardized: %.5f (%.2f) MSE” % (results.mean(), results.std()))
will not raise any error.
Am I missing something?
The results I get are strongly different and I don’t think that this can be due to the stochasticity of the NN behaviour.
Thanks Charlotte, that looks like a recent change for Keras 2.0. I will update the examples soon.
Thank you!
Hey Jason,
I tried the first part and got a different result for the baseline.
I figured that the
estimator = KerasRegressor(build_fn=baseline_model, nb_epoch=100, batch_size=5, verbose=0)
is not working as expected for me as it takes the default epoch of 10. When I change it to epochs=100 it works.
I just read the above comment, it seems like they changed that in the API
Neural networks are a stochastic algorithm that gives different results each time they are run (unless you fix the seed and make everything else the same).
See this post:
https://machinelearningmastery.com/randomness-in-machine-learning/
Hi Jason,
how can i get regression coefficients?
Use an optimization algorithm to “find them”.
Stochastic gradient descent with linear regression may be a place to start:
https://machinelearningmastery.com/simple-linear-regression-tutorial-for-machine-learning/
Hi Jason,
How do i find the regression coefficients if it’s not a linear regression.Also how do i derive a relationship between the input attributes and the output which need not necessarily be a linear one?
You only have regression coefficients for linear algorithm like linear regression.
Dear Jason,
Thanks for your tutorials!!
I made it work in a particle physics example I’m working on, and I have 2 questions.
1) Imagine my target is T=a/b (T=true_value/reco_value). If I give to the regression both “a” and “b” as features, then it should be able to find exactly the correct solution every time, right? Or there is some procedure that try to avoid overtraining, and do not allow to give a results precise at 100%? I ask because I tried, and I got “good” performances, not optimal as I would expect (if it has “a” and “b” it should be able to find the correct T in the test too at 100% ). If I remove b from the regression, and I add other features, then y_hat/y_test is peaking at 0.75, meaning the the regression is biassed. Could you help me understanding these two facts?
2) I want to save the regression in order to use it later. After the training I do: a) estimator.model.save_weights and b) open(‘models/’+model_name, ‘w’).write(estimator.model.to_json()).
Estimator is “estimator = KerasRegressor(build_fn=baseline_model, nb_epoch=100, batch_size=50, verbose=1)”. How can I later use those 2 files to directly make predictions?
Thanks a lot,
Luca
Sorry, I’m not sure I follow your first question, perhaps you can restate it briefly?
See this post on saving and loading keras models:
https://machinelearningmastery.com/save-load-keras-deep-learning-models/
Hi Jason,
my point is the following. The regression is trained on a set of features (a set of floats), and it provides a single output (a float), the target. During the training the regression learn how to guess the target as a function of the features.
Of course the target should not be function of the features, otherwise the problem is trivial, but I tried to test this scenario as an initial check. What I did (as a test) is to define a target that is division of 2 features, i.e. I’m giving to the regression “a” and “b”, and I’m saying that the target to find is a/b. In that simple case, the regression should be smart enough to understand during the training that my target is simply a/b. So in the test it should be able to find the correct value with 100% precision, i.e. dividing the 2 features. What I found is that in the test the regression find a value (y_hat) that is close to a/b, but not exactly a/b. So I was wondering why the regression is behaving like that.
Thanks,
Luca
This is a great question.
At best machine learning can approximate a function, some approximations are better than others.
That is the best that I can answer it.
Hi Jason,
thanks for your posts, I really enjoy them. I have a quick question: If I want to use sklearn’s GridSearchCV and :
model.compile(loss=’mean_squared_error’
in my model, will the highest score correspond to the combination with the *highest* mse?
If that’s the case I assume there is a way to invert the scoring in GridSearchCV?
When using MSE you will want to find the config that results in the lowest error, e.g. lowest mean squared error.
Dear Jason
I have datafile with 7 variables, 6 inputs and 1 output
#from sklearn.cross_validation import train_test_split
#rain, test = train_test_split(data2, train_size = 0.8)
#train_y= train[‘Average RT’]
#train_x= train[train.columns.difference([‘Average RT’])]
##test_y= test[‘Average RT’]
#est_x= test[test.columns.difference([‘Average RT’])]
x=data2[data2.columns.difference([‘Average RT’])]
y=data2[‘Average RT’]
print x.shape
print y.shape
(1035, 6)
(1035L,)
# define base model
def baseline_model():
# create model
model = Sequential()
model.add(Dense(7, input_dim=7, kernel_initializer=’normal’, activation=’relu’))
model.add(Dense(1, kernel_initializer=’normal’))
# Compile model
model.compile(loss=’mean_squared_error’, optimizer=’adam’)
return model
# fix random seed for reproducibility
#seed = 7
#numpy.random.seed(seed)
# evaluate model with standardized dataset
estimator = KerasRegressor(build_fn=baseline_model, nb_epoch=100, batch_size=5, verbose=0)
kfold = KFold(n_splits=5, random_state=seed)
results = cross_val_score(estimator, x,y, cv=kfold)
print(“Results: %.2f (%.2f) MSE” % (results.mean(), results.std()))
but getting error below
ValueError: Error when checking input: expected dense_15_input to have shape (None, 7) but got array with shape (828, 6)
Also i tried changing
model.add(Dense(7, input_dim=7, kernel_initializer=’normal’, activation=’relu’))
to
model.add(Dense(6, input_dim=6, kernel_initializer=’normal’, activation=’relu’))
because total i have 7 variables out of which 6 are input, 7th Average RT is output
could u help pls
could you help pls
there is non linear relationship also bw o/p and i/p, as ai am trying keras neural to develop relationship that is non linear by itself
If you have 6 inputs and 1 output, you will have 7 rows.
You can separate your data as:
Then, you can configure the input layer of your neural net to expect 6 inputs by setting the “input_dim” to 6.
Does that help?
Dear Jason
and if I have 2 output, can I write
y = data[:, 0:6]
y = data[:, 6:7]
?
Not quite.
You can retrieve the 2 columns from your matrix and assign them to y so that y is now 2 columns and n rows.
Perhaps get more comfortable with numpy array slicing first?
sir plz give me code of “to calculayte cost estimation usin back prpoation technique uses simodial activation function”
See this post:
https://machinelearningmastery.com/implement-backpropagation-algorithm-scratch-python/
Hi Jason,
I’m new in deep learning and thanks for this impressive tutorial. However, I have an important question about deep learning methods:
How can we interpret these features just like lasso or other feature selection methods?
In my project, I have about 20000 features and I want to selected or ranking these features using deep learning methods. How can we do this?
Thank you!
Great question.
I would recommend performing feature selection as a pre-processing step.
Here’s more information on feature selection:
https://machinelearningmastery.com/an-introduction-to-feature-selection/
Hi,
Thks a lot for this post.
is there a way to implement a Tweedie regression in thsi framework ?
A
Sorry, I have not heard of “tweedie regression”.
Hi,
Thank you for the sharing.
I met a problem, and do not know how to deal with it.
When it goes to “results = cross_val_score(estimator, X, Y, cv=kfold)”, I got warnings shown as below:
C:\Program Files\Anaconda3\lib\site-packages\ipykernel\__main__.py:11: UserWarning: Update your
Dense
call to the Keras 2 API:Dense(13, input_dim=13, kernel_initializer="normal", activation="relu")
C:\Program Files\Anaconda3\lib\site-packages\ipykernel\__main__.py:12: UserWarning: Update your
Dense
call to the Keras 2 API:Dense(1, kernel_initializer="normal")
C:\Program Files\Anaconda3\lib\site-packages\keras\backend\tensorflow_backend.py:2289: UserWarning: Expected no kwargs, you passed 1
kwargs passed to function are ignored with Tensorflow backend
warnings.warn(‘\n’.join(msg))
I’ve tried to update Anaconda and its all packages,but cannot fix it.
Hi Jason,
I have a classic question about neural network for regression but I haven’t found any crystal answer. I have seen the very good performances of neural network on classification for image and so on but still doubt about its performances on regression. In fact, I have tested with 2 cases of data linear and non linear, 2 input and 1 output with random bias but the performances were not good in comparison with other classic machine learning methods such as SVM or Gradient Boosting… So for regression, which kind of data we should apply neural network? Whether the data is more complexity, its performance will be better?
Thank you for your answer in advance. Hope you have a good day 🙂
Deep learning will work well for regression but requires larger/harder problems with lots more data.
Small problems will be better suited to classical linear or even non-linear methods.
Thank you Jason,
Return in your examples, I have one question about the appropriate number of neurons should be in each hidden layer and the number of hidden layers in a network. I have read some recommendations such that number of hidden layer neurons are 2/3 of size of input layer and the number of neurons it should (a) be between the input and output layer size, (b) set to something near (inputs+outputs) * 2/3, or (c) never larger than twice the size of the input layer to prevent the overfitting. I doubt about these constraints because I haven’t found any mathematical proofs about them.
With your example, I increase the number of layers to 7 and with each layer, I use a large number of neurons (approximately 300-200) and it gave MSQ to 0.1394 through 5000 epochs. So do you have any conditions about these number when you build a network?
No, generally neural network configuration is trial and error with a robust test harness.
Hi jason.Can i apply regression for Autoencoders?
Yes, but I do not have examples sorry.
Hi Jason
Thank you for the great tutorial code! I have some questions regarding regularization and kenel initializer.
I’d like to add L1/L2 regularization when updating the weights. Where should I put the commands?
I also have a question abut assigning ” kernel_initializer=’normal’,” Is it necessary to initialize normal kernel?
Thanks!
KK
Here is an example of weight regularization:
https://machinelearningmastery.com/use-weight-regularization-lstm-networks-time-series-forecasting/
I would recommend evaluating different weight initialization schemes on your problem.
Thanks Jason.
I have one more question. I will use convolution2D with dropout. Do I still need to use L1/L2 regularization if I have dropout in my model?
Thanks!
KK
Try with and without and compare performance.
Dear Dr.,
I need you favor on how to use pre trained Keras based sequential model for NER with input text.
Example if “word1 word2 word3.” is a sentence with three words, how I can convert it to numpy array expected by Keras to predict each words NE tag set from the loaded pretrained Keras model.
With regards,
Convert the words to integers first.
I am getting a rate of more than 58 every time.
Here’s the exact code being used:
#Dependencies
from numpy.random import seed
seed(1)
import pandas
from keras.models import Sequential
from keras.layers import Dense
from keras.wrappers.scikit_learn import KerasRegressor
from sklearn.model_selection import cross_val_score
from sklearn.model_selection import KFold
from sklearn.preprocessing import StandardScaler
from sklearn.pipeline import Pipeline
# load dataset
dataframe = pandas.read_csv(“housing.csv”, delim_whitespace=True, header=None)
dataset = dataframe.values
# split into input (X) and output (Y) variables
X = dataset[:,0:13]
Y = dataset[:,13]
# Basic NN model using Keras
def baseline_model():
# create model
model = Sequential()
model.add(Dense(13, input_dim=13, kernel_initializer=’normal’, activation=’relu’))
model.add(Dense(1, kernel_initializer=’normal’))
# Compile model
model.compile(loss=’mean_squared_error’, optimizer=’adam’)
return model
#seed = 1
# evaluate model with standardized dataset
estimator = KerasRegressor(build_fn=baseline_model, nb_epoch=100, batch_size=5, verbose=0)
#kfold = KFold(n_splits=10, random_state=seed)
results = cross_val_score(estimator, X, Y, cv=10)
print(“Results: %.2f (%.2f) MSE” % (results.mean(), results.std()))
What do you mean by “a rate of more than 58”?
Thank you very much,
Cant we use CNN instead of Dense layers? in case we want to use CNN, should we use conv2d or simply conv?
In regression problems using deep architectures, can we use AlexNet, VGGNet, and the likes just like how we use them with images?
I would appreciate if you could have an example in this regard as well
Best Regards
I would not recommend a CNN for regression. I would recommend a MLP.
The shape of your input data (1d, 2d, …) will define the type of CNN to use.
Why you recommend MLP instead of CNN?
CNN is for sequence data or image data.
Great tutorial! I liked to save the weight that I adjusted in training, how can I do it?
This tutorial will show you how to save network weights:
https://machinelearningmastery.com/save-load-keras-deep-learning-models/
Thank you very much.
I have a question.
Is this tutorial suitable for wind speed prediction?
Try it and see.
Hi, Thank you for the tutorial. Few questions here.
1. What is the differences when we use
KerasRegressor(build_fn=baseline_model, nb_epoch=100, batch_size=5, verbose=0)
and with
model.fit(x_train, y_train, batch_size=batch_size,epochs=epochs,verbose=1,validation_data=(x_test, y_test))? AFAIK, with when using KerasRegressor, we can do CV while can’t on model.fit. Am I right? Will both result in the same MSE etc?
2. How do create a neural network that predict two continuous output using Keras? Here, we only predict one output, how about two or more output? How do we implement that? (Multioutput regression problem?)
Correct, using the sklearn wrapper lets us use tools like CV on small models.
You can have two outputs by changing the number of nodes in the output layer to 2.
Thanks for the reply.
Does that mean that with sklearn wrapper model and with model.fit(without sklearn) model are able to get the same mse if both are given same train, valid, and test dataset (assume sklearn wrapper only run 1st fold)? Or there are some differences behind the model?
I read about the Keras Model class (functional API) ( https://keras.io/models/model/ ). Is the implementation of the Model class,
model = Model(inputs=a1, outputs=[output1, output2])
the same as adding 1 node more at the output layer? If no, what’s the differences?
Same keras model under the covers.
from keras.layers.core import Dense,Activation,Dropout
from json import load,dump
from sklearn.metrics import mean_squared_error
from sklearn.metrics import mean_absolute_error
from keras.models import Sequential
from keras2pmml import keras2pmml
from pyspark import SparkContext,SparkConf
from pyspark.mllib.linalg import Matrix, Vector
from elephas.utils.rdd_utils import to_simple_rdd,to_labeled_point
from elephas import optimizers as elephas_optimizers
from elephas.spark_model import SparkModel
from CommonFunctions import DataRead,PMMLGenaration,ModelSave,LoadModel,UpdateDictionary,ModelInfo
from keras import regularizers
from sklearn.metrics import r2_score
from keras.optimizers import SGD
#from keras.models import model_from_config
#from keras.utils.generic_utils import get_from_module
class SNNReg:
def train(self,sc,xml,data,hdfs_path):
## Variable initialization ##
hActivation = xml.HiddenActivation
#print hActivation
nodeList = map(int,(xml.NodeList.split(“,”)))
#print nodeList
Accuracy = xml.Accuracy
#print Accuracy
lossFn = xml.LossFunction
#print nodeList
optimi = xml.Optimizer
#print optimi
hCount = int(xml.HiddenNodeCount)
#print hCount
inputDim = int(xml.InputDimension)
opNodes = int(xml.OutputNodes)
print (‘opNodes’,opNodes)
nbEpoch = int(xml.NumEpoch)
batchSize = int(xml.BatchSize)
#settings default paramerters if not the provided the values for it
if hActivation==””:
hActivation=”relu”
if lossFn==””:
lossFn=”mean_squared_error”
if optimi==””:
optimi=”adam”
if Accuracy==””:
Accuracy=”Accuracy”
print “now going to read ”
#print(“lossFn”,lossFn)
X,Y = DataRead(self,xml.NeuralNetCategory,xml.NeuralNetType,data,xml.ColumnNames,xml.TargetColumnName,xml.InputDimension)
# Creating a sequential model for simple neural network
model=Sequential()
model.add(Dense(nodeList[0],input_dim = inputDim,init=’normal’,activation =hActivation ))
# Creating hidden model nodes based on the hidden layers count
if hCount > 1:
for x in range(1,hCount):
model.add(Dense(nodeList[x],init=’normal’,activation = hActivation))
model.add(Dense(opNodes,activation=’linear’))
# Compile model
print “model complilation stage”
model.compile(loss = lossFn, optimizer=optimi)
rdd =to_simple_rdd(sc,X,Y)
print rdd
#adam= elephas_optimizers.Adam()
adam = elephas_optimizers.Adam()
#adagrad = elephas_optimizers.Adagrad()
#adadelta = elephas_optimizers.Adadelta()
#print (“type of rdd”,type(rdd))
print “now going to create spark model using elphass”
# Creating Spark elephas model from the spark model
print(“no of workers”,int(sc._conf.get(‘spark.pangea.ae.workers’)))
sparkModel = SparkModel(sc,
model,
optimizer=adam,
frequency=’epoch’,
mode=’asynchronous’,
master_loss=lossFn,
num_workers=int(sc._conf.get(‘spark.pangea.ae.workers’)))
# Train Spark model
print “now it is going to run train fucntion”
sparkModel.train(rdd,nb_epoch=nbEpoch, batch_size=batchSize)
i am trying to implement regression in Neural networks usign elphas and keras in python in a distributed way,but while trianing the i am getting to much high loss values , what i have to do ,give me any suggestions for go further.
Sorry I cannot help with distributing a Keras model.
two small points:
1. please mention in the text that it is required to have TensorFlow installed
2. CSV, means comma separated file, but data in the file are not separated by commas. not a big deal though
Thanks for the suggestions.
Thank you for the nice tutorial! In the post, you used “relu”, but I was wondering how to customize the activation function?
You can use sigmoid or tanh if you prefer.
Hi Jason,
Thanks for the great tutorial!
What are the advantages of the deep learning library Keras (with let’s say TensorFlow as the backend) over the sklearn neuron network function MLPRegressor? In both cases, the procedure (input) is very similar, where you have to decide which architecture, activation functions, and solver you want to use.
Thanks,
Don
Speed of development and size of community.
Thanks for the quick reply!
Can you please elaborate a little bit more?
When you are writing speed of development, can you please give a few practical examples for when it matters or what exactly you mean? When you are writing size of community, do you mean that the Keras/TensorFlow community is larger than the sklearn one? If not, what do you mean?
In addition, can you please add a few words on the epochs and batch_size parameters? Why is epochs used and not some tolerance, which makes more sense to me? Does it make sense that sometimes when I increase the the epocks value, the score decreases?
Thanks a lot!
Don
Yes, I believe it is easier/faster to develop models with Keras than other tools currently available.
I believe the Keras community is active and this is important to having the library stay current and useful. Keras is complementary to sklearn, tensorflow and theano.
One epoch is one pass through all training samples. One epoch is comprised of one or more batches. One batch involves a pass through one or more samples before updating the network weights.
