Getting started in applied machine learning can be difficult, especially when working with real-world data.
Often, machine learning tutorials will recommend or require that you prepare your data in specific ways before fitting a machine learning model.
One good example is to use a one-hot encoding on categorical data.
- Why is a one-hot encoding required?
- Why can’t you fit a model on your data directly?
In this post, you will discover the answer to these important questions and better understand data preparation in general in applied machine learning.
Kick-start your project with my new book Data Preparation for Machine Learning, including step-by-step tutorials and the Python source code files for all examples.
Let’s get started.
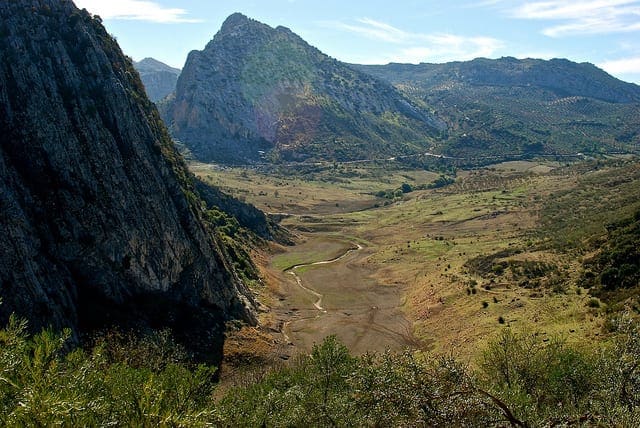
Why One-Hot Encode Data in Machine Learning?
Photo by Karan Jain, some rights reserved.
What is Categorical Data?
Categorical data are variables that contain label values rather than numeric values.
The number of possible values is often limited to a fixed set.
Categorical variables are often called nominal.
Some examples include:
- A “pet” variable with the values: “dog” and “cat“.
- A “color” variable with the values: “red“, “green” and “blue“.
- A “place” variable with the values: “first”, “second” and “third“.
Each value represents a different category.
Some categories may have a natural relationship to each other, such as a natural ordering.
The “place” variable above does have a natural ordering of values. This type of categorical variable is called an ordinal variable.
Want to Get Started With Data Preparation?
Take my free 7-day email crash course now (with sample code).
Click to sign-up and also get a free PDF Ebook version of the course.
What is the Problem with Categorical Data?
Some algorithms can work with categorical data directly.
For example, a decision tree can be learned directly from categorical data with no data transform required (this depends on the specific implementation).
Many machine learning algorithms cannot operate on label data directly. They require all input variables and output variables to be numeric.
In general, this is mostly a constraint of the efficient implementation of machine learning algorithms rather than hard limitations on the algorithms themselves.
This means that categorical data must be converted to a numerical form. If the categorical variable is an output variable, you may also want to convert predictions by the model back into a categorical form in order to present them or use them in some application.
How to Convert Categorical Data to Numerical Data?
This involves two steps:
- Integer Encoding
- One-Hot Encoding
1. Integer Encoding
As a first step, each unique category value is assigned an integer value.
For example, “red” is 1, “green” is 2, and “blue” is 3.
This is called a label encoding or an integer encoding and is easily reversible.
For some variables, this may be enough.
The integer values have a natural ordered relationship between each other and machine learning algorithms may be able to understand and harness this relationship.
For example, ordinal variables like the “place” example above would be a good example where a label encoding would be sufficient.
2. One-Hot Encoding
For categorical variables where no such ordinal relationship exists, the integer encoding is not enough.
In fact, using this encoding and allowing the model to assume a natural ordering between categories may result in poor performance or unexpected results (predictions halfway between categories).
In this case, a one-hot encoding can be applied to the integer representation. This is where the integer encoded variable is removed and a new binary variable is added for each unique integer value.
In the “color” variable example, there are 3 categories and therefore 3 binary variables are needed. A “1” value is placed in the binary variable for the color and “0” values for the other colors.
For example:
1 2 3 4 |
red, green, blue 1, 0, 0 0, 1, 0 0, 0, 1 |
The binary variables are often called “dummy variables” in other fields, such as statistics.
For a step-by-step tutorial on how to one hot encode categorical data in Python, see the tutorial:
Further Reading
- Categorical variable on Wikipedia
- Nominal category on Wikipedia
- Dummy variable on Wikipedia
Summary
In this post, you discovered why categorical data often must be encoded when working with machine learning algorithms.
Specifically:
- That categorical data is defined as variables with a finite set of label values.
- That most machine learning algorithms require numerical input and output variables.
- That an integer and one hot encoding is used to convert categorical data to integer data.
Do you have any questions?
Post your questions to comments below and I will do my best to answer.
You didn’t mention that if we have a categorical variable with 3 categories, we only need to define 2 one-hot variables to save us from linear dependency.
Hi Jason,
Thanks so much for the great, straightforward tutorial. I am trying to use the scikit-learn methods to determine how to convert my categorical data and I have a couple of questions. First can you also use get_dummies and would that work just as well even though you end up with 1 less binary column? Also once you have converted the categorical variable you have several new binary columns but you also still have the original text form of the categorical variable. Should I drop that text version of the categorical variable or just leave it there in the dataset?
Thanks so much for your help,
Barbara DiLucchio
Yes, drop the original untransformed data.
Hi Jason,
Great post – quick question I’m looking at a solution that requires categorical data to be converted for processing – was going to use label encoding followed by one hot as you have outlined above – following this example if I have 10 features (say 20 by 10) with three categories of data in each – will the result be a 20 by 30 data-set and is it now in the correct format for scaling and PCA
PCA would not be appropriate for one hot encoded categorical data.
Jason, I’ve found one-hot encoding to be incredibly effective for PCA. What are the arguments against?
r
library(ds.anomaly)
#>
#> Attaching package: 'ds.anomaly'
#> The following object is masked from 'package:stats':
#>
#> biplot
library(magrittr)
library(ggplot2)
names(iris)[names(iris) == "Species"] <- "Iris."
# Standard PCA
eg % do_T2(exclude. = "Iris.", method = "svd")
eg %>% biplot(group. = "Iris.")

r
# One-hot encoded classifier
eg % do_T2()
eg %>% biplot(group. = "Iris.")

Created on 2019-01-16 by the [reprex package](https://reprex.tidyverse.org) (v0.2.1)
I would expect the encoded vectors to be too sparse for PCA, perhaps I’m wrong.
I’m kinda agree with Jason that data will to too sparse for PCA (although well scaled). Also, the resulting one-hot encoded vectors are linearly independent rendering PCA ineffective (quite a complex algorithm it is). Please correct me if I’m wrong.
HI Jason,
I love your articles.
Is there any guidance when we should use one-hot encoding and when we should use frequency / lift ratio /Rgression coeff for the categorical variable?
Also, if there are many categorical variables, do we need to check multi-collinearity after one hot encoding (e.g. VIF)?
Thanks for the answer in advance?
I recommend testing a suite of methods for a model/dataset and choose an representation based on model performance.
I also recommend testing an embedding, they work well for categorical features, especially high cardinality with interactions.
Totally agree..I had the same confusion while reading the same part of article..
Goooood article tho.Thanks
HHi jason.I truly following you alot and really appreciate your effort and ease of tutorials.just a question,How one hot encoding would work for multilabel class and in coming tutorials could you help in featureselection of text data for muticlass and multilabel classification using keras.i tried multiclass for 90 datapoints. And used keras for mlp,cnn and rnn where each datapoint is long paragraph with labels but accuracy i got is 37.5 prcent. Let me know if you have any suggestions
The one hot vector would have a length that would equal the number of labels, but multiple 1 values could be specified.
