Sequence classification is a predictive modeling problem where you have some sequence of inputs over space or time, and the task is to predict a category for the sequence.
This problem is difficult because the sequences can vary in length, comprise a very large vocabulary of input symbols, and may require the model to learn the long-term context or dependencies between symbols in the input sequence.
In this post, you will discover how you can develop LSTM recurrent neural network models for sequence classification problems in Python using the Keras deep learning library.
After reading this post, you will know:
- How to develop an LSTM model for a sequence classification problem
- How to reduce overfitting in your LSTM models through the use of dropout
- How to combine LSTM models with Convolutional Neural Networks that excel at learning spatial relationships
Kick-start your project with my new book Deep Learning for Natural Language Processing, including step-by-step tutorials and the Python source code files for all examples.
Let’s get started.
- Jul/2016: First published
- Update Oct/2016: Updated examples for Keras 1.1.0 andTensorFlow 0.10.0
- Update Mar/2017: Updated example for Keras 2.0.2, TensorFlow 1.0.1 and Theano 0.9.0
- Update May/2018: Updated code to use the most recent Keras API, thanks Jeremy Rutman
- Update Jul/2022: Updated code for TensorFlow 2.x and added an example to use bidirectional LSTM
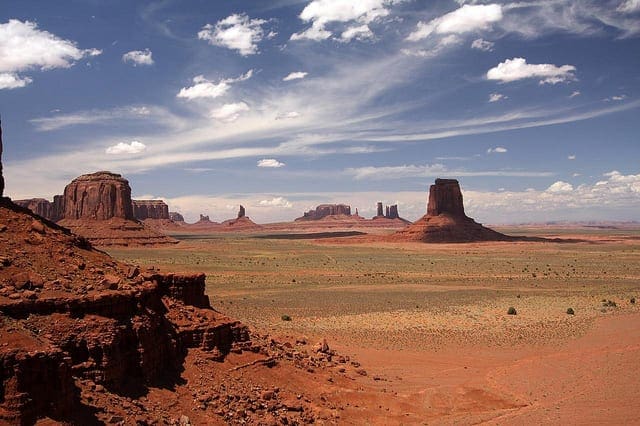
Sequence classification with LSTM recurrent neural networks in Python with Keras
Photo by photophilde, some rights reserved.
Problem Description
The problem that you will use to demonstrate sequence learning in this tutorial is the IMDB movie review sentiment classification problem. Each movie review is a variable sequence of words, and the sentiment of each movie review must be classified.
The Large Movie Review Dataset (often referred to as the IMDB dataset) contains 25,000 highly polar movie reviews (good or bad) for training and the same amount again for testing. The problem is to determine whether a given movie review has a positive or negative sentiment.
The data was collected by Stanford researchers and used in a 2011 paper where a 50/50 split of the data was used for training and testing. An accuracy of 88.89% was achieved.
Keras provides built-in access to the IMDB dataset. The imdb.load_data() function allows you to load the dataset in a format ready for use in neural networks and deep learning models.
The words have been replaced by integers that indicate the ordered frequency of each word in the dataset. The sentences in each review are therefore comprised of a sequence of integers.
Word Embedding
You will map each movie review into a real vector domain, a popular technique when working with text—called word embedding. This is a technique where words are encoded as real-valued vectors in a high dimensional space, where the similarity between words in terms of meaning translates to closeness in the vector space.
Keras provides a convenient way to convert positive integer representations of words into a word embedding by an Embedding layer.
You will map each word onto a 32-length real valued vector. You will also limit the total number of words that you are interested in modeling to the 5000 most frequent words and zero out the rest. Finally, the sequence length (number of words) in each review varies, so you will constrain each review to be 500 words, truncating long reviews and padding the shorter reviews with zero values.
Now that you have defined your problem and how the data will be prepared and modeled, you are ready to develop an LSTM model to classify the sentiment of movie reviews.
Need help with LSTMs for Sequence Prediction?
Take my free 7-day email course and discover 6 different LSTM architectures (with code).
Click to sign-up and also get a free PDF Ebook version of the course.
Simple LSTM for Sequence Classification
You can quickly develop a small LSTM for the IMDB problem and achieve good accuracy.
Let’s start by importing the classes and functions required for this model and initializing the random number generator to a constant value to ensure you can easily reproduce the results.
1 2 3 4 5 6 7 8 9 |
import tensorflow as tf from tensorflow.keras.datasets import imdb from tensorflow.keras.models import Sequential from tensorflow.keras.layers import Dense from tensorflow.keras.layers import LSTM from tensorflow.keras.layers import Embedding from tensorflow.keras.preprocessing import sequence # fix random seed for reproducibility tf.random.set_seed(7) |
You need to load the IMDB dataset. You are constraining the dataset to the top 5,000 words. You will also split the dataset into train (50%) and test (50%) sets.
1 2 3 |
# load the dataset but only keep the top n words, zero the rest top_words = 5000 (X_train, y_train), (X_test, y_test) = imdb.load_data(num_words=top_words) |
Next, you need to truncate and pad the input sequences, so they are all the same length for modeling. The model will learn that the zero values carry no information. The sequences are not the same length in terms of content, but same-length vectors are required to perform the computation in Keras.
1 2 3 4 |
# truncate and pad input sequences max_review_length = 500 X_train = sequence.pad_sequences(X_train, maxlen=max_review_length) X_test = sequence.pad_sequences(X_test, maxlen=max_review_length) |
You can now define, compile and fit your LSTM model.
The first layer is the Embedded layer that uses 32-length vectors to represent each word. The next layer is the LSTM layer with 100 memory units (smart neurons). Finally, because this is a classification problem, you will use a Dense output layer with a single neuron and a sigmoid activation function to make 0 or 1 predictions for the two classes (good and bad) in the problem.
Because it is a binary classification problem, log loss is used as the loss function (binary_crossentropy in Keras). The efficient ADAM optimization algorithm is used. The model is fit for only two epochs because it quickly overfits the problem. A large batch size of 64 reviews is used to space out weight updates.
1 2 3 4 5 6 7 8 9 |
# create the model embedding_vecor_length = 32 model = Sequential() model.add(Embedding(top_words, embedding_vecor_length, input_length=max_review_length)) model.add(LSTM(100)) model.add(Dense(1, activation='sigmoid')) model.compile(loss='binary_crossentropy', optimizer='adam', metrics=['accuracy']) print(model.summary()) model.fit(X_train, y_train, validation_data=(X_test, y_test), epochs=3, batch_size=64) |
Once fit, you can estimate the performance of the model on unseen reviews.
1 2 3 |
# Final evaluation of the model scores = model.evaluate(X_test, y_test, verbose=0) print("Accuracy: %.2f%%" % (scores[1]*100)) |
For completeness, here is the full code listing for this LSTM network on the IMDB dataset.
1 2 3 4 5 6 7 8 9 10 11 12 13 14 15 16 17 18 19 20 21 22 23 24 25 26 27 28 29 |
# LSTM for sequence classification in the IMDB dataset import tensorflow as tf from tensorflow.keras.datasets import imdb from tensorflow.keras.models import Sequential from tensorflow.keras.layers import Dense from tensorflow.keras.layers import LSTM from tensorflow.keras.layers import Embedding from tensorflow.keras.preprocessing import sequence # fix random seed for reproducibility tf.random.set_seed(7) # load the dataset but only keep the top n words, zero the rest top_words = 5000 (X_train, y_train), (X_test, y_test) = imdb.load_data(num_words=top_words) # truncate and pad input sequences max_review_length = 500 X_train = sequence.pad_sequences(X_train, maxlen=max_review_length) X_test = sequence.pad_sequences(X_test, maxlen=max_review_length) # create the model embedding_vecor_length = 32 model = Sequential() model.add(Embedding(top_words, embedding_vecor_length, input_length=max_review_length)) model.add(LSTM(100)) model.add(Dense(1, activation='sigmoid')) model.compile(loss='binary_crossentropy', optimizer='adam', metrics=['accuracy']) print(model.summary()) model.fit(X_train, y_train, epochs=3, batch_size=64) # Final evaluation of the model scores = model.evaluate(X_test, y_test, verbose=0) print("Accuracy: %.2f%%" % (scores[1]*100)) |
Note: Your results may vary given the stochastic nature of the algorithm or evaluation procedure, or differences in numerical precision. Consider running the example a few times and compare the average outcome.
Running this example produces the following output.
1 2 3 4 5 6 7 |
Epoch 1/3 391/391 [==============================] - 124s 316ms/step - loss: 0.4525 - accuracy: 0.7794 Epoch 2/3 391/391 [==============================] - 124s 318ms/step - loss: 0.3117 - accuracy: 0.8706 Epoch 3/3 391/391 [==============================] - 126s 323ms/step - loss: 0.2526 - accuracy: 0.9003 Accuracy: 86.83% |
You can see that this simple LSTM with little tuning achieves near state-of-the-art results on the IMDB problem. Importantly, this is a template that you can use to apply LSTM networks to your own sequence classification problems.
Now, let’s look at some extensions of this simple model that you may also want to bring to your own problems.
LSTM for Sequence Classification with Dropout
Recurrent neural networks like LSTM generally have the problem of overfitting.
Dropout can be applied between layers using the Dropout Keras layer. You can do this easily by adding new Dropout layers between the Embedding and LSTM layers and the LSTM and Dense output layers. For example:
1 2 3 4 5 6 |
model = Sequential() model.add(Embedding(top_words, embedding_vecor_length, input_length=max_review_length)) model.add(Dropout(0.2)) model.add(LSTM(100)) model.add(Dropout(0.2)) model.add(Dense(1, activation='sigmoid')) |
The full code listing example above with the addition of Dropout layers is as follows:
1 2 3 4 5 6 7 8 9 10 11 12 13 14 15 16 17 18 19 20 21 22 23 24 25 26 27 28 29 30 31 32 |
# LSTM with Dropout for sequence classification in the IMDB dataset import tensorflow as tf from tensorflow.keras.datasets import imdb from tensorflow.keras.models import Sequential from tensorflow.keras.layers import Dense from tensorflow.keras.layers import LSTM from tensorflow.keras.layers import Dropout from tensorflow.keras.layers import Embedding from tensorflow.keras.preprocessing import sequence # fix random seed for reproducibility tf.random.set_seed(7) # load the dataset but only keep the top n words, zero the rest top_words = 5000 (X_train, y_train), (X_test, y_test) = imdb.load_data(num_words=top_words) # truncate and pad input sequences max_review_length = 500 X_train = sequence.pad_sequences(X_train, maxlen=max_review_length) X_test = sequence.pad_sequences(X_test, maxlen=max_review_length) # create the model embedding_vecor_length = 32 model = Sequential() model.add(Embedding(top_words, embedding_vecor_length, input_length=max_review_length)) model.add(Dropout(0.2)) model.add(LSTM(100)) model.add(Dropout(0.2)) model.add(Dense(1, activation='sigmoid')) model.compile(loss='binary_crossentropy', optimizer='adam', metrics=['accuracy']) print(model.summary()) model.fit(X_train, y_train, epochs=3, batch_size=64) # Final evaluation of the model scores = model.evaluate(X_test, y_test, verbose=0) print("Accuracy: %.2f%%" % (scores[1]*100)) |
Note: Your results may vary given the stochastic nature of the algorithm or evaluation procedure, or differences in numerical precision. Consider running the example a few times and compare the average outcome.
Running this example provides the following output.
1 2 3 4 5 6 7 |
Epoch 1/3 391/391 [==============================] - 117s 297ms/step - loss: 0.4721 - accuracy: 0.7664 Epoch 2/3 391/391 [==============================] - 125s 319ms/step - loss: 0.2840 - accuracy: 0.8864 Epoch 3/3 391/391 [==============================] - 135s 346ms/step - loss: 0.3022 - accuracy: 0.8772 Accuracy: 85.66% |
You can see dropout having the desired impact on training with a slightly slower trend in convergence and, in this case, a lower final accuracy. The model could probably use a few more epochs of training and may achieve a higher skill (try it and see).
Alternately, dropout can be applied to the input and recurrent connections of the memory units with the LSTM precisely and separately.
Keras provides this capability with parameters on the LSTM layer, the dropout for configuring the input dropout, and recurrent_dropout for configuring the recurrent dropout. For example, you can modify the first example to add dropout to the input and recurrent connections as follows:
1 2 3 4 |
model = Sequential() model.add(Embedding(top_words, embedding_vecor_length, input_length=max_review_length)) model.add(LSTM(100, dropout=0.2, recurrent_dropout=0.2)) model.add(Dense(1, activation='sigmoid')) |
The full code listing with more precise LSTM dropout is listed below for completeness.
