Machine learning algorithms cannot work with categorical data directly.
Categorical data must be converted to numbers.
This applies when you are working with a sequence classification type problem and plan on using deep learning methods such as Long Short-Term Memory recurrent neural networks.
In this tutorial, you will discover how to convert your input or output sequence data to a one hot encoding for use in sequence classification problems with deep learning in Python.
After completing this tutorial, you will know:
- What an integer encoding and one hot encoding are and why they are necessary in machine learning.
- How to calculate an integer encoding and one hot encoding by hand in Python.
- How to use the scikit-learn and Keras libraries to automatically encode your sequence data in Python.
Kick-start your project with my new book Long Short-Term Memory Networks With Python, including step-by-step tutorials and the Python source code files for all examples.
Let’s get started.
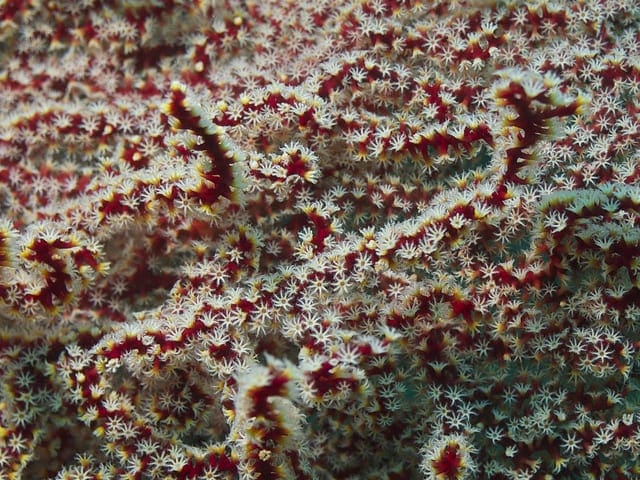
How to One Hot Encode Sequence Classification Data in Python
Photo by Elias Levy, some rights reserved.
Tutorial Overview
This tutorial is divided into 4 parts; they are:
- What is One Hot Encoding?
- Manual One Hot Encoding
- One Hot Encode with scikit-learn
- One Hot Encode with Keras
What is One Hot Encoding?
A one hot encoding is a representation of categorical variables as binary vectors.
This first requires that the categorical values be mapped to integer values.
Then, each integer value is represented as a binary vector that is all zero values except the index of the integer, which is marked with a 1.
Worked Example of a One Hot Encoding
Let’s make this concrete with a worked example.
Assume we have a sequence of labels with the values ‘red’ and ‘green’.
We can assign ‘red’ an integer value of 0 and ‘green’ the integer value of 1. As long as we always assign these numbers to these labels, this is called an integer encoding. Consistency is important so that we can invert the encoding later and get labels back from integer values, such as in the case of making a prediction.
Next, we can create a binary vector to represent each integer value. The vector will have a length of 2 for the 2 possible integer values.
The ‘red’ label encoded as a 0 will be represented with a binary vector [1, 0] where the zeroth index is marked with a value of 1. In turn, the ‘green’ label encoded as a 1 will be represented with a binary vector [0, 1] where the first index is marked with a value of 1.
If we had the sequence:
1 |
'red', 'red', 'green' |
We could represent it with the integer encoding:
1 |
0, 0, 1 |
And the one hot encoding of:
1 2 3 |
[1, 0] [1, 0] [0, 1] |
Why Use a One Hot Encoding?
A one hot encoding allows the representation of categorical data to be more expressive.
Many machine learning algorithms cannot work with categorical data directly. The categories must be converted into numbers. This is required for both input and output variables that are categorical.
We could use an integer encoding directly, rescaled where needed. This may work for problems where there is a natural ordinal relationship between the categories, and in turn the integer values, such as labels for temperature ‘cold’, warm’, and ‘hot’.
There may be problems when there is no ordinal relationship and allowing the representation to lean on any such relationship might be damaging to learning to solve the problem. An example might be the labels ‘dog’ and ‘cat’
In these cases, we would like to give the network more expressive power to learn a probability-like number for each possible label value. This can help in both making the problem easier for the network to model. When a one hot encoding is used for the output variable, it may offer a more nuanced set of predictions than a single label.
Need help with LSTMs for Sequence Prediction?
Take my free 7-day email course and discover 6 different LSTM architectures (with code).
Click to sign-up and also get a free PDF Ebook version of the course.
Manual One Hot Encoding
In this example, we will assume the case where we have an example string of characters of alphabet letters, but the example sequence does not cover all possible examples.
We will use the input sequence of the following characters:
1 |
hello world |
We will assume that the universe of all possible inputs is the complete alphabet of lower case characters, and space. We will therefore use this as an excuse to demonstrate how to roll our own one hot encoding.
The complete example is listed below.
1 2 3 4 5 6 7 8 9 10 11 12 13 14 15 16 17 18 19 20 21 22 |
from numpy import argmax # define input string data = 'hello world' print(data) # define universe of possible input values alphabet = 'abcdefghijklmnopqrstuvwxyz ' # define a mapping of chars to integers char_to_int = dict((c, i) for i, c in enumerate(alphabet)) int_to_char = dict((i, c) for i, c in enumerate(alphabet)) # integer encode input data integer_encoded = [char_to_int[char] for char in data] print(integer_encoded) # one hot encode onehot_encoded = list() for value in integer_encoded: letter = [0 for _ in range(len(alphabet))] letter[value] = 1 onehot_encoded.append(letter) print(onehot_encoded) # invert encoding inverted = int_to_char[argmax(onehot_encoded[0])] print(inverted) |
Running the example first prints the input string.
A mapping of all possible inputs is created from char values to integer values. This mapping is then used to encode the input string. We can see that the first letter in the input ‘h’ is encoded as 7, or the index 7 in the array of possible input values (alphabet).
The integer encoding is then converted to a one hot encoding. This is done one integer encoded character at a time. AÂ list of 0 values is created the length of the alphabet so that any expected character can be represented.
Next, the index of the specific character is marked with a 1. We can see that the first letter ‘h’ integer encoded as a 7 is represented by a binary vector with the length 27 and the 7th index marked with a 1.
Finally, we invert the encoding of the first letter and print the result. We do this by locating the index of in the binary vector with the largest value using the NumPy argmax() function and then using the integer value in a reverse lookup table of character values to integers.
Note: output was formatted for readability.
1 2 3 4 5 6 7 8 9 10 11 12 13 14 15 16 17 |
hello world [7, 4, 11, 11, 14, 26, 22, 14, 17, 11, 3] [[0, 0, 0, 0, 0, 0, 0, 1, 0, 0, 0, 0, 0, 0, 0, 0, 0, 0, 0, 0, 0, 0, 0, 0, 0, 0, 0], [0, 0, 0, 0, 1, 0, 0, 0, 0, 0, 0, 0, 0, 0, 0, 0, 0, 0, 0, 0, 0, 0, 0, 0, 0, 0, 0], [0, 0, 0, 0, 0, 0, 0, 0, 0, 0, 0, 1, 0, 0, 0, 0, 0, 0, 0, 0, 0, 0, 0, 0, 0, 0, 0], [0, 0, 0, 0, 0, 0, 0, 0, 0, 0, 0, 1, 0, 0, 0, 0, 0, 0, 0, 0, 0, 0, 0, 0, 0, 0, 0], [0, 0, 0, 0, 0, 0, 0, 0, 0, 0, 0, 0, 0, 0, 1, 0, 0, 0, 0, 0, 0, 0, 0, 0, 0, 0, 0], [0, 0, 0, 0, 0, 0, 0, 0, 0, 0, 0, 0, 0, 0, 0, 0, 0, 0, 0, 0, 0, 0, 0, 0, 0, 0, 1], [0, 0, 0, 0, 0, 0, 0, 0, 0, 0, 0, 0, 0, 0, 0, 0, 0, 0, 0, 0, 0, 0, 1, 0, 0, 0, 0], [0, 0, 0, 0, 0, 0, 0, 0, 0, 0, 0, 0, 0, 0, 1, 0, 0, 0, 0, 0, 0, 0, 0, 0, 0, 0, 0], [0, 0, 0, 0, 0, 0, 0, 0, 0, 0, 0, 0, 0, 0, 0, 0, 0, 1, 0, 0, 0, 0, 0, 0, 0, 0, 0], [0, 0, 0, 0, 0, 0, 0, 0, 0, 0, 0, 1, 0, 0, 0, 0, 0, 0, 0, 0, 0, 0, 0, 0, 0, 0, 0], [0, 0, 0, 1, 0, 0, 0, 0, 0, 0, 0, 0, 0, 0, 0, 0, 0, 0, 0, 0, 0, 0, 0, 0, 0, 0, 0]] h |
Now that we have seen how to roll our own one hot encoding from scratch, let’s see how we can use the scikit-learn library to perform this mapping automatically for cases where the input sequence fully captures the expected range of input values.