Thanks for the suggestion.
This post suggests ways to lift deep learning model skill:
https://machinelearningmastery.com/improve-deep-learning-performance/
Very helpful. I discovered the limits to using categorical data with trees and random forests. Wasn’t sure of the correct solution until I saw your post on one-hot encoding. For those interested, I also discovered your post on how to program this in python and other languages.
https://machinelearningmastery.com/how-to-one-hot-encode-sequence-data-in-python/
Thanks.
What are the cons of one hot encoding ??? Supposed that you have some categorical features with each one with 500 or more differents values !! So when you do one hot encoding you will have many colums in the dataset does it still good for a machine learning algorithm ???
Great question!
The vectors can get very large, e.g. the length of all words in your vocab in an NLP problem.
Large vectors make the method slow (increased computational complexity).
In these cases, a dense representation could be used, e.g. word embeddings in NLP.
Hi Jason, thanks again for your amazing pedagogy.
Back to the Espoirt question, I face this problem with 84 user_ID. I do a OHE of them and, like you said when I fit the data with a SVM classifier, it’s look like I fall in a infinite loop. So taking in to account the fact that I am not in the NLP case, how can I fixe this ?
Thanks.
What do you mean you fall into an infinite loop?
Very helpful post, Jason!
Espoir raised my question here but I did not undestand how to apply your answer to my case. I have 11+ thousand different products id. The database has about 130 thousand entries. This easily leads to MemoryError when using OHE. What approach/solution should I look for?
Ouch.
Maybe you can use efficient sparse vector representations to cut down on memory?
Maybe try exploring dense vector methods that are used in NLP. Maybe you can something like a word embedding and let the model (e.g. a neural net) learn the relationship between different input labels, if any.
Hello Jason how do we retrieve the features back after OHE if we need to present it visually?
You can reverse the encoding with an argmax() (e.g. numpy.argmax())
Thank you for these wonderful posts!
Does data have to be one-hot encoded for classification trees and random forests as well or they can handle data without it? Or just try which gives better results?
No, trees can deal with categories as-is.
In case of high cardinality, wont the model start grouping integer labels into ranges ?
Yes.
Hi Jason, this post is very helpful, thank you!!
Question- In general what happens to model performance, when we apply One Hot Encoding to a ordinal feature? Would you suggest only to use integer encoding in case of ordinal features?
It really depends on the problem and the meaning of the feature being encoded.
If in doubt, test.
I see, thanks!
hey Jason,
As usual this is another useful post on feature representation of categorical variables. Since logistic regression fits a separation line on the data points of the form w1X1 + w2X2 +.. where X are features such as categorical variables- Places,color etc, and w are weights, intuitively X can take only numerical values for the line to fit. Is this a right intuition?
Yes, regression algorithms like logistic regression require numeric input variables.
Thanks a lot for your clarifying. I love your blogs and daily email digests. They help me to understand key concepts & practical tips easily.
Thanks Raj.
nice!
Thanks.
very well explained..thanks
Thanks, I’m glad it helped.
I love your blog!
One question: if we use tree based methods like decision tree, etc. Do we still need one-hot encoding?
Thanks you very much!
No Jie. Most decision trees can work with categorical inputs directly.
Thank you very much!
No probs.
How? Any resource link would be appreciated
Thanks,
Yash
I’m not aware of python implementations that support categorical data directly. I know the algorithm does, perhaps you can implement it yourself.
Hi Jason, loving the blog … a lot!
I’m using your binary classification tutorial as a template (thanks!) for a retail sales data predictor. I’m basically trying to predict future hourly sales using product features and hourly weather forecasts, trained on historical sales and using above/below annual average sales as my binary labels.
I have encoded my categorical data and I get good accuracy when training my data (87%+), but this falls down (to 26%) when I try to predict using an unseen, and much smaller data set.
As far as I can see my problem is caused by encoding the categorical data – the same categories in my unseen set have different codes than in my model. Could this be the cause of my poor prediction performance: the encoded prediction categories are not aligned to those used to train and test the model? If so how do you overcome these challenges in practice?
Hope it makes sense.
Nice work Andrew!
Your model might be overfitting, try a smaller model, try regularization, try a large dataset, try less training.
Here are more ideas:
https://machinelearningmastery.com/improve-deep-learning-performance/
I hope that helps as a start.
Hey Jason, didn’t think I had ‘that’ problem, but I probably do 🙂
Many thanks.
Appreciable and very helpful post, thank you!!!
Question: What is the best way to one hot encode an array of categorical variables?
I have also startup with a AI post you can also find some knowledge over there: Thebigmoapproach.com/
There are many ways and “best” is defined by the tools and problem.
Here are a few ways:
https://machinelearningmastery.com/how-to-one-hot-encode-sequence-data-in-python/
hi Jason:
One question, take the “color” variable as an example,if the color is ‘red’ , then after one-hot encoding ,it becomes 1,0,0 . So,can we think that it generates three features from one feature?
It has been added two columns,is that right?
Correct Tom!
Hi Jason, if my input data is [1 red 3 4 5], if use one hot encoder, red become [1,0,0], ]does it mean that the whole features of the input data is extended?
input data now is [1 1 0 0 3 4 5]
Sorry, I don’t follow. Perhaps you can restate your question?
Hi Jason, Thank you for the reply.
For example, if I have 4 feature of my input, [121 4 red 10; 100 3 green 7; 110 8 blue 6]
For the first row, the value related to each feature–feature 1:121, feature 2:4, , feature: red, feature 4: 10.
I want to use one hot encoder now, red = [1,0,0], green = [0,1,0], blue = [0,0,1].
So my input become [121 4 1,0,0 10; 100 3 0,1,0] 7; 110 8 0,0,1 6] , after one hot encoding, we now have 6 features, so I use the new data for training, it that right?
Thanks.
Hello DR. Brownlee,
I am training a model to detect attacks and i need someone like you to help me detect the mistakes in my code because my training is not producing any better results. Kindly alert me if you will be interested to help me.
Thank you
Sorry, I do not have the capacity to review your code.
I am using Tensorflow developing the mode,and would want to know how your book can help me do that. since it makes reference to Keras. Thank you
My deep learning book shows how to bring deep learning to your projects using the Keras library. It does not cover tensorflow.
Keras is a library that runs on top of tensorflow and is much easier to use.
This is not entirely correct as far as I understand. As Varun mentioned, you need to have one less column (n-1 columns). What has been described is dummy-encoding (which is not one-hot-encoding). There is a major problem with dummy encoding which is perfect collinearity with the intercept value. As the sum of all the dummy values of one category (n columns) is ALWAYS equal to 1. So it’s basically an intercept
Other way around I believe. Dummy encoding is n-1 columns, one hot has n columns.
Hi Jason,I have 6 categorical values which are present in the data that I have. The data that I have has many missing categorical values that are left as empty strings. What to do if I have missing categorical values? Do I need to OHE them also? or how to deal with the categorial feature with missing values?
I’m using sci-kit learn and trying out many algorithms for my dataset.
I list some ways to handle missing data here:
https://machinelearningmastery.com/handle-missing-data-python/
Hi Jason,
First thank you for your post !
There is something i did not understand about your explanation : let’s take the color example (so red is 1, green is 2, blue is 3).