1 2 3 4 5 6 7 8 9 10 11 12 13 14 15 16 17 18 19 20 21 22 23 24 25 26 27 28 29 |
# LSTM with dropout for sequence classification in the IMDB dataset import tensorflow as tf from tensorflow.keras.datasets import imdb from tensorflow.keras.models import Sequential from tensorflow.keras.layers import Dense from tensorflow.keras.layers import LSTM from tensorflow.keras.layers import Embedding from tensorflow.keras.preprocessing import sequence # fix random seed for reproducibility tf.random.set_seed(7) # load the dataset but only keep the top n words, zero the rest top_words = 5000 (X_train, y_train), (X_test, y_test) = imdb.load_data(num_words=top_words) # truncate and pad input sequences max_review_length = 500 X_train = sequence.pad_sequences(X_train, maxlen=max_review_length) X_test = sequence.pad_sequences(X_test, maxlen=max_review_length) # create the model embedding_vecor_length = 32 model = Sequential() model.add(Embedding(top_words, embedding_vecor_length, input_length=max_review_length)) model.add(LSTM(100, dropout=0.2, recurrent_dropout=0.2)) model.add(Dense(1, activation='sigmoid')) model.compile(loss='binary_crossentropy', optimizer='adam', metrics=['accuracy']) print(model.summary()) model.fit(X_train, y_train, epochs=3, batch_size=64) # Final evaluation of the model scores = model.evaluate(X_test, y_test, verbose=0) print("Accuracy: %.2f%%" % (scores[1]*100)) |
Note: Your results may vary given the stochastic nature of the algorithm or evaluation procedure, or differences in numerical precision. Consider running the example a few times and compare the average outcome.
Running this example provides the following output.
1 2 3 4 5 6 7 |
Epoch 1/3 391/391 [==============================] - 220s 560ms/step - loss: 0.4605 - accuracy: 0.7784 Epoch 2/3 391/391 [==============================] - 219s 560ms/step - loss: 0.3158 - accuracy: 0.8773 Epoch 3/3 391/391 [==============================] - 219s 559ms/step - loss: 0.2734 - accuracy: 0.8930 Accuracy: 86.78% |
You can see that the LSTM-specific dropout has a more pronounced effect on the convergence of the network than the layer-wise dropout. Like above, the number of epochs was kept constant and could be increased to see if the skill of the model could be further lifted.
Dropout is a powerful technique for combating overfitting in your LSTM models, and it is a good idea to try both methods. Still, you may get better results with the gate-specific dropout provided in Keras.
Bidirectional LSTM for Sequence Classification
Sometimes, a sequence is better used in reversed order. In those cases, you can simply reverse a vector x
using the Python syntax x[::-1]
before using it to train your LSTM network.
Sometimes, neither the forward nor the reversed order works perfectly, but combining them will give better results. In this case, you will need a bidirectional LSTM network.
A bidirectional LSTM network is simply two separate LSTM networks; one feeds with a forward sequence and another with reversed sequence. Then the output of the two LSTM networks is concatenated together before being fed to the subsequent layers of the network. In Keras, you have the function Bidirectional()
to clone an LSTM layer for forward-backward input and concatenate their output. For example,
1 2 3 4 |
model = Sequential() model.add(Embedding(top_words, embedding_vecor_length, input_length=max_review_length)) model.add(Bidirectional(LSTM(100, dropout=0.2, recurrent_dropout=0.2))) model.add(Dense(1, activation='sigmoid')) |
Since you created not one, but two LSTMs with 100 units each, this network will take twice the amount of time to train. Depending on the problem, this additional cost may be justified.
The full code listing with adding the bidirectional LSTM to the last example is listed below for completeness.
1 2 3 4 5 6 7 8 9 10 11 12 13 14 15 16 17 18 19 20 21 22 23 24 25 26 27 28 29 30 |
# LSTM with dropout for sequence classification in the IMDB dataset import tensorflow as tf from tensorflow.keras.datasets import imdb from tensorflow.keras.models import Sequential from tensorflow.keras.layers import Dense from tensorflow.keras.layers import LSTM from tensorflow.keras.layers import Bidirectional from tensorflow.keras.layers import Embedding from tensorflow.keras.preprocessing import sequence # fix random seed for reproducibility tf.random.set_seed(7) # load the dataset but only keep the top n words, zero the rest top_words = 5000 (X_train, y_train), (X_test, y_test) = imdb.load_data(num_words=top_words) # truncate and pad input sequences max_review_length = 500 X_train = sequence.pad_sequences(X_train, maxlen=max_review_length) X_test = sequence.pad_sequences(X_test, maxlen=max_review_length) # create the model embedding_vecor_length = 32 model = Sequential() model.add(Embedding(top_words, embedding_vecor_length, input_length=max_review_length)) model.add(Bidirectional(LSTM(100, dropout=0.2, recurrent_dropout=0.2))) model.add(Dense(1, activation='sigmoid')) model.compile(loss='binary_crossentropy', optimizer='adam', metrics=['accuracy']) print(model.summary()) model.fit(X_train, y_train, epochs=3, batch_size=64) # Final evaluation of the model scores = model.evaluate(X_test, y_test, verbose=0) print("Accuracy: %.2f%%" % (scores[1]*100)) |
Note: Your results may vary given the stochastic nature of the algorithm or evaluation procedure, or differences in numerical precision. Consider running the example a few times and compare the average outcome.
Running this example provides the following output.
1 2 3 4 5 6 7 |
Epoch 1/3 391/391 [==============================] - 405s 1s/step - loss: 0.4960 - accuracy: 0.7532 Epoch 2/3 391/391 [==============================] - 439s 1s/step - loss: 0.3075 - accuracy: 0.8744 Epoch 3/3 391/391 [==============================] - 430s 1s/step - loss: 0.2551 - accuracy: 0.9014 Accuracy: 87.69% |
It seems you can only get a slight improvement but with a significantly longer training time.
LSTM and Convolutional Neural Network for Sequence Classification
Convolutional neural networks excel at learning the spatial structure in input data.
The IMDB review data does have a one-dimensional spatial structure in the sequence of words in reviews, and the CNN may be able to pick out invariant features for the good and bad sentiment. This learned spatial feature may then be learned as sequences by an LSTM layer.
You can easily add a one-dimensional CNN and max pooling layers after the Embedding layer, which then feeds the consolidated features to the LSTM. You can use a smallish set of 32 features with a small filter length of 3. The pooling layer can use the standard length of 2 to halve the feature map size.
For example, you would create the model as follows:
1 2 3 4 5 6 |
model = Sequential() model.add(Embedding(top_words, embedding_vecor_length, input_length=max_review_length)) model.add(Conv1D(filters=32, kernel_size=3, padding='same', activation='relu')) model.add(MaxPooling1D(pool_size=2)) model.add(LSTM(100)) model.add(Dense(1, activation='sigmoid')) |
The full code listing with CNN and LSTM layers is listed below for completeness.
1 2 3 4 5 6 7 8 9 10 11 12 13 14 15 16 17 18 19 20 21 22 23 24 25 26 27 28 29 30 31 32 33 |
# LSTM and CNN for sequence classification in the IMDB dataset import tensorflow as tf from tensorflow.keras.datasets import imdb from tensorflow.keras.models import Sequential from tensorflow.keras.layers import Dense from tensorflow.keras.layers import LSTM from tensorflow.keras.layers import Conv1D from tensorflow.keras.layers import MaxPooling1D from tensorflow.keras.layers import Embedding from tensorflow.keras.preprocessing import sequence # fix random seed for reproducibility tf.random.set_seed(7) # load the dataset but only keep the top n words, zero the rest top_words = 5000 (X_train, y_train), (X_test, y_test) = imdb.load_data(num_words=top_words) # truncate and pad input sequences max_review_length = 500 X_train = sequence.pad_sequences(X_train, maxlen=max_review_length) X_test = sequence.pad_sequences(X_test, maxlen=max_review_length) # create the model embedding_vecor_length = 32 model = Sequential() model.add(Embedding(top_words, embedding_vecor_length, input_length=max_review_length)) model.add(Conv1D(filters=32, kernel_size=3, padding='same', activation='relu')) model.add(MaxPooling1D(pool_size=2)) model.add(LSTM(100)) model.add(Dense(1, activation='sigmoid')) model.compile(loss='binary_crossentropy', optimizer='adam', metrics=['accuracy']) print(model.summary()) model.fit(X_train, y_train, epochs=3, batch_size=64) # Final evaluation of the model scores = model.evaluate(X_test, y_test, verbose=0) print("Accuracy: %.2f%%" % (scores[1]*100)) |
Note: Your results may vary given the stochastic nature of the algorithm or evaluation procedure, or differences in numerical precision. Consider running the example a few times and compare the average outcome.
Running this example provides the following output.
1 2 3 4 5 6 7 |
Epoch 1/3 391/391 [==============================] - 65s 163ms/step - loss: 0.4213 - accuracy: 0.7950 Epoch 2/3 391/391 [==============================] - 66s 168ms/step - loss: 0.2490 - accuracy: 0.9026 Epoch 3/3 391/391 [==============================] - 73s 188ms/step - loss: 0.1979 - accuracy: 0.9261 Accuracy: 88.45% |
You can see that you achieve slightly better results than the first example, although with fewer weights and faster training time.
You might expect that even better results could be achieved if this example was further extended to use dropout.
Resources
Below are some resources if you are interested in diving deeper into sequence prediction or this specific example.
- Theano tutorial for LSTMs applied to the IMDB dataset
- Keras code example for using an LSTM and CNN with LSTM on the IMDB dataset.
- Supervised Sequence Labelling with Recurrent Neural Networks, 2012 book by Alex Graves (and PDF preprint).
Summary
In this post, you discovered how to develop LSTM network models for sequence classification predictive modeling problems.
Specifically, you learned:
- How to develop a simple single-layer LSTM model for the IMDB movie review sentiment classification problem
- How to extend your LSTM model with layer-wise and LSTM-specific dropout to reduce overfitting
- How to combine the spatial structure learning properties of a Convolutional Neural Network with the sequence learning of an LSTM
Do you have any questions about sequence classification with LSTMs or this post? Ask your questions in the comments, and I will do my best to answer.
It’s geat!
Thanks Atlant.
How do you get to the 16,750? 25,000/64 batches is 390.
Thanks!
I am confused about LSTM input/output dimensions, specifically in keras library. How do keras return 2D output while its input is 3D? I know it can return 3D output using “return_sequence = Trure,” but if return_sequence = False, how can it deal with 3D and produces 2D output? For example, if input data of shape (32, 16, 20), 32 batch size, 16 timestep, 20 features, and output of shape (32, 100), 32 batch size, 100 hidden states; how keras processes input of 3d and returns output 2d. Additionally, how can concatenate input and hidden state if they don’t have the exact dimensions?
Hi Hajar…You may find the following helpful:
https://machinelearningmastery.com/reshape-input-data-long-short-term-memory-networks-keras/
Hi, I have a question if anyone can answer that. I have tabuler data. Mulltiple colums i.e 4 columns, have text data. just like here. https://github.com/IBM/KPA_2021_shared_task. How i will create that tabuler data into metrixes and apply lstm model on it.
LSTMs are not appropriate for tabular data, they require sequence data.
This may help:
https://machinelearningmastery.com/faq/single-faq/what-is-the-difference-between-samples-timesteps-and-features-for-lstm-input
Hey Jason,
Congrats brother, for continuous great and easy to adapt/understanding lessons. I am just curious to know unsupervised and reinforced neural nets, any tutorials you have?
Regards,
Sahil
Thanks Sahil.
Sorry, no tutorials on unsupervised learning or reinforcement learning with neural nets just yet. Soon though.
Hi, great stuff you are publishing here thanks.
Would this network architecture work for predicting profitability of a stock based time series data of the stock price.
For example with data samples of daily stock prices and trading volumes with 5 minute intervals from 9.30am to 1pm paired with YES or NO to the stockprice increasing by more than 0.5% the rest of the trading day?
Each trading day is one sample and th3 entire data set woule for example the last 1000 trading days.
If this network architecture is not suitable what other would you suggest testing our?
Again thanks for this super resdource.
Thanks Søren.
Sure, it would be worth trying, but I am not an expert on the stock market.
So, the end result of this tutorial is a model. Could you give me an example how to use this model to predict a new review, especially using new vocabularies that don’t present in training data? Many thanks..
I don’t have an example Naufal, but the new example would have to encode words using the same integers and embed the integers into the same word mapping.