I’m a little confused. If you define x as:
X = dataset[:,0:13]
then the last column in X is the same as Y. Shouldn’t X be:
X = dataset[:,0:12]
and then
Y = dataset[:,13]
If you define X to include the outputs, why wouldn’t it just set all the weights for dataset[0:12] to zero then perfectly fit the data since it already knows the answer?
> X = [0,1,2,3,4]
> print(X[0:3])
[0, 1, 2]
End index is exclusive.
Yes. The more questions I get like this, the more I feel I need a post on basic numpy syntax.
What are methods to validate the regression model in keras?Please can you help in that
You can estimate the skill of a model on unseen data using a validation dataset when fitting the model.
See the validation_split and validation_data arguments to the fit() function:
https://keras.io/models/sequential/
how can we recognize the keras regresssion model and classification model with code.
By the choice of activation function on the output layer and the number of nodes.
Regression will use a linear activation, have one output and likely use a mse loss function.
Classification will use a softmax, tanh or sigmoid activation function, have one node per class (or one node for binary classification) and use a log loss function.
Thanks for your tutotials and I find it helpful. However, I have a question.
When I use checkpoint callbacks in estimator.fit, it save a best trained weights, as a hd5 file.
But I cant load this pre trained weights, caz estimator does not have the method of load_weights which is one in keras models. What can I do, thank you!!!
You must load the weights as a Keras model.
Learn more in this tutorial:
https://machinelearningmastery.com/check-point-deep-learning-models-keras/
Hello, Jason
Thanks for the amazing tutorial! I learned alot from your blogs.
I have a question about np.random.seed.
What does the ‘np.random.seed’ actually do?
You explained that it is for reproducibility above but I didn’t understand what it means..
Thank you and hope you have a great one!
Best,
Paul
Many machine learning algorithms are stochastic by design:
https://machinelearningmastery.com/randomness-in-machine-learning/
We can remove this randomness in tutorials (purely for demonstration purposes) by ensuring we have the same amount of randomness each time the code is run:
https://machinelearningmastery.com/reproducible-results-neural-networks-keras/
This is not recommended for evaluating models in practice:
https://machinelearningmastery.com/evaluate-skill-deep-learning-models/
Does that help Paul?
Could you suggest the hidden activation functions for regression Neural networks other than relu.
Yes, sigmoid and tanh were used for decades before relu came along.
I have given tanh to regression model usign keras,i am not getting good results,you said tanh also supported for regression,please give me any suggesstions.
No, use a “linear” activation on the output layer for regression problems.
yeah of course , for output layer i have given the linear activation function only,but i am talking about hidden activation function i have given relu,if i would give tanh i am not getting good results.
I’m sorry to hear that. I have a list of ideas to try in this post:
https://machinelearningmastery.com/improve-deep-learning-performance/
Hi Jason,
Now that Keras and Tensorflow are available in R (RStudio) do you have any plans on doing above tutorial in R? I’ve got you book where you process the Boston Housing dataset using cubist and would love to see/run a direct comparison to get a sense of what improvement is possible.
Perhaps in the future, thanks for the suggestion Wayne.
My problem is that everything is hidden in the Pipeline object. How do I pull out the components, such as the model predict method, then to pull out the predicted values to plot against the input values.
Don’t use the Pipeline and pass data between the objects manually.
I have train the keras model, i need the logic for model.predict() ,how we are predicting the the values on test data,i have logic for predict_classes,but i don’t have logic for predict ,Please can you tell me logic for model.predict.
def predict_proba(self, X):
a = X
for i in range(self._num_layers):
g = self._activations[i]
W = self._weights[i]
b = self._biases[i]
a = g(np.dot(a, W.T) + b)
print(len(a))
return a
def predict_classes(self, X):
probs = self.predict_proba(X)
print(np.argmax(probs,1))
return np.argmax(probs,1)
predict_classes for classification.
i need predict logic for regression.
You can use the predict() function:
Hi Jason,
Thanks for the blog. I am trying to use the example for my case where I try to build a model and evaluate it for audio data. I use only spectrum data. Original data are in .wav format.
However, I am getting an error
“TypeError: can’t pickle NotImplementedType objects”
in line results = cross_val_score(pipeline, X, Y, cv=kfold)
My data is very small, only 5 samples.
Do you have any idea for this error?
Best,
Mustafa
I would recommend talking to the people from which you got the pickled data.
Hello Mustafa,
how is your pipeline initialized/defined? I had the exactly same error message at a line where I used cross_val_score with the KerasRegressor estimator. When you use something like
estimator = KerasRegressor(build_fn=myModel, nb_epoch=100, batch_size=5, verbose=0)
with a Keras-Model “myModel” and NOT with a function called “myModel” to return the model after compiling it like in the tutorial at the beginning you should get the same Pickle error. You can reproduce it with the tutorial code via myModel=baseline_model().
I hope this is helpful…
Best regards,
Heringsalat
why we are caluculating error rather than accuracy in regression problem,why accuracy does not make sence regression ,Please can you explain it.
We are not using accuracy. We are calculating error, specifically mean squared error (MSE).
why we are calculating mse rather than accuracy sir?
Because it is a regression problem and accuracy is only for classification problems.
while i am calulating loss and mse i am getting same values for regression,is that loss and mse are same in regression or different,if it is different ,how it is different,please can you explain it.
Loss is the objective minimized by the network. If you use mse as the loss, then you will not need to track mse as a metric as well. They will be the same thing.
Hello!
I am trying to train a ppg signal to estimate the heart rate i.e BPM.
Do you think it is appropriate to follow this structure?
If not please kindly help me by suggesting better methods.
Thank You!
Perhaps. Also consider a time series formulation. Evaluate every framing you can think of.
Hi Jason! 🙂
Thank you for great post! 🙂 I have a question about StandardScaler and Normalization.
What is difference between them? Also, can I use Min Max scaler instead of StandardScaler?
Thanks in advance.
Best,
Paul
Normalization via the MinMaxScaler scales data between 0-1. Standardization via the StandardScaler subtracts the mean to give the distribution a mean of 0 and a standard deviation of 1.
Standardization is good for Gaussian distributions, normalization is good otherwise.
Ah ha! Thanks for replying me back! 🙂
I’ll try MinMaxScaler()
Best,
Paul
Good luck Paul.
Hi Jason,
Thanks for the tutorial it’s really interesting.
Could you explain a bit further why you used Standardization and not Normalization please ?
Do the features have a Gaussian distribution ? How do you know if the features have a Gaussian distribution ?
I am currently working on house prices prediction on the Ames Housing dataset : do you recommand that I use Standardization or Normalization ?
You’re welcome.
Yes, see this:
https://machinelearningmastery.com/standardscaler-and-minmaxscaler-transforms-in-python/
Features do not have to have a Gaussian distribution, it is a good idea to only use standardisation if the data is gaussian though.
Hi Jason,
Again, its very informative blog. I am implementing keras in R but I couldn´t find keras regressor to fit the model. Do you have workaround for this or could you please suggest what can be used as an alternative?
Sorry, I don’t know about Keras in R.
Hey Jason – Thanks for the post.
I’d love to hear about some other regression models Keras offers and your thoughts on their use-cases.
Thanks,
James
What do you mean James? Do you have an example?
Hi David,
Thanks of the tutorials. These have been very helpful both for the implementation side to getting an insight about the possibilities of machine learning in various fields.
I was trying to run the code in section 2 and came across the following error:
………………….
Traceback (most recent call last):
File “regression.py”, line 48, in
results = cross_val_score(estimator, X, Y, cv=kfold)
File “/home/mjennet/anaconda2/lib/python2.7/site-packages/sklearn/model_selection/_validation.py”, line 321, in cross_val_score
pre_dispatch=pre_dispatch)
File “/home/mjennet/anaconda2/lib/python2.7/site-packages/sklearn/model_selection/_validation.py”, line 195, in cross_validate
for train, test in cv.split(X, y, groups))
File “/home/mjennet/anaconda2/lib/python2.7/site-packages/sklearn/externals/joblib/parallel.py”, line 779, in __call__
while self.dispatch_one_batch(iterator):
File “/home/mjennet/anaconda2/lib/python2.7/site-packages/sklearn/externals/joblib/parallel.py”, line 625, in dispatch_one_batch
self._dispatch(tasks)
File “/home/mjennet/anaconda2/lib/python2.7/site-packages/sklearn/externals/joblib/parallel.py”, line 588, in _dispatch
job = self._backend.apply_async(batch, callback=cb)
File “/home/mjennet/anaconda2/lib/python2.7/site-packages/sklearn/externals/joblib/_parallel_backends.py”, line 111, in apply_async
result = ImmediateResult(func)
File “/home/mjennet/anaconda2/lib/python2.7/site-packages/sklearn/externals/joblib/_parallel_backends.py”, line 332, in __init__
self.results = batch()
File “/home/mjennet/anaconda2/lib/python2.7/site-packages/sklearn/externals/joblib/parallel.py”, line 131, in __call__
return [func(*args, **kwargs) for func, args, kwargs in self.items]
File “/home/mjennet/anaconda2/lib/python2.7/site-packages/sklearn/model_selection/_validation.py”, line 437, in _fit_and_score
estimator.fit(X_train, y_train, **fit_params)
File “/home/mjennet/anaconda2/lib/python2.7/site-packages/keras/wrappers/scikit_learn.py”, line 137, in fit
self.model = self.build_fn(**self.filter_sk_params(self.build_fn))
File “regression.py”, line 35, in baseline_model
model.add(Dense(13, input_dim=13, kernel_initializer=’normal’, activation=’relu’))
File “/home/mjennet/anaconda2/lib/python2.7/site-packages/keras/layers/core.py”, line 686, in __init__
super(Dense, self).__init__(**kwargs)
File “/home/mjennet/anaconda2/lib/python2.7/site-packages/keras/engine/topology.py”, line 307, in __init__
assert kwarg in allowed_kwargs, ‘Keyword argument not understood: ‘ + kwarg
AssertionError: Keyword argument not understood: kernel_initializer
……………….
I tried to get more insight about the problem and came across the problem described by Mr. Partha which seems to be similar as mine and hence checked the version of Keras. The version of keras that I am using is 1.1.1 with tensorflow(1.2.1) as backend. Can you help me with this?
My name is Jason.
It looks like you need to update to Keras 2.
Apologies Mr. Jason
I tried to upgrade Keras as well as other dependencies but again the same error pops up. I am currently working on Keras 2.1.1 with Numpy 1.13.3 and scipy 1.0.0
I am surprised as your error suggests an older version of Keras.
I have a good list of places to get help with Keras here that you could try:
https://machinelearningmastery.com/get-help-with-keras/
Hi Jason,
Thanks for the example. I get the same error too. Keras/Theano/sklearn: 2.1.2/0.90/0.19.1. Mohit, were you able to debug it?
Sorry to hear that, I normally think it would be a version issue, but you look up to date.
I don’t have any good ideas, let me know if you learn more?
Hello Jason,
I used r2 metric on above code and figured that wider model has better score than deeper model. does this mean wider model is better than deeper? is r2 score a good metric to rate a regression model in this case?
Generally, neural network models are stochastic, meaning that they can give different results each time they are run:
https://machinelearningmastery.com/randomness-in-machine-learning/
I generally recommend this process to effectively evaluate neural networks:
https://machinelearningmastery.com/evaluate-skill-deep-learning-models/
Hello Jason, I am using your code from section
”
import numpy
import pandas
…
”
to this section
”
kfold = KFold(n_splits=10, random_state=seed)
results = cross_val_score(estimator, X, Y, cv=kfold)
print(“Results: %.2f (%.2f) MSE” % (results.mean(), results.std()))
”
but mean and std values are always higher than your result
”
Results: 60.40 (41.96) MSE
”
where is the problem??
Machine learning algorithms are stochastic, it may simply be different results on different hardware/library versions.
See this post:
https://machinelearningmastery.com/randomness-in-machine-learning/
The result of cross_val_score
”
[ 10.79553125, 7.68724794, 11.24587975, 27.62757629,
10.6425943 , 8.12384602, 4.93369368, 91.03362441,
13.37441713, 21.56249909]
”
are “Mean square error” ?? or something else??
If they are MSE,
can I say this prediction model is very bad??
The score are MSE. You could take the sqrt to convert them to RMSE.
How good a score is, depends on the skill of a baseline model (e.g. they’re relative) on the problem and domain knowledge (e.g. their interpretation).
I am having issues with cross_val_score. Whenever I run the code, I get the error:
#TypeError: The added layer must be an instance of class Layer. Found:
Suggestions?
Thank you!
The full output:
Traceback (most recent call last):
File “Y:\Tutorials\Keras_Regression_Tutorial\Keras_Regression_Tutorial\module1.py”, line 39, in
results = cross_val_score(estimator, X, Y, cv=kfold)
File “C:\Users\Gabby\y35\lib\site-packages\sklearn\model_selection\_validation.py”, line 321, in cross_val_score
pre_dispatch=pre_dispatch)
File “C:\Users\Gabby\y35\lib\site-packages\sklearn\model_selection\_validation.py”, line 195, in cross_validate
for train, test in cv.split(X, y, groups))
File “C:\Users\Gabby\y35\lib\site-packages\sklearn\externals\joblib\parallel.py”, line 779, in __call__
while self.dispatch_one_batch(iterator):
File “C:\Users\Gabby\y35\lib\site-packages\sklearn\externals\joblib\parallel.py”, line 625, in dispatch_one_batch
self._dispatch(tasks)
File “C:\Users\Gabby\y35\lib\site-packages\sklearn\externals\joblib\parallel.py”, line 588, in _dispatch
job = self._backend.apply_async(batch, callback=cb)
File “C:\Users\Gabby\y35\lib\site-packages\sklearn\externals\joblib\_parallel_backends.py”, line 111, in apply_async
result = ImmediateResult(func)
File “C:\Users\Gabby\y35\lib\site-packages\sklearn\externals\joblib\_parallel_backends.py”, line 332, in __init__
self.results = batch()
File “C:\Users\Gabby\y35\lib\site-packages\sklearn\externals\joblib\parallel.py”, line 131, in __call__
return [func(*args, **kwargs) for func, args, kwargs in self.items]
File “C:\Users\Gabby\y35\lib\site-packages\sklearn\externals\joblib\parallel.py”, line 131, in
return [func(*args, **kwargs) for func, args, kwargs in self.items]
File “C:\Users\Gabby\y35\lib\site-packages\sklearn\model_selection\_validation.py”, line 437, in _fit_and_score
estimator.fit(X_train, y_train, **fit_params)
File “C:\Users\Gabby\y35\lib\site-packages\tensorflow\contrib\keras\python\keras\wrappers\scikit_learn.py”, line 157, in fit
self.model = self.build_fn(**self.filter_sk_params(self.build_fn))
File “Y:\Tutorials\Keras_Regression_Tutorial\Keras_Regression_Tutorial\module1.py”, line 25, in baseline_model
model.add(Dense(13, input_dim=13, kernel_initializer=’normal’, activation=’relu’))
File “C:\Users\Gabby\y35\lib\site-packages\tensorflow\contrib\keras\python\keras\models.py”, line 460, in add
‘Found: ‘ + str(layer))
TypeError: The added layer must be an instance of class Layer. Found:
Press any key to continue . . .
Sorry, I have not seen this error before.
Confirm that you Python libraries including Keras and sklearn are up to date.
Confirm that you copied all of the code from the example.
Hello! Thanks for really great tutorial. It’s help a lot!
But I have a question: Do you know how Can I use StandarsScaler in a pipeline, when I deal with CNN and 2D images? My X data has shape e.g. (39, 256, 256, 1).
It works perfectly without StandardScaler, but with StandardScaler I’ve got following error:
ValueError: Found array with dim 4. StandardScaler expected <= 2.
Do you know how can I convert my input data and where in order to work with CNN, 2D images and StandardScaler?
I would recommend using the built-in data scaling features for images built into Keras:
https://machinelearningmastery.com/image-augmentation-deep-learning-keras/
Thanks a lot, for quick response! It’s good to know that Keras has already ImageDataGenerator for augmenting images.
I have one more question, do you know how can I rescale back outputs from NN to original scale? I mean if ImageDataGenerator has something similar to StandardScaler.inverse_transform() from sci-kit learn?
I’m not sure, I don’t think so.
If the image is an input, why would you need to reverse the operation?
In my case output of my network is based on actual values of pixels. I think, that in my case I will simply omit standardization. But thank you for mentioning ImageDataGenerator, it will help me much in other cases 🙂
Hello,
Thank you very much for your post
I use the data you uploaded.
However, when I print the MSE, it noticed that : Found input variables with inconsistent numbers of sample [506, 1]. It is the final sample in the data.
please help me
Thank you very much
Sorry, I don’t follow, can you restate the issue please?
At the end of step 2, evaluate the baseline model, I could’t print because that error:
” Found input variables with inconsistent numbers of sample [506, 1]. It is the final sample in the data.”
Perhaps double check that you copied all of the code exactly?
My mistake. I splitted data into columns already in Excel by “Text to Columns” function.
Thank you so much 🙂
Thank you so much for these articles. Two questions:
1) You state “a mean squared error loss function is [used]….This will be the same metric that we will use to evaluate the performance of the model.” I see where ‘mean_squared_error’ is passed as the ‘loss’, but there no ‘metrics=[…]’ arg passed. Does Keras simply use the ‘loss’ function as the metric, if no metric is specified?
2) I recreated this experiment and added the arg “shuffle=True” to the KFold function. This appears to improve performance down to 13.52 (6.99) MSE (wider_model). Any thoughts on this potential optimization? It seemed almost “too good to be true”.
Thanks!
Yes, Keras will report the loss and the metrics during training, this post might help you understand what is going on:
https://machinelearningmastery.com/custom-metrics-deep-learning-keras-python/
Great work.
The result might not be real (e.g. not statistically significant), consider this methodology for evaluating deep learning model skill:
https://machinelearningmastery.com/evaluate-skill-deep-learning-models/
Hello
The NN model you created contains 1 output.
I have a problem with more than 1 output.
I want to apply this code by modifying it. Is it ok ?
Can you suggest some solutions or notice to solve the problem?
Thank you very much
Yes, you can change the number of outputs.
Hello Jason,
For multiple outputs, do I still compile the model using the “model.compile(loss=’mean_squared_error’, optimizer=’adam’)”? How does the code compute a mean squared error in case of multiple outputs?
Thanks
Yes.