I did not understand the “ordinal relationship between catégories” : does the One-Hot-Encode allow better accuracy for some learning algorithms than these categories? (So far here’s what I thought: the algorithm reads 1,2 or 3 instead of red, green or blue, and makes the necessary correlations for predictions, and that has no impact on the predictions accuracy.)
Hmm. Sorry for not being clearer.
Ordinal means ordered. Some categories are naturally ordered and in these cases some algorithms may give better results by using just an integer encoding.
For problems where the categories are not ordered, the integer encoding may result in worse performance than one hot encoding because the algorithm may assume a false ordering based on the assigned numbers.
Does that help?
Bit confused. In the case we ordered integer labels correctly, do we need one hot encoding? Actually it is bit stupid that labeling impacts to acc. I thought one hot labeling is for simplicity but you say that in the case of integer label which is not well ordered.
Is there any reason why we use one hot encoding in the case we order integer labels correctly?
I accept what u explained why we need to use integer encoding instead of character labeling.
Thank you
I was saying that if your variable values are not ordinal and you treat them as ordinal when fitting the model (e.g. not use one hot encoding), you may loose skill.
Does that help?
I have data from 20 000 stores. Each store has it’s integer ID. This ID is meaningless, just ID. Should I add 20 000 binary variables to datatset? And 20 000 neurons in input layer of LTSM? It sounds frightening…
No, drop the id unless you have a hunch that it is predictive (e.g. numbering maps to geographical region and regions have similar outcomes).
Ok, I have latitude and longitude of each store. Should I use them instead of ID? Similar question. I have 17 states of weather (cloudy, rainy, etc.). Should I replace them with 17 binary variables? Or should I try to give integer code to them to show similarity of heavy rain to rain and light rain, sunny to partial clouds and heavy clouds?
There are no rules, I would encourage you to try many different framings and see what works best for your specific data.
I have some biases that I could suggest, but it would be better (your results would be better) if you use experiments to discover what works for your problem.
Yes, it’s right, thanks
Great post Jason! I’m glad I came across it. It really helped me to understand the need for one hot encoding. I’m new to machine learning and I am currently running xgboost in R for a classification problem.
I have 2 questions:
(1) If my target variable (the variable I want to predict) is categorical, should I also convert it into numeric form using hot encoding or will a simple label encoding suffice?
(2) Are there specific R packages for one hot encoding of features?
It really depends on the method. It can help.
Sorry, I don’t recall if you must encode variables for xgboost in R, it has been a long time.
Most R ML methods handle factors without the need for explicit one-hot coding. But xgboost is an exception, so you need to use a function like sparse.model.matrix() to encode your data set before passing it to xgboost. (This function actually encodes factors as “indicator variables” rather than one-hot encoding, but the general idea is the same.)
ice.
you haven’t replied to the first question jason.
I need to know the same
(1) If my target variable (the variable I want to predict) is categorical, should I also convert it into numeric form using hot encoding or will a simple label encoding suffice?
Typically all categorical variables are encoded when modeling because machine learning algorithms must work with numbers.
This includes inputs and outputs to the model.
Should this mean one-hot encoding in particuilar or would integer encoding suffice?
Perhaps try both.
Hello Jason
I have dataset having numeric and nominal type. It also has missing values. For nominal datatype, first I applied Labelencoder() to convert them into numeric values, but along with my two categories(normal, abnormal), it also assigns a code to NaN. In such scenario how can I impute values by its Mean?
You can impute with the mode in this case.
Hi Jason,
Since the number of columns created for a categorical column after applying OneHotEncoding is equal to the number of unique values in that categorical column; often it happens that the number of features in the tested model is not equal to the number of features on the dataset to be predicted after applying OHE similarly on the categorical fields. In such cases model throws an error while predicting since it expects equal number of features both in the training and to be predicted dataset. Can you please advise how to handle such situation ?
The same transform object used for training is then used for test or any other data. It can be saved to disk if need be.
Hi Jason,
I couldn’t get what do you mean by “same transform object”. ? The training dataset structure (number of initial features) is same both for Training and Testing/to-be-predict dataset. But the uniqueness of values under each feature/column may differ which is quite natural. Therefore OneHotEncoding or pandas get_dummies create different number of encoded features in Test/to-be-predict dataset than the training dataset. How to deal with this issue – that is what my question.
Need your advise please.
Thanks.
Sorry. To be clearer, you can train the transform objects on the training data and use them on the test set and other new data.
The transform objects may be the label encoder and the one hot encoder.
The training data should be such that it covers all possible labels for a given feature.
Does that help?
Hi Jason,
should I do one-hot encode for two level categorical variables? like variable only contains (yes. no) converts to two variable (0,1) and (1,0)
Thanks.
Generally, this is not needed.
For One-Hot Encoding (OHE) of a categorical variable State with 4 values: NJ, NY, PA, DE
We can remove one of them, say DE, to reduce complexity.
So if NJ=0, and NY=0, and PA=0, then it is DE
Is removing one recommended?
This becomes more obvious in the case of a binary categorical variable.
Thanks.
If you can simplify the data, then I would recommend doing that.
Always test the change on model skill though.
One Hot Encoding via pd.get_dummies() works when training a data set however this same approach does NOT work when predicting on a single data row using a saved trained model.
For example, if you have a ‘Sex’ in your train set then pd.get_dummies() will create two columns, one for ‘Male’ and one for ‘Female’. Once you save a model (say via pickle for example) and you want to predict based on a single row you can only have either ‘Male’ or ‘Female’ in the row and therefore pd.get_dummies() will only create one column. When this occurs the number of columns no longer matching the number of columns you trained your model on and errors out.
Do you know a solution to this issue? My actual need uses Zip Code rather than Sex which is more complex.
I recommend using LabelEncoder and OneHotEncoders from sklearn on a reasonable sample of your data (all cases covered) and then pickle the encoders for later use.
Thank you!!!
Hi Jason,
I am piggybacking on some of the other questions re: n-1 encoding and n encoding. I have a dataset where I predict price based on day of week using sklearn LinearRegression (also playing with Ridge). I used DictVectorizer in sklearn to prep my data and I end up with 7 columns for day of week, rather than 6. In some of the questions above, you indicate simpler is better…though you do say to “test the change on model skill.” Could you elaborate on that – for example, what are the practical implications of using one or the other for a dataset like mine (features = days of week; target = price)? My model seems to spit out a reasonable y-intercept, though I’m not sure exactly what the y-intercept is because my model has no [0, 0, 0, 0, 0, 0, 0] for day (i.e., no “reference” day).
Is there a mathematical reason to use n-1 vs n encoding? I hope this makes sense. I’ve Googled like 50 times and can’t find an article that really gets into this. Thank you.
If your goal is the best model skill, then use whatever works to improve that skill.
No need for idealized justifications.
Very helpful, thanks.
May I ask that if I have 4 possible letters in a string that I would like to encode (let’s say A B C D), what is better for neural networks? One-ho or integer encoding. The groups have no order, so I would say one-hot but I do not know whether neural network could deal with integer encoding in this case (it would mean a quarter of the features as one-hot encoding).
Thank you!
One hot if there is no ordinal relationship between the labels.
I am total noob to this so maybe a silly question but this can only be applied when categories are less in number and the problem is about classification right?
One hot encoding can be used on input features for any type of problem and on the output feature for classification problems.
Hello jason i have question: If there are categorical variable like 1st class, 2nd class, 3rd class fror housing price prediction , if i am converting with OneHotcoding so how algorithm will judge the ranking part of housing ? Yes it does convert it into binary but does it also taking car of ranking of that categorical variable? & One more question is to get binary output “pd.get_dummy” is useful or One HotEncoder is useful ?