Thanks Jason for excellent article.
to predict i did below things, please correct i am did wrong. you said to embed..i didnt get that. how to do that.
text = numpy.array([‘this is excellent sentence’])
#print(text.shape)
tk = keras.preprocessing.text.Tokenizer( nb_words=2000, lower=True,split=” “)
tk.fit_on_texts(text)
prediction = model.predict(numpy.array(tk.texts_to_sequences(text)))
print(prediction)
Thanks Jason for excellent article.
to predict i did below things, please correct i am did wrong. you said to embed..i didnt get that. how to do that.
text = numpy.array([‘this is excellent sentence’])
#print(text.shape)
tk = keras.preprocessing.text.Tokenizer( nb_words=2000, lower=True,split=” “)
tk.fit_on_texts(text)
prediction = model.predict(sequence.pad_sequences(tk.texts_to_sequences(text),maxlen=max_review_length))
print(prediction)
You can use below code to predict sentiment of new reviews..
However, it will simply skip words out of its vocabulary..
Also, you can try increasing “top_words” value before training so that u can cover more number of words.
Thanks for sharing!
Embed refers to the word embedding layer:
https://keras.io/layers/embeddings/
def conv_to_proper_format(sentence):
>>sentence=text.text_to_word_sequence(sentence,filters=’!”#$%&()*+,-./:;?@[\\]^_`{|}~\t\n’,lower=True,split=” “)
>>sentence=numpy.array([word_index[word] if word in word_index else 0 for word in sentence])#Encoding into sequence of integers
>>sentence[sentence>5000]=2
>>L=500-len(sentence)
>>sentence=numpy.pad(sentence, (L,0), ‘constant’)
>>sentence=sentence.reshape(1,-1)
>>return sentence
Use this function on ur review to convert into proper format and then model.predict(review1) will give u answer.
Hello Jason! Great tutorials!
When I attempt this tutorial, I get the error message from imdb.load_data :
TypeError: load_data() got an unexpected keyword argument ‘test_split’
I tried copying and pasting the entire source code but this line still had the same error.
Can you think of any underlying reason that this is not executing for me?
Sorry to hear that Joey. It looks like a change with Keras v1.0.7.
I get the same error if I run with version 1.0.7. I can see the API doco still refers to the test_split argument here: https://keras.io/datasets/#imdb-movie-reviews-sentiment-classification
I can see that the argument was removed from the function here:
https://github.com/fchollet/keras/blob/master/keras/datasets/imdb.py
Option 1) You can remove the argument from the function to use the default test 50/50 split.
Option 2) You can downgrade Keras to version 1.0.6:
Remember you can check your Keras version on the command line with:
I will look at updating the example to be compatible with the latest Keras.
I got it working! Thanks so much for all of the help Jason!
Glad to hear it Joey.
I have updated the examples in the post to match Keras 1.1.0 and TensorFlow 0.10.0.
Hi, Jason.
A quick question:
Based on my understanding, padding zero in front is like labeling ‘START’. Otherwise it is like labeling ‘END’. How should I decide ‘pre’ padding or ‘post’ padding? Does it matter?
Thanks.
I don’t think I understand the question, sorry Chong.
Consider trying both padding approaches on your problem and see what works best.
Hi, Jason.
Thanks for your reply.
I have another quick question in section “LSTM For Sequence Classification With Dropout”.
model.add(Embedding(top_words, embedding_vector_length, input_length=max_review_length, dropout=0.2))
model.add(Dropout(0.2))
…
Here I see two dropout layers. The second one is easy to understand: For each time step, It just randomly deactivates 20% numbers in the output embedding vector.
The first one confuses me: Does it do dropout on the input? For each time step, the input of the embedding layers should be only one index of the top words. In other words, the input is one single number. How can we dropout it? (Or do you mean drop the input indices of 20% time steps?)
Great question, I believe it drops out weights from the input nodes from the embedded layer to the hidden layer.
You can learn more about dropout here:
https://machinelearningmastery.com/dropout-regularization-deep-learning-models-keras/
Can the dropout applied in the Embedding layer be thought of as randomly removing a word in a sentence and forcing the classification not to rely on any word?
I don’t see why not – off the cuff.
Why did you say the input is a number? It should be a sentence transformed to it’s word embedding. For example, if length of embedding vector is 50 and sentence has at most 500 words, this will be a (500,50) matrix. I think, what is does is to drop some features in the embedding vector, out of total of 50.
Hi,
It may be a late reply, but I would like to share my thinkings on prepadding. The reason for prepadding instead of postpadding is that for recurrent neural networks such as LSTMs, words appear earlier gets less updates, whereas words appear most recently will have a bigger impact on weight updates, according to the chain rule. Padding zeros at begining of a sequence will let rear content be better learned.
Li
Thanks for sharing!
Hi Jason
Thanks for providing such easy explanations for these complex topics.
In this tutorial, Embedding layer is used as the input layer as the data is a sequence of words.
I am working on a problem where I have a sequence of images as an example and a particular label is assigned to each example. The number of images in the sequence will vary from example to example. I have the following questions:
1) Can I use a LSTM layer as an input layer?
2) If the input layer is a LSTM layer, is there still a need to specify the max_len (which is constraint mentioning the maximum number of images an example can have)
Thanks in advance.
Interesting problem Harish.
I would caution you to consider a suite of different ways of representing this problem, then try a few to see what works.
My gut suggests using CNNs on the front end for the image data and then an LSTM in the middle and some dense layers on the backend for transforming the representation into a prediction.
I hope that helps.
Thanks you very much Jason.
Can you please let me know how to deal with sequences of different length without padding in this problem. If padding is required, how to choose the max. length for padding the sequence of images.
Padding is required for sequences of variable length.
Choose a max length based on all the data you have available to evaluate.
Thank you for your time and suggestion Jason.
Can you please explain what masking the input layer means and how can it be used to handle padding in keras.
Hi Harish,
I am working on a similar problem and would like to know if you continued on this problem? What worked and what did not?
Thanks in advance
Hi Jason,
Thanks for this tutorial. It’s so helpful! I would like to adapt this to my own problem. I’m working on a problem where I have a sequence of acoustic samples. The sequences vary in length, and I know the identity of the individual/entity producing the signal in each sequence. Since these sequences have a temporal element to them, (each sequence is a series in time and sequences belonging to the same individual are also linked temporally), I thought LSTM would be the way to go.
According to my understanding, the Embedding layer in this tutorial works to add an extra dimension to the dataset since the LSTM layer takes in 3D input data.
My question is is it advisable to use LSTM layer as a first layer in my problem, seeing that Embedding wouldn’t work with my non-integer acoustic samples? I know that in order to use LSTM as my first layer, I have to somehow reshape my data in a meaningful way so that it meets the requirements of the inputs of LSTM layer. I’ve already padded my sequences so my dataset is currently a 2D tensor. Padding with zeros however was not ideal because some of the original acoustic sample values are zero, representing a zero-pressure level. So I’ve manually padded using a different number.
I’m planning to use a stack of LSTM layers and a Dense layer at the end of my Sequential model.
P.s. I’m new to Keras. I’d appreciate any advice you can give.
Thank you
I’m glad it was useful Gciniwe.
Great question and hard to answer. I would caution you to review some literature for audio-based applications of LSTMs and CNNs and see what representations were used. The examples I’ve seen have been (sadly) trivial.
Try LSTM as the first layer, but also experiment with CNN (1D) then LSTM for additional opportunities to pull out structure. Perhaps also try Dense then LSTM. I would use one or more Dense on the output layers.
Good luck, I’m very interested to hear what you come up with.
Hi Gciniwe
Its interesting to see that I am also working on a similar problem. I work on speech and image processing. I have a small doubt. Please may I know how did you choose the padding values. Because in images also, we will have zeros and unable to understand how to do padding.
Thanks in advance
When i run the above code , i am getting the following error
:MemoryError: alloc failed
Apply node that caused the error: Alloc(TensorConstant{(1L, 1L, 1L) of 0.0}, TensorConstant{24}, Elemwise{Composite{((i0 * i1) // i2)}}[(0, 0)].0, TensorConstant{280})
Toposort index: 145
Inputs types: [TensorType(float32, (True, True, True)), TensorType(int64, scalar), TensorType(int64, scalar), TensorType(int64, scalar)]
Inputs shapes: [(1L, 1L, 1L), (), (), ()]
Inputs strides: [(4L, 4L, 4L), (), (), ()]
Inputs values: [array([[[ 0.]]], dtype=float32), array(24L, dtype=int64), array(-450L, dtype=int64), array(280L, dtype=int64)]
Outputs clients: [[IncSubtensor{Inc;:int64:}(Alloc.0, Subtensor{::int64}.0, Constant{24}), IncSubtensor{InplaceInc;int64::}(Alloc.0, IncSubtensor{Inc;:int64:}.0, Constant{0}), forall_inplace,cpu,grad_of_scan_fn}(TensorConstant{24}, Elemwise{tanh}.0, Subtensor{int64:int64:int64}.0, Alloc.0, Elemwise{Composite{(i0 – sqr(i1))}}.0, Subtensor{int64:int64:int64}.0, Subtensor{int64:int64:int64}.0,
any idea why? i am using theano 0.8.2 and keras 1.0.8
I’m sorry to hear that Nick, I’ve not seen this error.
Perhaps try the Theano backend and see if that makes any difference?
I got the same problem and I have no clue how to solve it..
Hi Jason,
I have one question. Can I use RNN LSTM for Time Series Sales Analysis. I have only one input every day sales of last one year. so total data points is around 278 and I want to predict for next 6 months. Will this much data points is sufficient for using RNN techniques.. and also can you please explain what is difference between LSTM and GRU and where to USE LSTM or GRU
Hi Deepak, My advice would be to try LSTM on your problem and see.
You may be better served using simpler statistical methods to forecast 60 months of sales data.
Jason, this is great. Thanks!
I would also love to see some unsupervised learning to know how it works and what the applications are.
Hi Corne,
I tend not to write tutorials on unsupervised techniques (other than feature selection) as I do not find methods like clustering useful in practice on predictive modeling problems.
Thanks for writing this tutorial. It’s very helpful. Why do LSTMs not require normalization of their features’ values?
Hi Jeff, great question.
Often you can get better performance with neural networks when the data is scaled to the range of the transfer function. In this case we use a sigmoid within the LSTMs so we find we get better performance by normalizing input data to the range 0-1.
I hope that helps.
Hi Jason, thanks for a great tutorial!
I am trying to normalize the data, basically dividing each element in X by the largest value (in this case 5000), since X is in range [0, 5000]. And I get much worse performance. Any idea why? Thanks!
No. Try other scaling methods.
Hi, Jason! Your tutorial is very helpful. But I still have a question about using dropouts in the LSTM cells. What is the difference of the actual effects of droupout_W and dropout_U? Should I just set them the same value in most cases? Could you recommend any paper related to this topic? Thank you very much!
I would refer you to the API Lau:
https://keras.io/layers/recurrent/#lstm
Generally, I recommend testing different values and see what works. In practice setting them to the same values might be a good starting point.
Hello,
thanks for the nice article. I have a question about the data encoding: “The words have been replaced by integers that indicate the ordered frequency of each word in the dataset”.
What exactly does ordered frequency mean? For instance, is the most frequent word encoded as 0 or 4999 in the end?
Great question Jeff.
I believe the most frequent word is 1.
I believe 0 was left for use as padding or when we want to trip low frequency words.
Thank you for your very useful posts.
I have a question.
In the last example (CNN&LSTM), It’s clear that we gained a faster training time, but how can we know that CNN is suitable here for this problem as a prior layer to LSTM. What does the spatial structure here mean? So, If I understand how to decide whether a dataset X has a spatial structure, then will this be a suitable clue to suggest a prior CNN to LSTM layer in a sequence-based problem?
Thanks,
Mazen
Hi Mazen,
The spatial structure is the order of words. To the CNN, they are just a sequence of numbers, but we know that that sequence has structure – the words (numbers used to represent words) and their order matter.
Model selection is hard. Often you want to pick the model that has the mix of the best performance and lowest complexity (easy to understand, maintain, retrain, use in production).
Yes, if a problem has some spatial structure (image, text, etc.) try a method that preserves that structure, like a CNN.
Hi Jason, great post!
I have been trying to use your experiment to classify text that come from several blogs for gender classification. However, I am getting a low accuracy close to 50%. Do you have any suggestions in terms of how I could pre-process my data to fit in the model? Each blog text has approximately 6000 words and i am doing some research know to see what I can do in terms of pre-processing to apply to your model.
Thanks
Wow, cool project Eduardo.
I wonder if you can cut the problem back to just the first sentence or first paragraph of the post.
I wonder if you can use a good word embedding.