You can choose to calculate error for each output time step or for all time steps together. I cover this a little here:
https://machinelearningmastery.com/multi-step-time-series-forecasting-long-short-term-memory-networks-python/
How about if the outputs at each time step have different units (or in case or a simple dense feedforward network there are multiple outputs at the end, with each output having different units of measurement?). In that case, with different units and possible different orders of magnitude ranges for the outputs, it might not be sensible to take a simple RMSE etc. What would you suggest then to combine such different outputs together into a single loss function?
I would recommend rescaling outputs to something sensible (e.g. 0-1) before fitting the model.
Hey Jason,
I’m getting an error when running this code. I have karas 2, and scikit learn .17 installed. I keep getting this error:
Connected to pydev debugger (build 172.3968.37)
Using TensorFlow backend.
Traceback (most recent call last):
File “/home/b/pycharm-community-2017.2.3/helpers/pydev/pydevd.py”, line 1599, in
globals = debugger.run(setup[‘file’], None, None, is_module)
File “/home/b/pycharm-community-2017.2.3/helpers/pydev/pydevd.py”, line 1026, in run
pydev_imports.execfile(file, globals, locals) # execute the script
File “/home/b/PycharmProjects/ANN1a/ANN2-Keras1a”, line 6, in
from sklearn.model_selection import cross_val_score
ImportError: No module named model_selection
Backend TkAgg is interactive backend. Turning interactive mode on.
Is it saying I have no module for Sklearn because I only have .17 instead of the current version which i think is .19? I’m having a lot of trouble updating my scikit-learn package.
You will need to update your sklearn to 0.18 or higher.
Hey Jason I need some help with this error message. I’m not sure whats going on with it.
‘ValueError: could not convert string to float: Close’
I think it may be talking about one of my columns in my dataset.csv file which is named ‘Close’.
Here is the code:
import numpy
import pandas
from keras.models import Sequential
from keras.layers import Dense
from keras.wrappers.scikit_learn import KerasRegressor
from sklearn.model_selection import cross_val_score
from sklearn.model_selection import KFold
from sklearn.preprocessing import StandardScaler
from sklearn.pipeline import Pipeline
# load dataset
dataframe = pandas.read_csv(“PTNprice.csv”, delim_whitespace=True, header=None, usecols=[1,2,3,4])
dataset = dataframe.values
# split into input (X) and output (Y) variables
X = dataset[:,0:4]
Y = dataset[:,1]
# define the model
def larger_model():
# create model
model = Sequential()
model.add(Dense(100, input_dim=4, kernel_initializer=’normal’, activation=’relu’))
model.add(Dense(50, kernel_initializer=’normal’, activation=’relu’))
model.add(Dense(1, kernel_initializer=’normal’))
# Compile model
model.compile(loss=’mean_squared_error’, optimizer=’adam’)
return model
# fix random seed for reproducibility
seed = 7
numpy.random.seed(seed)
# evaluate model with standardized dataset
numpy.random.seed(seed)
estimators = []
estimators.append((‘standardize’, StandardScaler()))
estimators.append((‘mlp’, KerasRegressor(build_fn=larger_model, epochs=50, batch_size=5, verbose=0)))
pipeline = Pipeline(estimators)
kfold = KFold(n_splits=10, random_state=seed)
results = cross_val_score(pipeline, X, Y, cv=kfold)
print(“Standardized: %.2f (%.2f) MSE” % (results.mean(), results.std()))
Are you using the code and the data from the tutorial?
Did you copy it all exactly, including indenting?
Jason,
No my code is modified to try and handle a new data text. I felt the data was very similar to the original dataset. I actually got it to work with no errors. I just changed header=none to header=1
code:
# load dataset
dataframe = pandas.read_csv(“PTNprice.csv”, delim_whitespace=True, header=1, usecols=[1,2,3,4])
dataset = dataframe.values
# split into input (X) and output (Y) variables
X = dataset[:,0:4]
Y = dataset[:,1]
It took longer then expected for the script to finish. I’m trying to get it to make a prediction as well, but my output is less the satisfactory. Here is what the out gave me. What does this mean do you think?
output:
Larger: 0.00 (0.00) MSE
[ 0.78021598 0.79241288 0.81000006 …, 3.64232779 3.59621549
3.79605269]
My data is just stock prices from a 10 year period example: 0.75674 0.9655 3.753 1.0293
columns set up like this.
You may need to tune the model for your specific problem, here are some ideas on how to get better skill:
https://machinelearningmastery.com/improve-deep-learning-performance/
Hi Jason,
thank you so much, these courses are great, and very helpful !
I have written the code, following yours, but with the only difference that I have not used Pipeline, and take care of the scaling separately
seed = 7
np.random.seed(seed)
X = (X – X.mean(axis=0))/X.std(axis=0)
estimator = KerasRegressor(build_fn=baseline_model, nb_epoch = 100, batch_size=5,verbose=0)
kfold = KFold(n_splits=10,random_state=seed)
results = cross_val_score(estimator, X, y, cv=kfold)
For some reason, I don’t understand, your method constantly produces better results. Any idea why it performs better ?
Thanks a lot
It might be a statistical fluke, try varying the random seed and repeat the experiment 10 to 30 times and take the average score for each model.
This post has more ideas on effective ways to evaluate stochastic algorithms:
https://machinelearningmastery.com/evaluate-skill-deep-learning-models/
Thank you very much. I will do that.
Jason,
Just wanted to stop by and say thanks again!
I’ve been tweaking my learning models for the past three days and this is what I got
Larger: 0.12 (0.36) MSE
That’s pretty good right? I’m using a different data set from you, but it is very similar in structure. I was having a underfitting problem with my model for a while and I was getting like 500%(500%) error. I realized that I needed to make it a bit more complex. So I tripled my features, made my layers deeper and wider at the same time, as well as up the amount of epochs to 50000 and batch size to 10000. I also changed the number of splits from 10 to 25.
Question: Will I be able to get a smaller error% or is “Larger: 0.12 (0.36) MSE” about the lowest I can expect?
thanks again Jason,
Brence
Nice work.
I’m not sure of the limits of this problem, push as much as you have time/interest. In practice “good” is relative to what you have achieved previously. This is a good lesson in applied ML!
Dear Sir.
I applied your code and used it to predict successfully.
As same as my last question. I want to add 1 more output: the age of house: has built in 5 years, 7 years, 10 years….. for instance. The price and age are independent.
This is not a classify problem as I know.
So, would you suggest a code or what should I do next to solve the problem, please ?
Thank you very much
Is it possible to do a recursive multi step forecast prediction with this regression model?
I’m not sure how this code would fit into this.
prediction(t+1) = model(obs(t-1), obs(t-2), …, obs(t-n))
prediction(t+2) = model(prediction(t+1), obs(t-1), …, obs(t-n))
Yes, perhaps this post could be used a template:
https://machinelearningmastery.com/multi-step-time-series-forecasting-long-short-term-memory-networks-python/
Hi Jason,
Thank you for your tutorial. I just tried running your sample code for step 2 but unfortunately obtained a negative MSE which obviously does not make sense.
Results: -57.82 (42.31) MSE
Any ideas?
The code is exactly the same with minor exception that I had to changed
model.compile(loss=’mean_square_error’,optimizer=’adam’)
to:
model.compile(loss=’mse’,optimizer=’adam’)
Thanks
Toby
Yes, sklearn inverts minimizing scores to make them maximizing for optimization. Just take the absolute value.
Great thanks. Yes I found this out after I posed the question.
Nice.
Hi Jason,
Thank you this amazing tutorial. I just wanted to know what are the ways such that we can predict the output of neural network for some specific values of X and compare the performance by plotting the predicted and actual value
Thanks
Mohit
It really depends on the application as to what and how to plot.
Hi Jason,
first: thanks for this and all your other amazing tutorials. Really helps a lot as a beginner to get actual useful advice.
However, I reached a point where I’m looking for further advice – hope you can help me out!
I understand the concept of regression and MSE – in my case, I try to predict two values based on various other parameters. It’s really not complicated and the correlation between the values is pretty clear, so I think this shouldn’t be a problem.
Now when having a value predicted, I don’t want to know the MSE but I’d rather know, if the prediction is within a certain range from the original value.
Example:
‘accepted’ range: y +/- 0,1
y = 1
y^ = 1,08
y – y^ = | 0,08| –> OK, because it’s within y +/- 0,1.
Is there a way to do this in Python or KERAS? I just started working with it, so any advice would be helpful. Thanks!
You can calculate a confidence interval for linear models.
I have an example for linear regression on time series here that might give you ideas:
https://machinelearningmastery.com/time-series-forecast-uncertainty-using-confidence-intervals-python/
Thanks! I will look into that.
Hi Jason,
Thank you for your tutorial.
I want to know
if a regression problem dataset a 10000 feature. the input_dim is so big …….but all the feature are meaningful(it’s an procedure data) and can’t be delete.
how to change this example to handle my problem,and what should i care,is there any trick?
Perhaps you can use a projection such as PCA? SVD? or others?
Hi Jason – Thank you for all these tutorials. These are awesome!!
Since the NN architecture is black box. Is there a way to access hidden layer data for debugging? When I run the regression code (from above) I get slightly different numbers. Thx again!
You will get different numbers every time you run the same algorithm on the same data Steve. This is a feature, not a bug:
https://machinelearningmastery.com/randomness-in-machine-learning/
You can access the layers on the model as an array: model.layers I think.
Hi Jason,
when calling model.predict() the predicted value has no sense in terms of house prices.
for instance line 15 of House pricing dataset
0.63796 0.00 8.140 0 0.5380 6.0960 84.50 4.4619 4 307.0 21.00 380.02 10.26 18.20
last value (18.20) is house price in 1000$
Xnew= array([[ 0.63796, 0.00, 8.140, 0, 0.5380, 6.0960, 84.50, 4.4619, 4, 307.0, 21.00, 380.02, 10.26]])
ynew=model.predict(Xnew)
ynew
Out[114]: array([[-0.09053693]], dtype=float32)
what does -0.09053693 mean?
Could you please amend your code with full code of predict function.
Perhaps the example you ran scaled the data prior to modeling, if so, you can invert the scaling transform on the prediction to return to original units.
Hey Jason,
Is it possible to get an prediction output for each column used in the dataset? Like for example the dataset was made up of
12 1 22 45
2 34 55 8 like this. Could I get it to give me four output numbers, one for each column in the dataset?
Yes, you can call model.predict()
but how do I predict for more then one column in the dataset? My dataset has 6 of them, and my output always has just 5 columns. Which leads me to believe that its just predicting for one column instead of all 6. Could I accomplish this by setting the output layer to have more then one neuron?
You could configure the model to predict a vector via the number of neurons in the output layer.
You could configure the model output one column at a time via an encoder-decoder model.
I have examples of each on the blog.
That is exactly what I was looking for. I found your examples on the blog. Thank you so much Jason.
Glad to hear it.
Hi Jason,
Thank you for your tutorial! I’m not a programmer or anything, in fact, I’ve never wriiten a line of code my entire life. But I find your tutorial very helpful.
Recently I came acoss a regression problem and I tried to solve it using deep learning. So I followed this article and step by step I got Keras up and running and got a result. The problem is I don’t know how to tune the neural network and optimize it. The result I got is far from satisfactory. Do I have to adjust the parameter of the model one by one and see how it goes or is there a quicker way to optimize the neural network?
Also I see there’s a mini-course here, and I tried so sign up for it but I didn’t get the email. Maybe because I’m from China or anything, I don’t know. Is there any crash course I can get? cause I know nothing about Python yet…
Well done!
This post will show you how to tune a network:
https://machinelearningmastery.com/improve-deep-learning-performance/
You can access the mini course here:
https://machinelearningmastery.com/applied-deep-learning-in-python-mini-course/
Thank you for your response. I have another quesion though. The lines involving the ‘estimator’ is for training the model, right? How can I save the model and use it for prediction?
See this post:
https://machinelearningmastery.com/save-load-keras-deep-learning-models/
Thank you so much! I’ve learnt a lot. In this example I can use pipeline.fit(X,Y) to train the model and use pipeline.predict(X) for prediction, is that right? I think the ‘pipeline’ in the tutorial involves the standardization process. So when I use pipeline.predict(X) I can just put in raw data and get the prediction and the prediction will be the inverse-standardization result. Am I understanding this right?
I believe so.
Hello, I need to ask for this line X = dataset[:,0:13], as I can see from the data set it contains 14 columns (0 to 13) and the last column is the labels column then this line should be X = dataset [:,0:12]. is it correct or I’m wrong?
No. You can learn more about slicing arrays here:
https://machinelearningmastery.com/index-slice-reshape-numpy-arrays-machine-learning-python/
Hi Jason,
I am trying to apply the code in this tutorial to forecast my time series data. But since the beginning when I am trying to split the data into X and Y, I am getting an error “TypeError: unhashable type: ‘slice'”. Unfortunately I cannot find the source of it.
Can you help me?
Thanks in advance!
runfile(‘D:/LOCAL_DROPBOX/MasterArbeit_Sammlung_V01/Python/MasterArbeit/ARIMA/Test/BaselineRegressionKNN.py’, wdir=’D:/LOCAL_DROPBOX/MasterArbeit_Sammlung_V01/Python/MasterArbeit/ARIMA/Test’)
[[‘3,6’ ‘20,3’ ‘0’ …, 173 1136 0]
[‘11,4’ ‘18,8’ ‘15,2’ …, 105 1676 0]
[‘8,9’ ‘15,3’ ‘1,4’ …, 372 733 0]
…,
[‘-2,3’ ‘4,5’ ‘0’ …, 0 0 0]
[‘0,2’ ‘7,9’ ‘0’ …, 0 0 0]
[‘-3,5’ ‘4,4’ ‘0’ …, 0 0 0]]
Traceback (most recent call last):
File “”, line 1, in
runfile(‘D:/LOCAL_DROPBOX/MasterArbeit_Sammlung_V01/Python/MasterArbeit/ARIMA/Test/BaselineRegressionKNN.py’, wdir=’D:/LOCAL_DROPBOX/MasterArbeit_Sammlung_V01/Python/MasterArbeit/ARIMA/Test’)
File “C:\Users\Tanya\Anaconda3\lib\site-packages\spyder\utils\site\sitecustomize.py”, line 710, in runfile
execfile(filename, namespace)
File “C:\Users\Tanya\Anaconda3\lib\site-packages\spyder\utils\site\sitecustomize.py”, line 101, in execfile
exec(compile(f.read(), filename, ‘exec’), namespace)
File “D:/LOCAL_DROPBOX/MasterArbeit_Sammlung_V01/Python/MasterArbeit/ARIMA/Test/BaselineRegressionKNN.py”, line 25, in
X = dataset[:,0:8]
File “C:\Users\Tanya\Anaconda3\lib\site-packages\pandas\core\frame.py”, line 2139, in __getitem__
return self._getitem_column(key)
File “C:\Users\Tanya\Anaconda3\lib\site-packages\pandas\core\frame.py”, line 2146, in _getitem_column
return self._get_item_cache(key)
File “C:\Users\Tanya\Anaconda3\lib\site-packages\pandas\core\generic.py”, line 1840, in _get_item_cache
res = cache.get(item)
TypeError: unhashable type: ‘slice’
You can learn more about array slicing here:
https://machinelearningmastery.com/index-slice-reshape-numpy-arrays-machine-learning-python/
Hello,
Regarding to “A further extension of this section would be to similarly apply a rescaling to the output variable such as normalizing it to the range of 0-1”.
I do not know how can I get that the StandarScaler object also apply the transformation to the ouput variable Y, instead of applying it only over X . I did the following
results = cross_val_score(pipeline, preprocessing.scale(X), preprocessing.scale(Y), cv=kfold)
However, in this way the preprocessing step is made prior to the kfold cross validation, and not in each fold execution as in your previous example.
Yes, the data preparation would have to happen prior to cross validation.
How we can draw the relation between the expected values and the prediction one
I would recommend using matplotlib.
One more question, How can I use k-fold cross validation with CNN model?
The same as with any model. What problem are you having exactly?
I’m using CNN for rgression problem and I split the data into train, validation, and test sets but I have overfitting problem. So, I’m thinking to use cross validation but I don”t know how can I do it.
I show how to evaluate deep learning models here:
https://machinelearningmastery.com/evaluate-skill-deep-learning-models/
Hi, Jason
When I search some tutorials on google, if your posts appears, I always check your blog first.
Thanks very much.
I hope they help.
Hi, Jason
What is the activation function of the output layer? You didn’t write it.
Thanks.
Linear, which is the default.
Hello Jason,
I used your code, but get different results:
Results: -114.64 (82.76) MSE
Standardized: -29.57 (27.85) MSE
Larger: -23.46 (27.29) MSE
Wider: -22.91 (29.25) MSE
Why are they negative?
Nice work. The negative results are caused by sklearn inverting the loss function. This is a relatively new thing.
This is confusing. Results are so different!
How to interpret this error in percentage? Is there a way?
I have studied the Ng’s courses on deeplearning_dot_ai, but he only introduced classification problems.
How to understand how good error is for the case of regression? Will there be any difference from you example for a vector-regression (output is a vector) problem?
Thank you.
Yes, compare the model skill to a baseline model like a Zero Rule algorithm.
Improves are relative, not absolute.
Hi Jason,
How do you get predicted y values for plotting when using a pipeline and k-fold cv? Also, suppose you had a separate X_test, how would you predict y_hat from it? So, I am envisioning a scenario where you have a training set and a separate test set (as in Kaggle competitions). You build your pipeline and k-fold cv on the training set and predict on the test set. But, your training set is scaled as a part of the pipeline. How could you apply the same scaling on X_test?
We don’t predict with CV, it is only a method for estimating model skill. Learn more here:
https://machinelearningmastery.com/train-final-machine-learning-model/
Hi Jason;
How do you design a Keras model that returns multiple outputs (lets say 4) instead of single output in regression problems?
You can output a vector with multiple units in the output layer.
Do you mean I should change the model design by editing last line before compiling from:
model.add(Dense(1, kernel_initializer=’normal’))
to:
model.add(Dense(4, kernel_initializer=’normal’))
?
Yes.
I obtained similar results like WonderingStranger. I’m new to deep learning. So I did not understand what I need to do with your response. I would appreciate if you explain in more detail.
Hello, how do i save the weights. I checked your link for saving, but you are not using the pipeline method on that one.
I tried kfold.save_weigths, but got an error
You might need to keep a reference to your model (somehow?) and use the Keras API to save the weights.