One Hot Encode with scikit-learn
In this example, we will assume the case where you have an output sequence of the following 3 labels:
1 2 3 |
"cold" "warm" "hot" |
An example sequence of 10 time steps may be:
1 |
cold, cold, warm, cold, hot, hot, warm, cold, warm, hot |
This would first require an integer encoding, such as 1, 2, 3. This would be followed by a one hot encoding of integers to a binary vector with 3 values, such as [1, 0, 0].
The sequence provides at least one example of every possible value in the sequence. Therefore we can use automatic methods to define the mapping of labels to integers and integers to binary vectors.
In this example, we will use the encoders from the scikit-learn library. Specifically, the LabelEncoder of creating an integer encoding of labels and the OneHotEncoder for creating a one hot encoding of integer encoded values.
The complete example is listed below.
1 2 3 4 5 6 7 8 9 10 11 12 13 14 15 16 17 18 19 20 |
from numpy import array from numpy import argmax from sklearn.preprocessing import LabelEncoder from sklearn.preprocessing import OneHotEncoder # define example data = ['cold', 'cold', 'warm', 'cold', 'hot', 'hot', 'warm', 'cold', 'warm', 'hot'] values = array(data) print(values) # integer encode label_encoder = LabelEncoder() integer_encoded = label_encoder.fit_transform(values) print(integer_encoded) # binary encode onehot_encoder = OneHotEncoder(sparse=False) integer_encoded = integer_encoded.reshape(len(integer_encoded), 1) onehot_encoded = onehot_encoder.fit_transform(integer_encoded) print(onehot_encoded) # invert first example inverted = label_encoder.inverse_transform([argmax(onehot_encoded[0, :])]) print(inverted) |
Running the example first prints the sequence of labels. This is followed by the integer encoding of the labels and finally the one hot encoding.
The training data contained the set of all possible examples so we could rely on the integer and one hot encoding transforms to create a complete mapping of labels to encodings.
By default, the OneHotEncoder class will return a more efficient sparse encoding. This may not be suitable for some applications, such as use with the Keras deep learning library. In this case, we disabled the sparse return type by setting the sparse=False argument.
If we receive a prediction in this 3-value one hot encoding, we can easily invert the transform back to the original label.
First, we can use the argmax() NumPy function to locate the index of the column with the largest value. This can then be fed to the LabelEncoder to calculate an inverse transform back to a text label.
This is demonstrated at the end of the example with the inverse transform of the first one hot encoded example back to the label value ‘cold’.
Again, note that input was formatted for readability.
1 2 3 4 5 6 7 8 9 10 11 12 13 14 15 16 |
['cold' 'cold' 'warm' 'cold' 'hot' 'hot' 'warm' 'cold' 'warm' 'hot'] [0 0 2 0 1 1 2 0 2 1] [[ 1. 0. 0.] [ 1. 0. 0.] [ 0. 0. 1.] [ 1. 0. 0.] [ 0. 1. 0.] [ 0. 1. 0.] [ 0. 0. 1.] [ 1. 0. 0.] [ 0. 0. 1.] [ 0. 1. 0.]] ['cold'] |
In the next example, we look at how we can directly one hot encode a sequence of integer values.
One Hot Encode with Keras
You may have a sequence that is already integer encoded.
You could work with the integers directly, after some scaling. Alternately, you can one hot encode the integers directly. This is important to consider if the integers do not have a real ordinal relationship and are really just placeholders for labels.
The Keras library offers a function called to_categorical() that you can use to one hot encode integer data.
In this example, we have 4 integer values [0, 1, 2, 3] and we have the input sequence of the following 10 numbers:
1 |
data = [1, 3, 2, 0, 3, 2, 2, 1, 0, 1] |
The sequence has an example of all known values so we can use the to_categorical() function directly. Alternately, if the sequence was 0-based (started at 0) and was not representative of all possible values, we could specify the num_classes argument to_categorical(num_classes=4).
A complete example of this function is listed below.
1 2 3 4 5 6 7 8 9 10 11 12 13 |
from numpy import array from numpy import argmax from keras.utils import to_categorical # define example data = [1, 3, 2, 0, 3, 2, 2, 1, 0, 1] data = array(data) print(data) # one hot encode encoded = to_categorical(data) print(encoded) # invert encoding inverted = argmax(encoded[0]) print(inverted) |
Running the example first defines and prints the input sequence.
The integers are then encoded as binary vectors and printed. We can see that the first integer value 1 is encoded as [0, 1, 0, 0] just like we would expect.
We then invert the encoding by using the NumPy argmax() function on the first value in the sequence that returns the expected value 1 for the first integer.
1 2 3 4 5 6 7 8 9 10 11 12 13 14 |
[1 3 2 0 3 2 2 1 0 1] [[ 0. 1. 0. 0.] [ 0. 0. 0. 1.] [ 0. 0. 1. 0.] [ 1. 0. 0. 0.] [ 0. 0. 0. 1.] [ 0. 0. 1. 0.] [ 0. 0. 1. 0.] [ 0. 1. 0. 0.] [ 1. 0. 0. 0.] [ 0. 1. 0. 0.]] 1 |
Further Reading
This section lists some resources for further reading.
- What is one hot encoding and when is it used in data science? on Quora
- OneHotEncoder scikit-learn API documentation
- LabelEncoder scikit-learn API documentation
- to_categorical Keras API documentation
- Data Preparation for Gradient Boosting with XGBoost in Python
- Multi-Class Classification Tutorial with the Keras Deep Learning Library
Summary
In this tutorial, you discovered how to encode your categorical sequence data for deep learning using a one hot encoding in Python.
Specifically, you learned:
- What integer encoding and one hot encoding are and why they are necessary in machine learning.
- How to calculate an integer encoding and one hot encoding by hand in Python.
- How to use the scikit-learn and Keras libraries to automatically encode your sequence data in Python.
Do you have any questions about preparing your sequence data?
Ask your questions in the comments and I will do my best to answer.
Thank you for this post! It came really timely. A question. Utility to_categorical(data) accepts vector as input. What would one do in case you have a 2D tensor as input? Would you loop over number of samples (could be several hundred of thousands entries + do the model training in a loop) or one would have to do seq = tokenizer.texts_to_sequences(inputseq) and then tokenizer.sequences_to_matrix(seq, mode=’binary’)?? The last option will give a 2D tensor as output in form array([ 0., 1., 1., …, 0., 0., 0.]). In that case model training goes as usual, but for decoding predictions one would have to loop to find all maximums (argmax give ONLY the first maximum).
Ouch, it is hard to give good advice without specifics. Choose a formulation that preserves the structure of your sequence.
when will you do padding.? before or after sequences_to_matrix?
Try both and see what works best for your specific dataset.
how do I convert a one hot encoded dataset to a label encoded. ie from multicolumn to two column
Awesome post again Jason! Thank you. I’ve just added it to my ML checklist.
Excellent Franco!
how do we perform integer encoding in keras ? Is there any inbuild function like LabelEncoder in scikit ?