I also wonder if you can use a CNN instead od LSTM to make the classification – or at least compare CNN alone to CNN + LSTM and double done on what works best.
Generally, here is a ton of advice for improving performance on deep learning problems:
https://machinelearningmastery.com/improve-deep-learning-performance/
Hi Jason,
Thank you for your time for this very helpful tutorial.
I was wondering if you would have considered to randomly shuffle the data prior to each epoch of training?
Thanks
Hi Emma,
Great question. The data is automatically shuffled prior to each epoch by the fit() function.
See more about the shuffle argument to the fit() function here:
https://keras.io/models/sequential/
Hi Jason,
Can you please show how to convert all the words to integers so that they are ready to be feed into keras models?
Here in IMDB they are directly working on integers but I have a problem where I have got many rows of text and I have to classify them(multiclass problem).
Also in LSTM+CNN i am getting an error:
ERROR (theano.gof.opt): Optimization failure due to: local_abstractconv_check
ERROR (theano.gof.opt): node: AbstractConv2d{border_mode=’half’, subsample=(1, 1), filter_flip=True, imshp=(None, None, None, None), kshp=(None, None, None, None)}(DimShuffle{0,2,1,x}.0, DimShuffle{3,2,0,1}.0)
ERROR (theano.gof.opt): TRACEBACK:
ERROR (theano.gof.opt): Traceback (most recent call last):
File “C:\Anaconda2\lib\site-packages\theano\gof\opt.py”, line 1772, in process_node
replacements = lopt.transform(node)
File “C:\Anaconda2\lib\site-packages\theano\tensor\nnet\opt.py”, line 402, in local_abstractconv_check
node.op.__class__.__name__)
AssertionError: AbstractConv2d Theano optimization failed: there is no implementation available supporting the requested options. Did you exclude both “conv_dnn” and “conv_gemm” from the optimizer? If on GPU, is cuDNN available and does the GPU support it? If on CPU, do you have a BLAS library installed Theano can link against?
I am running keras in windows with Theano backend and CPU only.
Thanks
Hi Jason,
Can you tell me how the IMDB database contains its data please? Text or vector?
Thanks.
Hi Thang Le, the IMDB dataset was originally text.
The words were converted to integers (one int for each word), and we model the data as fixed-length vectors of integers. Because we work with fixed-length vectors, we must truncate and/or pad the data to this fixed length.
Thank you Jason!
So when we call (X_train, y_train), (X_test, y_test) = imdb.load_data(), X_train[i] will be vector. And if it is vector then how can I convert my text data to vector to use in this?
Hi Le Thang, great question.
You can convert each character to an integer. Then each input will be a vector of integers. You can then use an Embedding layer to convert your vectors of integers to real-valued vectors in a projected space.
Hi Jason,
As I understand, X_train is a variable sequence of words in movie review for input then what does Y_train stand for?
Thank you!
Hi Quan Xiu, Y is the output variables and Y_train are the output variables for the training dataset.
For this dataset, the output values are movie sentiment values (positive or negative sentiment).
Thank you Jason,
So when we take X_test as input, the output will be compared to y_test to compute the accuracy, right?
Yes Quan Xiu, the predictions made by the model are compared to y_test.
The performance of this LSTM-network is lower than TFIDF + Logistic Regression:
https://gist.github.com/prinsherbert/92313f15fc814d6eed1e36ab4df1f92d
Are you sure the hidden state’s aren’t just counting words in a very expensive manor?
It’s true that this example is not tuned for optimal performance Herbert.
This leaves a rather important question, does it actually learn more complicated features than word-counts? And do LSTM’s do so in general? Obviously there is literature out there on this topic, but I think your post is somewhat misleading w.r.t. power of LSTM’s. It would be great to see an example where an LSTM outperforms a TFIDF, and give an idea about the type and size of the data that you need. (Thank you for the quick reply though 🙂 )
LSTM’s are only neat if they actually remember contextual things, not if they just fit simple models and take a long time to do so.
I agree Herbert.
LSTMs are hard to use. Initially, I wanted to share how to get up and running with the technique. I aim to come back to this example and test new configurations to get more/most from the method.
That would be great! It would also be nice to get an idea about the size of data needed for good performance (and of course, there are thousands of other open questions :))
Many thank your post, Jason. It’s helpful
I have some short questions. First, I feel nervous when chose hyperparameter for the model such as length vectors (32), a number of Embedding unit (500), a number of LSTM unit(100), most frequent words(5000). It depends on dataset, doesn’t it? How can we choose parameter?
Second, I have dataset about news daily for predicting the movement of price stock market. But, each news seems more words than each comment imdb dataset. Average each news about 2000 words, can you recommend me how I can choose approximate hyperparameter.
Thank you, (P/s sorry about my English if have any mistake)
Hi Huy,
We have to choose something. It is good practice to grid search over each of these parameters and select for best performance and model robustness.
Perhaps you can work with the top n most common words only.
Perhaps you can use a projection or embedding of the article.
Perhaps you can use some classical NLP methods on the text first.
Thank you for your quick response,
I am a newbie in Deep Learning, It seems really difficult to choose relevant parameters.
How do you get to the 16,750? 25,000/64 batches is 390.
Thanks!
According to my understanding, When training, the number of epoch often more than 100 to evaluate supervised machine learning result. But, In your example or Keras sample, It’s only between 3-15 epochs. Can you explain about that?
Thanks,
Epochs can vary from algorithm and problem. There are no rules Huy, let results guide everything.
So, How we can choose the relevant number of epochs?
Trial and error on your problem, and carefully watch the learning rate on your training and validation datasets.
Im looking for benchmarks of LSTM networks on Keras with known/public datasets.
Could you share what hardware configuration the examples in this post was run on (GPU/CPU/RAM etc)?
Thx
I used AWS with the g2.2xlarge configuration.
Is it possible in Keras to obtain the classifier output as each word propagates through the network?
Hi Mike, you can make one prediction at a time.
Not sure about seeing how the weights propagate through – I have not done this myself with Keras.
Hi,
What are some of the changes you have to make in your binary classification model to work for the multi-label classification?
also instead of a given input data such as imdb in number digit format, what steps do you take to process your raw text format dataset to make it compatible like imdb?
Great Job Jason.
I liked it very much…
I would really appreciate it if you tell me how we can do Sequence Clustering with LSTM Recurrent Neural Networks (Unsupervised learning task).
Sorry, I have not used LSTMs for clustering. I don’t have good advice for you.
Hi Jason,
Your book is really helpful for me. I have a question about time sequence classifier. Let’s say, I have 8 classes of time sequence data, each class has 200 training data and 50 validation data, how can I estimate the classification accuracy based on all the 50 validation data per class (sth. like log-maximum likelihood) using scikit-learn package or sth. else? It would be very appreciated that you could give me some advice. Thanks a lot in advance.
Best regards,
Ryan
Hi Ryan, this list of classification measures supported by sklearn might help as a start:
http://scikit-learn.org/stable/modules/classes.html#classification-metrics
Logloss is a very useful measure for evaluating the performance of learning algorithms on multi-class classification problems:
http://scikit-learn.org/stable/modules/generated/sklearn.metrics.log_loss.html#sklearn.metrics.log_loss
I hope that helps as a start.
Hi Jason, Thank you so much. I will try this logloss.
Let me know how you go.
Hi Jason,
Which approach is better Bags of words or word embedding for converting text to integer for correct and better classification?
I am a little confused in this.
Thanks in advance
Hi Shashank, embeddings are popular at the moment. I would suggest both and see what representation works best for you.
Hi Jason, thank you for your tutorials, I find them very clear and useful, but I have a little question when I try to use it to another problem setting..
as is pointed out in your post, words are embedding as vectors, and we feed a sequence of vectors to the model, to do classification.. as you mentioned cnn to deal with the implicit spatial relation inside the word vector(hope I got it right), so I have two questions related to this operation:
1. Is the Embedding layer specific to word, that said, keras has its own vocabulary and similarity definition to treat our feeded word sequence?
2. What if I have a sequence of 2d matrix, something like an image, how should I transform them to meet the required input shape to the CNN layer or directly the LSTM layer? For example, combined with your tutorial for the time series data, I got an trainX of size (5000, 5, 14, 13), where 5000 is the length of my samples, and 5 is the look_back (or time_step), while I have a matrix instead of a single value here, but I think I should use my specific Embedding technique here so I could pass a matrix instead of a vector before an CNN or a LSTM layer….
Sorry if my question is not described well, but my intention is really to get the temporal-spatial connection lie in my data… so I want to feed into my model with a sequence of matrix as one sample.. and the output will be one matrix..
thank you for your patience!!
33202176/33213513 [============================>.] – ETA: 0s 19800064/33213513 [================>………….] – ETA: 207s – ETA: 194s____________________________________________________________________________________________________
Layer (type) Output Shape Param # Connected to
====================================================================================================
embedding_1 (Embedding) (None, 500, 32) 160000 embedding_input_1[0][0]
____________________________________________________________________________________________________
lstm_1 (LSTM) (None, 100) 53200 embedding_1[0][0]
____________________________________________________________________________________________________
dense_1 (Dense) (None, 1) 101 lstm_1[0][0]
====================================================================================================
Total params: 213301
____________________________________________________________________________________________________
None
Epoch 1/3
Kernel died, restarting
pip install -U numpy
solves the problem
Thanks for sharing!
Hi Jason,
Thanks for the nice article. Because IMDb data is very large I tried to replace it with spam dataset. What kind of changes should I make in the original code to run it. I have asked this question in stack-overflow but sofar no answer. http://stackoverflow.com/questions/41322243/how-to-use-keras-rnn-for-text-classification-in-a-dataset ?
Any help?
Great idea!
I would suggest you encode each word as a unique integer. Then you can start using it as an input for the Embedding layer.
Hi Jason,
Thanks for the post. It is really helpful. Do I need to configure for the tensorflow to make use of GPU when I run this code or does it automatically select GPU if its available?
These examples are small and run fast on the CPU, no GPU is required.
I tried it on CPU and it worked fine. I plan to replicate the process and expand your method for a different use case. Its high dimensional compared to this. Do you have a tutorial on making use of GPU as well? Can I implement the same code in gpu or is the format all different?
Same code, use of the backend is controlled by the Theano or TensorFlow backend that you’re using.
Jason,
Thanks for the interesting tutorial! Do you have any thoughts on how the LSTM trained to classify sequences could then be turned around to generate new ones? I.e. now that it “knows” what a positive review sounds like, could it be used to generate new and novel positive reviews? (ignore possible nefarious uses for such a setup 🙂 )
There are several interesting examples of LSTMs being trained to learn sequences to generate new ones… however, they have no concept of classification, or understanding what a “good” vs “bad” sequence is, like yours does. So, I’m essentially interested in merging the two approaches — train an LSTM with a number of “good” and “bad” sequences, and then have it generate new “good” ones.
Any thoughts or pointers would be very welcome!
I have not explored this myself. I don’t have any offhand quips, it requires careful thought I think.
This post might help with the other side of the coin, the generation of text:
https://machinelearningmastery.com/text-generation-lstm-recurrent-neural-networks-python-keras/
I would love to hear how you get on.
Thanks, if you do come up with any crazy ideas, please let me know :).
One pedestrian approach I’m thinking off is having the classifier used to simply “weed out” the undesired inputs, and then feed only desired ones into a new LSTM which can then be used to generate more sequences like those, using the approach like the one in your other post.
That doesn’t seem ideal, as it feels like I’m throwing away some of the knowledge about what makes an undesired sequence undesired… But, on the other hand, I have more freedom in selecting the classifier algorithm.
Thank you for this tutorial.
Regarding the variable length problem, though other people have asked about it, I have a further question.
If I have a dataset with high deviation of length, say, some text has 10 words, some has 100000 words. Therefore, if I just choose 1000 as my maxlen, I lost a lot of information.
If I choose 100000 as the maxlen, I consume too much computational power.
Is there a another way of dealing with that? (Without padding or truncating)
Also, can you write a tutorial about how to use word2vec pretrained embedding with RNN?
Not word2vec itself, but how to use the result of word2vec.
The counting based word representation lost too much semantic information.
Great questions Albert.
I don’t have a good off-the-cuff answer for you re long sequences. It requires further research.
Keen to tackle the suggested tutorial using word2vc representations.
I only have biology background, but I can reproduced the results. Great.
Glad to hear it Charles.
Hi Jason, i noted you mentioned updated examples for Tensorflow 0.10.0. I can only see Keras codes, am i missing something?
Thanks.
Hi Jax,
Keras runs on top of Theano and TensorFlow. One or the other are required to use Keras.
I was leaving a note that the example was tested on an updated version of Keras using an updated version of the TensorFlow backend.