A one hot encoding is for a classification problem, not regression.
The house price is a regression problem where we predict a quantity.
Hi Jason..I have a data with string values having more than 500 unique values. How can I encode it so that can pass it to Ml algorithm. Is this good candidate for categorical encoding?
That is a lot. I would recommend NLP representations such as bag of words or word embeddings.
I have posts on both, start here:
https://machinelearningmastery.com/start-here/#nlp
Hi Jason,
This is a great post, thanks for providing such valuable info. My question is:
If we have many colors in the color column, say 25 colors, what if we encode the colors in 3 columns with RGB values instead of 25 binary columns? Do you see any abnormality with this approach?
No problem, it would be a binary vector with 25 elements.
Hi Jason.
I am an intern in data science, no exprience in datas. Thanks for your posts and e-mails that boosted my confidence to start my intern on ML and Deep learning.
currentley, i got a dateset of 10 GB and after i make a preliminary investagation on the data i found out the following.
feature ‘x1’ has 78 unique categories
feature ‘x2’ has 24 unique categories
feature ‘x3’ has 24 unique categories
feature ‘x4’ has 35 unique categories
feature ‘x5’ has 40 unique categories
feature ‘x6’ has 106 unique categories
feature ‘x7’ has 285629 unique categories
feature ‘x8’ has 523912 unique categories
feature ‘x8’ has 27 unique categories
feature .x9’ has 224 unique categories
feature ‘x10’ has 108 unique categories
feature ‘x11’ has 98 unique categories
feature ‘x12’ has 10 unique categories
feature ‘x13’ has 1508604 unique categories
feature ‘x14’ has 15 unique categories
feature ‘x15’ has 1323136 unique categories
feature ‘x16’ has 3446828 unique categories feature ‘x17’ has 10 unique categories
feature ‘x18’ has 200 unique categories
feature ‘x19’ has 2575092 unique categories
feature ‘x20’ has 197957 unique categories
how you you deal with this data set….. it has categorical and int attributes. it a classification problem. just predict the out come either lets say 0 nor 1. how you handle the category or how would you encode this attributes.
should i simply use label encoder , one hot encoder or dummies. is it possible to encode such a big categories after all?
i am confused where to start.
Looking forwards for your suggestions and help
That is a lot of categories.
Perhaps you can remove some features?
Perhaps you can consolidate the categories for each feature?
You can get started with feature selection here:
https://machinelearningmastery.com/an-introduction-to-feature-selection/
The article boils down to one sentence:
“using this encoding and allowing the model to assume a natural ordering between categories may result in poor performance or unexpected results (predictions halfway between categories)”
And that’s enough said. Thanks.
Not quite.
That applies to the integer encoding, not the one hot encoding.
In fact, that is the problem that the one hot encoding will over come.
I disagree with one hot encoding approach. I mean, it depends on the algorithm. My opinion is based on playing around with categorical data and various algorithms on many Kaggle competitions with real world data.
For example, LightGBM can offer a good accuracy when using native categorical features. Not like simply one-hot coding, LightGBM can find the optimal split of categorical features. Such an optimal split can provide the much better accuracy than one-hot coding solution. (official documentation: http://lightgbm.readthedocs.io/en/latest/Advanced-Topics.html)
PS. Compared to other GBMs (native gbm, h2o gbm or even xgboost), lightgbm is far ahead in terms of speed and accuracy.
Thanks for the note Pranav.
Pranav, can you provide a link to any benchmark results which show superior speed and accuracy of lightgbm relative to xgboost?
Excuse me!
I am confused by dtype of dummy variable versus normal numeric variable.
Take Python for example,
If I using get_dummies function in pandas to convert category variable to dummy variable, it will return binary, and the dtype of those dummy variables are integer. How python determine those “int” variables are dummy variable, why python would not confused those ‘int’ with other normal numeric variables?
Would Python treat those dummy variables(dtype = int) as a numeric variable?
(int type is one of numeric type and computable, isn’t it?)
This question may be a bit stupid, but it really confusing me for a while…
Thanks for your help!
This is the plan, to have the algorithm treat them like other numeric variables.
Does that help?
Although algorithm treat them like other numeric variables, but the model can work just like there have category variables and numeric variables, right?
In R, I can make a variable as “factor” by “as.factor”, and give to the model directly, it’s very intuition, so when I using Python to do the same thing, I got confused, although I originally know it should be preprocess by get dummy…
I think that’s concept of dummy variable…but I got a lost for how dummy variable work in programming language…
Thank for your help !
With sklearn and keras, you must integer encode or one hot encode.
This may not be the case with other libraries in Python this may not be the case.
Thanks a lot!
Hai @Jason Brownlee. How can we have constant dummy variables if we want to predict new dataset?
I have a case, i have generated a model using variables that converted into dummy variables. It has 13 dummy variables after onehotEncoded. I make model from Neural Networks.
Now i’m going to predict some rows of new dataset. Of course the data must be onehotEncoded. But the result is 9 dummy variables after onehotEncoded.
I’m still confused how to understand this problem. It seems that there are unbalancing between those kind of dummy variables. Is there any solutions to solve this problem?
You must use the same encoding process on new data that was used for the training data.
You might need to save the objects involved.
Hello, @Jason Brownlee,
Can you guide me about Info column w.r.t protocol column in .pcap (network capture file).
How should I deal with Info column, at the end I have to apply classification.
Regards,
Sorry, I am not familiar with your dataset.
Can you please list which kind of ML algorithms do not handle categorical variable properly and meed one-hot or dummy coding, unlike decision trees.
It depends on the implementation of the algorithm (e.g. the library).
For example, in Python, pretty much all the sklearn implementations require input categorical variables to be encoded.
jason – thanks for all your help and previous responses to everyone’s questions, it’s genuinely appreciated. but here’s another…
i’m looking to predict monthly household KwH usage using 100+ variables related to socio-economics, demographics, housing features, quantity/quality of appliances, etc. since many of the variables have naturally occurring NAs as a result of previously contingent “No” or 0 answers, I’m considering one hot encoding the categoricals to “get rid” of the NAs…i’m also going to bin the ordinal outcome variable to try out classification algorithms as well…thoughts?
thanks again for your help!
Perhaps try imputing the missing values with an average from recent days/weeks/months?
This might help too:
https://machinelearningmastery.com/handle-missing-timesteps-sequence-prediction-problems-python/
Great article Jason. Would be incredibly nice if you could advise me on a slightly related problem.
I have an ordinal column, say proficiency = beginner, intermediate, advanced, expert.
After label encoding it, I get (0, 1, 2, 3).
Another is a numeric column age.
The last column is a boolean, is_certified (1, 0)
I am wondering whether I’ve to standardize proficiency column(similar to age) as it a freshly made numeric column.
Try it with and without and see how it impacts model skill.
I’m sure you’re sick of discussing this, but I feel my dummy vs one-hot situation differs from the previously discussed situations enough to warrant a response.
Lets say you were encoding a checkers board, you’d be able to represent the occupancy of each square (4 possible pieces, the men and kings of each color) with a length 5 one-hot encoding, or 4 dummy variables.
Intuitively it makes sense for the 4 dummy variables to represent the 4 possible pieces which can occupy a square, and to have the unoccupied square be represented by all unoccupied dummy variables (zero values). But, just as easily, the dummy variable for White Kings could be swapped with unoccupied and represent the same data.