Hi Jason,
is there any way to input the standardized data into the lstm model (create_model). The reason is that due the input shape of lstm which only allow 3D..however, to do standardizing, it can only accept 2d shape. hope to get some comment from you.. thank you
Standardize prior to reshaping.
thanks jason for the response..I appreciate it
Hi Jason,
I am still new in this
thank you for your explanation step by step
I want to ask about the detail in housing.csv and how to predict the value
for example we want to predict the last attribute of the dataset
by using estimator.predict
Thank you
You can use:
yhat = model.predict(X)
Does that help?
Hi Jason,
Thanks for the great tutorial. your site makes me younger 🙂
Is there any way to print/export actual and predicted house prices.
In addition, woud you please suggest a visualization way for R2?
Thanks!
You can make predictions as follows:
yhat = model.predict(X)
X = dataset[:,0:11]
Y = dataset[:,11]
Traceback (most recent call last):
File “”, line 5, in
Y = dataset[:,11]
IndexError: index 11 is out of bounds for axis 1 with size 1
Please help to resolve this issue.
Thanks jason for this wonderful post.
Ensure you copy all of the code from the example.
Hi Jason!
Thanks for the great tutorial.
I did all the examples above and then I tried to fit baseline_model by using
“baseline_model.fit(X,Y, nb_epoch=50, batch_size=5)” this command, I got “AttributeError: ‘function’ object has no attribute ‘fit'” this error message. what’s the problem?
I googled exact same message above but I didn’t get anything about model.fit error.
You called a function on a function. The variable for the model is called “model”. Call functions on that.
Hi Jason, I’m having a problem, but I’m not sure why. This is a dataset with 7 columns (6 inputs and 1 output).
Code:
Error:
Thanks and any help would be appreciated!
It looks like there is a problem with the shape of your data not matching the expectations of the model.
Change the model or change the data.
Thanks, Jason, a good tutorial.
But I have a question that we only specify one loss function ‘mse’ in the compile function, that means we could only see MSE in the result. Is there any way to see the multiple accuracies at the same time in the result? Thanks
You can use the Keras API and specify metrics, learn more here:
https://machinelearningmastery.com/custom-metrics-deep-learning-keras-python/
Hi Jason,
Thanks for the tutorial! I have one question: if you use StandardScaler for the dataset, isn’t this affecting the units ($) of the cross validation score (MSE)? Thanks.
Yes, we must invert the transform on the predictions prior to estimating model skill to ensure units are in the same scale as the original data.
But it looks like you’re not doing it but still mentioning square thousand dollars as units, am I missing something?
correct, I do not covert back original units (dollars), so instead I mention “squared dollars” e.g. $^2.
Hey Jason, I have the following two questions:
How can we use the MAE instead of the MSE? and
How can we compute the Spearman’s rank correlation coefficients?
You can specify the loss or the metric as ‘mae’.
You can save the predictions can use scipy to calculate the spearmans correlation between your predictions and the expected outcomes.
I’m trying to save the predictions and expected outcomes of the model by using this
code:
for test in kfold.split(X, Y):
print (model.predict(X[test]))
print (Y[test])
Is it ok?
I would recommend training a final model and using that to make predictions, more about that here:
https://machinelearningmastery.com/train-final-machine-learning-model/
Hi,
Thanks for your practical, useful and understandable blog posts.
I used this post to evaluate my MLP model, but Can we use this method to evaluate LSTM as well?
Thanks
For sequence prediction, often different model evaluation methods are needed. Such as walk-forward validation:
https://machinelearningmastery.com/backtest-machine-learning-models-time-series-forecasting/
Thank you Jason for great posts,
I have difficulty in understanding the MSE and MAE meaning. I cannot understand how to interpret this number? For this specific example what is the range of ‘mse’ or ‘mae’?
Because I am working on a large dataset and I am getting mae like 400 to 800 and I cannot figure out what does it mean. Could you please help me?
Thanks
Good qusetion.
You can take the square root of the MSE to return the units back to the same units of the variable used to make the prediction.
The MAE is in the same units as the output variable.
The error values can be interpreted in the context of the distribution of the output variable.
You can determine if the skill of the model is good by comparing it to the error scores from a baseline method, such as predicting the average outcome from the training set for each prediction on the test set.
Does that help?
So, ‘mse’ and ‘mae’ is not percentage and it can be any number (even very big depending on output variable), right?
It means if we are predicting price of house and our output is like $1000, then mae equals to 100 means we have about a hundred dollar error in predicting the price. Did I get it correctly?
Thank you again
Correct.
I really appreciate it.
Hi Jason and thank you a lot for the post.
I have a question in addition to what Sarah asked: should I apply the square root also to “results.std()” to get a closer idea of the relationship between the error and the data?
In the article you achieved a MSE=20 with std~22, but if we calculate the square root for MSE I somehow understand that we should also do that with the standard deviation, right?
However, if that is the case, if we need to calculate the square root, wouldn’t the original value be the variance? In other words, is the “results.std()” in the next line actually the std or is it the variance?
Thanks
No. Take the square root on the raw MSE values, then calculate summary stats like mean and standard deviations.
Note that nb_epoch has been deprecated in KerasRegressor, should use epochs now in all cases. https://github.com/keras-team/keras/issues/6521
Thanks, fixed.
Hi Jason,
Great blog posts. Helped me a lot in my work. I have created a similar multi-layer model for learning and predicting from a shock physics dataset (11 input parameters and a time-series output).
I was wondering how I could be able to get the uncertainty information as well as the predicted output from the estimators? Do you have a blog or piece of keras code that can get me started?
thanks a lot.
Basically, my model looks like this:
# define baseline model
def baseline_model_full():
# create model
model = Sequential()
model.add(Dense(numOfParams, input_dim=numOfParams, kernel_initializer=’normal’, activation=’relu’))
model.add(Dense(900, kernel_initializer=’normal’))
# Compile model
model.compile(loss=’mean_squared_error’, optimizer=’adam’)
return model
I fit this with with training input and output data and then I provide it a new input for its prediction. I was wondering, if I can also get the uncertainty of the model for this prediction along with the predicted output.
Thanks
Good question. You could use predict_proba() to get a probabilistic output.
Does that help?
Hi Jason,
Thank you for the quick response! Yes, I was able to use predict_proba() to get probability values. I am noticing that, the probability values are rather small although the prediction quality is quite good.
I had 200 test inputs of shape (200,11) and the predicted output was of shape (200,900). The output probability shape was also (200,900) and the maximum value of this prediction probability was only 0.024. So, any suggestions on how to interpret these probability values?
Thanks again.
Perhaps the model is not confident.
After a closer look, I see that the predict() and predict_proba() are actually giving the same array as output; and it is the predictions, not the probabilities. Have you seen this?
thanks
I have not sorry, perhaps contact Keras support:
https://machinelearningmastery.com/get-help-with-keras/
I looked at this and seems like both the functions are just the same
https://github.com/keras-team/keras/blob/master/keras/models.py
I think this might be the reason why I am getting the same output. But, unlike some other comments over the internet that suggest that we should get the probability as the output for both the functions, I think I am getting the predictions in both the cases.
Do you have any suggestions on this?
Why are you using 50 epochs in some cases and 100 on others?
That seems like the best explanation of why you find ‘wider’ (with 200 epochs) is better than ‘larger’ (with 50 epochs).
And sure enough, I found ‘larger’ with 100 epochs beats ‘wider’ with 100 epochs:
Larger(100 epochs): 22.28 (26.54) MSE
Yes, I was demonstrating how to be systematic with model config, not the best model for this problem.
Jason,
I am trying to use CNN for signal processing.
Wonder if it is possible? Could you point me to any references?
Specific example:
I have an audio signal of some length, let us say 100 samples.
I would like to find a filter that produces a delta spike out of my signal.
In other words, training with my signal should output [1, 0, 0, …… 0, 0 ] – delta spike.
Thanks a lot,
Paul
Perhaps try a search on google scholar.
Perhaps take a look at LSTMs, I have seen them used more for working with signal data, e.g. audio data for speech recognition problems. for example:
https://machinelearningmastery.com/start-here/#lstm
I hope that helps as a soft pointer.
Does number epoch depends on number of data i have.
For example i have around 400,000+ data, what should be number of epochs
More data may require more learning/epochs.
Thank you jason ur blog is wonderful place to learn Machine Learning for beginners
Thanks, I’m glad to hear that.
Jason i came across while trying to learn about neural network about dead neurons while training how do i identify dead neurons while training using keras
and also how to eliminate that i am eager to know that
thanking you in advance
Thanks, that is a great topic. Sorry, I don’t have material on it. Perhaps I can cover it in the future.
Jason i really want to know the maths behind neural network can u share a place where i can learn that from i want to know how it makes the prediction in linear regression
Neural network and linear regression are two different methods.
Learn about the math for neural networks in this book:
https://amzn.to/2KuhGPP
Learn about the math for linear regression in this book:
https://amzn.to/2wM6Jr4
thank you jason that was really good resource
Hi Jason-
Thanks for the great input.
I am uing the following code to predicts Boston Homa prices:
# Artificial Neural Network
# Regression Example With Boston Dataset: Baseline
# Importing the libraries
import numpy
from pandas import read_csv
from keras.models import Sequential
from keras.layers import Dense
from keras.wrappers.scikit_learn import KerasRegressor
from sklearn.model_selection import cross_val_score
from sklearn.model_selection import KFold
from sklearn.preprocessing import StandardScaler
from sklearn.pipeline import Pipeline
import matplotlib.pyplot as plt
# Importing the dataset
dataframe = read_csv(“housing.csv”, delim_whitespace=True, header=None)
dataset = dataframe.values
# Split into input (X) and output (Y) variables
X = dataset[:,0:13]
y = dataset[:,13]
# Create model
model = Sequential()
model.add(Dense(13,input_dim=13, init=’normal’, activation=’relu’))
model.add(Dense(1, init=’normal’))
# Compile model
model.compile(loss=’mean_squared_error’, optimizer=’adam’, metrics=[‘accuracy’])
# Fit the model
history = model.fit(X, y, validation_split=0.20, epochs=150, batch_size=5, verbose=0)
# Make predictions
predictions = model.predict(X)
# list all data in history
print(history.history.keys())
# summarize history for accuracy
plt.plot(history.history[‘acc’])
plt.plot(history.history[‘val_acc’])
plt.title(‘model accuracy’)
plt.ylabel(‘accuracy’)
plt.xlabel(‘epoch’)
plt.legend([‘train’, ‘test’], loc=’upper left’)
plt.show()
However, as you can see from the graph, my accuracy is very low. Why is that?
You cannot measure accuracy for regression problems. Learn more here:
https://machinelearningmastery.com/faq/single-faq/how-do-i-calculate-accuracy-for-regression
So how can i visualise the predictions and the actual numbers in a plot?
You can make predictions by calling predict(), learn more here:
https://machinelearningmastery.com/how-to-make-classification-and-regression-predictions-for-deep-learning-models-in-keras/
You can create a plot using matplotlib plot() function.
Thanks for the reply. I fixed the problem in visualization. And now I am trying to scale the inputs.
However, when I tried to scale the dataset, it says: “Reshape your data either using array.reshape(-1, 1) if your data has a single feature or array.reshape(1, -1) if it contains a single sample.”
How do I fix it?
Here is the code I use:
from sklearn.preprocessing import StandardScaler
sc_X = StandardScaler()
X_train = sc_X.fit_transform(X_train)
X_test = sc_X.transform(X_test)
sc_y = StandardScaler()
y_train = sc_y.fit_transform(y_train)
y_test = sc_y.transform(y_test)
The error suggests you are providing a 1D array, and you must change it to a 2D array, perhaps one column with multiple rows (n, 1)
You can learn more about reshaping numpy arrays here:
https://machinelearningmastery.com/index-slice-reshape-numpy-arrays-machine-learning-python/
Hi Jason! Great job, thank you! I’m a new in ML. I have tried to repeat your test, but i’ve changed it a little. In addition, i’ve used not a CSV dataset, but integrated in Keras and splitted on train and test by Keras authors. And i didn’t use CV. So I have one question. I’ve got MSE=12 on test data and MSE=3 on train. Is it normal for such case or mistake? It is much less than MSE=21. Code below:
batch_size = 32
epochs = 1000
model_name = ‘model_proba.h5′
(x_train, y_train), (x_test, y_test) = boston_housing.load_data()
scaler = StandardScaler()
scaler.fit(x_train)
x_train = scaler.transform(x_train)
x_test = scaler.transform(x_test)
input_shape = x_train.shape
model = Sequential()
model.add(Dense(100, input_dim=input_shape[1], activation=’relu’))
model.add(Dropout(0.3))
model.add(Dense(20, activation=’relu’))
model.add(Dropout(0.1))
model.add(Dense(1))
model.compile(loss=’mean_squared_error’, optimizer=’adadelta’, metrics=[‘accuracy’])
earlystopper = EarlyStopping(patience=100, verbose=1)
checkpointer = ModelCheckpoint(model_name, verbose=1, save_best_only=True)
history = model.fit(x_train, y_train, batch_size=batch_size, epochs=epochs,verbose=1,
callbacks=[earlystopper, checkpointer])
scoret = model.evaluate(x_train, y_train, verbose=0)
score = model.evaluate(x_test, y_test, verbose=0)
print(‘Train loss:’, scoret[0])
print(‘Train accuracy:’, scoret[1])
print(‘Test loss:’, score[0])
print(‘Test accuracy:’, score[1])
It sounds like your model might be a little overfit on the training dataset.
Thanks for answer. I agree about training dataset. But I’ve got low MSE=12 (instead of typically MSE=21) on test dataset. What does it mean? Is this overfit model?
A low error is good. A low error on the test set is not overfitting. It might mean the model is good or that the result is a statistical fluke.
1. Hey, when you are doing results.mean() this would give you the mean of the the cross val scores for the K fold splits, would you not want the means to come higher as we finetune the models?
results.std() should reduce as we want variance to be low, but why is the mean reducing good?
2. When you apply the K fold using pipeline, does it standardize your each training split independently?
3. Rather than appending estimator and standard scaler, could we have directly entered them as a list or dictionary ?
Ideally we want a higher mean and smaller stdev, if possible.
Yes, any transformers within the pipeline are fit on the training folds and applied to test fold.
Yes, you can provide a list to the Pipeline.
hi Jason , I an new to keras and your blog is helping me a lot .
I am trying two piece code 1. using sklearn 2. using Keras .
The 1st model give me a very good prediction (diabetes_y_pred ).
The 2nd model give me a very bad prediction (diabetes_y_pred) .
Can you tell me why ? Only If I increase the epoch size in the model.fit in keras the values of diabetes_y_pred gets better.
sklearn : code
import matplotlib.pyplot as plt
import numpy as np
from sklearn import datasets, linear_model
from sklearn.metrics import mean_squared_error, r2_score
# Load the diabetes dataset
diabetes = datasets.load_diabetes()
# print (diabetes.keys())
# #print (diabetes.data)
# print (diabetes.DESCR)
# print (diabetes.feature_names)
#print (diabetes.target)
# Use only one feature
diabetes_X = diabetes.data[:, np.newaxis, 2]
# print (diabetes_X.shape)
# print (diabetes.data)
# print (diabetes_X)
# Split the data into training/testing sets
diabetes_X_train = diabetes_X[:-20]
diabetes_X_test = diabetes_X[-20:]
print (len(diabetes_X_train))
# Split the targets into training/testing sets
diabetes_y_train = diabetes.target[:-20]
diabetes_y_test = diabetes.target[-20:]
# Create linear regression object
regr = linear_model.LinearRegression()
# Train the model using the training sets
regr.fit(diabetes_X_train, diabetes_y_train)
# Make predictions using the testing set
diabetes_y_pred = regr.predict(diabetes_X_test)
print (“predict”,diabetes_y_pred)
# The coefficients
print(‘Coefficients: \n’, regr.coef_)
# The mean squared error
print(“Mean squared error: %.2f”
% mean_squared_error(diabetes_y_test, diabetes_y_pred))
# Explained variance score: 1 is perfect prediction
print(‘Variance score: %.2f’ % r2_score(diabetes_y_test, diabetes_y_pred))
# Plot outputs
plt.scatter(diabetes_X_test, diabetes_y_test, color=’black’)
plt.plot(diabetes_X_test, diabetes_y_pred, color=’blue’, linewidth=3)
plt.xticks(())
plt.yticks(())
plt.show()
Keras :code
import matplotlib.pyplot as plt
import numpy as np
from sklearn import datasets, linear_model
from keras.models import Sequential
from keras.layers.core import Dense, Activation
# Load the diabetes dataset
diabetes = datasets.load_diabetes()
diabetes_X = diabetes.data[:, np.newaxis, 2]
diabetes_X_train = diabetes_X[:-20]
diabetes_X_test = diabetes_X[-20:]
diabetes_y_train = diabetes.target[:-20]
diabetes_y_test = diabetes.target[-20:]
model = Sequential()
#model.add(Dense(2,1,init=’uniform’, activation=’linear’))
model.add(Dense(1, input_dim=1, kernel_initializer=’uniform’, activation=’linear’))
model.compile(loss=’mse’, optimizer=’rmsprop’)
model.fit(diabetes_X_train, diabetes_y_train, epochs=10000, batch_size=16,verbose=1)
#model.fit(diabetes_X_train, diabetes_y_train, epochs=1, batch_size=16,verbose=1)
score = model.evaluate(diabetes_X_test, diabetes_y_test, batch_size=4)
diabetes_y_pred = model.predict(diabetes_X_test,verbose=1)
print (“predict”,diabetes_y_pred)
# Plot outputs
plt.scatter(diabetes_X_test, diabetes_y_test, color=’black’)
plt.plot(diabetes_X_test, diabetes_y_pred, color=’blue’, linewidth=3)
plt.xticks(())
plt.yticks(())
plt.show()
This post will give you ideas on how to tune your model:
https://machinelearningmastery.com/machine-learning-performance-improvement-cheat-sheet/
Thanks Jason , I am able to get a better prediction by changing the below in keras and reduce the loss by changing this. However the same task is performed in Sklearn under 5 sec , however in the Keras + TF I have to run epoch=10000 with a batch_size=64, why so ?
model = Sequential()
#model.add(Dense(2,1,init=’uniform’, activation=’linear’))
model.add(Dense(1, input_dim=1, kernel_initializer=’glorot_uniform’, activation=’linear’))
sgd = SGD(lr=0.5, momentum=0.9, nesterov=True)
model.compile(loss=’mse’, optimizer=’sgd’)
model.fit(diabetes_X_train, diabetes_y_train, epochs=10000, batch_size=64,verbose=1)
It is jus ta worked example for regression, not a demonstration of how to best solve the specific problem.