I am not sure I understand how recurrence and sequence work here.
I would expect you’d feed a sequence of one-hot vectors for each review, where each one-hot vector represents one word. This way, you would not need a maximum length for the review (nor padding), and I could see how you’d use recurrence one word at a time.
But I understand you’re feeding the whole review in one go, so it looks like e feedforward.
Can you explain that?
Hi Kakaio,
Yes, indeed we are feeding one review at a time. It is the input structured we’d use for a MLP.
Internally, consider the LSTM network as building up state on the sequence of words in the review and from that sequence learning the appropriate sentiment.
how is the LSTM building up state one the sequence of words leveraging recurrence? you’re feeding the LSTM all the sequence at the same time, there’re no time steps.
Hi Kakaop, quite right. The example does not leverage recurrence.
From this tutorial how can I predict the test values and how to write to a file? Are these predicted values generate in the encoded format?
Guys, this is a very clear and useful article, and thanks for the Keras code. But I can’t seem to find any sample code for running the trained model to make a prediction. It is not in imdb.py, that just does the evaluation. Does any one have some sample code for prediction to show?
Hi Bruce,
You can fit the model on all of the training data, than forecast for new inputs using:
Does that help?
That’s not the hard part. However, I may have figured out what I need to know. That is take the result returned by model.predict and take the last item in the array as the classifications. Any one disagrees?
Hi, it’s the awesome tutorial.
I have a question regarding your model.
I am new to RNN, so the question would be stupid.
Inputting word embedding layer is crucial in your setting – sequence classification rather than prediction of the next word??
Generally, a word embedding (or similar projection) is a good representation for NLP problems.
Hi Jason,
great tutorial. Really helped me alot.
I’ve noticed that in the first part you called fit() on the model with “validation_data=(X_test, y_test)”. This isn’t in the final code summary. So I wondered if that’s just a mistake or if you forgot it later on.
But then again it seems wrong to me to use the test data set for validation. What are your thoughts on this?
The model does not use the test data at this point, it is just evaluated on it. It helps to get an idea of how well the model is doing.
What happen if the code uses LSTM with 100 units and sentence length is 200. Does that mean only the first 100 words in the sentence act as inputs, and the last 100 words will be ignored?
No, the number of units in the hidden layer and the length of sequences are different configuration parameters.
You can have 1 unit with 2K sequence length if you like, the model just won’t learn it.
I hope that helps.
Hi Jason,
in the last part the LSTM layer returns a sequence, right? And after that the dense layer only takes one parameter. How does the dense layer know that it should take the last parameter? Or does it even take the last parameter?
No, in this case each LSTM unit is not returning a sequence, just a single value.
Hi Jason,
Very interesting and useful article. Thank you for writing such useful articles. I have had the privilege of going through your other articles which are very useful.
Just wanted to ask, how do we encode a new test data to make same format as required for the program. There is no dictionary involved i guess for the conversion. So how can we go about for this conversion? For instance, consider a sample sentence “Very interesting article on sequence classification”. What will be encoded numeric representation?
Thanks in advance
Great question.
You can encode the chars as integers (integer encode), then encode the integers as boolean vectors (one hot encode).
Great article Jason. I wanted to continue the question Prashanth asked, how to pre-process the user input. If we use CountVectorizer() sure, it will convert it in the required form but then words will not be same as before. Even a single new word will create extra element. Can you please explain, how to pre-process the user input such that it resembles with the trained model. Thanks in advance.
You can allocate an alphabet of 1M words, all integers from 1 to 1M, then use that encoding for any words you see.
The idea is to have a buffer in your encoding scheme.
Also, if you drop all low-frequency words, this will give you more buffer. Often 25K words is more than enough.
Your answer honestly cleared many doubts. Thanks a lot for the quick reply. I have an idea now about, what to do.
I’m glad to hear that Manish.
I have dataset just a vector feature like [1, 0,5,1,1,2,1] -> y just 0,1 binary or category like 0,1,2,3. I want to use LSTM to classify binary or category, how can i do it guys, i just add LSTM with Dense, but LSTM need input 3 dimension but Dense just 2 dimension. I know i need time sequence, i try to find out more but can’t get nothing. Can u explain and tell me how. pls, Thank you so much
You may want to consider a seq2seq structure with an encoder for the input sequence and a decoder for the output sequence.
Something like:
I have a tutorial on this scheduled.
I hope that helps.
thanks you, i will try to find out, then response you.
You’re welcome.
Ay, i have 1 question in another your post about why i use function evaluate model.evaluate(x_test, y_test) to get accuracy score of model after train with train dataset , but its return result >1 in some case, i don’t know why, it make me can’t beleive in this function. Can you explain for me why?
Sorry I don’t understand your question, perhaps you can rephrase it?
I don’t know the result return by function evaluate >1, but i thinks it should just from 0 -> 1 ( model.evaluate(x_test,y_test) with model i had trained it before with train dataset)
Hi Jason, Can you explain your code step by step Jason, i have follow tutorial : https://blog.keras.io/building-autoencoders-in-keras.html but i have some confused to understand. :(.
If you have questions about that post, I would recommend contacting the author.
Hi Dear Joson
I am new to deep learning and intends to work on keras or tensorflow for corpus analysis. May you help me or send me basic tutorials
regards
Mazhar Ali
Sorry, I only have tutorials for Keras:
https://machinelearningmastery.com/start-here/#deeplearning
Thank you for your friendly explanation.
I bought a lot of help from your books.
Are you willing to add examples of fit_generator and batch normalization to the IMDB LSTM example?
I was told to use the fit_generator function to process large amounts of data.
If there is an example, it will be very helpful to book buyers.
I would like to add this kind of example in the future. Thanks for the suggestion.
Hi Jason
I would like to know where I can read more about dropout and recurrent_dropout. Do you know some paper or something to explore it?
Thanks!
I have a tutorial on dropout here:
https://machinelearningmastery.com/dropout-regularization-deep-learning-models-keras/
I have a post on recurrent dropout scheduled for the blog soon.
Hi Jason,
I’ve a problem with the shape of my dataset
x_train = numpy.random.random((100, 3))
y_train = uti.to_categorical(numpy.random.randint(10, size=(100, 1)), num_classes=10)
model = Sequential()
model.add(Conv1D(2,2,activation=’relu’,input_shape=x_train.shape))
model.compile(loss=’binary_crossentropy’, optimizer=’adam’, metrics=[‘accuracy’])
model.fit(x_train,y_train, epochs=150)
I have tried to create random a dataset, and pass at CNN with 1D, but I don’t know why, the Conv1D accepts my shape (I think that put automaticly the value None), but the fit doesn’t accept (I think becaus the Conv1D have accepted 3 dimension). I have this error:
ValueError: Error when checking model input: expected conv1d_1_input to have 3 dimensions, but got array with shape (100, 3)
Your input data must be 3d, even if one or two of those dimensions have a width of 1.
Hi Jason,
Thanks for an awesome article!
I wanted to ask for some suggestions on training my data set. The data I have are 1d measurements taken at a time with a binary label for each instance.
Thanks to your blogs I successfully have built a LSTM and it does a great job at classifying the dominant class. The main issue is that the proportion of 0s to 1s is very high. There are about .03 the number of 1s as there are 0s. For the most part, the 1s occur when there are high values of these measurements. So, I figured I could get a LSTM model to make better predictions if a model could see the last “p” measurements. Intuitively, it would recognize an abnormal increase in the measurement and associate that behavior with a output of 1.
Knowing some of this basic basckground could you suggest a structure that may
1.) help exploit the structure of abnormally high measurement with outputs of 1
2.) help with the low exposure to 1 instances
Thanks for any help or references!
cheers!
Hi Len,
Perhaps you can use some of the resampling methods used for imbalanced datasets:
https://machinelearningmastery.com/tactics-to-combat-imbalanced-classes-in-your-machine-learning-dataset/
Hi, that’s a great tutorial!
Just wondering: as you are paddin with zeros, why aren’t you setting the Embedding layer flag mask_zero to True?
Without doing that, the padded symbols will influence the computation of the cost function, isn’t it?
That is a good suggestion. Perhaps that flag did not exist when I write the example.
If you see a benefit, let me know.
Hi Jason,
Great tutorial! Helped a lot.
I’ve got a theoretical question though. Is sequence classification just based on the last state of the LSTM or do you have to take the dense layer for all the hidden units(100 LSTM in this case). Is sequence classification possible just based on the last state? Most of the implementations I see, there is dense and a softmax to classify the sequence.
We do need the dense layer to interpret what the LSTMs have learned.
The LSTMs are modeling the problem as a function of the input time steps and of the internal state.
Hi Jason,
Can you tell me about time_step in LSTM?, with example or something to easy understand. If my data have 2 dimension, [[1,2]…[1,3]] ouput: [1,…0], so with keras, LSTM layer need 3 dimension, so i just can reshape input data to 3 dimension with time_step =1, can train it like this?, with time_step> 1 is it better, i want to know mean of time_step in LSTM, thank you so much for read my question.
You can, but it is better to provide the sequence information in the time step.
The LSTM is developing a function of observations over prior time steps.
Hi Jason,
First of ali, thank you for your great explanation.
I am considering setting up an aws g2.2xlarge instance according to your explanation in another post . Would you have some benchmark (ex: time of 1 epoch of one of the above examples) so that I can compre with my current hardware?
Sorry, I don’t have any execution time benchmarks.
I generally see great benefit from large AWS instances in terms getting access to a lot more memory (larger datasets) when using LSTMs.
I see a lot more benefit running CNNs on GPUs than LSTMs on GPUs.
Hi Jason,
I am also curious in the problem of padding. I think pad_sequence is the way to obtain fixed length of sequences. However, instead of padding zeros, can we actually scale the data?
Then, the problem is 1) if scaling sequences will distort the meaning of sentences given that sentences are represented as sequences and 2) how to choose a good scale factor.
Thank you.
Great question.
Generally, a good way to reduce the length of sequences of words is first remove the low frequency words, then truncate the sequence to a desired length or pad out to the length.
For using LSTM, why we still need to scale the input sequence to the fixed size? Why not build some model like seq2seq just multi-input to one-output
Even with seq2seq, you must vectorize your input data.
I saw the data loaded from IMDB, which has already be encoded as numbers.
Why do we need another Embedding layer to encoding?
(X_train, y_train), (X_test, y_test) = imdb.load_data(num_words=top_words)
print X_train[1]
The output is
[1, 194, 1153, 194, 2, 78, 228, 5, 6, 1463, 4369,…
The embedding is a more expressive representation which results in better performance.
Thanks Jason for your article and answering comments also. Can I use this approach to solve my issue described in this stack-overflow question? Please take a look at that.
http://stackoverflow.com/questions/43987060/pattern-recognition-or-named-entity-recognition-for-information-extraction-in-nl/43991328#43991328
Perhaps, I would recommend finding some existing research to template a solution.
Thanks Jason for your article. I have implemented a CNN followed by LSTM neural network model in keras for sentence classification. But after 1 or 2 epoch my training accuracy and validation accuracy stuck to some number and do not change. Like it has stuck in some local minima or some other reason. What should i do to resolve this problem. If i use only CNN in my model then both training and validation accuracy converges to good accuracy. Can you help me in this. I couldn’t identify the problem.
Here is the training and validation accuracy.