Not really. You can do it manually.
If you’re working with text, there are tools here:
https://keras.io/preprocessing/text/
Hi Jason,
Great article!
What if the sequence was not representative of all possible values, and we don’t know the num of classes to set the num_classes argument?
Thanks.
Yes, that is a challenge.
Off the cuff, you may need to re-encode data in the future. Or choose an encoding that has “space” for new values that you have not seen yet. I expect there is some good research on this topic.
Informative post as always!
I understand how this is used to train a model.
However, how can you ensure that data you want to get predictions for is encoded in the same way as the training data? E.g. ‘hot’ maps to column 3.
For my application once the model is trained it will need to provide predictions at a later date and on different machines.
Great question!
It must be consistent. Either you use the same code, and/or you save the “model” that performs the transform.
Thank u!!!!!! great work……….
Thanks.
Hey there,
Great tutorial as always!
Quick question though – what if my dataset contains both categorical and continuous values? Wouldn’t OH encoding encode the entire dataset, when all I really need is just the categorical columns encoded?
Also would the recommended flow be to (a) scale the numeric data only (b) encode the whole dataset?
Or (a) encode the whole dataset and then (b) scale all values?
I would recommend only encoding the categorical variables.
podi naayae
Nice post!
Hi there,
I am working on model productization. To reduce the data processing gap between experiment and production, would like to embed one hot encoding in Keras model. The questions are
1. When using “to_categorical”, it will convert categorical on the fly and it seems break the encoding. For example, we have “apple”, “orange” and “banana” when training model. After rollout the model, there include unseen category such as “lemon”. How can we handle this kind of scenario?
2. As want to embed the encoding in Keras. Therefore, trying to not using sklearn label encoder and one hot encoder. Does it possible to do that?
Please kindly advise.
Perhaps you can develop your own small function to perform the encoding and perform it consistently?
Hi Jason,
Love the website! Had a quick question:
If I have a X dataset of 6 categorical attributes, where each attribute has lets say the following number of unique categories respectively [4, 4, 4, 3, 3, 3]. Also lets say there are 600 instances
When I LabelEncode and then OneHotEncode this X dataset, I now rightly get a sequence of 21 0-1 values after the toarray() method on the fit_transform.
However, when I input the X dataset like that, i.e. with a shape of (600, 21), I get a much worse error than if I had just left it LabelEncoded and with a shape of (600, 6).
My question is am I doing something wrong? Should I be re-grouping the sequence of 21 integers that I get back into their respective clusters? For ex: I get this array for the first row as a result of the OneHotEncode:
[0, 0, 0, 1, 0, 0, 0, 0, 1, 0, 0, 0, 1, 0, 0, 1, 0, 0, 1, 0, 1, 0]
so should I group the digits back into something like this
[(0, 0, 0, 1), (0, 0, 0, 1), (0, 0, 0, 1), (0, 0, 1), (0, 0, 1), (0, 1, 0)]
where now we again have a (600, 6) shape for my input dataset?
I tried creating a numpy array with this formulation but the sci-kit decision tree classifier checks and tries to convert any numpy array where the dtype is an object, and thus the tuples did not validate.
Essentially, I want to know whether the (600, 21) shape is causing any data loss being in that format. And if it is what is the best way to regroup the encodings into their respective attributes so I can lower my error.
Thank you!
Hmmm.
The data should be 500, 21 after the encoding, so far so good.
No need to group.
Skill better or worse depends on the algorithm and your specific data. Everything is a test to help us discover what works for our problem.
Consider trying more algorithms.
Consider trying encoding only some variables and leaving others as-is or integer encode.
Brainstorm more things to try, see this post for ideas:
https://machinelearningmastery.com/machine-learning-performance-improvement-cheat-sheet/
I hope that helps as a start.
Okay, great! I just wasn’t sure whether to debug the onehot encoder or try other things. Looks like trying other things is the way to go.
Thanks you so much for your help Jason!
Hii,
Great tutorial as always!
I am having a doubt…Why we are reshaping the integer_encoded vector
“integer_encoded = integer_encoded.reshape(len(integer_encoded), 1)”?
Great question, because the sklearn tools expect 2D data as input.
Hello,
Thanks a lot for your great posts!
There are some studies using only the index of the words without turning them into one-hot,
such as:
[7, 4, 11, 11, 14, 26, 22, 14, 17, 11, 3]
they just use this sequence as input. I’d like to ask that since we generate indexes starting from 0, wouldn’t it be a problem when we’re doing 0-padding?
Thanks a lot!
The 0 is often reserved for “no word” or “unknown word”.
Ok, but in one-hot form, why didn’t we reserve 0 position for unknown word?
This happens automatically when using libraries such as Keras to perform the encoding. We can also do that manually.
I’m doing it manually, at some point I became suspicious whether I was doing wrong, this is how I create char-index dictionaries.
ch_ind = dict((c, i+1) for i, c in enumerate(s_chars))
ind_ch = dict((i+1, c) for i, c in enumerate(s_chars))
then this is how I create my one hot encoding,
X = np.zeros((MAX_LEN, len(ch_ind)))
for i, ch in enumerate(line[:MAX_LEN]):
X[i, (ch_ind[ch])] = 1
return X
This means that I won’t have the following encoding, since I dont have a char at point 0,
[1 0 0 0 …]
But does this really achieve saving point 0 for padding?
Thanks a lot for your time!
Hi Jason,
What if we have categorical variables with levels more than 500? How to deal with this, Is it OHE? Which will in return gives high number columns.
Try it and see.
how to proceed when all input data are categories?
You can use a label encoder and perhaps even a one hot encoder on all of them.
Hello Dr.Jason,
I have a CSV dataset where some of the values are floating point values while the rest are labels. If i use one-hot encoding for the labels, they will be converted into binary vectors. However, the other values are floating.
I want to train a classifier on this data. Is it correct to train a classifier using a dataset with combination of binary vectors and floating point values? Shouldn’t all the parameters in the dataset should be of the same data-type?
Yes, train on the combined original and encoded variables.
Hi Jason,
your articles are an excellent source for newbies like me! Thank you indeed for putting so much energy into it, Sir!
I found that pandas.Dataframe has a nice method to do one-hot encoding as well by using get_dummies which is very handy ( https://pandas.pydata.org/pandas-docs/stable/generated/pandas.get_dummies.html ).
Thanks.
I have a ? in hypothroid dataset , how do i handle this type of stuff. pls let me know your good solution
This process will help you work through your predictive modeling problem:
https://machinelearningmastery.com/start-here/#process
Dear jason,
Once we use one_hot_encoder to represent categorical attributes that take a large number of values ,we can end up having a very large matrix. Could you please explain the idea behind “Embeddings” in Neural networks to overcome this issue?
Regards.
Sure, see these posts:
https://machinelearningmastery.com/?s=word+embedding&submit=Search
Hi Jason,
Great post as always. Since categorical(non-numeric) data in most cases has to be One Hot Encoded, just wondering why there is no direct method which takes categorical data and returns a one-hot-encoded data set directly instead of the user always having to call label Encoder to get the data integer encoded first?
Sometimes there is benefit on working with the integer encoded values instead.
Hi Jason,
I appreciate the clear tutorial u have written, especially for a novice like me.
I want to gain a probability output instead of the label of class as an output.
I know I should apply “”model.add(Dense(2, activation=’sigmoid’))”” instead of applying “”model.add(Dense(1, activation=’sigmoid’))”” and also i should use commands which in “One Hot Encode with Keras”.
But I doubt whether I should put class labels in data = [1, 3, 2, 0, 3, 2, 2, 1, 0, 1] or not. I mean instead of data = [1, 3, 2, 0, 3, 2, 2, 1, 0, 1] should I write data =[0,1]. I have a binary classification problem for text dataset.
would u mind please answering me by a tutorial link or even by writing command?
Waiting for your replying.
You can make probability predictions by calling predict_proba() on your model.