Hi Jason,
Thanks for your numerous tutorials here! I have two questions:
1) Does StandardScaler() only scale the inputs X? Is it common to leave the output unscaled?
2) I have troubles using callbacks (for loss history in my case) and validation data (to get validation loss) with the KerasRegressor wrapper. Do you know how to do this?
Have a nice day.
For regression, it can be a good idea to scale the output variable as well.
I recommend not using the wrapper with callbacks.
Thank you Jason. This is a great place to start building own applications.
Thanks, I’m glad it helps.
Hello,
Great explanation,Thank you!
I applied this same logic and tweaked the initialisation according to the data I’ve got and cross_val_score results me in huge numbers. Could you please tell me why and what is to be done to get the correct accuracy(0.0-1.0) range.
Output:
Results: -99691729670.42 (106055766245.87) MSE
(My program’s aim to predict transaction amount based on past data, so it’s categorical data converted to one hot representaion)
Perhaps rescale your dat prior to modeling?
Perhaps tune the model to your specific problem?
Here are some more ideas:
https://machinelearningmastery.com/improve-deep-learning-performance/
Hi Jason how to select the best weights for the neural network using call backs,val loss as monitoring
Nice work.
Jason,
This is great stuff. Thank you.
I’ve a question. For Base Model,
print(“Results: %.2f (%.2f) MSE” % (results.mean(), results.std()))
I am getting:
Results: -27.40 (13.92) MSE
How to interpret negative number?
Regards
Ignore the sign.
sklearn will invert loss functions so that it can maximize them.
Thank you Jason
hello Jason , as i am working on regression related problem with 39998 rows and 20 coloumns in my training set and same array size(39998 cross 20) for target dataset , so i want to find the MSE on different architechture, i tried to code it but it gives some error or not gives proper MSE values ,Kindly help i am new in this field and stuck from last two weeks .my coded part will be given below
from keras.models import Sequential
from keras.layers import Dense,Activation
import numpy as np
import tensorflow as tf
from matplotlib import pyplot
from sklearn.datasets import make_regression
from sklearn.metrics import mean_squared_error
from keras.wrappers.scikit_learn import KerasRegressor
from sklearn.preprocessing import StandardScaler
from keras import models
from keras import layers
import matplotlib as plt
from sklearn.neural_network import MLPRegressor
seed = 7
np.random.seed(seed)
from scipy.io import loadmat
dataset = loadmat(‘matlab2.mat’)
Bx=basantix[:, 50001:99999]
Bx=np.transpose(Bx)
Fx=fx[:, 50001:99999]
Fx=np.transpose(Fx)
from sklearn.cross_validation import train_test_split
Bx_train, Bx_test, Fx_train, Fx_test = train_test_split(Bx, Fx, test_size=0.2, random_state=0)
scaler = StandardScaler() # Class is create as Scaler
scaler.fit(Bx_train) # Then object is created or to fit the data into it
Bx_train = scaler.transform(Bx_train)
Bx_test = scaler.transform(Bx_test)
def build_model():
model = models.Sequential()
model.add(layers.Dense(20, activation=’tanh’, input_shape=(Bx.shape[1],)))
model.add(layers.Dense(10, activation=’relu’))
model.add(layers.Dense(20))
model.compile(optimizer=’sgd’, loss=’mean_squared_error’)
return model
model = build_model()
model.fit(Bx_train, Fx_train,epochs=1000, batch_size=20, verbose=0)
test_mean_squared_error_score = model.evaluate(Bx_test, Fx_test)
What error?
Hello jason, I am working for detecting valence and arousal. So I need two output for MLP one for Arousal and other for Valence. I want to calculate the cross validation for r- squared score for both valence and arousal. I can able to train with two separate MLP model with one output but can’t train with one MLP with two output. The size of X and Y in load() function below is (2232 ,160) and (2232, 2) respectively.
X, Y = load()
kfold = StratifiedKFold(n_splits=10, shuffle=True, random_state=seed)
cvscores = []
for train, test in kfold.split(X, Y):
model = Sequential()
model.add(Dense(150, input_dim=160, kernel_initializer=’normal’, activation=’tanh’))
model.add(Dropout(0.5))
model.add(Dense(90, input_dim=160, kernel_initializer=’normal’, activation=’tanh’))
model.add(Dropout(0.5))
model.add(Dense(17,kernel_initializer=’normal’,activation = ‘tanh’))
model.add(Dropout(0.5))
model.add(Dense(10,kernel_initializer=’normal’,activation = ‘tanh’))
model.add(Dropout(0.5))
model.add(Dense(2, kernel_initializer=’normal’, activation = ‘tanh’))
model.compile(loss=’mse’, optimizer=’adam’, metrics = [‘mse’])
checkpointer = ModelCheckpoint(filepath=”model.h5″, verbose=1, save_best_only=True)
earlystopping = EarlyStopping(patience=50)
history = model.fit(X[train], Y[train], epochs=300, batch_size=100, verbose=1,callbacks=[earlystopping,checkpointer])
scores = model.predict(X[test])
accuracy = r2_score(Y[test], scores)
cvscores.append(accuracy* 100)
#print(“%.2f%% (+/- %.2f%%)” % (numpy.mean(cvscores), numpy.std(cvscores)))
print(‘the r-squared score for each fold’,cvscores)
print(“the mean of 10 fold cross validation is”, numpy.mean(cvscores))
print(“the maximum accuracy is “,max(cvscores))
However, I got the error in for loop: for train, test in kfold.split(X,Y):
The error massage is: cls_test_folds = test_fold[y==cls] IndexError: too many indices for array.
Thanks in advance
Perhaps you can use a multi-output model as described here:
https://machinelearningmastery.com/keras-functional-api-deep-learning/
hi, I am a fresh man to deep learning and learn from the wider_model, code is here:
def wider_model():
# create model
model = Sequential()
model.add(Dense(20, input_dim=X_train.shape[1], kernel_initializer=’normal’, activation=’relu’))
model.add(Dense(15, kernel_initializer=’normal’, activation=’relu’))
model.add(Dense(15, kernel_initializer=’normal’, activation=’relu’))
model.add(Dense(10, kernel_initializer=’normal’, activation=’relu’))
model.add(Dense(10, kernel_initializer=’normal’, activation=’relu’))
model.add(Dense(1, kernel_initializer=’normal’))
# Compile model
model.compile(loss=’mean_squared_error’, optimizer=’adam’)
return model
and three example of train data is
1,0.0,7,1,37.0,3,15.0,110106014000.0,110108004000.0,10,17721,2111,1160,2340,106,14115,699,2198
1,1.0,5,11,34.0,3,46.0,500108101000.0,500112012000.0,11,18615,2161,292,2188,407,15728,368,2246
1,0.0,5,19,35.0,3,37.0,120104003000.0,120105002000.0,11,5900,1251,209,469,87,5135,131,1222
what confused me was all my test data of predict result is the same, can you give me some suggestion, thanks.
Perhaps the model requires further training or tuning?
Yeah, thanks for your response. I change epochs from 500 to 1500, it really make difference( predict output are not the same), but no obvious effect.
Hi Jason, sorry if this question has been asked already but I could not find it: what is your justification for using KerasRegressor instead of the .fit( ) method? Thanks!
Good question.
You can use the Keras API directly if you wish.
For beginners, it can be helpful to use the sklearn wrappers in order to leverage all of the sklearn tools, like data prep, grid search, metrics, etc.
Hi, Jason good tutorial. I want to know how can we do mulitiout regresson using deep learning? here in simple regression problem output value is one where if there are more target varibles in the output to do some kind of quality analysis..how can we do?
I mean I want to build a cnn network whuch take image as an input and produce multiple output value like size,depth,colour value aur some other numerical features, so it slike cnn using multout regression
Change the number of nodes in the output layer to the number of outputs required.
No its like two output are totally diffrent to each other. for same input , in intermidiate layer neuron for one output weight value update need to increase and for other output weight update need to decrease. One more thing input in an image matrix not any statistical data.
So I couldnot figure out what to do?
You must flatten an input matrix to a vector to input it for an MLP.
Is there any good article.. as i have to do multiout regression using deep neural network to measure performance parameter as continuous value and input is 2d image..like if i give image of electrical fan as input ..it produce output voltage,current capacity,wind speed,etc…like that
Hello mr Jason, thank you for this tutorial.
I am trying to make a regression model that predicts multiple outputs (3 or more) , using 9 inputs. The problem is that my inputs have the same scale ( between 0 and 1), but my outputs don’t. Can you tell me how can I build a model that standardize my multiple outputs, or is it not necessary ?
Thank you in advance.
Perhaps try with and without data scaling and compare the performance of the resulting model?
Hi Jason, in the above example, I just have to split the data into training and testing data without worrying about splitting the data into validation data right? And then i use this line
results = cross_val_score(pipeline, X_test, Y_test, cv=kfold)
where X_test is the input testing data
and Y_test is the output testing data that is to be compared with training data.
Or I don’t need to train/test split the data?
Thanks in advance.
That line performs k-fold cross-validation:
https://machinelearningmastery.com/k-fold-cross-validation/
Oh so it means that after performing k-cross validation, then i can use
scores = model.evaluate(X_test, Y_test) to evaluate model on the test data?
That is one approach.
Hi,Jason.
I use previous data to predict the current landuse.The training data and the testing data have an accuracy of 0.8.however, when I intend to use the current data to predict the future landuse, after model.predic,the result of the prediction is all negative numbers that is quite different from the real situation.
How to solve this problem, thank you!
Perhaps ensure that the data used to make a prediction is prepared in exactly the same way as data used to train the model, e.g. any standardization, normalization, etc.
I am little bit confused. Which variable is used to evaluate?
model.evaluate(X_test, Y_test)
does not work because model is defined inside a function. So after k-fold cross validation which variable is to be used to evaluate the model or predict the data?
THanks,
The model is evaluated on the test dataset.
You can learn more about test datasets here:
https://machinelearningmastery.com/difference-test-validation-datasets/
I meant which variable should i use. I got my answer in one of your comments. Perhaps it is
estimator.model.evaluate
Thanks.
In Section 3. you say that a further extension would be to normalise the output variable. However, intuitively this doesn’t make sense to me.
Surely a non-linear transform of the target variable would impair the training process of the model as the loss that the model is minimising will be understated. I expect that after training on the normalised target, the values predicted by the model would result in a much greater loss after being passed through the inverse of the normalisation function and compared against the true results.
In addition, wouldn’t normalisation restrict the predicitons between the maximum and minimum values that the target variables took in the training data? If you were to use this approach you would have to be confident that your sample accurately represented any extremes of the population.
Normnalization is a linear transform.
Many algorithms prefer to work with variables with the same scale, e.g. 0-1. E.g. methods that use a weighted sum or distance measures.
You’re right of course – I feel foolish for saying that normalisation was a non-linear transfrom in hindsight!
After a bit more thought I could see that if your target variables were very large (as might be for the case for housing prices), this could result in very steep gradients in your search space that might lead to numerical instability or overshooting by the gradient descent algorithm.
Thanks for the response!
Hi Jason, thank you for your efforts providing us with such wonderful examples.
according to the documentation, the cross_val_score returns an ‘Array of scores of the estimator for each run of the cross validation.’
What are these scores exactly? And why does only taking the mean (see: results.mean) provide us with the mean Squared error?
Yes, the array is the results from each model evaluated on each held out fold.
Hi,how can calculate percentage of squared error per sample and find out mean ?
Like percentage error for prediction of one sample and corresponding true value for that output…for all the samples and take mean of diifrence. In the output I have 4 neurons so I am predicting 4 continuous value. Per out put I want to find percentage of error and at the end mean of all erros for all 4 output values seprately.
You can calculate the error for one sample directly.
Hi Jason,
Thank you so much for this tutorial. I have a few questions. I have seen in other tutorials people defining a model, and then calling model.fit to train. How is this different than what you have done here? Also, if I wanted to save this model with all of its weights and biases and archetecture, how could I do that?
Thank you!
Here we are using the sklearn wrapper instead of using the Keras API directly.
You can use the Keras API directly and then save your model, here’s an example:
https://machinelearningmastery.com/save-load-keras-deep-learning-models/
Thank you! What would change if I used the keras API directly to create this model?
I have a suite of tutorials, you can start here:
https://machinelearningmastery.com/start-here/#deeplearning
Hi Jason, I’m learning a lot from your tutorials. Many thanks for your efforts!
I have a problem with regression: it appears to me that almost any neural net I design does not perform better than linear regression. So, I picked up your code from here, and compared the results with results from scikit-learn’s linear_model.LinearRegression. I use cross validation with the linear regressor as well (10 folds) and get a ‘neg_mean_squared_error’ score of -34.7 (45.57) MSE.
I find it difficult to compare these results with the results from your neural net as there is so much variation in the neural net results. Different experiment runs (with 10 fold cross validation) give me mean mse values from 31 to 39. Am I doing something wrong?
In general, are neural nets well-suited for regression?
I’m a newbee, and would really appreciate any suggestions you have for me. Thanks in advance!
It really depends on the problem. Some problems are linear and better solved by a linear method.
Neural nets are suited for noisy non-linear regression problems.
Thanks Jason, I perhaps should have clarified that the comparison I presented was on the Boston housing dataset.
So, I picked up your code from here, and compared the results of the neural net on the Boston housing dataset with results from scikit-learn’s linear_model.LinearRegression. I use cross validation with the linear regressor as well (10 folds) and get a ‘neg_mean_squared_error’ score of -34.7 (45.57) MSE.
This particular value does seem a little worse than the neural net performance you report, but it is not always so. Other experiment runs give me mean mse values from 31 to 39, some of which are quite comparable to the neural net results. Is this what you would expect or do you suspect that there might be something I’m doing wrong here.
Thanks again for your efforts, and for taking the time to answer all the comments!
Performance depends on the chosen model, it’s configuration, the preparation of the data and much more.
Never assume that one method is better than another for a dataset, use experiments to discover what works then use that.
Hi Jason,
If I understand it correctly, after each epoch run the algorithm tries to decrease the losses by adjusting the weights right? So, I suppose the final epoch shows the loss results corresponding to the most optimal set of weights. Why are these particular, final loss values for each cross validation not in the ‘results’ array?
It looks like Ãn the ‘results’ include the mean (or something) value of the loss values corresponding to each epoch. Why is this?
Not quite, the model can overfit the training data resulting in worse performance on the hold out set.
It’s hard to get “optimal” weights. Almost all of the field is focused on this optimization problem with different model types.
hi, I m getting same value for prediction with all test samples using ‘tanh’ activation function…but if i use relu function prediction get changed with all test samples.what is the reason behind this? is it vanishing gradient problem that makes network predicts same value for each test sample?
ex…with ‘tanh ‘y1_pred=0.8, y2_pred=0.8 y3_pred=0.8 its constant prediction for all samples
but if i use ‘relu’ y1_pred=0.8 y2_pred=0.87,y3_pred=0.9 which is ok as per my data.
I’m not really sure what you’re asking?
I would encourage you to use the activation function that results in the best performance for your model.
I am asking…same constant prediction value for all the test samples with ‘tanh’ activation .
When I use ‘relu’ function I am getting proper continuous changing value not constant predicction for all test samples.
What is reason behind this?Is it vanishing gradient problem with ‘tanh’
I don’t know what you are doing or seeing, sorry.
I doubt you are seeing a vanishing gradient problem because you are seeing continuous output.
Very well explained tutorial Jason. What are you thoughts on,
– When to modify the number of neurons in a layer?
– When to modify the number of layers in a network?
Thanks.
Always tune the number of nodes and layers for your specific problem. More here:
https://machinelearningmastery.com/faq/single-faq/how-many-layers-and-nodes-do-i-need-in-my-neural-network
Hi Jason,
I’m getting negative value of average MSE. Am I doing anything wrong?
Results: -5.76 (7.16) MSE
Standardized: -1.81 (4.37) MSE
And can we rescale only the output variable to (0-1) or should we rescale the entire dataset after standardization?
Yes, sklearn inverts the mse. I explain more here:
https://machinelearningmastery.com/faq/single-faq/why-are-some-scores-like-mse-negative-in-scikit-learn
Hi Jasone.
My dependent variables are categorical.
I transfer them with LabelEncoder(), to number [1, 2, ….18]. Then, I have 18 classes.
In my ANN model (Keras). I use :
———————————————
# Adding the input layer and the first hidden layer
classifier.add(Dense(output_dim = 6, init = ‘uniform’, activation = ‘relu’, input_dim = 1094))
# Adding the second hidden layer
classifier.add(Dense(output_dim = 6, init = ‘uniform’, activation = ‘relu’))
# Adding the output layer
classifier.add(Dense(output_dim = 18, init = ‘uniform’, activation = ‘sigmoid’))
# Compiling the ANN
classifier.compile(optimizer = ‘adam’, loss = ‘sparse_categorical_crossentropy’, metrics = [‘accuracy’])
# Fitting the ANN to the Training set
classifier.fit(X_train, y_train, batch_size = 10, nb_epoch = 100)
# In[Predicting the Test set results]
y_pred = classifier.predict(X_test)
# In[real result into y_pred2]
y_prd2 = np.argmax(y_pred, axis=1)
———————————————
y-pred is beetwin [0,1] and number of column is equal my classes 18.
I use np.argmax to extract one classe (Returns the indices of the maximum values along an axis.)
What you think of my activation functions (relu, relu and sigmoid) ? I can use “softmax” in Output?
If your dependent variable (target variable) is categorical, then you have a classification problem.
I recommend this tutorial:
https://machinelearningmastery.com/tutorial-first-neural-network-python-keras/
Thank you!
Yes, I know I have classification problem and I have 18 classes.
But not binary classes.
You sent me to tutorial of binary Output !!!
I developed my model I only search, if I have error or something
Here is an example of multi-class classification with Keras:
https://machinelearningmastery.com/multi-class-classification-tutorial-keras-deep-learning-library/
I found this same code on Kaggle but it doesn’t seem like credit was given:
https://www.kaggle.com/hendraherviawan/regression-with-kerasregressor/notebook
That’s a shame.
Hola Jason,
Thank you. I have 2 questions.
1) Do you have more post or cases study on regression ? could you provide me with the links? I think there are more logistic regression and multi-class classification than pure regression post on your big numbers of tutorials.
2) In the last output layer corresponding to a dense layer of the model, when you omit the activation argument is by default equal to “linear”? is there any other useful activation or are always = “linear” in the case of regression analysis?
many thanks
many thanks for your help
I don’t have a lot on regression, it’s an area I need to focus on more. I do have more on time series (regression) than I do vanilla regression.