Epoch 1/20
1472/1500 [============================>.] -8s – loss: 0.5327 – acc: 0.8516 – val_loss: 0.3925 – val_acc: 0.8460
Epoch 2/20
1500/1500 [==============================] – 10s – loss: 0.3733 – acc: 0.8531 – val_loss: 0.3755 – val_acc: 0.8460
Epoch 3/20
1500/1500 [==============================] – 8s – loss: 0.3695 – acc: 0.8529 – val_loss: 0.3764 – val_acc: 0.8460
Epoch 4/20
1500/1500 [==============================] – 8s – loss: 0.3700 – acc: 0.8531 – val_loss: 0.3752 – val_acc: 0.8460
Epoch 5/20
1500/1500 [==============================] – 8s – loss: 0.3706 – acc: 0.8528 – val_loss: 0.3763 – val_acc: 0.8460
Epoch 6/20
1500/1500 [==============================] – 8s – loss: 0.3703 – acc: 0.8528 – val_loss: 0.3760 – val_acc: 0.8460
Epoch 7/20
1500/1500 [==============================] – 8s – loss: 0.3700 – acc: 0.8528 – val_loss: 0.3764 – val_acc: 0.8460
Epoch 8/20
1500/1500 [==============================] – 8s – loss: 0.3697 – acc: 0.8531 – val_loss: 0.3752 – val_acc: 0.8460
Epoch 9/20
1500/1500 [==============================] – 8s – loss: 0.3708 – acc: 0.8530 – val_loss: 0.3758 – val_acc: 0.8460
Epoch 10/20
1500/1500 [==============================] – 8s – loss: 0.3703 – acc: 0.8527 – val_loss: 0.3760 – val_acc: 0.8460
Epoch 11/20
1500/1500 [==============================] – 8s – loss: 0.3698 – acc: 0.8531 – val_loss: 0.3753 – val_acc: 0.8460
Epoch 12/20
1500/1500 [==============================] – 8s – loss: 0.3699 – acc: 0.8531 – val_loss: 0.3758 – val_acc: 0.8460
Epoch 13/20
1500/1500 [==============================] – 8s – loss: 0.3698 – acc: 0.8531 – val_loss: 0.3753 – val_acc: 0.8460
Epoch 14/20
1500/1500 [==============================] – 10s – loss: 0.3700 – acc: 0.8533 – val_loss: 0.3769 – val_acc: 0.8460
Epoch 15/20
1500/1500 [==============================] – 9s – loss: 0.3704 – acc: 0.8532 – val_loss: 0.3768 – val_acc: 0.8460
Epoch 16/20
1500/1500 [==============================] – 8s – loss: 0.3699 – acc: 0.8531 – val_loss: 0.3756 – val_acc: 0.8460
Epoch 17/20
1500/1500 [==============================] – 8s – loss: 0.3699 – acc: 0.8531 – val_loss: 0.3753 – val_acc: 0.8460
Epoch 18/20
1500/1500 [==============================] – 8s – loss: 0.3696 – acc: 0.8531 – val_loss: 0.3753 – val_acc: 0.8460
Epoch 19/20
1500/1500 [==============================] – 8s – loss: 0.3696 – acc: 0.8531 – val_loss: 0.3757 – val_acc: 0.8460
Epoch 20/20
1500/1500 [==============================] – 8s – loss: 0.3701 – acc: 0.8531 – val_loss: 0.3754 – val_acc: 0.8460
I provide a list of ideas here to help you improve the performance on your deep learning projects:
https://machinelearningmastery.com/improve-deep-learning-performance/
Jason, thaks for yor great post.
I am beginner with DL.
If I need to include some behavioral features to this analysis, let say: age, genre, zipcode, time (DD:HH), season (spring/summer/autumn/winter)… could you give me some hints to implement that?
TIA
Each would be a different feature on the input data.
Remember, input data must be structured [samples, timesteps, features].
My data is of shape (8000,30) and i need to use 30 timesteps.
I do
model.add(LSTM(200, input_shape=(timesteps,train.shape[1])))
but when i run the code it give me and error
ValueError: Error when checking input: expected lstm_20_input to have 3 dimensions, but got array with shape (8000, 30)
How to change the shape of the training data in the format you mentioned
Remember, input data must be structured [samples, timesteps, features]. (8000,30,30)
This post will help you with the shape of your data for LSTMs:
https://machinelearningmastery.com/reshape-input-data-long-short-term-memory-networks-keras/
Hi,
How can I use my own data, instead of IMDB for training?
Thanks
Kadir
You will need to encode the text data as integers.
Hello Dr.Jason,
I am very thankful for your blog-posts. They are undoubtedly one of the best on the internet.
I have one doubt though. Why did you use the validation dataset as x_test and y_test in the very first example that you described. I just find it a little bit confusing.
Thanks in advance
Thanks.
I did it to give an idea of skill of the model as it was being fit. You do not need to do this.
i added dropout on CNN+RNN like you said and it gives me 87.65% accuracy. I still not clear the purpose of combining both as i thought CNN is for 2D+ input like image or video. But anyway, your tutorial gives me a great starting point to dive into RNN. Many thanks!
Glad to hear it.
Thanks for the post.
If I am understanding right, after the embedding layer EACH SAMPLE (each review) in the training data is transformed into a 32 by 500 matrix. When taking an analogy from audio spectrogram, it is a 32-dim spectrum with 500 time frames long.
With the equivalence or analogy above, I can perform audio waveform classification with audio raw spectrogram as the input and class labels (whatever it is, might be audio quality good or bad) in exact the same code in this post (except the embedding layer). Is it correct?
Furthermore, I am wondering about why should the length of the input be the same, i.e. 500 in the post. If I am doing in the context of online training, in which a single sample is fed into the model at a time (batch size is 1), there should be no concern about varying length of samples right? That is, each sample (of varying length without padding) and its target are used to train the model one after another, and there is no worry about the varying length. Is it just the issue of implementation in Keras, or in theory the input length of each sample should be the same?
Hi Fred,
Yes, try it.
The vectorized input requires all inputs to have the same length (for efficiencies in the backend libraries). You use zero-padding (and even masking) to meet this requirement.
The size parameters are fixed in the definition of the network I believe. You could do tricks from batch to batch re-defining+compiling your network as you go, but that would not be efficient.
Thanks for your reply, I will try it.
I was just wondering if the RNN or LSTM in theory requires every input to be in a same length.
As far as I know, one of the superiorities of RNN over DNN is that it accepts varying-length input.
It doesn’t bother me If the requirement is for efficiency issue in Keras, and the zero’s (if zero-padding is used) is regarded to carry zero information. In the audio spectrogram case, would you recommend zero-padding the raw waveform (one-D) or spectrogram (two-D)? With the analogy to your post, the choice would be the former though.
Hi Fred,
Padding is not required by LSTMs in theory, it is only a limitation of efficient implementations that require vectorized inputs.
A fair tradeoff for most applications perhaps.
Hi Jason,
Is there a way in RNN (keras implementation) to control for the attention of the LSTM.
I have a dataset where 100 time series inputs are fed as sequence. I want the LSTM to give more importance to the last 10 time series inputs.
Can it be done?
Thanks in advance.
Yes, but you must code a custom layer to do the attention.
I hope to cover attention models for LSTMs soon.
Hi Jason,
After building and saving the model I want to use it for a prediction on new texts but I don’t know how to preprocess the plain text in order to use them for predictions. I have searched about it and find this way:
text = np.array([‘this is a random sentence’])
tk = keras.preprocessing.text.Tokenizer( nb_words=2000, lower=True,split=” “)
predictions = loaded_model.predict(np.array(tk.fit_on_texts(text)))
but this is not working for me and showing this error:
ValueError: Error when checking : expected embedding_1_input to have 2 dimensions, but got array with shape ()
Can You please tell me the proper way to preprocess the text. Any help is greatly appreciated.
Thanks
Generally, you need to integer encode the words.
Thanks for the reply
I converted my string like this:
text = ‘It is a good movie to watch’
import keras.preprocessing.text
text = keras.preprocessing.text.one_hot(text, 5000, lower=True, split=” “)
text = [text]
text = sequence.pad_sequences(text, 500)
predictions = loaded_model.predict(text)
But got the output as: [[ 0.10996077]]
Shouldn’t it be close to 1?
Many Thanks
Sorry, I don’t follow. Why do you expect te output to be 1? What are you predicting?
What I interpret is that 1 is the label for positive sentiment and since I am using a positive statement to predict I am expecting output to be 1.
I had made a mistake in the last comment by using model.predict() to get class labels, the correct way to get the label is model.predict_classes() but still, it’s not giving proper class labels.
So my question is whether I made a mistake in converting text into one-hot vector or is it the right way to do it.
Many Thanks
As long as you are consistent in data preparation and in interpretation at the other end, then you should be fine.
Can you do a tutorial for preprocessing text dataset and then passing them as input using word embeddings? Thanks!
Great suggestion. It is high on my list!
Can we use sequence labelling problem over continous variable. I have datasets of customer paying their debt within due date, buffer period and beyond buffer period. Basis on this I want to score the customer from good to bad. Is it posible using sequence labelling.
Perhaps, I’m not sure I understand your dataset. Can you give a one-case example?
Hi Jason, great tutorial!
I have data as follows
Text Alpha-Numeric Label
“foo” A1034 A
“bar” A1234 B
I have already mapped an LSTM model from Text column to label column. However, I need to add the Alpha-numeric Column with the Text as an additional feature to my LSTM model. How can I do that in Keras?
Consider a one hot encoding.
Hi, it was really great and I am happy that this tutorial was my first practical project in LSTM. I need to have f-measures, False Positives and AUC instead of “accuracy” in your code. Do you have any idea how to get them?
Thank you in advance.
Sajad,
You can make predictions, then use the array of predictions and expected value to calculate these scores using sklearn:
http://scikit-learn.org/stable/modules/classes.html#sklearn-metrics-metrics
I have a question about built-in embedding layer in Keras.
I have done word embedding with word2vec model which is working based on the semantic similarity of the words–those in the same context are more similar. I am wondering whether Keras embedding layer is also following the w2v model or it has its own algorithm to map the words into vectors?
Based on what semantics it map the words to vectors?
Great question, there’s a bit more on it here:
https://keras.io/layers/embeddings/
Thanks Jason!
I went through most of the documentations about this but none of them mention about the semantic behind this biult-in word embedding process.
I would like to know it because right now I keep thinking that the process inside this method is possibly causing the low accuracy!
Please keep me posted if you find anything about it.
I would recommend reading the code here:
https://github.com/fchollet/keras/blob/master/keras/layers/embeddings.py#L11
Hi Jason,
Excellent article. I am trying to use CNN to model time series data and feed into LSTM for supervised learning. I have a 2d matrix with columns representing previous n-time steps and rows representing the different price levels each time steps visited:
Price Bar0 Bar1 Bar2 Bar3 Bar4 Bar5 …
0 0 0 1 1 0 0
1 1 0 1 1 0 1
2 1 1 1 1 1 1
3 1 1 0 1 1 0
4 0 0 0 0 1 0
…
this matrix will represent, price data of:
High Low
Bar0 3 1
Bar1 3 2
Bar2 2 0
Bar3 3 0
Bar4 4 2
Bar5 2 1
Could you tell me how to adapt your 1-d CNN to 2-d CNN?
hi Jason,
Great post for me.
But I want to ask you about: length vector in Embedded layer, you said “the first layer is the Embedded layer that uses 32 length vectors to represent each word” , why you choose 32 instead of another number like 64 or 128, Can you give me some best practice, or reason for your choose.
Thanks you so much.
Trial and error. You could experiment with other representations and see what works best for your problem.
Thanks Jason.
You’re welcome.
@Jason,
“Sequence classification is a predictive modeling problem where you have some sequence of inputs over space or time and the task is to predict a category for the sequence.”
this is inspiring. I am thinking about to use sequence classification to IRIS dataset.
Do you think it works ?
The iris flowers dataset is not a sequence classification problem. It is just a classification problem.
@Jason,
Do you mean that:
I can not use LSTM for IRIS classification? I am working on IRIS like dataset. So I m exploring all possible classifiers. You have one here in your website. Besides,
I have tried RBM in SKLearn, it did not work as my inputs are not binary inputs like MNIST dataset (even after SKLearn’s preprocessing.binarizer() function). I think they were wrong to say that RBM In SKLearn works for data in range of [0,1], it only works for 0 and 1.
(by the way I send you my code to for reference)
I also have tried probablistic neural net (PNN), which yields only 78% accuracy, low and no way to increase layers of PNN as it is single layer net (from Neupy).
Now I came to RNN, but you said that.
No, the iris dataset is not a sequence classification problem and the LSTM would be a bad fit.
@Jason,
What would you suggest ? I need your expert advice.
I tried RBM in sklearn, it did not work.
You said ,RNN would not work for it.
I think, CNN clearly does not work for it.
Do DBN and VAE left?
I wish to classify IRIS in 3 different ways. I did one only.
Consider SVM, CART, and kNN.
@Jason,
Thank you. I’ve already tried kNN and SVM . There were good, gave good results.
I have a feeling that Deep Learning methods yields even better results to my dataset. Do you have other suggessions in Deep Learning! this is my dataset:
https://www.dropbox.com/s/4xsshq7nnlhd31h/P7_all_Data.csv?dl=0
You could try a multilayer perceptron neural network.
Jason,
I did try multi-layer perceptron. Result was good.
I want to use deep neural net of more than 3 layers.
What do you think about convolutional neural network?
I originally think it is impossible. But, now thinking about it again.
You can do what you wish. CNNs are designed for spatial input and the iris flower dataset does not have a spatial input.
Hi Jason, I want ask what is the use of Dropout, It makes the accuracy lower, so does this mean the dropout is bad for machine learning? thank you!
Dropout can improve the performance of the model on some problems.
See this post on dropout:
https://machinelearningmastery.com/dropout-regularization-deep-learning-models-keras/
Hey Jason! Great Post 🙂 Really helped me in my internship this summer. I just wanted to get your thoughts on a couple things.