Would the less intuitive dummy encoding likely perform as well as the more intuitive one? (or more generally, do all dummy encodings perform similarly?)
If they don’t yield the same performance, and the problem didn’t have an intuitive solution, is there a way to guide which variable should be omitted in the dummy encoding (assuming the model is much too complicated to try all possibilities)?
Also, in a situation such as the checkers occupancy example where one of the variables in the one-hot encoding would signify the lack of the others (an empty square), could/should this affect how the representation is chosen?
Anyway, thanks for the article! It is always nice to learn the proper terms for what you’ve been working with.
Problem representation is hard, and mostly art.
You want to expose enough information to allow the model to interpret the situation and learn, e.g. offer a gradient for improvement.
For board game representations, perhaps checkout the decades of work in global optimization/genetic algorithms where hard work has been done on how to best represent game state?
Hi sir,
I have a categorical feature in my data. I had one hot encoded that feature and trained a model. I want this model to predict for a new data(single observation). How can i provide this new observation with categorical field to the model.
New data must be prepared the same way as the training data.
Hi Jason,
Suppose i have a data set in which more than two input variable has categorical data type , how can i apply onehotencoder on it and how will we cater dummy variable trap.
Encode each variable one at a time.
I am doing an analysis of Diamond dataset.I have a variable that has levels Fair,good,Excellent.Should I use code like this-stone$cut<-revalue(stone$cut,c("Fair"=1,"Good"=2,"Ideal"=3,"Premium"=4,"Very Good"=5)) after converting it into factor? OR should I use this code-
stone$cut<-factor(stone$cut,ordered=T,levels=c("Fair","Good","Ideal","Premium","Very good"),labels=c(1,2,3,4,5))?
hi
how can i do map the categorical
values to numerical representations.
a) Days of the week
b) Letters of the Alphabet
c) Postal codes
May them anyway you wish, as long as it is consistent.
hi Jason, i wanna ask you about encoding ages so ia, working with sentences i need to estimate age from the sentence so i have used LSTM model but the results were so poor
i have done the POS i code the, using One-Hot representation and also for the ages
i have 78 age possible starting from 15 till 94 so i coded them using one hot the accuracy was 38% so i need to know what has to be done
example: POS = VERB = 10000000000, POS = NOUN = 01000000000
Age = 15-25 = 1000000, Age = 25-35 = 0100000
Interesting. Perhaps search on scholar.google.com for similar problems? You might have to get creative.
Hi Jason, and thanks for all the well-written and useful posts.
I have a quick question. Why would you do integer encoding when you could do a direct one-hot one?
Pandas seems to do that when calling get_dummies() on a categorical variable
Thank you!
Categories that have an ordinal relationship may be more meaningfully represented as integers to some models.
Hi Jason, Do I need to perform encoding when I use categorical features in neural networks? Thanks
Yes, try an integer encoding for ordinal variables, try one hot encoding or an embedding for categorical variables.
Do we need OneHot Encoding when the attribute has only two categorical value?
Generally no, but try it and evaluate the impact on model performance.
Hi,
Are the bits of one hot vectors always comma/space separated or they can be concatenated next to one another like binary numbers? What is their exact nature as per ML theory? I need to know this for my current project.
Thanks.
Each binary variable is normally a separate column.
Hi Jason,
What if you have a one-hot encoding you’d like to feed in to an LSTM? I know Keras expects:
[num_samples, num_timesteps, num_features]
Now suppose each feature in num_features has a one-hot encoding associated with it. Then I would have this shape:
[num_samples, num_timesteps, num_features, one_hot_shape]
Would it be okay to do this:
[num_samples, num_timesteps, num_features*one_hot_shape]
Are there any situations in which this is a bad idea?
No. The one hot encoding creates many features. If you have multiple one hot encoded features as input, then the binary vectors are concatenated as input.
Thank you for the reply. Okay, does that mean I should concatenate the binary vectors that are each one_hot_shape long, so that I have num_features of these one_hot_shape vectors, and then feed that in to the LSTM?
Yes.
Hi Jason,
we are using one-hot encoding in our logistic regression model. How do we interpret coefficients, specifically the ‘estimate’ value in the summary table?
What do you mean by: “estimate value in the summary table”?
Hi jason, Its possible use one-hot encoding for Company_Id in my neural network?
There are 1200 ids, its a lot.
Integer Encoding ponders the company and can skew my model.
What do you recommend?
Yes, try it and compare results to an integer encoding.
Do you have to one hot encode (or use dummy values) on just categorical values that are string s, or do you have to do such with ALL categorical variables? Even is the variables are integers?
You don’t have to, but perhaps compare performance with and without the encoding to confirm it adds value.
If you data such as zipcode in column. Would you recommend using integer encoding or one hot encoding?
Try both, and also try an embedding. I expect the embedding would work better.
Hi Jason,
In the case of Customer Churn, do we need to perform one-hot encoding for monthly data ( say 3 months) and turn them into columns? What happens when there are multiple rows per customer? Do the classifiers like Logistic, DT, Random Forests read this right? Or is there an absolute need to always have one row per customer to achieve the binary classification correctly from these algorithms?
Typically data is denormalized so that you have one row per entity that is being modelled, e.g. one per customer.
Hi Jason,
If all my categorial variables are two-dimensional, then is one-hot encoding neccessary?
It may not be, you could compare a binary input (integer input) to a one hot encoded input.
Hi Jason thank you for the post is very useful.
I have a question I have to present loop characteristics to my model: the features in this case are loop nest level and their size. the loop contains instructions so each loop has a vector of instruction and each instruction has it’s own characteristics
my features would look approximately like this
I really feel lost how to present them especially some of my input are scalar and some are vector and some are id and some are independent from others :!.
I would be very glad for any suggestion. thank you so much :).
Sorry, I don’t have the capacity to review and comment on this.
Generally, I would recommend brainstorming many different framings of the problem, prototype each and see what works well for your specific dataset.
Yes true, I did a kinda of what you said and I reach to summarize my problem on inputs like this
00- I’m using my NN as continuous function to predict a factor for kinda of loop optimization called loop unrolling.
01- I have a variable length of input.
02- I have to give my input at once.
03- RNN will not work fro me for the second reason.
04- If i use MLPs I have to fix the number of input in this case I may process to “0” padding but according to what i read about “0” padding it’s not a good solution and may affect the accuracy when we may have a lot of inputs set to “0” over all the examples of training.
05- Maybe I have to change the structure of features to get fixed number of inputs.
Just that I’m not sure about “0” padding if is that bad :!. Is this solution depend on the nature of the problem I mean for some problem this may be an efficient solution while for some others not ?
Thank you for any suggestion.
If you use a masking layer, the padded values are ignored.
Hi jason
i have a dataset of numeric values some of them are categorical like cities code ,banks code … , should i use encoding or just convert them to category type ?
I recommend trying a few different encodings for each and see what results in the best model performance.
Hi Jason! Great Post! I have a multi-class classification problem where my categories are related, like the “place” example you have provided. The problem is they are related in a 2D environment. My classes are (x,y) pairs on a Cartesian plane. I can assign integers to each position and even use one hot encoding, but I’m afraid that the relationship between coordinates/positions will not be preserved. Is there a solution for this problem? Or is there another way to model this problem other than multi-class classification? Thanks!
Perhaps explore an embedding, perhaps with different resolutions for input?
Hey jason,
what to do when you have about 300 unique values in column for ex : i have city column in which there are about 300 unique cities what should i do in that case.
should i use OneHotEncoder , will that be okay to have that much of columns in dataset.