It’s always linear for regression. Sometimes sigmoid or tanh if you need real outputs in a bounded domain.
Hii Jason,
I have two questions for you.
1. In the above example we are getting one column of output my question is how can i get two column of output at the same time.
2. http://archive.ics.uci.edu/ml/datasets/Wine+Quality ….. For this type of dataset how can i implement regression and classification in the same model.
Thanks you for all your tutorials.
To get two column output, change the output layer to have 2 nodes.
Maybe you have have two output submodels, one for regression and classification. I have not tried this so I don’t know if it will work.
Perhaps fit one model for regression, then fit another model to interpret the first model as a classification output.
I got a negative value for the baseline model , is there a problem? I don’t understand why!!
No problem, the API has changed since the post was written, more here:
https://machinelearningmastery.com/faq/single-faq/why-are-some-scores-like-mse-negative-in-scikit-learn
Hi,
First of all, thank you for this post.
My question is: if I have (for example) two outputs , I should change only the columns number in Y definition and the neurons number in the output layer to 2?
Thanks in advance.
Correct.
Hi,
I tried this code for regression with 2 outputs , I didn’t get any error while executing , but at the end I get:
Result: nan (nan) MSE ???
Sorry to hear that, perhaps try updating your python libraries?
Perhaps try re-running the example a few times?
Did you resolve the nan issue? I got the same results. Here are my library versions:
scipy: 1.3.1
numpy: 1.16.4
matplotlib: 3.1.1
pandas: 0.25.1
statsmodels: 0.10.1
sklearn: 0.21.2
Theano:1.0.4
tensorflow 2.0.0
keras: 2.3.1
Ok I found the problem. When you download the housing data, dont open it in excel, just copy paste the data as is into a notepad text file and save as csv. If you do something in excel (text to columns) then nans get introduced in the data.
I see, thanks for sharing!
Which example in the above tutorial are you getting a nan with exactly?
Hi,
Thanks a lot for this excellent tutorial!
I have a question regarding string inputs to the neural network model.
I have 6 different categorical data input columns (A, B, C, D, E, F) and four of them has 5 different input values and two of them has 4 different input values. For example input A can have values A1, A2, A3, A4 and A5. Simliary for inputs B, C and D. And input E can have values E1, E2, E3 and E4. Similary for input F. I encoded then using labelencoder first and then I used Onehotencoder as mentioned in your post (https://machinelearningmastery.com/how-to-one-hot-encode-sequence-data-in-python/). So after one hot encoding I have 28 columns in my input numpy array (4 inputs with 5 settings each and 2 inputs with 4 settings each encoded using onehot encoding). My question is what will be the input dimension and layers to the command model.add(Dense(layers, input_dim))? Since you have 13 inputs you specified the input_dim as 13, in my case after one hot encoding I have 28 input columns. Will it be 28 and I have to specify to the model that it is one hot encoded? Could you please suggest how can I do this?
If each sample was encoded and has 28 values, then the input shape is 28.
Hi,
Thanks for the reply.
For example if one of my sample [‘A2’ ‘B5’ ‘C5’ ‘D4’ ‘E5’ ‘F1’] looks like this after encoding [0. 1. 0. 0. 0. 0. 0. 0. 0. 1. 0. 0. 0. 0. 1. 0. 0. 0. 1. 0. 0. 0. 0. 1. 1. 0. 0. 0.]. So actually this does not represent 28 binary inputs, but it represents 6 one hot encoded inputs. Would the model give a wrong prediction if it considers this as 28 binary inputs?
I don’t know how well your model will work on your data.
Your representation is correct, 28 inputs features/variables.
You might also like to try other representations, such as an integer encoding and an embedding.
Hi Jason, is root mean squared error also a good means of evaluation to understand the context of the problem is thousands of dollars? It’s been a while since I read your other post but I could swear it was rmse.. when would you use mse vs rmse for reporting results? Thanks
Generally MSE is used for loss and RMSE is used to report the estimated performance of the model, mainly because RMSE is in the original units, where MSE units are squared original units.
Hi Jason when reporting results MSE and RSME these always logged over each epoch and it’s a mean value from first epoch to last, right??
MSE is reported each epoch.
You can calculate RMSE from MSE by taking the square root.
Sorry to be beating a dead horse. So MSE is reported at each epoch and stored in a python list. Then the mean value of all MSE’s is calculated when the training is finished followed by the square root of all MSE’s to calculate the overall RSME. That’s correct, right? Thanks Jason
RSME = math.sqrt(statistics.mean(MSEs))
It would be better/more-correct to calculate the mean RMSE value directly, rather than the mean MSE and then square root.
Alternately, you can also track the RMSE as a metric each epoch:
https://machinelearningmastery.com/custom-metrics-deep-learning-keras-python/
Thanks for the link Jason. Can you give me a tip on how to create a loss plot from the code in this blog post using the KerasRegressor method and passing a function. It seems like it’s easier to create a loss plot with a history = model.fit() method but the code here doesn’t use model.fit()
I recommend using the Keras API directly in order to retrieve and plot the history.
Hi Jason, if I wanted calculate RSME from the last line in your code:
print("Standardized: %.2f (%.2f) MSE" % (results.mean(), results.std()))
Would I need to do:
print("RMSE", math.sqrt(results.mean()))
OR
print("RMSE", math.sqrt(results.std()))
?You can calculate the RMSE from MSE by taking the square root.
Im using a different dataset than the Boston housing… Is there any recommendations for these parameters?
batch_size=5, verbose=0
OR is it just additional parameters to experiment with to achieve best results?
Thank you for your great tutorials…
The verbose parameter controls what is printed during training.
For more on batch size, see this post:
https://machinelearningmastery.com/how-to-control-the-speed-and-stability-of-training-neural-networks-with-gradient-descent-batch-size/
It is a great tutorial overall. But I keep coming to errors, and finally stuck at this one.
‘TypeError: zip() argument after * must be an iterable, not KerasRegressor’
Could you help me with this? It is something wrong in the line, ‘ pipeline = Pipeline(estimators)’
Thank you!
Sorry to hear that, I have some suggestions here as a first step:
https://machinelearningmastery.com/faq/single-faq/why-does-the-code-in-the-tutorial-not-work-for-me
I AM GETTING NEGATIVE MSE. IS IT NORMAL???
Yes, this is a common question that I answer here:
https://machinelearningmastery.com/faq/single-faq/why-are-some-scores-like-mse-negative-in-scikit-learn
Please correct me If I am wrong! In the above problem we are using RELU activation function and MSE as the loss function right??
However, my question is why not a linear activation function?
Also, my second question may be out of the scope of the above article –
In this paper https://medium.com/the-theory-of-everything/understanding-activation-functions-in-neural-networks-9491262884e0, for linear activation function there is mentioned a couple of issues like :
1) Output value is not bounded (Which is not a problem in my case)
2) No point of stacking more than one input layer…because it would ideally lead to a linear function only. Also, the gradient remains constant all along!!
I am a beginner in this…any suggestion / study material to help me better understand the issue with using a linear activation function, how to overcome that problem, How is RELU relevant for the house prediction problem, can I apply it in my case??
Thanks a lot in advance!
Specifically I am working on developing a model that predicts multiple targets/target variables that are supposed to be continuous values!
Incorrect. The model is using a linear activation in the output layer.
It is a very good tutorial overall. But I keep getting negative MSE from the beginning using same data and code. Any suggestion?
The scikit-learn library will invert the MSE, you can ignore the sign.
I have 5 real values outputs(among 16 parameters, 11 are inputs and 5 are outputs of continues variables). How can I train the neural network? Which optimization algorithm is the best? what are the performance evaluation metrics for such a network? Thank you very much
Perhaps try a range of model configurations and tune the learning rate and capacity.
I give some ideas here:
https://machinelearningmastery.com/start-here/#better
Could you hep me in integrating Genetic Algorithm with Neural Networks using Back-propagation to predict real values quantity (to solve regression problem) with 8 inputs and 4 outputs. I would be very grateful if I am privileged to have python code for this, with a sample data-set. Thank you very much
Sorry, I don’t have an example of using a genetic algorithm for finding neural net weights. I hope to give an example in the future.
Thank you very much!
You’re welcome.
Looking for Back-propagation python code for Neural Networks prediction model for Regression problems. Actually for Classification problems you have given us lots of samples. Any help for Neural Network Samples for regression problems using Back-propagation methods? Thanks
Yes, the tutorial above uses backprop for regression.
Hi Jason,
thanks a lot for all your tutorials, it is really helpful.
i have a question, is there any type of ANN that takes several inputs and predict several outputs, where the each output is linked to different inputs. e.g let’s say output y1 is linked to x1,x2and x3 where y2 is linked to x1 and x4.
thanks a lot in advance
Yes, all neural nets can do this.
Perhaps start with a simple MLP and specify the number of outputs required as the number of nodes in the output layer.
Hi sir. i have 2 datasets in .mat files.
my project is to predict pitch from mfcc using dnn.
mfcc values are independent values and pitch are dependent.
mfcc are nx26 matrix and pitch is nx1 matrix.
i have split the data into train and test and again i have split train data into train and validation.
i am using sequential model with 4 hidden layers each containing 256 neurons.i have trained the model and everything is going fine. But, i am getting very high rmse value of 53.77.
what should i do to reduce the rmse value.
here is my code:
from scipy.io import loadmat
from sklearn.cross_validation import train_test_split
import numpy as np
import sys
import math
from keras.models import Sequential
from keras.layers import Dense,Flatten
from keras.callbacks import EarlyStopping
df=loadmat(“mfcc.mathandles1”)
df1=loadmat(“pitch.mathandles1”)
x=df[‘list1’]
y=df1[‘list1′]
#split into train and test
xtrain,xtest,ytrain, ytest = train_test_split(x,y,test_size=0.3,random_state=10)
#split into validation
xtrain,xval,ytrain, yval = train_test_split(xtrain,ytrain,test_size=0.3,random_state=10)
#sequential model
model=Sequential()
#input layer
model.add(Dense(26,input_shape=(26,)))
#hidden layers
model.add(Dense(256,activation=’relu’))
model.add(Dense(256,activation=’relu’))
model.add(Dense(256,activation=’relu’))
model.add(Dense(256,activation=’relu’))
#output layers
model.add(Dense(1,activation=’linear’))
#now our model is trained
#compile the model
model.compile(optimizer=’adam’,loss=’mae’,metrics=[‘mse’])
#set earlyy stopping monitor so the model stops training when it wont improve anymore
earlystopmonitor=EarlyStopping(monitor=’val_loss’,mode=’min’,verbose=1,patience=3)
validset=([xval,yval])
#train the model
model.fit(xtrain,ytrain,nb_epoch=50,validation_data=validset,callbacks=[earlystopmonitor])
#prediction on test data
predictions=model.predict(xtest)
print “predictions:”,predictions
#find error i.e find how much loss
error=predictions-ytest
sqr=error**2
#print “sqr:”,sqr
#find mse for test data
mse=np.mean(sqr)
print “mse for test data is:”,mse
#find rmse
rmse=math.sqrt(mse)
print “rmse of test data:”,rmse
#get loss and accuracy
val_loss,val_acc=model.evaluate(xtest,ytest)
print “val_loss:”,val_loss
print “val_acc:”,val_acc
please do help me out in this.
I have some suggestions here:
https://machinelearningmastery.com/start-here/#better
Thanks Jason for the tutorial. I have a quick question.
I want to build a model to predict the steering angle of an rc car. The input to the model will be images collected from a raspberry pi camera and the targeted outputs signal values ranging from 1000 to 2000. The targeted output values are not continuous . They exist in the form : 1000, 1004, 1008, 1012…
So, should I treat this as a classification or a regression problem ? Do I need to rescale the output and map them to smaller values such that 1000–>0, 1004–>1, 1008–>2, 1012–> 3… In other words what kind of pre-processing technique could I apply to the target output to make my model more efficient ?
Thank you in advance…
Sounds great.
Sounds like regression, but perhaps try modeling it as both a regression and classification problem and see what works well/best.
Test a suite of preprocessing to see what works for your choice of problem framing and algorithms. E.g. angles, integers, floats, ordinal categories, etc.
Hi Jason, this is excellent! I am hoping to learn to incorporate neural network regression into my work and this will help me a lot.
I have a question regarding the difference between neural networks for classification and regression; as I understand, the output activation function in a classification neural network, for example sigmoid, results in a value from 0 to 1 which we can then translate into a class (from a probability with softmax, or just picking the output neuron with the highest output.)
However, I am confused about the difference between this approach and regression applications. Since we try to predict continuous values that extend beyond [0,1], it seems to me that an activation function is not appropriate. How does the regression approach handle this?
Thank you for your time!
Surendra
Good question, I answer it here:
https://machinelearningmastery.com/faq/single-faq/how-can-i-change-a-neural-network-from-regression-to-classification
Does that help?
Yes it does, thank you!
I see that the output function is set to “linear” and the keras documentation refers to this as “identity” – does that simply refer to the sum of the weighted outputs from the last hidden layer? So effectively no output activation function?
Thanks!
Surendra
Identity means multiplied by 1 (i.e. unchanged). It outputs the weighted sum directly, as you say.
Excellent, I really appreciate it!
Hi, its great tutorial,
do you have any tutorial about Residual connection in Keras ?
Yes, this will help:
https://machinelearningmastery.com/how-to-implement-major-architecture-innovations-for-convolutional-neural-networks/
Hi Jason,
Great tutorial. still very fruitful to continue the machine learning process, after all these years studying.
1) I extend your code to implement also ‘MinMaxScaler’ Module, but the results are worst than ‘StandardScaler’ (e.g. 42.7 mean ‘mse’ vs 21.7 )
Why? when it is recommended to use one vs other module?
2) I extend your code to implement dropout layers and/or ‘ l1_l2’ Keras regularizers, but the results are little worst (e.g. 38.5 mean ‘mse’ vs 21.7 9 (in addition to more complex computation or network).
it seems clear the network propose here is very simple (e.g. around several thounds of weights or params to be trained) but also the dataset is small (506 instances with 13 features). So it seems there is not overfitting requirement so no need to implement regularizers such as l1_l2, dropout layers, etc. Ok.
I was thinking to propose a new simple definition of some parameter (or rate9 that relate amount of weights divided by the amount of input samples and or input features) to relate model complexity vs dataset size ? in order to anticipate when we are going deal with overfitting (or not). have you read or know something about it ? I can not figure out I am the first one…
2.1) In order to overcome overfitting there is a ‘concept’ called data augmentation for image datasets. It seem to generate additional images by ‘distorting’ original images. Ok .
But what about when we have data as is it the case of BostonHouses, etc? Do we have a similar Keras tools for not imaging preprocessing?
The only thing I am going to explore is applying GAN (adding Gaussian Noise to data) but I am not sure is there anymore tools or if it have the same effect of data augmentation for these kind of data (e.g. Boston Houses Prices).
3) By the way, I also extend the code to implement manually a exactly equal network model of the one your titled ‘wider’ network, in order to plot training and validation learning curve (via history function of .fit method), but I am confused because I obtained ver small values such as ‘0.01’ for ‘val_mean_squared_error’ parameter vs the ’21. 8′ value shown for mean of ‘mse obtained using the sklearn kfold tool with pipeline. But the rest or results are very similar…
Any idea of this fact? am I comparing not such equal parameters?
Jason, thank you for your great job, still opening the way for all of us.
JG
Great questions as always JG!
I always value your comments.
Normalization is a good default, and standarization is good when data is gaussian. Nevertheless, always test both (and no scaling) and use what gives the best results.
https://machinelearningmastery.com/how-to-improve-neural-network-stability-and-modeling-performance-with-data-scaling/
Theories/heuristics on setting number of nodes/layers are unreliable and have been for decades. Treat as a hyperparameter and tune. Alternately, provide excess capacity and use regularization to cut overfitting.
https://machinelearningmastery.com/how-to-control-neural-network-model-capacity-with-nodes-and-layers/
Yes, we can add gaussian noise to existing samples as a type of data augmentation, can be effective:
https://machinelearningmastery.com/train-neural-networks-with-noise-to-reduce-overfitting/
More here:
https://machinelearningmastery.com/how-to-improve-deep-learning-model-robustness-by-adding-noise/
If you are using an sklearn pipeline with scaling, then reported error will be on the scaled data I believe.
thank you for your fedd back Jason!
I continued reading your broad, deep and well structured multiple machine learning tutorials
Thanks JG.
Hi there,
What can I say… you saved my day. Excellent tutorial.
I am working with a dataset of about 63Million rows and 17 features… and will try subsampling, it is becoming unworkable on my PC (32GB RAM).
Your tutorial helped me with serious doubts I had.
Thanks, I’m glad it helped.
Well done on your progress!
Hi, sir
I have a problem.
I have built model but i can’t get probability result
In building model, i have softmax as a activation function.
I tried to get the probability result to use prediction function but i had not.
Could you explain this?
Perhaps the examples in this post will help:
https://machinelearningmastery.com/how-to-make-classification-and-regression-predictions-for-deep-learning-models-in-keras/
Thank you sir!
I have already above article but i didn’t find a answer.
I have built the model following:
def build_model(input_shape=(28, 28, 1), classes=charset_size):
img_input = Input(shape=input_shape)
x = Conv2D(32, (3, 3), activation=’relu’, padding=’same’,name=’block1_conv1′
(img_input)
x = Conv2D(64, (3, 3), activation=’relu’, padding=’same’,
name=’block1_conv3′)(x)
x = Conv2D(64, (3, 3), activation=’relu’, padding=’same’,
name=’block1_conv4′)(x)
x = MaxPooling2D((2, 2), strides=(2, 2), name=’block2_pool’)(x)
x = Dropout(0.1)(x)
x = Flatten(name=’flatten’)(x)
conv_out = (Dense(128, activation=’relu’, kernel_constraint=max_norm(3)))(x)
x1 = Dense(charset_size, activation=’softmax’)(conv_out)
lst = [x1]
model = Model(inputs=img_input, outputs=lst)
return model
def train(model):
train_datagen = ImageDataGenerator(
rescale=1. / 255,
rotation_range=0,
width_shift_range=0.1,
height_shift_range=0.1
)
test_datagen = ImageDataGenerator(rescale=1. / 255)
train_generator = train_datagen.flow_from_directory(
train_data_dir,
target_size=(img_width, img_height),
batch_size=128,
color_mode=”grayscale”,
class_mode=’categorical’)
validation_generator = test_datagen.flow_from_directory(
test_data_dir,
target_size=(img_width, img_height),
batch_size=128,
color_mode=”grayscale”,
class_mode=’categorical’)
model.summary()
model.compile(loss=keras.losses.categorical_crossentropy,
optimizer=keras.optimizers.Adam(),
metrics=[‘accuracy’])
model.fit_generator(train_generator,
steps_per_epoch=nb_samples_per_epoch,
epochs=nb_nb_epoch,
validation_data=validation_generator,
validation_steps=nb_validation_samples)
# — get prediction —
img = cv2.imread(filepath)
x = img_to_array(img)
x = x.reshape((-1,) + x.shape)
prediction = model.predict(x)
what’s wrong?