1. I’ve trained with about 400k documents in total and I’m getting an accuracy of ~98%. I always get vary when my model does ‘too’ well. Is that a fair cause-effect due to the enormous dataset ?
2. When I think of CNN’ing+max_pooling word vectors(Glove), I think of the operation basically meshing the word vectors for 3 words(possibly forming like a phrase representation).Am I right in my thought process ?
3. I’m still a little unclear on what the LSTM learns. I understand its not a typical seq-2-seq problem, so what do those 100 LSTM units hold ?
Thanks so much again for the great tutorial! 🙂
I’m glad to hear that Daniel.
Maybe you want to test the model on a hold out set to see if the model skill is real or overfit.
Something like that, pooling does good nonlinear things that may not relate back to word vectors/words cleanly.
They hold a function of input and prior items in the input sequence. It’s complex for sure.
Hello Jason,
I wonder how 100 neurons in the LSTM layer would be able to accept the 500 vectors/words? I thought that the size of the LSTM layer should be equivalent to the length of the input sequence!
Good question, no the layers do not need to have the same number of units.
For example, If I had a vector of length 5 as input to a single neuron, then the neuron would have 5 weights, one for each element. We do not need 5 neurons for the 5 input elements (although we could), these concerns are separate and decoupled.
Thanks for your reply.
But here we have already each input as a vector not a scalar! would that mean in this case that each neuron will receive 5 vectors each of them 32 dimensional? so each neuron will have 5*32=160 weights? and if so, what is the advantage of that over having every neuron process only one word/vector?
For an MLP, word vectors are concatenated as you say and each neuron gets a lot of inputs.
LSTMs, on the other hand, treat each word as one input in a sequence and process them one at a time.
The idea is called “distributed representation” where all neurons get all inputs and they selectively learn different parts to focus on.
This is key to neural networks.
Hi Jason,
consider we have 500 sequences with 100 elements in each sequence.
if we do the embedding in a 32 dimensions vector, we will have a 100*32 matrix for each sequence.
Now assume we are using only a layer of LSTM(20) in our project. I am a bit confused in practice:
I know that We have a hidden layer with 20 LSTM units in parallel. I want to know how Keras gives a sequence to the model. Does it give the same 32 dimension vectors to all LSTM units at a time in order and an iteration finishes at time [t+100]? (this way I think all units give the same (copy) value after training, and it is equivalent to having only on unit), OR it gives 32dim vectors 20 by 20 to the the model in order and iteration ends at time [t+5]?
Thank you in advance,
Sajad
Good question.
So, the 100 time steps are passed as input to the model with 500 samples and 1 feature, something like [500, 100, 1].
The Embedding will transform each time step into a 32 dimensional vector.
The LSTM will process the sequence one time step at a time, so one 32-dimensional embedding at a time.
Each memory cell will get the whole input. They all have a go at modeling the problem. An error propagated from deeper layers will encourage the hidden LSTM layer to learn the input sequence in a specific way, e.g. classify the sequence. Each cell will learn something slightly different.
Does that help?
Thank you for your clear answer.
1) I am working on malware detection using LSTM, so I have malware activities in a sequence. As another question, I want to know more about Embedding layer in Keras. In my project I have to convert elements into integer numbers to feed Embedding layer of Keras. I guess Embedding is a frozen neural network layer to convert elements of a sequence to a vector in a way that relations between different elements are meaningful, Right? I would like to know if there is any logical issue of using Embedding in my project.
2) Do you know any references (book, paper, website etc.) for Embedding in Keras (academic/non-academic)? I need to draw a figure describing Embedding training network.
Thank you for your patience,
Sajad
The Embedding has weights that are leared when you fit the model.
You can use pre-trained weights from a word2vec or glove run if you like. Learning custom weights for your task is often better.
I have a few posts scheduled on how the learned embedding layer works, that should be out next month. For now, this might be a good place for you to start:
https://en.wikipedia.org/wiki/Word_embedding
The Keras Embedding layer are just weights – vectors learned for each word in the input vocab. Very simple to describe.
Thank you Jason.
That’s great, I am waiting for your posts on embedding.
Thanks Sajad.
Hey Jason, this post was great for me.
As a question I would like to know how to set the number of LSTM units in the hidden layer?
Is there any relationships between the number of samples (sequences) and the number of hidden units?
I have 400 sequences with 5000 element in each. How many LSTM units should I use? I know that I should test model with different number of hidden units but I am looking for an upperbound and lowerbound for number hidden units.
saho,
There is no analytical way to configure a neural network. I recommend trial and error, grid search, random search or copy configurations from other models.
great work ! what if i want to apply this code on simple sentence sequence classification. how can we do that? how we are going to manipulate the data
.
thank you
Sure.
I would recommend spending time cleaning the data, then integer encode it ready for the model. I recommend an embedding layer on the front of the model.
thank you … how can i replace imdb data with my own data that is composed of simple sentences? and how can i change the program accordingly?
Load the text data, clean the text data, then encode your words as integers.
See the Keras Tokenizer as a start:
https://keras.io/preprocessing/text/#tokenizer
Hi Jason! First thanks for your amazing web!
And now comes the question: In my case I am trying to solve a task classification problem. Each task is described by 57 time series with 74 time steps each. For the training phase I do have 100 task examples of 10 different classes.
This way, I have created a [100,74,57] input and a [100,1] output with the label for each task.
This is, I have a multivariate time series to multilabel classification problem.
What type of learning structure would you suggest? I am aware that I may need to collect/generate more data but I am new both in python and deep learning and I am having some trouble creating a small running example for multivariate ts -> multilabel classification.
Thanks!
For multi-class classification, you will need a one hot encoding of your output variable so the dimensions will be [100,10] and then use a softmax activation function in the output layer to predict the outcome probability across all 10 classes.
For the specific model, try MLPs with sliding window, then maybe some RNNs like LSTMs to see if they can do better.
Thanks for your tutorial. My problem is classfication a packet (is captured everytime with many features) whether normal or abnormal. I would like to adapt LSTM to my own problem. My data are matrixes: X_train(4000,41), Y_train(4000,1), X_test(1000,41), Y_test(1000,1) – Y is label. One of 41 feature is time, others are input variables. I think, I have to extract time feature from 41 features, is it correct. Is this process in Keras?
First, I am confusing how to reshape my data in a meaningful way so that it meets the requirements of the inputs of LSTM layer. I expect my data like this:
x_train.shape = (4000,1,41) #simple, I set time step=1, later it will be changed > 1 to classify from many packets in time step
y_train.shape = (4000,1,1)
How to transform my data to structure above?
Second, I think, the Embedding layer is not suitable to my problems, is it right?. My model is built:
model = Sequential()
model.add(LSTM(64, input_dim=41, input_length=41) # ex, 64 LSTM unints
model.add(Dense(1, activation=’sigmoid’))
model.compile(loss=’binary_crossentropy’, optimizer=’adam’, metrics=[‘accuracy’])
model.fit(X_train, Y_train, epochs=20, batch_size=100)
I’m new to LSTM, Can you give any advice for my problem. Thank you very much
It sounds like you have 40K time steps, these would then need to be split into sub-sequences of 100 samples of 400 time steps.
You would then have input like: [100, 400, 41].
The input shape would be (400, 41).
Does that help?
Thanks Jason. That means batch_size=100. Right? I can have my first layer like this:
model.add(LSTM(64, input_dim=41, input_length=400) #hidden 1: 64
Or:
model.add(LSTM(64, batch_input_shape=(100, 1, 41), stateful=True))
Which one is correct? How to set time_step in the first code line.
Can you help me fix that?. Many thanks
You can set the shape of your data in terms of time steps (x) and features (y) like this:
input_shape=(x, y)
Thanks for your enthusiasm,
I try to build model with my data that I follow your comments, but I get errors:
timesteps=2
train_x=np.array([train_x[i:i+timesteps] for i in range(len(train_x)-timesteps)]) #train_x.shape=(119998, 2, 41)
train_y=np.array([train_y[i:i+timesteps] for i in range(len(train_y)-timesteps)]) #train_y.shape=(119998, 2, 1)
input_dim=41 #features
#1.define the network
model=Sequential()
model.add(LSTM(100,input_shape=(timesteps,input_dim)))
model.add(Dense(1,activation=’sigmoid’))
#2. compile the network
model.compile(loss=’binary_crossentropy’,optimizer=’adam’,metrics=[‘accuracy’])
#3. fit the model
model.fit(train_x,train_y, epochs=100, batch_size=10,)
Error:
File “test_data.py”, line 53, in
model.fit(train_x,train_y, nb_epoch=100, batch_size=10,)
File “/home/keras/models.py”, line 870, in fit
initial_epoch=initial_epoch)
File “/home/keras/engine/training.py”, line 1435, in fit
batch_size=batch_size)
File “/home/keras/engine/training.py”, line 1315, in _standardize_user_data
exception_prefix=’target’)
File “/home/engine/training.py”, line 127, in _standardize_input_data
str(array.shape))
ValueError: Error when checking target: expected dense_1 to have 2 dimensions, but got array with shape (119998, 2, 1)
May be I have problem with ouput shape? how can I fix?
Thank you
The output of your network expects 1 feature. Reshape y to be (119998, 1).
Hi Jason,
I replaced my output shape to:
train_y=np.array(train_y[:119998) #train_y.shape=(119998, 1)
Finally, it works!
I have more question, Do Keras support for implementation on GPU?
Thanks
Glad to hear that.
Keras runs on top of Theano and TensorFlow. These underlying math libraries provide support for GPUs.
Hi Jason.
I think that maybe I was wrong when preparing input data to LSTM.
I have input and label like this: train_x(4000,41) and train_y(4000,1)
Before, I used:
timesteps=2
train_x=np.array([train_x[i:i+timesteps] for i in range(len(train_x)-timesteps)]) #train_x.shape=(119998, 2, 41)
train_y=np.array(train_y[:119998) #train_y.shape=(119998, 1)
===> It is wrong because rows are overlapped and train_y maybe taken wrong
Now, I correct like this:
train_x = reshape(int(train_x.shape[0]/timesteps), timesteps, train_x.shape[1])
In my data, each instance has multiple features so I want to keep features as it is, means multiple features in the same time.
Help me correct my misunderstand about input data
train_y = reshape(int(train_y.shape[0]/timesteps), train_y.shape[1]) # error: IndexError: tuple index out of range ???
And I concern the time feature is or is not included in input data (because I read a post: https://machinelearningmastery.com/time-series-prediction-lstm-recurrent-neural-networks-python-keras/).
I read many your articles in machinelearningmastery.com, so I maybe confused
Many thanks
Sorry, I’m not sure I follow your sequence prediction problem.
Can you give me a small example, e.g. one sample?
My data has n packets, each packet has many features f (one of them is time), example:
f1 f2 f3 … label
pkt1 2 3 3 0
pkt2 1 3 5 1
pkt3 2 3 2 1
pkt4 5 3 1 0
pkt5 5 3 2 1
….
ex: timesteps=2, each subsequence has 2 rows. After shape, like these:
[[[2 3 3 0]
[3 5 1 1]]
[[3 5 1 1]
[2 3 2 1]]
…. ]
or: separate:
[[[2 3 3 0]
[3 5 1 1]]
[[2 3 2 1]
[5 3 1 0]]
… ]
When split label from that input data. I see if timesteps=1, label will match to every rows, easy to get. But if timesteps >1, which label will be taken for matching to each subsequence (on 1st row or 2nd row).
Can you help me clear that confusion? (2 questions: overlap or separate? and get label)
Many thanks
Perhaps this post will help you prepare your data:
https://machinelearningmastery.com/convert-time-series-supervised-learning-problem-python/
Thanks Jason.
I know that post. That means preparing data for prediction model and classification model is the same?
The approach will help with preparing sequence data in general, not just time series.
Hi Jason
After considering carefully about preparing data for LSTM in Keras. I realise that term “feature” doesn’t mean its original meaning (also know as attributes, fields in dataset), actually it is the number of columns after converting multivariate Time Series into supervised learning data. It is based on real features and look_back, calculated as real_feature multiplied by look_back. Am i right?
I followed https://machinelearningmastery.com/multivariate-time-series-forecasting-lstms-keras/
.
Thanks Jason and machinelearningmastery.com
In time series, parallel series would be “features” and lag observations for one series would be time steps for the LSTM.
Hi Jason, nice article. I have one question though. what changes I have to make to do multi-class classification instead of binary classification?
Good question.
Change the output layer to have one neuron per class, change the activation function to be softmax on the output layer and change the loss function to be categorical_crossentropy.