What should i do in that condition plz explain??
If they are unique for each row, perhaps drop them?
If they are used across more than one row, try an integer encoding, one hot encoding and if you’re using neural nets, perhaps an embedding?
How the hot encoding solves the problem? can you please give me an exact answer?
What problem exactly?
Hi Jason, great content as always.
If I may ask, why don’t we use labels instead of one-hot encoding if we can see a linear relationship between the labels and the dependent variable ?
for example:- if we get a car dealership data and consider the car type data, we find hatchbacks to be the most affordable, then sedans, then maybe trucks, etc. So if we can encode these categories in order of their price buckets, would it be a viable option ?
Machine learning algorithms work with numbers.
A label is a string.
We can model the string as an integer, called integer encoding and this makes sense if the labels have an ordinal relationship, like days of the week.
We can model the string labels using a one hot encoding, which makes more sense for nominal relationship, e.g. car types.
Good Morning Jason,
I have a quick question. I am trying to use KNN algorithm on a data set with both categorical and numerical variables. I have scaled the numerical variables and I have used one-hot encoding for categorical variables. When I am calculating the distance metric, I computed it as follows: when I compare two samples one with Male and the other with Female
Male (1,0)
Female (0,1)
The distance computation is abs((1-0) + (0-1)) = 2.
In some cases, this is not classifying properly. I guess it is because the distance 2 is a very large number when compared to the other distance contributed by numeric features because they are scaled to be between 0 and 1.
Do I need to scale these one hot encoded variables also to avoid this issue and how do I scale them? Please advise
Thanks
Satya
Typically we do not need to scale one hot encoded features.
Thanks Jason for your quick response. One more quick question.
sex_male sex_female
eg1 1 0
eg2 0 1
The distance between eg1 and eg2, would it be 2 because they differ in two bits? Or anything more to be done? Please advise.
Thanks
Satya
It depends on the distance calculation you wish to use.
Should it be square root of 2? because it is diagonal in vector space.
Do you mean euclidean distance?
https://en.wikipedia.org/wiki/Euclidean_distance
Good Morning Jason,
My data set has both categorical and numerical variables as follows – I have used one-hot encoding for sex variable.- trying to calculate the distance between instance_1 and instance_2.
Instance_1
—————-
sex_male 1
sex_female 0
salary 0.4
service 0.6
Instance_2
—————
sex_male 0
sex_female 1
salary 0.6
service 0.7
How do I calculate the distance between these two – I took it as follows
abs(0-1) + abs(1-0) + sqrt((06 -04)^2 + (0.7 – 0.6)^2)
1 + 1 + some small number
So the distance seems to be influenced much more by the categorical variables? Please advise.
Thanks,
Satya
Perhaps try euclidean distance:
https://en.wikipedia.org/wiki/Euclidean_distance
Or Manhattan distance:
https://en.wikipedia.org/wiki/Taxicab_geometry
Hi Jason,
If I use get dummies on the training data, I get new columns as “columnName_cellValues” based on different categories, so I build the model and save it,
Later we load the model and a new test data(only one record), at that point how to get the new columns names in the test data based on its values to match the column names in Model.
Thanks
Data must be encoded the same way. One way to achieve this is to fit the encoding on the training dataset and to save the encoding object for later reuse.
Thanks for the reply,
I am encoding the binary columns using LabelEncoder, then get_dummies for columns with more than 2 unique values, may I know how to save for future please
cat_columns = df.dtypes[df.dtypes == “object”].index
binary_cols = [col for col in cat_columns if len(df[col].unique())==2]
df[binary_cols] = df[binary_cols].apply(lambda col: LabelEncoder().fit_transform(col))
more_than_3_columns = [col for col in cat_columns if len(df[col].unique())>2]
df = pd.get_dummies(df, columns=more_than_3_columns, drop_first=True)
Thanks
Nice work!
You can use pickle to save objects:
https://machinelearningmastery.com/save-load-machine-learning-models-python-scikit-learn/
Hi James I had a question regarding one-hot encoding.
I have a categorical variable called country. It has 149 countries. Creating one hot encoding for large number of countries would impact the performance since most of the values would be 0.
How do you deal with the situation when there is a huge amount of categorical feature values.
Should I go with the simple label encoding?
Thanks
A 150 element vector is not a big deal. I would not worry about it.
I have worked with 50K one hot encoded vectors without incident.
Hi Jason,
Good article.I have 5 dependent variable and each one has more than 5 categories and I have applied one hot encoding technic but I doesn’t know which column should be removed after one hot encoding
The original categorical features (before the encoding) are removed.
Hi Jadon,
thank you for the useful tutorial.
I apply SVM, KNN, Decision tree, Naive Bayes, and random forest to classify Statlog (Heart) Data Set containing sex feature which is male and female.
I used Integer Encoding ie, male=1 and female =1.
is it necessary to use one-hot encoding? if yes, what is the reason in performance?
I am really passionate to know the answer.
Best
Mary
It is a good idea to test diffrent approaches because often our intuition fails.
Hi,
Let’s say I have a column of categorical data with 3 unique values like France, Germany, Spain. So after label encoding and one hot encoding, I get three additional columns that have a combination of 1s and 0s. I read somewhere in the Internet that just label encoding gives the algorithm an impression that the values in the column are related. So we one hot encode. After one hot encoding it gives 3 additional columns of 1s and 0s. How does any machine learning algorithm knows that the values are not related?
They are just inputs to the model and a given model will figure out how to best use them.
A better way may be to use a learned embedding that explicitly relates them.
Jason,
I have 4 categorical columns in my dataset out of which 3 are nominal and 1 is ordinal. My idea is to encode using pd.factorize or preprocessing.LabelEncoder but without any order for 3 nominal and with order for 1 ordinal varaiable (since output results only in one dimension for each column) . I prefer not to go with one hot encoding for nominal variables because it increases dimensionality significantly.
How to control the ordering or the weightage part when using pd.factorize() or preprocessing.LabelEncoder()??
Good question.
It might be easier to perform the mapping your self with some custom code.
But how to ensure the model doesn’t assume order for nominal variables?
If a OHE is used, order will not be assumed.
Increase in dimensionality is not desired in my case and at same time model shouldn’t assume any order for nominal variables.
Problem with pd.factorize and labelencoder is model might assume some order and problem with OHE is increase in dimensionality …..how to overcome both?
You can use an embedding, that is more representative for each category and has lower dimensionality (you can choose it).
Dear Brownlee,
I am a reader of your great blog, but i refereed to this page from here:
https://www.kaggle.com/niyamatalmass/logistic-regression-in-tensorflow-for-beginner
The tutorial refers the reader here for explanation of why they convert numerical features to one-hot encoding before feeding them to LR.
The problem here is that your post just speaks about converting categorical data to one-hot, not speaking about why we need to convert integer values to one-hot.
If the integer encodes a variable that is in fact not ordinal, then a one hot encoding is a good idea.
If it is ordinal, a one hot encoding may still be helpful, it might be worth trying anyway.
As for why the page linked to this post, perhaps contact the author of that page?
Hi Jason,
Nice post!
I have two questions for you.
I applied one-hot encoding to my binary classification problem (say positive example label is [0, 1] and negative example is [1, 0]), then the AUC score is much better than using [1] for positive example and [0] for negative example. Do you know the reason for this?