For a binary outcome, you can use a single node in the output layer and the outcome will be a 0 or 1, not a one hot encoded class.
Hi dear Jason,
I appreciate the time you spend replying me.
I do not want to achieve 0,1 outcome. I want to achieve possible output such as 90% for class “good”, 10% for class “bad”.
I do not want to gain neither the label as output nor f-measure or accuracy.
I want to gain similarity measure = probability measure for lstm, cnn, rnn. I should write k fold cross-validation to build a model which can achieve similarity measure for each label.
Please give me a tutorial to write k fold-cross validation to building a model to achieving possible output by a similarity measure.
“””If I want to mention briefly, I want to read about how I can write k-fold cross validation commands and also i want to know what the function of similarity measure is in Keras?”””
Thank u for helping a novice so clear.
Best wishes
This is a probability outcome. You can achieve this with a binary outcome bu calling predict() and using the value and 1 – value to get the probabilities for class 1 and class 0 respectively.
Cross validation is only used to estimate the skill of the model. After you have an estimate of model skill, the CV folds and models are discarded and you can fit a final model to be used to make predictions. Learn more about this here:
https://machinelearningmastery.com/train-final-machine-learning-model/
Hi Jason,
Thank you for spending your time for replying.
Hoping to solve the question.
Best wishes.
Maryam
Jason,
Great post. You’re making me into a machine learning ninja! Do you think one-hot encoding is a good choice for ordinal data like cold,warm,hot? That is by encoding into separate columns don’t you lose the relationship that those three are on a continiuum. I thought the general approach to data preparation is to expose my knowledge of each variable to the machine learning algorithm. It seems that one-hot encoding obfuscates it in this case. Do you think leaving those three as integers might be a better choice? What would you recommend?
thanks,
Tim
It can make the relationship simpler for an algorithm, vectorized rather than compound.
In general, test and demonstrate the change improves model skill.
Hi Jason, great tutorial! Do you know how I would go about combining multiple one-hot vectors of different lengths? Let’s say that in addition to each letter’s one-hot coding I also have other categories such as gender and country.
Also, what if I need to combine these with an integer such as age?
Cheers! 🙂
If I follow, you could have other variables “next to” the one hot encoded inputs to form a very long input vector.
what great work and makes life easier approach on ML
Hi Jason, recently, iam working with a data that has 921179 rows and about 32 columns. out of the 32 columns, the 22 are Object types and i was trying to encode the dataset using label encoder and oneHotEncoder.
1, each columns has at least 20 unique values. there will be hounders of categories together.
2, is it a right way to encode with labelEncoder and then with one hot Encoder to such a big data.
or if you can suggest me an easier approach.
tnx inadvance!
Perhaps try a suite of approaches and evaluate them based on their impact on model skill.
– try integer encoding.
– try one hot encoding
– try removing them
– try grouping labels and then encoding.
– …
Let me know how you go.
thanks for the quick response!
– i imported the dataset using pandas : df = pd.read_csv(‘name.csv’)
– then i selected only object categories : df = df.select_dtypes(include=[‘object’])
-label encoding df1.apply(LabelEncoder().fit_transform) – worked fine till this point.
– then i tried to create dummy variables , this is where it went wrong
– df.apply(OneHotEncoder(categorical_features=’all’).fit_transform) – i got error message like this
File “”, line 1, in
X = one.fit_transform(X1)
File “/Users/afoto/anaconda2/lib/python2.7/site-packages/sklearn/preprocessing/data.py”, line 2019, in fit_transform
self.categorical_features, copy=True)
File “/Users/afoto/anaconda2/lib/python2.7/site-packages/sklearn/preprocessing/data.py”, line 1809, in _transform_selected
X = check_array(X, accept_sparse=’csc’, copy=copy, dtype=FLOAT_DTYPES)
File “/Users/afoto/anaconda2/lib/python2.7/site-packages/sklearn/utils/validation.py”, line 433, in check_array
array = np.array(array, dtype=dtype, order=order, copy=copy)
ValueError: could not convert string to float: no
Perhaps confirm that you were applying the one hot encoding on the integer encoded var and not the original string variable?
thanks for a response.
lately, i sow one tutorial on handling many categorical attributes. the approach is simple as follows;
1, iterate through all attributes with object data type object and then identify the unique categories
2, if the number of categories is more than 15 or 20, for instance a feature called nationality and we found out that the majority is 4000 = Americans, 200 europeans , 10 Africans and 50 Indians, 2 Chinese and etc.
3, we create a dummy variables for the Americans and Europeans and other( where the other is the remaining counties)
this approach i found it to be intuitive and easier to implement.
looking forward for your invaluable comments and feedbacks.
Thanks and Cheers!
Yes, I like this approach.
Hello can you share with me the link that allowed you to implement it because I have the same problem with my project
Hey Jason,
It was an excellent article with exhaustive coverage. However, I have one doubt. I used the following code to use one hot encode for some categorical variables, but, the model fit throws error after successfully using one hot encoding. There is no error if I use ordinal encoding. Here is the code:
#ONE HOT ENCODING
from sklearn.preprocessing import OneHotEncoder
def one_hot_encode_features(df_train,df_test):
features = [‘Fare’, ‘Cabin’, ‘Age’, ‘Sex’]
#features = [ ‘Cabin’, ‘Sex’]
df_combined = pd.concat([df_train[features], df_test[features]])
for feature in features:
le = preprocessing.LabelEncoder()
onehot_encoder = OneHotEncoder()
le = le.fit(df_combined[feature])
integer_encoding_train=le.transform(df_train[feature])
integer_encoding_test=le.transform(df_test[feature])
integer_encoding_train = integer_encoding_train.reshape(len(integer_encoding_train), 1)
integer_encoding_test = integer_encoding_test.reshape(len(integer_encoding_test), 1)
df_train[feature] = onehot_encoder.fit_transform(integer_encoding_train)
df_test[feature] = onehot_encoder.fit_transform(integer_encoding_test)
return df_train, df_test
data_train, data_test = one_hot_encode_features(data_train, data_test)
#FITTING THE MODEL:
from sklearn.naive_bayes import GaussianNB
from sklearn.metrics import make_scorer, accuracy_score
from sklearn.model_selection import GridSearchCV
clf = GaussianNB()
acc_scorer = make_scorer(accuracy_score)
clf.fit(X_train, Y_train)
Sorry, I cannot debug your code.
Perhaps try posting your code to the developers on stackoverflow?
Hey Jason,
Here is a question not related to code debugging, I received a one hot encoded sparse matrix after following the steps in this article. However, I am finding trouble to add it to my training dataframe.
f_train[feature] = onehot_encoder.fit_transform(integer_encoding_train) fills all the n rows with the same values. How can this be achieved correctly?
Great question, perhaps an hstack() might help.
This post will help:
https://machinelearningmastery.com/gentle-introduction-n-dimensional-arrays-python-numpy/
Okay, thanks Jason
is this method is advice able for large number of categorical variable say i have 1500 categories for one variable is it advisable to use one-hot encoding
Yes, it may be ok.
I also list some additional approaches here:
https://machinelearningmastery.com/faq/single-faq/how-do-i-handle-a-large-number-of-categories
Hi Jason, first I integer encoded the classes then converted that to one hot encoding. during prediction, I used reverse and got the class. Now I also want the confidence of the class. I am using keras and on using predict_prob I get ‘Model’ object has no attribute ‘predict_proba’ as I am not using sequential. any suggestions how to get the confidence of class?
If you are using softmax or sigmoid as the activation functions on the output layer, you can use the values directly as probability-like values.
when we use one hot encoding with sklearn, how do we check if the code is free of dummy trap.
what i meant is that if i have may features with numeric categorical values like feature x1: 3,2,1,5,3,4,2
and feature x2: 1,2,3,2,1,3 and so on
if i use one hot encoding all the categories in one go
oneHot =OneHotEncode(category_feature=[the number of to be encoded] -> example feature 1,2,4
the feature x1: have 4 categories and after one hot do we get 4 new features or 3 features. in get dummy we get 3, thus there is no dummy trap, hoe about in one hot encoder
You get n elements in the binary vector where n is the number of unique categories.
how can we use one hot encoding for next word prediction is there is post for doing this?
thank you
One hot encoding is an encoding scheme, not a prediction scheme.