Could you tell me about it more exactly?
This is a common question that I answer here:
https://machinelearningmastery.com/faq/single-faq/can-you-read-review-or-debug-my-code
Hi Jason,
Thanks for your helpful tutorial. I am a complete beginner and seem to be stumbling a lot! I’m trying to run this code on my own dataset, which also has 12 variables as input and 1 as output. When I run the code, either nothing at all happens, or I get the following error message:
Y = dataset[:,13]
IndexError: index 13 is out of bounds for axis 1 with size 1
Any ideas what I’m doing wrong?
Many thanks, Amy
If you have 12 variables, change 13 to 12.
For more on how indexing arrays works in python see this:
https://machinelearningmastery.com/index-slice-reshape-numpy-arrays-machine-learning-python/
Thank you Mr Jason.
You’re welcome.
Hi,
I’ve a regression problem (Keras/TF) with values to be predicted that can be positive or negative.
In my case, an error of sign is a big error.
So I was wondering if there is any standard loss function or mechanism that can take this into account or if a custom loss is needed?
Regards
Perhaps scale the data prior to fitting the model. It makes a big difference.
Hi, I did that already.
However the point here is that for example if the target to be predicted is -0.1, I would rather like the model to predict -0.25 rather than +0.05 (same for positive target).
I don’t follow, sorry.
I am building a deep network with 43 predictors. I am getting a good result from my model if I set the epoch value to 2000. Is that value acceptable??
Use whatever configuration gives the best results.
Thank you for your reply. Is it possible to fit a nonlinear equation through keras? I Have an equation: y = a*exp(-b*x) + c*(1-exp(-b*x)) where a,b,c are the coefficients I want to estimate. x predictor variable and y predicted variable
Sure, separate the data into examples of inputs and outputs and fit a model!
Is there any proper example available? I found this one -https://www.kaggle.com/vsunday/polynomial-regression-with-keras-just-for-fun
but it is hard to interpret as it has not been explained properly
Sorry, I don’t have an example, you can adapt the example in the above tutorial.
Hello Jason,
is it possible to insert callbacks into KerasRegressor or do something similar?
I tried it like this and got an error.
monitor_valloss_6= EarlyStopping(monitor=’val_loss’, patience=3)
…
regression_classifiers2.append((‘mlp’, KerasRegressor(build_fn=big_regression,
epochs=25, batch_size=1000, verbose=0,
callbacks=[monitor_valloss_6])))
RuntimeError: Cannot clone object , as the constructor either does not set or modifies parameter callbacks
Not really. It might be easier to use the standalone Keras API.
Thanks a lot for your answer.
You’re welcome.
Hi, I am using your tutorial to help me in my undergraduate thesis.
I have a data set of the daily polimerization values of a power transformers.
I have a total of 434 days of data. I had no problem to load my file (set X as days 1 to 434 and set Y as the polimerization values) and run your code. My only change was:
model.add(Dense(434, input_dim=1, kernel_initializer=’normal’, activation=’relu’))
Because I only have one input and 434 instances.
I want to use the regression example you gave to predict how will the polimerization be at, let’s say, day 1500. I have ran your example, and got the following output:
Baseline: -20.80 (22.63) MSE
Where in your code you define what is the exit? I am sorry, I am quite new to machine learning.
If I want to know how will be the output at day 1500, how should I procede?
Thanks in advance
It sounds like you are working with a time series forecasting problem.
I would recommend starting with the basics here:
https://machinelearningmastery.com/start-here/#timeseries
Then perhaps explore using neural networks for your problem here:
https://machinelearningmastery.com/start-here/#deep_learning_time_series
Hello, first of all, thanks for the great tutorial and for the whole site with so much usefull information.
I have a similar regression problem as this one, but with 2 inputs variables and 3 output variables (target). Two of those 3 targets have high values (around 1000~10000) and the third target got a really low value (around 0.1~0.9).
When i run my code, i’m getting a really high mse value at start, around 10 million, that quickly decreases to around 50000 after a few epochs. Could this be related to the magnitude difference between my output variables? And related to the metrics, wich one you advise someone to use in a regression problem?
Thanks.
It might be. I recommend scaling the data prior to modeling.
Hi Jason,
thank you for the great tutorial! I am trying to use your example, but the line
results = cross_val_score(pipeline, X,Y, cv=kfold) always produces an error
TypeError: can’t pickle _thread._local objects
Do you have an idea how I can fix this?
Thanks!
That is an odd error. I have some suggestions here:
https://machinelearningmastery.com/faq/single-faq/why-does-the-code-in-the-tutorial-not-work-for-me
hello,
i appreciate the tutorial greatly. i’m wondering if this model can be better with one-hot encoding, or is one-hot encoding unnecessary for this problem.
Thanks.
One hot encoding is for categorical variables, input or output. I don’t believe there are any categorical variables in this dataset.
I have a dataset that contains a few ? values and column titles. how can we integrate this code for this type of dataset? thanks
Remove the column titles from the dataset.
The ? might represent missing values, this will help:
https://machinelearningmastery.com/handle-missing-data-python/
Hi Jason,
How to handle very large datasets while doing regression in Keras. My data has around 30+ millions rows, What strategy would you suggest in my case?
Thanks!
Good question, I answer it here:
https://machinelearningmastery.com/faq/single-faq/how-to-i-work-with-a-very-large-dataset
How do I save the final model that is fit to the data?
Good question, see this tutorial:
https://machinelearningmastery.com/save-load-keras-deep-learning-models/
Hi there,
I tied an ANN on this dataset:
https://www.kaggle.com/c/house-prices-advanced-regression-techniques/overview
AND strangely I have got for 7 entries no predictions – so I had to fillna the missing values of the prediction.
Can you tell me what could be a reason for this?
If of any interest this is the model:
i = Input(shape=(D,))
x = BatchNormalization()(i)
x = Dense(500, activation=’relu’)(x)
x = BatchNormalization()(x)
x = Dense(300, activation=’relu’)(x)
x = BatchNormalization()(x)
x = Dense(100, activation=’relu’)(x)
x = BatchNormalization()(x)
x = Dense(1)(x)
model = Model(inputs=i, outputs=x)
model.compile(
loss=’mean_squared_error’,
optimizer=’adam’
)
r = model.fit(X_train_ss, y_train_ss, epochs=1000, batch_size=32)
with basically the following
This might help:
https://machinelearningmastery.com/faq/single-faq/can-you-read-review-or-debug-my-code
Hi Jason,
1) Is it possible to use MLP regression for predicting 4 different continuous values? Is it possible to train such a network?
2) I understand it is necessary to normalize the training and testing datasets. For regression, I normalize the attributes/features, X, but I want to know: is it also necessary to normalize the target outputs, Y?
I would greatly appreciate your reply. Thank you so much for sharing your knowledge!
Sure. Set the number of nodes in the output layer for the number of predictions you need per sample.
Yes, it is a good idea to normalize the target variable.
Hi Jason,
Thank you for your reply. In my application, the actual (before normalization) value of the output is important, in that they are coefficients which need to be used later on in my system. As such, by normalizing it, I would be losing the actual value of the coefficient. Is there any way to get around this?
Yes, you can use the same scaler object to invert the scaling afterward, e.g. scaler.inverse_transform().
Also, this might help:
https://machinelearningmastery.com/how-to-transform-target-variables-for-regression-with-scikit-learn/
And perhaps this:
https://machinelearningmastery.com/machine-learning-data-transforms-for-time-series-forecasting/
How do you freeze layers when using KerasRegressor wrapper?
You must freeze the layers on the Keras model directly.
I was having a terrible time with this example – getting stuck on an error that just couldn’t be solved, but eventually found the issue. For the sake fo helping others who may come across it:
As at today (23-01-20) If you are attempting to use MLFlow on this example, KerasRegressor will not function, returning the error:
‘ValueError: epochs is not a legal parameter’
This is caused by the autolog function in MLFlow (‘mlflow.keras.autolog()’). I’m unsure exactly why this is the case, but if you disable that, the example works as intended.
What is mlflow?
Why not use standalone keras as described in the tutorial?
MLFlow is an open-source tool for tracking experimental parameters and results, automatically. The idea is that when experimenting with a dataset, you’ll be messing with all sorts of parameters and settings, but may not have an ideal solution for storing all the different combinations you’ve tried. MLFlow just provides a clean UI for comparing experiments.
Using the standalone keras works fine – I was just trying to adapt it with this MLFlow to see how easily it could slot in.
Fascinating, thanks.
Can you please tell me how to make predictions on the test data using the pipeline?
yhat = pipeline.predict(newInput)
For more see this:
https://machinelearningmastery.com/make-predictions-scikit-learn/
Hi Jason,
Fantastic work.
In traditional regression problems, we need to make sure that our time series are stationary.
We also need to make sure that residuals are stationary and there is no autocorrelation in the residuals.
When performing a regression using a neural network, do we also need to make sure that our time series are stationary and that our residuals are stationary and non-autocorrelated.
Thank you!
Boris
Thanks.
Yes, often it is a good idea. Test with and without.
Hello,
Thank you for the tutorial. It’s a good work.
Man, what do you recommend for a multi-input multi-output regression problem. I have been trying so hard to increase the accuracy and decrease the loss function but it’s like nothing is working. The model is not increasing its accuracy from 40% and loss function is stuck at 44 something.
Any suggestions? I have tried everything changing the ANN structure, playing with different optimizers, loss functions, epochs, activation functions, preprocessing of input data using keras (but did not do the preprocessing on the output data). One thing I did about the output data is, output does not have a threshold in negative or in positive axis so I processed the data in the form of only 0 or positive by taking the absolute values and added more nodes in the output by introducing the signs of each output label (0 for negative, 1 for zero and 2 for positive) so initially my output labels were 6, now there are 12 output labels. Nothing has worked. Suggestions?
Current architecture: [Dense(32, input_shape(1, 6), activation =’relu’),
Dense(32, activation =’relu’),
Dense(32, activation =’relu’),
Dense(12, )]
I am open to suggestions.
Thanks.
Perhaps start here:
https://machinelearningmastery.com/multi-output-regression-models-with-python/
Then experiment with MLPs to see if they can do better – often not.
Thanks man!
You’re welcome.
Hi Jason,
Huge fan love your work. Weird question, when I build an MLP regressor in Keras, similar size and depth to what you have here, I’ll train it using MSE as the loss (have also tried with MAE and MAPE) and will converge to a very low loss value. I’ll do a forward pass on my test data (about 3000 entries) and take the average error, which will be crazy low, like .03%. So I think my model is incredible. Then I look at the forward pass predictions, and each one is the same value. No matter what I input to the model, it’s outputting the same numerical prediction, which happens to be extremely close to the mean of the target vector I input.
Essentially it appears that my MLP is learning the mean, then outputting the mean for each pass, regardless of the input. I’ve tried a few things:
– Data scaling (MinMaxScaler)
– Testing with other optimization functions (I prefer Adam, with a decreasing lr, which I’ve also modified to no avail)
– modifying the depth and width of the MLP – if I make the network shallower it wont converge, and after adding more than 2 layers it starts doing this mean approximation thing again
Any thoughts on this?
Thanks!
Perhaps the model has overfit, this might help diagnose the issue:
https://machinelearningmastery.com/learning-curves-for-diagnosing-machine-learning-model-performance/
I considered that as well – I output the MSE on the validation set with each training epoch (using and the training error is slightly higher than the validation error, but if I were to plot them it looks like the “good fit” graph from your post there, but the problem is that each output is an identical scalar value, regardless of the quantities in the input vector. The validation set error never exceeds the training set error.
Also, I saw a post that uses the validation_split command in Keras, I’m doing a TrainTestSplit using sklearn to split into test and validation sets. The issue is that every input from the validation set yields the exact same output value from the network.
Perhaps the validation set is not representative of the dataset? Perhaps try a 50/50 split or getting more data?
Hi Jason,
Thanks for the simple and yet infomative tutorial. Btw, regarding multi output, how should the syntax be?
My code is:
Y = dataset[:,13:14]
…
model.add(Dense(2, kernel_initializer=’normal’))
…
kfold = KFold(n_splits=10)
results = cross_val_score(estimator, X, Y, cv=kfold)
I got error:
ValueError: Error when checking model target: the list of Numpy arrays that you are passing to your model is not the size the model expected. Expected to see 3 array(s), but instead got the following list of 1 arrays: [array([[ 0.69945297, 0.13296847, 0.06292328],
…
I tried changing Y to:
results = cross_val_score(estimator, X, [Y[:,0],Y[:,1]], cv=kfold)
but there’s also error:
Found input variables with inconsistent numbers of samples: [72963, 3]
So what’s the correct syntax for multi output?
Thanks again.
See the examples here for multi-output time series with LSTM:
https://machinelearningmastery.com/how-to-develop-lstm-models-for-time-series-forecasting/
And here for MLPs:
https://machinelearningmastery.com/how-to-develop-multilayer-perceptron-models-for-time-series-forecasting/
Sure, thanks for the links!
You’re welcome.
Thanks a lot!
You’re welcome.
Dear Jason,
Can I use this regression model in NLP task where I want to predict a value using some documents
Yes, but perhaps these tutorials would be a better start:
https://machinelearningmastery.com/start-here/#nlp
Would you suggest this also for time series regression or would you use another machine learning approach?
I would recommend testing a suite of linear, ml, and deep learning methods to discover what works best, follow this framework:
https://machinelearningmastery.com/how-to-develop-a-skilful-time-series-forecasting-model/
Hey Jason, thanks a lot for your work! My question is why didn’t you proceed to a feature selection before having trained the neural network? Thanks in advance!
To focus the tutorial on the neural network – keep it simple.
Ok, thank you for your answer Jason! So… if I want to train a similar neural network, but for a linear model with 4 features, should I just change the number of neurons and the “input_dim” argument in the 2nd model.add() statement? Like:
model.add(Dense(4, input_dim = 4, kernel_initializer = ‘normal’, activation = ‘relu’)) # input_dim = nb d’inputs = nombre de noeuds dans le réseau
Thanks in advance (sorry for these questions, I’m still a beginner in NNs)!!
Yes, except the number of nodes in the first hidden layer is unrelated to the number of input features.
You only need to set the input_dim argument.
Ok, thanks a lot! So I keep the same number of nodes as you in my 3 NNs.
And just a last question Jason, is there any mean to display the cost function plot? I tried a several things and I did’nt work…
Many thanks in advance!
Perhaps this will help:
https://machinelearningmastery.com/display-deep-learning-model-training-history-in-keras/
Hi, how long does the first baseline model take to run approximately?
Thanks in advance
Seconds I think.
Hello Jason,
I want to build a model that predict whether the audio(.wav) file and the text string are same(nearly matches) or not. But I am unable to find any post regarding that.
Any help will be appreciated.
Thanks
That sounds like a great project.
Perhaps you can use a model to convert the audio into text then compare the text directly.
Hi Jason,
Thank you very much for your great tutorials!
I’ve run the regression code on Boston housing data and plotted the NN prediction on test data. It looks that the predictions have been shifted by an offset. Surprisingly it is the same for the training data… Please let me know your idea.
Thanks,
Do you mean time series forecasting?
If so, this is a common problem:
https://machinelearningmastery.com/faq/single-faq/why-is-my-forecasted-time-series-right-behind-the-actual-time-series
Thanks. you are a masterrr!!! Another great tutorial
Thank you!
can I select features by RFE than make regression with deep learning
Sure.
Hi Jason,
Do you’ve any post or example regarding regression using complex numbers. My input data is complex numbers and the output are real numbers. Currently I’m handling by splitting the complex number into real and imaginary part but not sure about its validity.
Sorry I do not.
Hi Jason, I am currently doing a regression on 800 features and 1 output. I am wondering how many layers and neurons should I use to achieve best outcome? How to do the tuning for this? Thank you!
Good question, this will help:
https://machinelearningmastery.com/faq/single-faq/how-many-layers-and-nodes-do-i-need-in-my-neural-network
Hi Jason,
Many thanks for another excellent article. I have managed to build an ANN and I was wondering how could I extract mathematical formulas that describe the model.
I have read scientific papers explaining the input they use, their hyper parameters & architecture of the ANN and they usually conclude by giving math equations for making predictions directly extracted from their ANN (including their input variables). I haven’t managed to find how to extract/formulate such formulas using Python.
Any pointers and help would be greatly appreciated!
Many thanks,
Iasonas
You cannot extract useful formulae from a model.
Hi, I have a single feature (input_dim=1) dataset with ~500 samples. How do I modify the code specifically, epoch, batch size and kfold count to get a good fit since I am noticing an extremely high MSE.
These tutorials will give you ideas on how to tune a neural net model:
https://machinelearningmastery.com/start-here/#better
Dear Jason,
You mentioned in the article that the Wider architecture yields better results than the Deeper architecture. Correct me if I’m wrong, but I believe it is the case in the article because you ran the code of the wider architecture for 100 epochs, while the deeper architecture for 50 epochs, in my tests when training both architectures with same number of epochs 50 or 100, the deeper architecture yields better results.
For Example:
With 50epochs:
Deeper model: -23.22 (25.95) MSE
Wider model: -24.30 (23.43) MSE
With 100 epochs:
Deeper model: -21.67 (23.85) MSE
Wider model: -22.50 (23.00) MSE
Nice work!
I got this error
File “C:\Users\Eng Maha\Regression_DL.py”, line 39, in
results = cross_val_score(estimator, X, Y, cv=kfold)
NameError: name ‘estimator’ is not defined
I suspect you have accidentally skipped some lines of code, perhaps this will help you copy-paste the example:
https://machinelearningmastery.com/faq/single-faq/how-do-i-copy-code-from-a-tutorial
Hi Jason!
Nice tutorial! I am completely new to regression problem using keras. What if my inputs are two matrice which shape are 10*41*81 and my outputs are two scalars? I have no idea how to deal with them….
I am looking forward to your reply!
Perhaps you can flatten the input to a vector?