Secondly, I notice if I use one-hot encoding as well as “roc_curve(y_test.ravel(), y_prob.ravel())”, the AUC can be 0.75. However, if I just use “roc_curve(y_test[:, 0], y_prob[:, 0]), the AUC is only 0.55. Does this mean my model is really poor?
Thank you so much!
Min
That’s interesting. No idea, it should be equivalent.
Not sure what the difference is between the two cases you’re comparing, sorry.
thanks, could you elaborate more on how the binary format of encoding of a variable helps in making sure it doesn’t abnormally influence ML decision.
as in 3 colors get encoded to 0,1,2 thats still a mathematical difference then to binary, which evens them out, i am kind new to this and missing the point on how the binary version evens out the 3 colours so they dont matter.
The encoding is not about modeling the problem, instead it is a way of providing the data to a given model in a consistent way (numbers instead of strings).
Does that help?
Dear Jason,
I really appreciate your post.
memory will increase while reading/parsing one hot encoded data. May i know the reason and how to avoid it. Here is the sample code
from sklearn.preprocessing import OneHotEncoder
enc = OneHotEncoder(handle_unknown=’ignore’)
df = pd.DataFrame(dataframe.iloc[:88000, 1:].values)
temp_df1 = df
enc.fit(temp_df1)
enc_t1=enc.transform(temp_df1).toarray()
temp_df = enc_t1
for data in temp_df:
temp_in=[]
for dt in data:
temp_in.append(dt)
it increase the memory size.
Here, if we comment the following 2 lines
for dt in data:
temp_in.append(dt)
memory does not increase.
Thanks in advance. Please clarify.
Some ideas:
You can use a smaller sample of your data.
You can use a machine with more RAM.
You can experiment with hierarchical one hot encodings.
Hello sir your post is very informative but here i face a problem that the dependent column(target class) of my data set has 38 features now i want to makes it numerical by the get_dummies method but it gives me 38 other columns now when i train a model i get this error that you can not use 38 columns as a target but use one column from this 38 columns.so kindly help me
Perhaps you can use this tutorial as a starting point and adapt it for your dataset:
https://machinelearningmastery.com/machine-learning-in-python-step-by-step/
i have categorical data frame so i need to use One hot encoding but not for all data and for the target for prediction i want to use label encoding can i do that ….
Sounds great.
i use this code to make predication for attack type so i use one hot encoding for 7 attributes and for target i used label encoding
but the data set content more than 180000 row with 8 attributes so this what am did i want to ensure its correct
l = LabelEncoder()
df1_onehot = df1.copy()
df1_onehot = pd.get_dummies(df1_onehot, columns=[‘gname’], prefix = [‘gname’])
df1_onehot = pd.get_dummies(df1_onehot, columns=[‘city’], prefix = [‘city’])
df1_onehot = pd.get_dummies(df1_onehot, columns=[‘region_txt’], prefix = [‘region_txt’])
df1_onehot = pd.get_dummies(df1_onehot, columns=[‘weaptype1_txt’], prefix = [‘weaptype1_txt’])
df1_onehot = pd.get_dummies(df1_onehot, columns=[‘country_txt’], prefix = [‘country_txt’])
#df1_onehot[‘attacktype1_txt’] = l.fit_transform(df1[‘attacktype1_txt’])
print(df1_onehot.head())
# Split-out validation dataset
from sklearn import model_selection
array = df1_onehot.values
X = array[:,0:11372]
Y = array[:,2]
validation_size = 0.20
seed = 4
X_train, X_validation, Y_train, Y_validation = model_selection.train_test_split(X, Y, test_size=validation_size, random_state=seed)
from sklearn.metrics import accuracy_score
from sklearn.linear_model import LogisticRegression
from sklearn.tree import DecisionTreeClassifier
from sklearn.neighbors import KNeighborsClassifier
from sklearn.discriminant_analysis import LinearDiscriminantAnalysis
from sklearn.naive_bayes import GaussianNB
from sklearn.svm import SVC
seed = 7
scoring = ‘accuracy’
models = []
models.append((‘LR’, LogisticRegression(solver=’liblinear’, multi_class=’ovr’)))
#models.append((‘LDA’, LinearDiscriminantAnalysis()))
#models.append((‘KNN’, KNeighborsClassifier()))
#models.append((‘CART’, DecisionTreeClassifier()))
#models.append((‘NB’, GaussianNB()))
#models.append((‘SVM’, SVC(gamma=’auto’)))
# evaluate each model in turn
results = []
names = []
for name, model in models:
kfold = model_selection.KFold(n_splits=10, random_state=seed)
cv_results = model_selection.cross_val_score(model, X_train, Y_train, cv=kfold, scoring=scoring)
results.append(cv_results)
names.append(name)
msg = “%s: %f (%f)” % (name, cv_results.mean(), cv_results.std())
print(msg)
Sorry, I don’t have the capacity to review/debug code.
Perhaps try posting it to stackoverflow?
Hi Jason,
thanks a lot for this article.
What do I do if I have hundreds of categories (product types)? I cannot use one hot encoding, its too many categories.
Thanks
Martin
You could use an embedding, see this tutorial:
https://machinelearningmastery.com/how-to-prepare-categorical-data-for-deep-learning-in-python/
Do we always(in all regression and classification methods) need to exclude one column during onehotencoding to avoid dummy variable trap?
Not in my experience. You one hot encode the variable, and remove the original.
Dear Jason,
Thank for your tutorial.
I have 02 Questions:
1, If an attribute has many categories and the object satisfies more than 01 category at the same time, how could I encode? For example, a story could be classified both “horror” and “happy ending”
2, if an attribute has 03 categories as in your example (red, green, blue), should we denote for 02 categories (for example, red and green) and 01 category (blue) plays as base group to avoid multicollinear (dummy trap)?
Thank you so much in advance?
The model can predict the probability for each category.
There are also so-called multi-label classification tasks where an observation may have multiple categories at once.
Hi Jason,
I love your content. I have a case where i have almost 200 categorical feature columns and none of them are in string/text form. These columns either have 0 or 1 as values or floats. Which technique should be used to deal with such categorical data? Thanking you in advance
Test a few and compare results. Use the methods that result in the best performing model.
Hello Jason,
I have a doubt regarding regression in one hot encoding, can you please help me for the solution
as in regression there are X columns which i am using as a predictor but it contain mix numeric and categorical data,so how I can write the python code logic to ignore numerical column and take categorical for one hot encoding?
how to find an encoding for column ‘Pincode’ contain more that 15000 unique values?
Good question, you can use a ColumnTransformer:
https://machinelearningmastery.com/columntransformer-for-numerical-and-categorical-data/
Hi Jason, Nice article. Have you done a blog on other type of encoding techniques like response encoding(target encoding)? If So, please share the links.
Not at this stage.
When to perform one hot encoding? Do we have to do it before or after train test split?
According to what I found in an article it says,
”split your dataset into train, test and CV datasets, before applying any encoding schemes. Otherwise we will have a data leakage problem. i.e exposing at least a portion of test data to train data”.
Whereas some other articles suggest to perform one hot encoding before train test split.
Thanks
San
Depends on the other operations you want to perform, e.g like feature selection.
In simple cases it is performed first.
Should one hot vectors be scaled with numerical attributes ?
No, one hot vectors do not require scaling.
hi jason, do w need to convert categorical to numbers before splitting into training and test ?
forgot to mention, i am doing it for a logistic regression model
Before or after would be fine as long as it is consistent.
Hi, very helpful tutorial. Thank you. I have a doubt about using one hot encoding with a categorical data that has just two labels. It is possible in that case to use just one feature in the data with either 0 or 1? Or i have to use [1,0] and [0,1]?