You must develop a model to make predictions. I recommend looking at language models:
https://machinelearningmastery.com/?s=language+model&post_type=post&submit=Search
I have a small issue concerning using the onehot encoding. I have a list of tokens and this list contains string, numbers and even special characters like this ”. I have done one hot encoding to this list, fed it into autoencoder model. Then, I fed to the model an unseen one hot encoded list. Then, the output from the autoencoder model is fed to inverse one hot encoding function. The generated list is not even close to the unseen list although the accuracy of my model is 0.9. Do you have any idea what is the problem ? I have used the one hot encoding functions from this tutorial.
Sounds like a bug in your implementation. I have some ideas here:
– Consider aggressively cutting the code back to the minimum required. This will help you isolate the problem and focus on it.
– Consider cutting the problem back to just one or a few simple examples.
– Consider finding other similar code examples that do work and slowly modify them to meet your needs. This might expose your misstep.
– Consider posting your question and code to StackOverflow.
how to deal with new category of a feature example
feature x has this categories while building model
2001
2002
2003
while using the model for prediction say i get 2004 as a value for that feature how do i deal with this using one hot encoder ???? also the sci-kit learn method for the same would be helpful
You must plan the encoding to support new categories in the future.
If the problem definition changes, you may need to ignore the change or rebuild the representation and model. Often the latter is cheap.
I have learned from your posts severally, and I wanted to thank you for taking the time to explain these concepts. It is really helpful for those of us starting up with machine learning. I am so grateful!
Thanks, I’m glad the posts help.
I have a column in pandas dataframe that contains thousands of unique character data. How to perform one hot encoding on this ? Actually I have 40 columns. Doing so will add 1000 extra columns.
1000 columns is not a lot, we may have tens or hundreds of thousands of columns in NLP problems.
Is there an alternative for one hot encoding ?
Yes, integer encoding or a word embedding.
Nice post!
Thanks.
Hi! I really liked your code (it helped me a lot!) and I have built upon it. This is a function which converts the given column to type category and correctly names the output columns. I thought I’d share it here:
Thanks for sharing!
Can we encode texts or xm elements fields to arrays not a single value (integer)
You can encode the words as number or letters as numbers. The former is more common and useful.
Hi Jason,
That is a nice post, thanks. Have you used one hot encoding as an input to a multiple linear regression and looked at the resulting coefficients? They are not what you might expect, but I can’t find a source where this is discussed. I wonder if you have come across anything on this?
If we take a random binary matrix with n rows and p columns representing p variables over n examples and a vector w of coefficients, then generate y=Xw we produce a data set of inputs X and outputs y. If we then use multiple linear regression to estimate w (which we already know, in this example as we used it to generate y), we get the values from w that we started with. This is true for almost any values in X where n>p EXCEPT where X is a representation of one hot encodings. In that particular case, the estimates of the coefficients in w are not the same as those used to generate y. Have you come across this phenomenon? I suspect it is because each coefficient is under constrained but I have never seen it discussed. It has interesting consequences for the interpretation of models built from on hot encoded variables, I think.
Linear regression is not great with encoded categorical vars. Perhaps try other methods, perhaps a decision tree?
Hi Jason, firstly thanks for the beautiful step-by-step explanation. I have a quick question (I’m a beginner to scikit-learn and ML as a whole):
1. I selected some input features list [X]
2. I found out the missing values in specific columns
3. For one of the columns that has missing values, let’s say the categories are [‘Fa’, ‘Gd’, ‘Ex’, ‘TA’, Nan]
4. I have done Number and a Binary (one-hot) encoding to get this:
Missing values: [nan, ‘Fa’, ‘TA’, ‘Ex’, ‘Gd’]
Number encoding: [4 1 3 0 2]
Binary/one-hot encoding:
[[0. 0. 0. 0. 1.]
[0. 1. 0. 0. 0.]
[0. 0. 0. 1. 0.]
[1. 0. 0. 0. 0.]
[0. 0. 1. 0. 0.]]
5. Now, HOW DO I FILL THESE VALUES BACK TO THE COLUMN FROM WHERE THEY WERE MISSING? Do I need to work on Imputation? Or create a new data frame?
Your help is much appreciated, and thanks in advance..
You drop the original column and concatenate the new columns with your remaining data.
Does that help?
That helps, thanks Jason. One other question would be this:
Say I have some columns with missing values and are categorical, like: {nan, ‘Gd’, ‘TA’, ‘Fa’, ‘Ex’}. Now I do the following:
1. Drop the column from original X dataframe
2. Do a number encoding on the categorical data: [4 2 3 1 0]
3. Next, I do a Binary One-hot encoding on these:[[0. 0. 0. 0. 1.]
[0. 0. 1. 0. 0.]
[0. 0. 0. 1. 0.]
[0. 1. 0. 0. 0.]
[1. 0. 0. 0. 0.]]
4. Now, my question here is, I have some numbers to play with in a column. Do I need to do any kind of imputation with (strategy=mean/median/most_frequent), or now directly I can add these columns with the number values to original dataframe and start my model?
Great question.
You can impute the missing values before hand as categorical values.
You can treat the nan as its own value.
You can delete those rows.
…
Try a few approaches and see which results in the best model performance.
Cool..thanks again Jason.. And, sorry about my dumb questions as I’m just 2 weeks old to Machine Learning..
> I have two data sets (train.csv and test.csv).
> It is evident that I should fit and train my model on “train”ing data and do the prediction with “test”ing data (validation)
>this is my question here:
when I do a split of test and validation data, it is very easy to do when we have one single dataframe:
train_X, val_X, train_y, val_y = [X, y, random_state=0]
> Now, when I have two dataframes – train.csv and test.csv, how do I address it? Like how do I split the data appropriately here
Train is split into train and validation.
You can learn more here:
https://machinelearningmastery.com/difference-test-validation-datasets/
Thank you for this post.
I am using your code to transform a list of DNA sequences that have the following structure: [[a,b,c,d,e],[f,g,h,i,j],[k,l,m,n]]. It is basically a list of lists containing letters.
I used the np.array function on my list of lists, but the fit_transform gave me an error of shape.
What do you suggest?
I’m not sure what you’re trying to do exactly.
Hello, Thank you for this great post.
I used your code to do a label encoder and one hot encoder, but when I call the inverse_transform I get this error:
inverted = label_encoder.inverse_transform([argmax(data_oh[0, :])])
File “…\Programs\Python\Python36\lib\site-packages\sklearn\preprocessing\label.py”, line 283, in inverse_transform
return self.classes_[y]
TypeError: only integer scalar arrays can be converted to a scalar index
Do you know what can be the problem?
My code is the following:
#integer encode
label_encoder_dict = defaultdict(LabelEncoder) #retain all columns LabelEncoder as dictionary.
integer_encoded = data_cat.apply(lambda x: label_encoder_dict[x.name].fit_transform(x))
#binary encode
oh = OneHotEncoder(handle_unknown=’ignore’, sparse=False)
integer_encoded = integer_encoded.as_matrix(integer_encoded.columns)
data_oh = self.oh.fit_transform(integer_encoded)
label_encoder = self.label_encoder_dict[‘DEST’]
inverted = label_encoder.inverse_transform([argmax(data_oh[0, :])])
Thank you!
I have not seen this before.
One tip is that the inverse transform expects the data to have the same shape and form as is provided by the transform() function.