Perhaps you can use a model that support more input dimensions like an LSTM or CNN-LSTM?
Thanks so much for your quick reply!! I will try to find me some tutorials regarding these models!
You’re welcome.
Hi,
I am trying to run this on a 6 dimensional input, but I’m getting this error:
ValueError: Input 0 of layer sequential_181 is incompatible with the layer: expected axis -1 of input shape to have value 6 but received input with shape (None, 1)
What might be the problem?
Thanks!
Perhaps check that you loaded your data as you expected and that the model is configured to expect your data.
Hi Jason!
Can I add a few CNN layers before all the dense layers? I have 130w inputs and 3 outputs, and I would like to have CNN layer to reduce the args somehow.
A 1D CNN is not appropriate for a regression problem unless your input data is a sequence.
Emmmm, my data are of oil field. They have a 151*72*64 shape. And I was thinking, maybe I could take 151 as width, 72 as height and 64 as channels. …? But I have no idea how to do it… Can 2D CNN work for my data?
You can specify the preferred shape for the input layer of your CNN model.
Perhaps start here:
https://machinelearningmastery.com/start-here/#dlfcv
WARNING:tensorflow:7 out of the last 12 calls to <function Model.make_test_function..test_function at 0x7f9390119400> triggered tf.function retracing. Tracing is expensive and the excessive number of tracings could be due to (1) creating @tf.function repeatedly in a loop, (2) passing tensors with different shapes, (3) passing Python objects instead of tensors. For (1), please define your @tf.function outside of the loop. For (2), @tf.function has experimental_relax_shapes=True option that relaxes argument shapes that can avoid unnecessary retracing. For (3), please refer to https://www.tensorflow.org/guide/function#controlling_retracing and https://www.tensorflow.org/api_docs/python/tf/function for more details.
I got this warning when change the model for 21 inputs. I changed the code as below,
X = dataset[:,0:21]
Y = dataset[:,21]
model.add(Dense(21, input_dim=21, kernel_initializer=’normal’, activation=’relu’))
sample data in csv is ,
0 0 1 0 0 0 0 0 0 0 0 0 1 0 1 0 0 1 0 0 1 5
Any idea on above?
Looks like a warning, perhaps you can safely ignore for now?
Perhaps search/post on stackoverflow?
The link (Boston house price dataset) in chapter 1 is not working.
Working link to ICS:
https://archive.ics.uci.edu/ml/machine-learning-databases/housing/housing.data
Since you have a working link already, you may just remove the first one.
Thanks for your work. Really helpful!
Thanks.
Hi Jason,
Can u tell me how to save this regression model and load that model and test with specific input?
Yes, see this tutorial on how to save and load a model:
https://machinelearningmastery.com/save-load-keras-deep-learning-models/
Hi, Jason, Thank for for the tutorial. I have this question. why is this code a deep network while the model have only one hidden layer? generally, a deep network have at least three hidden layesr.
All neural nets are referred to as deep learning now:
https://machinelearningmastery.com/what-is-deep-learning/
Dear Jason,
When I run the example in section 3, I got ‘Baseline: nan (nan) MSE’ as output. The CSV data I simply copied into a text file and I saved it as CSV(this has been suggested earlier in the comments section). The dataframes I can see in my variables and seem to be OK.
I do get a error when running the code, but this error did not stop the code:
2021-06-11 11:40:42.737123: I tensorflow/core/platform/cpu_feature_guard.cc:142] This TensorFlow binary is optimized with oneAPI Deep Neural Network Library (oneDNN)to use the following CPU instructions in performance-critical operations: AVX AVX2
To enable them in other operations, rebuild TensorFlow with the appropriate compiler flags.
2021-06-11 11:40:42.738253: I tensorflow/core/common_runtime/process_util.cc:146] Creating new thread pool with default inter op setting: 2. Tune using inter_op_parallelism_threads for best performance.
Do you might have a clue what is causing the NaN output?
Thank you in advance,
William
That is very odd, I have not seen that before.
Perhaps some of these tips will help:
https://machinelearningmastery.com/faq/single-faq/why-does-the-code-in-the-tutorial-not-work-for-me
Thank you for your reply, I will look into it.
> Reasonable performance for models evaluated using Mean Squared Error (MSE) are around 20 in squared thousands of dollars (or $4,500 if you take the square root). This is a nice target to aim for with our neural network model.
How do we determine the validity of the results, as mentioned here?
Many evaluation indices such as MSE state that “the smaller the better”, which is good for comparing two models, but I think it would be easier to explain to others if there is an index of how much value can be considered a valid result.
I compared the result to a naive model.
See this:
https://machinelearningmastery.com/faq/single-faq/how-to-know-if-a-model-has-good-performance
Thanks for the answer.
I see that it was already listed in QA.
I am sorry I did not check.
This article of yours answered my question.
https://machinelearningmastery.com/how-to-know-if-your-machine-learning-model-has-good-performance/
I would like to read it carefully.
For the baseline model, I refer to this.
https://machinelearningmastery.com/implement-baseline-machine-learning-algorithms-scratch-python/
For the regression model (which I am currently working on), I understood that I can compare these.
1. the evaluated value (e.g. RMSE) of the model with the central tendency (e.g. mean or median, mode, etc.) as the predictor as the baseline model
2. the value (e.g., RMSE) of the model you created (the model to be evaluated)
When the evaluation value is RMSE, “smaller (calculated by 2.) than (calculated by 1.)” is superior.
That’s what I mean. Is that correct?
If the performance of your model has better performance than a naive/baseline model on the same dataset and test harness, then that model has skill.
No problem!
I encountered with this error
C:\Anaconda\lib\site-packages\keras\engine\input_spec.py:250 assert_input_compatibility
raise ValueError(
ValueError: Input 0 of layer sequential_9 is incompatible with the layer: expected axis -1 of input shape to have value 13 but received input with shape (None, 10)
warnings.warn(“Estimator fit failed. The score on this train-test”
I don’t know which code caused this but the error message says it all. Likely that is some configuration error in the model, or your input is in a wrong shape.
Hi Brownlee,
Explanation is so neat and clear.
I have problem having signal strength quality of equipment’s(3,4,5,6,7,8) as target variable.
But need to prepare a regression model.
Type of output variable showing as integer. But these are classes right.
Now to prepare a nueral network,
do i need to change its type using one hot encoder.
How many neurons do i need to keep in output layer.
Please help me
For regression model, and the output (3,4,5,6,7,8) really means its value (i.e., not a category label), then you need only one neuron at output. If it is category label, which 3 not necessarily mean better or worse than 4 (i.e., no ordering relationship), then you need 6 neurons as there are 6 categories (and you’re not doing regression).
Using this model, how can we actually access / view the predicted values for datasets containing the 13 features without the price column?
Are you looking for model.predict() function?
Yes, but when I use the code
y_predict = model.predict(x_no_target) to predict the targets of this dataset (a subset of my original dataset containing the observations missing their target), I get a yellow warning on the word “predict” that says “Cannot find reference ‘predict’ in ‘function’ “.
Other NN tutorials I’ve done have not defined the model in a function the way you have, and the model.predict() function works fine in those tutorials, but here it gives me the warning then doesn’t spit anything out when I insert the following:
# Make a prediction
y_predict = baseline_model.predict(x_no_target)
# show the inputs and predicted outputs
for i in range(len(x_no_target)):
print(“X=%s, Predicted=%s” % (x_no_target[i], y_predict[i]))
Then in the Python Console I get “AttributeError: ‘function’ object has no attribute ‘predict'”
I think your “baseline_model” is not really a model created. Check the previous lines of code on how this is created. Quite likely you missed something.
Hi! Great tutorial!
How could I modify the code so that I know the values that the Neural Network predicts?
I need to compare each real output with the output calculated by the model.
Hi Marc…You may find the following of interest:
https://machinelearningmastery.com/regression-metrics-for-machine-learning/
Hello Jason,
How I can predict a new house price using trained model?
Hello Viktoriia…You are describing transfer learning:
https://machinelearningmastery.com/transfer-learning-for-deep-learning/
Dear, Jason.
In case the baseline model gives the best result (as is the case with my dataset), is that the model I should use? I mean, if the baseline model gave better results, it could not be perhaps because the different scales between columns would be causing only some of them to be considered by the model because they would (wrongly?) have a greater weight? Would it be correct to consider the baseline model as the best just because it has the best result (closer to 0) or is it always necessary to use a scaler when we have different orders of magnitude between columns? It seems to me that using tree-based machine learning techniques it is not necessary to scale the data, but it is necessary when using distance-based techniques. I’m right? How would it be in the case of neural networks?
Thank you.
Dear Jason, Is it possible to stack the NN regression?
using the same concept you used here?
‘Stacking Ensemble for Deep Learning Neural Networks in Python’
Hi Chucks…The following may be of interest to you:
https://towardsdatascience.com/just-keep-stacking-implement-stacking-regression-in-python-using-mlxtend-3250ff327ee5
Thanks a lot for such an informative tutorial
As you know, we have base_estimator Hyperparameter in BaggingRegressor Function.
Can we define an MLP Regressor Model with Keras and pass it to the BaggingRegressor’s base_estimator to improve the accuracy and reduce the loss and error?
Hi Mossi…I see no issue with this approach, however it is difficult to predict whether this will in fact improve accuracy. Move forward with your suggested implementation and let us know your findings.
Hi
How we should plot the training and validation loss curve per epoch in this case?
Appreciate your help and comments
Hi Faraz…You may find the following of interest:
https://machinelearningmastery.com/learning-curves-for-diagnosing-machine-learning-model-performance/
HI,
I have written a code in R which checks different combinations of parameters to find the best model( I mean I check the different number of input nodes, dropout, batch size, …..). I have paralyzed it to speed up it. In R I used “mclapply” to paralyze and I have run it on a GPU.
Now I would like to write the exact code in python.
So Do you have topics related to my work? I mean parallelization, testing different parameters, and saving the best model, …..
Thanks in advance for any helps,
Hi Shamim…You may find the following resource of interest:
https://towardsdatascience.com/parallelization-w-multiprocessing-in-python-bd2fc234f516
Hi Jason,
I’m new to neural networks and I found your tutorial to be very helpful, thanks!
I’ve copied your code verbatim and observe similar minimized error.
Standardized: -21.88 (24.81) MSE,
and when I plot loss vs. epoch the error is definitely being minimized…
So, all is well up to here.
However, I’m always seeking “sanity checks” and when I plot the model predictions,
model.predict(X) overlaid with actual (Y)
I notice that the relative scale (and sign) for model.predict(X) and actual (Y) are completely different (in my example by factor -12X)…and this scale factor appears to fluctuate randomly per each execution of the code.
I remember that we had to standardze the data in the pre-processing…do we need to un-standarize it to use it in the model.predict(X)?
Or maybe I missed a line of code when I copied/paste?
Any suggestions you might have would be awesome.
Much Obliged,
-Kevin
Hi Kevin…The following resource should add clarity:
https://machinelearningmastery.com/how-to-improve-neural-network-stability-and-modeling-performance-with-data-scaling/
Hi James, I want to create an Angle Prediction model in which I will feed the inputs of pixel coordinates (x, y) and true angles. They should get trained and predict the angle. Is this possible using the regression model? Suggest your thoughts. I would be grateful.
Hi Arshad…It would be a regression model. Once trained on coordinates and angles, given new coordinates the model would be able to predict new angles. Not sure how practical or accurate this would be. Let us know what you find out!
Hi Jason, i am facing the error:
ValueError Traceback (most recent call last)
in ()
28 pipeline = Pipeline(estimators)
29 kfold = KFold(n_splits=10)
—> 30 results = cross_val_score(pipeline, X, Y, cv=kfold, scoring=’neg_mean_squared_error’)
31 print(“Standardized: %.2f (%.2f) MSE” % (results.mean(), results.std()))
2 frames
/usr/local/lib/python3.10/dist-packages/sklearn/model_selection/_validation.py in cross_val_score(estimator, X, y, groups, scoring, cv, n_jobs, verbose, fit_params, pre_dispatch, error_score)
513 scorer = check_scoring(estimator, scoring=scoring)
514
–> 515 cv_results = cross_validate(
516 estimator=estimator,
517 X=X,
/usr/local/lib/python3.10/dist-packages/sklearn/model_selection/_validation.py in cross_validate(estimator, X, y, groups, scoring, cv, n_jobs, verbose, fit_params, pre_dispatch, return_train_score, return_estimator, error_score)
283 )
284
–> 285 _warn_or_raise_about_fit_failures(results, error_score)
286
287 # For callabe scoring, the return type is only know after calling. If the
/usr/local/lib/python3.10/dist-packages/sklearn/model_selection/_validation.py in _warn_or_raise_about_fit_failures(results, error_score)
365 f”Below are more details about the failures:\n{fit_errors_summary}”
366 )
–> 367 raise ValueError(all_fits_failed_message)
368
369 else:
ValueError:
All the 10 fits failed.
It is very likely that your model is misconfigured.
You can try to debug the error by setting error_score=’raise’.
Below are more details about the failures:
——————————————————————————–
7 fits failed with the following error:
Traceback (most recent call last):
File “/usr/local/lib/python3.10/dist-packages/sklearn/model_selection/_validation.py”, line 686, in _fit_and_score
estimator.fit(X_train, y_train, **fit_params)
File “/usr/local/lib/python3.10/dist-packages/sklearn/pipeline.py”, line 401, in fit
Xt = self._fit(X, y, **fit_params_steps)
File “/usr/local/lib/python3.10/dist-packages/sklearn/pipeline.py”, line 359, in _fit
X, fitted_transformer = fit_transform_one_cached(
File “/usr/local/lib/python3.10/dist-packages/joblib/memory.py”, line 353, in __call__
return self.func(*args, **kwargs)
File “/usr/local/lib/python3.10/dist-packages/sklearn/pipeline.py”, line 893, in _fit_transform_one
res = transformer.fit_transform(X, y, **fit_params)
File “/usr/local/lib/python3.10/dist-packages/sklearn/utils/_set_output.py”, line 140, in wrapped
data_to_wrap = f(self, X, *args, **kwargs)
File “/usr/local/lib/python3.10/dist-packages/sklearn/base.py”, line 881, in fit_transform
return self.fit(X, y, **fit_params).transform(X)
File “/usr/local/lib/python3.10/dist-packages/sklearn/preprocessing/_data.py”, line 824, in fit
return self.partial_fit(X, y, sample_weight)
File “/usr/local/lib/python3.10/dist-packages/sklearn/preprocessing/_data.py”, line 861, in partial_fit
X = self._validate_data(
File “/usr/local/lib/python3.10/dist-packages/sklearn/base.py”, line 565, in _validate_data
X = check_array(X, input_name=”X”, **check_params)
File “/usr/local/lib/python3.10/dist-packages/sklearn/utils/validation.py”, line 940, in check_array
raise ValueError(
ValueError: Found array with 0 feature(s) (shape=(11670, 0)) while a minimum of 1 is required by StandardScaler.
——————————————————————————–
3 fits failed with the following error:
Traceback (most recent call last):
File “/usr/local/lib/python3.10/dist-packages/sklearn/model_selection/_validation.py”, line 686, in _fit_and_score
estimator.fit(X_train, y_train, **fit_params)
File “/usr/local/lib/python3.10/dist-packages/sklearn/pipeline.py”, line 401, in fit
Xt = self._fit(X, y, **fit_params_steps)
File “/usr/local/lib/python3.10/dist-packages/sklearn/pipeline.py”, line 359, in _fit
X, fitted_transformer = fit_transform_one_cached(
File “/usr/local/lib/python3.10/dist-packages/joblib/memory.py”, line 353, in __call__
return self.func(*args, **kwargs)
File “/usr/local/lib/python3.10/dist-packages/sklearn/pipeline.py”, line 893, in _fit_transform_one
res = transformer.fit_transform(X, y, **fit_params)
File “/usr/local/lib/python3.10/dist-packages/sklearn/utils/_set_output.py”, line 140, in wrapped
data_to_wrap = f(self, X, *args, **kwargs)
File “/usr/local/lib/python3.10/dist-packages/sklearn/base.py”, line 881, in fit_transform
return self.fit(X, y, **fit_params).transform(X)
File “/usr/local/lib/python3.10/dist-packages/sklearn/preprocessing/_data.py”, line 824, in fit
return self.partial_fit(X, y, sample_weight)
File “/usr/local/lib/python3.10/dist-packages/sklearn/preprocessing/_data.py”, line 861, in partial_fit
X = self._validate_data(
File “/usr/local/lib/python3.10/dist-packages/sklearn/base.py”, line 565, in _validate_data
X = check_array(X, input_name=”X”, **check_params)
File “/usr/local/lib/python3.10/dist-packages/sklearn/utils/validation.py”, line 940, in check_array
raise ValueError(
ValueError: Found array with 0 feature(s) (shape=(11671, 0)) while a minimum of 1 is required by StandardScaler.
can you help with this? thanks a lot!
Hello…What code are you referencing? That will better enable us to provide recommendations.
thanks for the reply
the code i used is almost same as above:
from pandas import read_csv
from tensorflow.keras.models import Sequential
from tensorflow.keras.layers import Dense
from scikeras.wrappers import KerasRegressor
from sklearn.model_selection import cross_val_score
from sklearn.model_selection import KFold
from sklearn.preprocessing import StandardScaler
from sklearn.pipeline import Pipeline
# load dataset
dataframe = read_csv(“train-v3.csv”, delim_whitespace=True, header=None)
dataset = dataframe.values
# split into input (X) and output (Y) variables
X = dataset[1:,5:23]
Y = dataset[1:,1:2]
# define base model
def baseline_model():
# create model
model = Sequential()
model.add(Dense(13, input_shape=(18,), kernel_initializer=’normal’, activation=’relu’))
model.add(Dense(1, kernel_initializer=’normal’))
# Compile model
model.compile(loss=’mean_squared_error’, optimizer=’adam’)
return model
# evaluate model with standardized dataset
estimators = []
estimators.append((‘standardize’, StandardScaler()))
estimators.append((‘mlp’, KerasRegressor(model=baseline_model, epochs=50, batch_size=5, verbose=0)))
pipeline = Pipeline(estimators)
kfold = KFold(n_splits=10)
results = cross_val_score(pipeline, X, Y, cv=kfold, scoring=’neg_mean_squared_error’)
print(“Standardized: %.2f (%.2f) MSE” % (results.mean(), results.std()))
with different .csv and X Y
(X = dataset[1:,5:23]
Y = dataset[1:,1:2])
by the way, i run it with colab