You’re welcome.
Agreed. Perhaps compare a one hot encoding vs ordinal encoding with a model?
You articles help me a lot in every day! I have on question regarding one hot encoding and pd.get_dummies. Do you have the same outcome on the dataset?
What I am facing right now are 5 different services provided to a large group of customers. I am trying to make a model based on past customers what service would be the best fit for an existing customer.
After i do the pd_dummies i get the column that had 5 services divided in 4 column.
So i decide my X_train in my data set but how do 1 decide which is the y in my data set if i have 4 different columns now?
Sorry for being long and thank you for everything.
You’re welcome.
No, dummies has c-1 binary vars, one hot has c binary vars, where c is the number of classes.
Use dummy for linear model, use one hot for everything else.
I used hot encoding for my data set { 47000 * 57 } 57 columns of categorical data …and then applied CNN for the prediction , but in any case, MAE is not getting less than 8.5 . How I can improve it?
Here are some suggestions for improving neural network models:
https://machinelearningmastery.com/improve-deep-learning-performance/
Hello Jason
What if I have a categorical numerical feature. For instance if I have a feature like “Number of Doors” and it consists of values only the values (3, 4, 5 ) but each of these values keeps on repeating itself. In that case should one One-hot encoding .
You could compare leaving the field as is vs a one hot encoding and see which representation results in a better performing model.
I would think leaving the field as is would be sufficient.
The important point and real benefit of using one hot coding is to avoid confusion by ML. converting categorical value to numerical for e.g. A=1, B=2, C=3 and D = 4.
The problem with this solution is that ML algorithm may think 4 is greater than 1, which could cause problem.
This is where “one hot coding” comes to rescue.
Agreed!
Hello Mr Brownlee
Thanks for the article.
Wondering if you could help me with a problem i encountered when i tried to use OneHotEncoding. I am trying to transform a single column within my dataset comprising of company names. This ois the code I used
#Hot encoding the company name colum
from sklearn.compose import ColumnTransformer
from sklearn.preprocessing import OneHotEncoder
ct = ColumnTransformer(transformers=[(‘encoder’, OneHotEncoder(), [1])], remainder=’passthrough’)
X = np.array(ct.fit_transform(X))
but i received this error:
ValueError: could not convert string to float: ‘FLANG Group’
‘FLANG GROUP’ is just one of the company names. All companies are in the same format. In my csv file they are labeled as text.
how do I solve this?
your help will be highly appreciated.
Are you sure you’re specifying the right column index?
Yes, because the error is referring to one of the company names which i am trying to OneHotEncode
It can also be helpful to inspect the loaded data to confirm.
Also, perhaps run a small test with the OneHotEncoder with contrived strings in memory like “company one”, “company “two” and confirm your code works with them.
thank you for sharing this document but still now i can’t understand how one hot encoding is work for news text classification i have 10 category or label types
please help me how to convert categorical data in to numerical one I wat your comment
Here is an example:
https://machinelearningmastery.com/one-hot-encoding-for-categorical-data/
Thank you for sharing this document, I have a scenario where I have numerical columns with many repetitions for ex: multiple rows with the same numerical values. There are about 50 unique numerical values so directly one-hot encoding would add a lot of columns. If left the way it is, as stated above, the model could interpret say 25 as more than 10.
Any ideas on how this could be dealt with? I’d appreciate any suggestions you could help with.
Thanks in advance!
Perhaps try modeling the integers directly?
Hi Jason,
Thank you always for your wonderful explanations, I have a question, so I’m doing a regression task on an industrial data (it looks bad) ,so after the preprocessing and cleaning, I used multiple models but I got low performances… Do you have some experiences with bad datasets and how to handle this problem…
Thanks for advance
You’re welcome.
This may give you ideas:
https://machinelearningmastery.com/machine-learning-performance-improvement-cheat-sheet/
Hi Jason!
I am pretty confused what encoder should I use. For example if my data have no ordinal relationship, why would I use onehotencoder over labelencoder, and wouldn’t onehotencoder also generate a ton of features?
And if I have some data that have ordinal relationships, can’t I just use the ordinalencoder(from sklearn)? Isn’t the ordinalencoder best for encoding features with ordinal relationships
Can onehotencoder enocde ordinal relationships? And why would I use if there are ordinal relationships?
I don’t understand onehotencoder’s advantages over labelenocder and ordinalencoder, so please tell me, thank you!
It might be the case that even if you have an ordinal relationship in your data, that the model you choose works better with an embedding or one hot (or the reverse case). I recommend experimenting to discover what works best for your data and model/s.
Ok, thank you!
But how can I find the best encoding algorithm for my data? Or a more general question is that is there a way to search for a hyperparameter that is not a model’s hyperparameters, but it is a specific algorithm, like gridseaerching either a model using onehotencoder on the data has a better performance than a model using ordinalencoder on the data. I don’t really know these algorithms that will contribute to a model performance will count as hyperparameters, but can we use for example GridSearchCV to search for a model’s performance on for example onehotencoder vs ordinalencoder? The idea is that can we use hyperparameter search on for example which type of encoder to use, or which type of scaler to use, or which type of dimensionality reduction technique to use; measuring the model performance with these different types of these algorithms on the data. Is there a possible way to search for these ‘hyperparameter’ ?
Perhaps try a few approaches and use the method that results in the best performance for your data and model.
Wow great post!
I have one question.
I have 11 columns: 10 columns used to predict and 1 column that is the target.
De target columsn represents if the pacient will be absent or present to the appointment.
1=present 0=absent.
Should I apply on hot encodding to this target? I mean…. is binary. have any sense do that in this case?
Thanks!!
No.
How can we implement one hot encoding in LSTM. I have data and i want to use OHE for the days on the week. Making it 8 columns. How can i put this alltogether for my prediction
Hi Raphael…You may find the following of interest:
https://datascience.stackexchange.com/questions/45803/should-we-use-only-one-hot-vector-for-lstm-input-outputs
Thanks for your response. But after a lot of reading. I want to rephrase my question.
For LSTM time series prediction. How can one combine numerical and categorical values (which will be one hot encoded) in a vector as input for LSTM?
Hi Raphael…You will reshape the data as shown in the following tutorials:
https://machinelearningmastery.com/how-to-load-visualize-and-explore-a-complex-multivariate-multistep-time-series-forecasting-dataset/
https://machinelearningmastery.com/how-to-develop-rnn-models-for-human-activity-recognition-time-series-classification/
Thanks. But after we one hot encode, how do we put the data together to train the model.
I am working on power prediction using time series LSTM. I have implemented the model and my predictions were okay. Now i want to consider adding the weekdays for each time series so i’ll get a better power prediction, because each day (Mon to Fri) has it own effect on the power even at different time. I one hot encoded the week days and i now have 8 columns (Power and 7 days of the week). I have generated a sequence but i get alot of errors.
I’m yet to get the best practise on solving this type of question.
Hi Bobby…Do you have any specific questions that I may address?
Hi, do you have any references I could add to my thesis? Any good article comparing one hot encoding and non coded data?
Thanks in advance.
Hi Pau…You may find the following of interest:
https://www.researchgate.net/publication/320465713_A_Comparative_Study_of_Categorical_Variable_Encoding_Techniques_for_Neural_Network_Classifiers
https://ieeexplore.ieee.org/document/9512057
Do you know any article which go deeper on null values treatment?
Thanks for your attention and dedication.