Thanks for the great work. I have a dataset that has this structure:
down vote
unaccept
I reduced your dataset file to:
A
B
C
D
E
F
and I extracted the data from this dataset by the below code.
import re
with open (“test_dataset.log”, “r”) as myfile:
read_dataset = myfile.read()
i_ident = []
j_atr = []
find_ident = re.findall(r'(.*?)’, read_dataset, re.S)
ident_list = list(map(lambda x: x.replace(‘\n’, ‘ ‘), find_ident))
for i in range(len(ident_list)):
i_ident.append(str(ident_list[i]))
find_atr = re.findall(r'(.*?)’, read_dataset, re.S)
atr_list = list(map(lambda x: x.replace(‘\n’, ‘ ‘), find_atr))
#print(coref_list)
for i in range(len(atr_list)):
j_atr.append(str(atr_list[i]))
print(i_ident)
print()
print(j_atr)
Where I have stored the values of these data in i and j variable. I want to do the coreference resolution in the decision tree. For doing this task I have defined some functions like the following:
distance_feature(): distance between i and j according to the number of sentences. output: 0 or 1
Ispronoun_feature(): this feature is set to true if a noun phrase is a pronoun.
appositive_feature(): This feature checks if j is in apposition of i.
and more it is about 12 features that I have extracted. Now How can build my tree, if I want to change the data to one-hot encoding, you see the dataset structure which all unstructured. then in sci-kit learn how do I include all these functions to decide if i and j are coreferent. Please let me know if you have some ideas.
Thank you
Sorry, I don’t have the capacity to debug your code, I have some suggestions here:
https://machinelearningmastery.com/faq/single-faq/can-you-read-review-or-debug-my-code
Hello Jason,
I am using to_categorical of Keras and above u specifically said that when sequence starts with 0, but what if I want to use it on a sequence that starts with say 1. then how do we approach it?
I tried it when it always adds an extra column of 0’s in final encoding which is not good and I also tried num_classes argument but I am getting “IndexError” in it.
Why start at 1?
Hi Jason,
This is a great post. Label encoder will throw an error for unseen values in the test set and hence not able to proceed with One hot Encoding. How to tackle this problem in production systems ?
Ensure it is trained on data that contains all possible cases, e.g. is representative.
Hi Dr.jason,
Currently I want to do a feature selection over my dataset. My dataset contain 20 features which 7 of them are categorical and the rest are continuous features. However I found out that not all feature selection technique applicable for mixed (categorical+ continuous) dataset for example like PCA. After I read about one-hot-encoding, I feel like want to use it to transform all the categorical features into continuous features which mean to standardize the type all the features. After encoding, I will use PCA to reduce the data dimension. Is it a good idea?
Probably not. Perhaps work with all categorical variables separately then all numeric?
Hello Jason, great article.
I’ve got some categorical nominal data that I need to apply some type of dimension reduction technique to. What would be the difference in applying one-hot encoding and running PCA as opposed to applying Multiple Correspondence Analysis (MCA)? I’d imagine you would get similar results
A one hot encoding is a distributed representation, a PCA (and choosing the most relevant components) will remove linear dependences between inputs.
Hello Jason,
First of all, thanks for the article, it’s been really helpful.
Second: I have a few questions, I am, right now, working on an API that takes data from a survey and performs multiple linear regression analysis on it, this data could contain numerical and categorical questions, with that in mind:
– Say I already have a way of integer-encoding my categorical data, and my numerical data already comes in integers, would you say it’s more convenient to one-hot-encode any part of it?
– How would you one-hot encode a series of ranking style answers? Say, every respondent to the survey has to rank cats, dogs and hamsters as their favorite pets, giving answers like:
A1: cat, hamster, dog.
A2: dog, cat, hamster.
A3: hamster, dog, cat.
Thanks in advance.
I recommend try modeling with integer and one hot encoded and compare model skill to see if it makes a difference.
Free form text (words) can be integer encoded. Documents (lines or fields of text) can also be encoded as a binary vector called a bag of words:
https://machinelearningmastery.com/gentle-introduction-bag-words-model/
Hi
Many thanks to your incredible tutorial. I’m wondering that can i use 1 hot encoding form of the prediction to calculate some metrics like Accuracy, IoU, F1 score or i must transform in back to the reverse 1 hot encoding form to do it since this relates to the actual negative and actual positive of the Confusion Matrix (e.g in 1hot form: [0,0,1,0] and rev1hot: [2])
The one hot encoding is only used for modeling.
After the model has made a prediction, you can covert it back into a class integer using argmax(), then calculate accuracy.
Hi Jason,
I am working on a problem which has column of sequences and another column with value. The data is like:
Sequence CV
AAAAGHKLYH 0.5
AGLMcKAD 0.7
WMGKAAASFAAKm 0.56
I wanted to encode this data to numerical form and try some neural networks on it to predict CV, I didn’t get how to decode the sequence, the max length of sequence is 55.
If you one hot encode each char in the input sequence, there is no need to decode, but you can by using the argmax() function to get an integer and map the integer back to a char.
Thanks for the response.
Sorry, My question was wrong, I didn’t get how to input my sequence column to the encoder, I tried giving the the column as input but got Memory Error and tried giving it as numpy array still got the same error.
Hi Jason,
thank you for your useful pointers. It appears that the scikit-learn OneHotEncoder is capable of handling string labels directly without going through the LabelEncoder as above. Are there reasons for using the two step approach nonetheless?
Some sample code to illustrate one hot encoding of labels for string labeled data:
from sklearn.preprocessing import OneHotEncoder
# Create a one hot encoder and set it up with the categories from the data
ohe = OneHotEncoder(dtype=’int8′,sparse=False)
taxa_labels = np.unique(taxa[:,1])
ohe.fit(taxa_labels.reshape(-1,1))
# Create a categorical list of targets for each sample
y = ohe.transform(taxa[:,1].reshape(-1,1))
# Get string label from one hot encoder, might be useful later
labels= ohe.inverse_transform(y)
Nice tip, thanks!
Perhaps that is a new feature?
This seems to have been added from sklearn 0.20.3.
Still, it’s good to know of both these approaches. I was recently running test on an out of the box Deep Learning image provided by Google Compute Engine, and some awkward dependencies made it difficult to upgrade sklearn from the default version. I had to rewrite some of my code for this exact reason to be backwards compatible to this older version.
Thanks for letting me know.
This is super clear, thank you!
Per the sklearn-onehotencoder, can you place that resulting list in a pandas data frame?
Yes.
Thanks Jason! An extremely clear tutorial. I had one question – suppose we are not dealing with sequence data – say a dataset with random occurrence of ‘dog’ and ‘cat’ as pet which is a part of the input. In this case, though there is no ordinal sense,I feel integer encoding should work. Is there any need to implement one hot encoding?
It really depends on the dataset (how many classes) and on the algorithm.
Typically, two output classes are not integer or one hot encoded, instead, a model predicts a value between 0 and 1 for the two class values.
Thanks; actually it is one of the inputs – so the input can be cat (0) or dog(1) and they occur with equal frequency in the dataset and go in as an input to the model; there is obviously no ordinal sense. Is one-hot encoding necessary or is integer coding adequate?
Perhaps try both and see how the encoding impacts model performance?
Okay, I will try both …thanks. The tutorial was extremely helpful.
Thanks for a great post, Jason. I have a question about how to deal with numbers in a data frame that are really categorical.
Let’s say I have two columns called “Car Type” and “Engine Type”, each containing integers that represent some type. For example:
Car Type Engine Type
1 3
3 3
2 2
2 1
How can I replace these df columns using OHC when the same numbers appear in both? This isn’t a problem when the values are strings, since “Ford”, “GMC” etc. will just become columns whose values are 1 or 0. But obviously we can’t just have a column called “2”, and so I’m not sure what to do here.
Thanks for any advice you can provide!
Ok so what I did, which seems to work is:
car_type = df.pop(‘car_type’)
engine_type = df.pop(‘engine_type’)
df[‘car_type_1’] = (car_type == 1) * 1.0
df[‘car_type_2’] = (car_type == 2) * 1.0
df[‘car_type_3’] = (car_type == 3) * 1.0
df[‘engine_type_1’] = (engine_type == 1) * 1.0
df[‘engine_type_2’] = (engine_type == 2) * 1.0
df[‘engine_type_3’] = (engine_type == 3) * 1.0
This removes the original columns, and then creates 6 columns whose value is 1 if it is that type, and 0 if it is not. The only downside is that this is not efficient code and would be tedious to do for hundreds of features, but it’s not a bad start.
Here are some examples using numpy arrays directly:
https://machinelearningmastery.com/data-preparation-gradient-boosting-xgboost-python/
You can try modeling them as zeros, or you can try a one hot encoding. Perhaps try both and see which results in better performance.
Once you have created the encoding, you can add the columns back to the numpy array (hstack) or back to the dataframe.
Hi! Great and informative article. I just wanted to ask you, do we have to drop one column when one-hot encoding to avoid the Dummy Variable Trap? I ‘ve seen some who say we should drop them and others who don’t seem to mind attention to it, and I am a bit confused over which one is the correct approach. In other words, for example, when should we specify ‘drop_first=True’ when using pandas’ “get_dummies()”.
Thanks!
In practice, no.
So we don’t really have to do it in practice, thanks.
But when is this done in theory? I am curious.
Isn’t it done in Linear Regression models without regularization (In theory)? Please do let me know!
Hi
in One Hot Encode with scikit-learn , after encoding , how i can get spesific word label?
for example I want get integer code of (cold) = ? and then print for me 0
You can use the OneHotEncoder class:
https://scikit-learn.org/stable/modules/generated/sklearn.preprocessing.OneHotEncoder.html
Perhaps I don’t understand your question?
Hi Jason Brownlee,
I found an issue with example of ‘hello world’.
There is an error because of space (‘ ‘) in between hello and world.
integer_encoded = [char_to_int[char] for char in data]
KeyError: ‘ ‘
Regards,
Rajendra
Sorry to hear that, I have some suggestions here for you:
https://machinelearningmastery.com/faq/single-faq/why-does-the-code-in-the-tutorial-not-work-for-me
Hi jason, i have a data which contains both catogorial data and int data.
when i convert catogorial data into hotendioded vector and feed data to KNN clustering alforithm
i get error saying:
ValueError: setting an array element with a sequence.
is it because my array is now containing int and sequnce vector?
how to slove this?
Perhaps instead of OHE, try using different distance measures for the different variable types?
Hello
How can I prepare IP addresses in data fame for an ML model using one hot encording
Good question. I believe there are specific methods for representing IP addresses – you may need to check the literature.
Hello Jason,
array= ([0, 2, 1, 2, 0])
paff = to_categorical(array)
–> getting 3 Classes, correct
array= ([3, 2, 1, 2, 3]) getting 4 classes (incl. Null Vector)
Any reason why this happens? (pd.get_dummies gives me correctly 3 classes)
Perhaps specify the num_classes argument?
https://keras.io/utils/
Hello Jason,
Not really:
array= ([3, 2, 1, 2, 3])
paff = to_categorical(array, num_classes=4) #works only with num_classes=4
print(paff)
[[0. 0. 0. 1.]
[0. 0. 1. 0.]
[0. 1. 0. 0.]
[0. 0. 1. 0.]
[0. 0. 0. 1.]]
I really don’t get it. Neither do i need the extra Null-Vector, nor does training data always happen to have a 0 in the data. This solution always produces a matrice with an extra Null-Vector, and then you get a an keras-error because your matrice is 1 dimension larger than anticipated. Somehow i have the feeling i am missing something in keras’ idea about one hot encoding.
The function assumes class number starts at 0.
True. My conclusion: This is a weird function, only working properly (in a logical sense) with data containing a 0. Otherwise you have to keep in mind to get a larger dimension matrix. I’ll stick with pd.get_dummies() 😉
hi Jason
i have question regarding one hot encoded inputs were target is continues.
for simplicity lets assume that i have one continues output that depend about linearly on: one continues input but have different linearity dependent on one hot encoded variable. i would be thanks full for any help of how to implement this kind of system
One hot encoding is for categorical data, not real values.
If you want to explore encoding a numerical value, you can make it discrete + ordinal first.
hi sorry, just to make sure my question is understood output=V*input and V is depend on some categorized variable
Am I the only one who feels a bit ridiculous that Python, the most praised language for Data Science and Machine Learning, can’t auto-convert simple Categories, while R’s machine learning algorithms are doing just fine with Factors?
(Admittedly, I’m not a programmer and I like R)
It is not so much the language, as the tools.
R is super helpful, but also super messy.
Python is more spare. I think there is room for a caret like library that wraps all the helpful stuff in pandas/sklearn/keras/xgboost/etc.
sir how can I give labelled GT image as the train_label cnn in python to train my model by using the loss function as categorical_cross_entropy
What is a GT image?
Great tutorial Jason Brownlee.
I followed your tutorial and trying to apply one hot encoding for the following data. But I am confused about the output. Could you please tell me, is the output is right or wrong after one hot encoding?
X = [[‘A’, ‘G’, ‘T’, ‘G’, ‘T’, ‘C’, ‘T’, ‘A’, ‘A’, ‘C’],
[‘A’, ‘G’, ‘T’, ‘G’, ‘T’, ‘C’, ‘T’, ‘A’, ‘A’, ‘C’],
[‘G’, ‘C’, ‘C’, ‘A’, ‘C’, ‘T’, ‘C’, ‘G’, ‘G’, ‘T’],
[‘G’, ‘C’, ‘C’, ‘A’, ‘C’, ‘T’, ‘C’, ‘G’, ‘G’, ‘T’],
[‘G’, ‘C’, ‘C’, ‘A’, ‘C’, ‘T’, ‘C’, ‘G’, ‘G’, ‘T’]]
Y = np.array(X)
# one hot encoding
print(Y)
print(Y.shape)
onehot_encoder = OneHotEncoder(sparse=False)
onehot_encoded = onehot_encoder.fit_transform(Y)
print(onehot_encoded)
print(onehot_encoded.shape)
Output:
[[1. 0. 0. 1. 0. 1. 0. 1. 0. 1. 1. 0. 0. 1. 1. 0. 1. 0. 1. 0.]
[1. 0. 0. 1. 0. 1. 0. 1. 0. 1. 1. 0. 0. 1. 1. 0. 1. 0. 1. 0.]
[0. 1. 1. 0. 1. 0. 1. 0. 1. 0. 0. 1. 1. 0. 0. 1. 0. 1. 0. 1.]
[0. 1. 1. 0. 1. 0. 1. 0. 1. 0. 0. 1. 1. 0. 0. 1. 0. 1. 0. 1.]
[0. 1. 1. 0. 1. 0. 1. 0. 1. 0. 0. 1. 1. 0. 0. 1. 0. 1. 0. 1.]]
(5, 20)
Before applying one hot encoding the shape was (5,10) and after applying one hot encoding the shape of data is (5,20). So, I am confusing about the shape of data.
Not sure it is correct.
It looks like one “row” is a sequence of letters. Encoding the letters would give you a binary vector for each letter that would be concatenated into one long vector to represent a row.
So, the number of rows would stay at 5, each letter would be encoded as a 4 element vector (or something?), giving you a row of 4*10 elements.
Thank you Jason Brownlee for your reply. I also thought the same. After applying one hot encoding, the shape should be (5, 40) instead of (5,10). Do you know how I can I handle such kind of 2D array for one hot encoding?
Yes, transform the variables and concatenate the results.
You can use the one hot encoding in keras or scikit-learn and concat function for numpy arrays.
What specific problem are you having?
I didn’t get your answer. How can I get the correct shape for one hot encoding for this array? Could you please give me any example of one hot encoding by using 2D numpy array?
This is a demo data I made. I have to predict phenotype from genotype data like this. But before that I have to process data.
The examples in the above tutorial should help?
Perhaps some of the tutorials here:
https://machinelearningmastery.com/start-here/#nlp
Hello Jason,
for cancer survival prediction I have many attributes. suppose I have 20 nominal categorical attributes which after one-hot encoding the columns turn to 150 features. after calculation, I intend to know which attributes are important in the calculation. Here I have just some numbers which are the variables of the attributes. My question is how I can find out which features are important. To clarify my question. features 21, 45, 56, 74, 84 are important. How can I figure out where these numbers belong to?
That is challenging.
You can solve it with simple counting, e.g. n categories for each variable concatenated together.
Perhaps you can use RFE, where the RFE is used on each variable prior to being one hot encoded in your modeling pipeline. That would solve feature selection and give ideas on feature importance.
I had to face this error after checking this article i fixed it thank you to everyone
What error?
In the Fashion MNIST data-set , we converted each label from an integer to one hot encoded vectors. What was the dimension of these vectors? Enter only the integer value
what will be the answer for this
The one hot encoding will have the length of the number of classes. 10 classes means a one hot encoding with 10 elements.
Dear Jason,
How we can predict the next time step value for categorical variable in a time series problems? More specifically, If your input are multiple categorical variables is it possible to predict the value of those multiple categorical variables for the next time step? If yes, would you please give me a hint how should I do that?
Thanks
In the continues of the above-mentioned question, when I convert my variables to one-hot encoding, the shape of my train input would be (362, 3, 5, 9) which: (362 is number of samples), (3 is number of time step), (5 is the number of features), and (9 is the length array). The input shape would be like the following:
(362, 3, 5, 9)
[[[[1. 0. 0. 0. 0. 0. 0. 0. 0.]
[0. 0. 0. 0. 1. 0. 0. 0. 0.]
[0. 1. 0. 0. 0. 0. 0. 0. 0.]
[1. 0. 0. 0. 0. 0. 0. 0. 0.]
[0. 1. 0. 0. 0. 0. 0. 0. 0.]]
[[1. 0. 0. 0. 0. 0. 0. 0. 0.]
[0. 0. 0. 0. 1. 0. 0. 0. 0.]
[0. 1. 0. 0. 0. 0. 0. 0. 0.]
[1. 0. 0. 0. 0. 0. 0. 0. 0.]
[0. 1. 0. 0. 0. 0. 0. 0. 0.]]
[[1. 0. 0. 0. 0. 0. 0. 0. 0.]
[0. 0. 0. 0. 1. 0. 0. 0. 0.]
[1. 0. 0. 0. 0. 0. 0. 0. 0.]
[1. 0. 0. 0. 0. 0. 0. 0. 0.]
[0. 1. 0. 0. 0. 0. 0. 0. 0.]]]]
Would be possible to feed this 4D data to CNN or LSTM for predicting the next time step for each feature considering the 3D needed input for those neural network?
If yes, would you please give me a hint how should I do that?
Perhaps try it and see?
yhat = model.predict(newX)
/very nice post andthanx for sharing such information.
Thanks, you’re welcome.
Thank so much Jason. Just in time…
You’re welcome!
Dear Jason,
If I have 3 output features: Out1 ; Out2; Out3. Each of them has a value that varies from 1 to 5 as below:
[[0 4 0]
[0 2 0]
[1 0 0]
[2 0 0]
[2 0 0]
[2 0 0]
[1 0 1]
[0 0 1]
…….]
I understand if I apply one_hot_encoded with n_unique=5, it will be 15 output features. it is greater than the number of input. The result is terrible.
How can I use one_hot_encoded for this case?
Thank you so much!!
Yes, 15 input variables. That is not many, some problems may have thousands.
Compare a suite of algorithms.
Compare raw data with the same algorithm.
Compare to an embedding with a neural net.
ValueError: y should be a 1d array, got an array of shape (7343360, 2) instead.
when converting two labels
Sorry to hear that you’re having trouble, perhaps these tips will help:
https://machinelearningmastery.com/faq/single-faq/why-does-the-code-in-the-tutorial-not-work-for-me
how to apply above method for integers in y-train and y-test in multiclassification problem?
The above code is directly applicable.
If you’re having trouble, perhaps start here:
https://machinelearningmastery.com/one-hot-encoding-for-categorical-data/
hii dear, thanks alot for this it was really helpful and i have a doubt if possible please clarify it
I have some 1000s of files having total numbers in it in array form and i have another 5 labels seperately related to the same array each label related to each array data uniquely so how can i proceed to implement to show out of 5 labels 1 label as 0 and remaining 4 are 1s as output by loading that array data please help me if possible ..
Sorry, I don’t understand your question, perhaps you can elaborate or rephrase?
Dear Jason, very nice post!
One question that I never quite understood:
What exactly happens when we feed a, let’s say, vanilla Seq2Seq model, with one-hot vector representations?
Is it correct to say that during training the ‘black-box’ ‘transforms’ one-hot vector representations to dense vector representations that corresponds to the sequential knowledge?
Is there some advantage to feed a Seq2Seq with dense vectors instead of one-hot vectors when we train a model from scratch?
Many thanks again man, you help me a lot with your examples!
An LSTM takes input as [samples, timesteps, features], the one hot encoded input would be separate features.
A dense input, e.g. integer encoding or an embedding, might be more effective. Compare the performance of different methods.
Thanks this was really clear and easy to understand. Google often takes me to your website
Thanks. Glad you like it.
Hi,
Is there a way to perform mutual_info_regression after having applied One Hot Encoding? If not, does the “ranking problem” that characterizes LabelEncoder also influence mutual_infor_regression results?
Thank you
mutual_info_regression assumes that target variable is continuous. If the one-hot encoding applies on features, I believe it is still useful. But if it is on the target, I doubt, because the error might be large.
I read it really well.
For categorical data, machine learning can’t recognize it.
I know why you’re one-hot encoding.
Why do you do one-hot-encoding for integers?
Can’t machine learning recognize the integer type?
Model can recognize integer type but if you don’t want it to misunderstood integer type (like my phone number) as something on the face value while it is actually just a name, then you do one-hot encoding.
Dear Jason. I have checked that the current code for manual hot-encoded gives an erroneous result. The reason is that, when you create the dicts (lines 8 ands 9) you use the same ‘c’ variable and it seems that it doesn’t reinitialize when executing the ‘ennumerate’ function. If you print both dicts after creating you could see that the results are not symetric.
I solved it by changing the name for the ennumerate variable in the second dict.
Thanks a lot for your incredibly useful and interesting blog
Mario
Thanks for pointing that out. But the exact behavior should depend on the version of Python. The latest version should limit the scope of “c” to within the dict comprehension syntax so it should be just fine. For older versions, what you said can be an issue.
Sorry, it was my mistake while modifying the code… 🙂
array([1.9 , 1.635, 1.639, …, 1.704, 1.672, 1.596])
array([[0., 0., 0., …, 0., 1., 0.],
[0., 0., 0., …, 0., 1., 0.],
[0., 0., 0., …, 0., 1., 0.],
…,
[0., 0., 0., …, 0., 0., 1.],
[0., 0., 0., …, 0., 0., 1.],
[0., 0., 0., …, 0., 0., 1.]])
I have the numeric array that i want to predict and the day of the week which has been one hot encoded
How will i create a sequence and also fit this in a time series LSTM model
Hi Bobby…The following tutorial includes a discussion of data preparation:
https://machinelearningmastery.com/how-to-develop-lstm-models-for-time-series-forecasting/
what does it mean when an encoding returns all ‘1’s’ for a column.
Hi Michael…The following discussion may be of interest to you:
https://stackoverflow.com/questions/66029867/one-hot-encoding-returns-all-0-vector-for-last-categorical-value
I have a question suppose we categorize a sequence is a palindrome or not in our rnn structure how can we use one hot encode in our model
Hi Veday…You may find the following of interest:
https://www.geeksforgeeks.org/python-program-check-string-palindrome-not/