Thanks for nice reply. One last question, can I use negative values for LSTM and CNN? I have some data, one of the column has both positive and negative values. How to handle this? Thanks in advance.
Yes.
Generally, I would encourage you to rescale data to the range 0-1 prior to passing it to an LSTM layer.
Hi Jason,
It seems that I encounter a problem with the line “model.add(LSTM(100))” (OS: MAC)
Here is the TypeError: Expected int32, got of type ‘Variable’ instead.
Thank you very much !!!!!!!
That is a strange error, are you sure it is on that line? It does not make sense.
Perhaps ensure you have copied all of the lines and that you have the correct spacing/indenting?
Hi Jason, thanks for your post. it’s really helpful.
I have some questions, hope you help out.
1. I’m trying to classify intents for a data set containing comments from user. There are several intents corresponding to comments. But the language in my case is not English. So I understand that I have to build the data set to be similar to imdb’s one. But how can I do it. Do you have any instruction/guidelines to build data set like that.
2. Aside from data set, I think that I also have to build embedding vector for my own language. How can I do that.
Thank you in advanced. Hope to hearing from you soon.
I should have some posts on this soon.
Generally, you need to clean the data (punctuation, case, vocab), then integer encode it for use with a word embedding. See Keras’ Tokenizer class as a good start.
The Embedding layer will learn the weights for your data. You can try to train a word2vec model and use the pre-trained weights to get better performance, but I’d recommend starting with a learned embedding layer as a first step.
Hello, Jason,
Thank you for the great post.
Google has it’s NLP API: https://cloud.google.com/natural-language/docs/basics
You could admit that they give us a polarity of sentiment in the range of (-1, 1). The call it “score”.
Maybe you have a quick idea about how to do the same output using Keras while sentiment analysis?
As I understand this is not a classifier problem anymore. Any thoughts?
Sure, I have a few posts scheduled on this topic for later in the month/next month.
Oops, I sent my reply to the wrong post. Sorry. I fixed it.
Hi Jason,
thank you for your nice work in this website.
My question: In what cases RNN works better than LSTM? I know that LSTM is originated from RNN and attempts to eliminate the problem of vanishing gradient in RNN.. BUT in my case I am using malware behavioral sequence and I got this chart for TPR and FPR: https://imgur.com/fnYxGwK , the figures show TPR and FPR for different number of units in hidden layer.
Do you know why RNN works better in my project?
An LSTM is a type of RNN.
Hi Jason,
First off, great tutorial. Love the overall content that you provide.
I am working through a categorical classification task that involves evaluating a feature that can go as long as 27500 words. My problem is that there are other features that I need to feed into my RNN-LSTM as well. I had thought about combining the long text feature and the other features into one files – features separated by columns of course but I don’t think that will work? Instead, I was think to separate the long text feature into its own file and run that independently through the RNN and then take the other features Can you give me some pointers on how I can go about designed the layers for this challenge I’m facing?
You will need to split up your sequence into subsequences of 200-400 time steps max.
I give ideas here:
https://machinelearningmastery.com/handle-long-sequences-long-short-term-memory-recurrent-neural-networks/
Hi,Dr. Jason Brownlee. Thanks for your amazing web. I’m a start-learner on deep learning. I copy your code and run it, and I encounter a problem when loading imdb dataset. The messages are as follows:
Traceback (most recent call last):
File “F:\Study\0-MyProject\Test\SimpleLSTM.py”, line 13, in
(X_train, y_train),(X_test, y_test) = imdb.load_data(num_words = top_words)
File “C:\Users\llfor\AppData\Local\Programs\Python\Python35\lib\site-packages\keras\datasets\imdb.py”, line 51, in load_data
path = get_file(path, origin=’https://s3.amazonaws.com/text-datasets/imdb.npz’)
File “C:\Users\llfor\AppData\Local\Programs\Python\Python35\lib\site-packages\keras\utils\data_utils.py”, line 220, in get_file
urlretrieve(origin, fpath, dl_progress)
File “C:\Users\llfor\AppData\Local\Programs\Python\Python35\lib\urllib\request.py”, line 217, in urlretrieve
block = fp.read(bs)
File “C:\Users\llfor\AppData\Local\Programs\Python\Python35\lib\http\client.py”, line 448, in read
n = self.readinto(b)
File “C:\Users\llfor\AppData\Local\Programs\Python\Python35\lib\http\client.py”, line 488, in readinto
n = self.fp.readinto(b)
File “C:\Users\llfor\AppData\Local\Programs\Python\Python35\lib\socket.py”, line 575, in readinto
return self._sock.recv_into(b)
File “C:\Users\llfor\AppData\Local\Programs\Python\Python35\lib\ssl.py”, line 929, in recv_into
return self.read(nbytes, buffer)
File “C:\Users\llfor\AppData\Local\Programs\Python\Python35\lib\ssl.py”, line 791, in read
return self._sslobj.read(len, buffer)
File “C:\Users\llfor\AppData\Local\Programs\Python\Python35\lib\ssl.py”, line 575, in read
v = self._sslobj.read(len, buffer)
TimeoutError: [WinError 10060] 由于连接方在一段时间后没有正确答复或连接的主机没有反应,连接尝试失败。
Besides, sometimes it just said “fetch failure on https://s3.amazonaws.com/text-datasets/imdb.npz“.
Is it because imdb data source is not available or network is instability?
Actually I have manually downloaded the data from https://s3.amazonaws.com/text-datasets/imdb.npz.
So if I cannot load the data online, how can I deal with the data I’ve downloaded manually to use it?
I’ve try another code to load data: (X_train, y_train),(X_test, y_test) = imdb.load_data(path = “imdb_full.pkl”), and it’s not work neither.
I’m looking forward to your reply. Thanks again!
Looks like you might be having an internet connection issue.
Try deleting the half downloaded file in ~/.keras/datasets/ (if present) and try again.
Thanks for your reply. Now I can load the dataset. I still have two questions and need your help:
(1) You mentioned that we can “reproduce the results” by using the code “numpy.random.seed(7)”, but I still got different accuracies every time. Is that right that how I understood the code “numpy.random.seed(7)”?
(2) The results I have got are always about 50.6%, which is lower than yours. Why is there so big gap?
Thank you, and I’m looking forward to your reply~
Perhaps this post will help with reproducibility:
https://machinelearningmastery.com/reproducible-results-neural-networks-keras/
Sorry to hear that, generally neural networks are stochastic, the best way to evaluate them is this process:
https://machinelearningmastery.com/evaluate-skill-deep-learning-models/
Hey Jason,
This is an amazing post. I’m very new to nnets and now I have a question.
I do not understand the why you have picked LSTM and RNN for this semantic analysis. to be clear I don’t understand where the sequential part that allow us to use RNN and LSTM.
I’m wondering if you could explain this.
I also want to know if we can use LSTM for entity extraction (NLP) and where is a good data set to train our model.
Sure, check out this post on sequence prediction
https://machinelearningmastery.com/sequence-prediction/
Also check this post on the promise of RNNs:
https://machinelearningmastery.com/promise-recurrent-neural-networks-time-series-forecasting/
I’m sure LSTMs can be used for entity extraction, I do not have an example. My advice would be to search google scholar.
Hi Jason,
Would Feature Scaling help in this case as well? As the reviews are tokenized, the values can go from low to high depending the max number of words used.
Sure.
Thanks for sharing both the model and the code also your enthusiasm in answering all the questions. I built my model for sentence classification based on your cnn+lstm one and it is working well. I am relatively new to neural nets and hence I am trying to learn to interpret how different layer interact, specifically, what is the data shape like. So, given the example above, suppose our dataset has 1000 movie reviews, using a batch size of 64, for each batch, please correct me:
embedding layer: OUTPUT – 64 (sample size) x 500 (words) x 32 (features per word)
conv1d: INPUT – as above; OUTPUT – for *each word*, 32 feature maps x (32/3) features, where 3 is kernel size.
maxpooling1d: INPUT – as above; OUTPUT – for *each word*, and for *each feature map*, a 32/3/2 feature vector
lstm: INPUT – this is where I struggle to understand… 64 is the sample size, 500 is the steps, so should be 64 x 500 x FEATURES, but is FEATURES=32/3/2, or 32 x (32/3/2) where the first 32 is the feature maps from conv1d?
OUTPUT – for *each sample*, a 100-dim feature vector
Sounds good.
I would encourage you to try a suite of models on your problem to see what works best.
Hello, read your blog found it really help full however could you please guide me to a code sample as to how exactly hot encode my text for training, I have 20,000 reviews to train.
Or can i just using hashing technique where every word is signifying an integer?
So something like ;
I find the store good.
I find good.
Is represented as ;
1 2 3 4 5
1 2 5
As representing every character with an integer would be exhaustive i think!
And then i can probably run the further steps for padding e.t.c?
In this case how will i predict new sentences having some new words?
(which makes me re think should i assign every character to an integer) if so could you please show me a sample?
I recommend using an integer encoding for text.
Further, you can count the occurrence of each word, and reduce the size of the vocabulary to only the most frequent words.
I will have posts on how to do this on the blog soon.
I tried to create a model for text summarization in seq2seq with keras. Did not work well. The prediction shows the top words by frequency. I tried blacklisting the top words in english (‘a’, ‘an’, ‘the’ etc). The results were still not good. Some said that in 2016 that keras was not good for text summarization then. Wonder what is missing.
It is a hard problems that requires at least 1M examples and a large model.
I have a tutorial on text summarization scheduled for around Christmas.
Hello sir i am asad. i want to know how to load data set which is in .text file and text data of movie review. then how i can use it in recurrent neural network?
please tell me the complete procedure. remember data i have is locally in my computer
This post will show you how to encode the text for use with an LSTM:
https://machinelearningmastery.com/prepare-text-data-deep-learning-keras/
Hi Jason,
Thanks for the post. I just applied this approach in our use case which is quite similar to movie review sentiment classification. The accuracy of the model is very good ~94%.
BUT
I replaced all the frequency with random numbers and to my surprise the accuracy is still very good. (~94). The labels are the same as well.
Do you have any idea about this?
Thanks,
What do you mean exactly, I don’t follow what you changed?
Hey Jason,
amazing work and so up to date.
I would like to ask you, do you think this sequence classification model could be used to predict a category for a really large sequence of numbers, instead of words ??
Yes. Although, for very long sequences (>400 time steps) you may need to split them up into subsequences to fit your model.
See this post:
https://machinelearningmastery.com/handle-long-sequences-long-short-term-memory-recurrent-neural-networks/
Thanks a lot, I’ll give it a try !
Hi Jason,
I’m really puzzled. I seem to be the only one who can’t run the code you provided.
I’m using python 2.7, Keras-2.0.8, Tensorflow-0.12. I got an error at the line
model.add(LSTM(100)).
TypeError: expected int32, got list containing Tensors of type’_Message’ instead.
Can you please let me know which python, keras, tensorflow versions you’re using?
Thank you!
It looks like you need to upgrade your version of TensorFlow to at least 1.3 or better.
Hi jason,
I would like to let you know that I have written my first ML code following your step by step ML project. I am using a nonlinear dataset(nsl-kdd). My dataset is in CSV format. I want to model and train my dataset using lstm.
For MNIST dataset I have a code,
import tensorflow as tf
from tensorflow.examples.tutorials.mnist import input_data
from tensorflow.python.ops import rnn, rnn_cell
mnist = input_data.read_data_sets(“/tmp/data/”, one_hot = True)
hm_epochs = 3
n_classes = 10
batch_size = 128
chunk_size = 28
n_chunks = 28
rnn_size = 128
My question is according to my dataset how I can define the chunk size, number of chunks, and rnn size as new variables for my dataset.
As I am very much new so really confuse how I can model and train my dataset to find accuracy using lstm. I want to use LSTM as a classifier. I don’t know my questions to you is correct or not.
I really appreciate your help.
Sorry, I don’t have examples of working with tensorflow directly. I cannot give you good advice.
is it possible to written same code for Simple neural networks for text processing?
is it that best way to use keras for text processing or otherwise any other libraries are present to implement Neural networks for text processing.?
Yes, perhaps start with this post to prepare your text data:
https://machinelearningmastery.com/prepare-text-data-deep-learning-keras/
Hi Jason,
This post and the comments have helped me immensely. Thanks! I am question regarding this sentence –
“The IMDB review data does have a one-dimensional spatial structure in the sequence of words in reviews and the CNN may be able to pick out invariant features for good and bad sentiment. This learned spatial features may then be learned as sequences by an LSTM layer.”
I am not able to visualize how CNN will process words. Also, Could u please throw some light on spatial structure for words?
Words are ordered in a sentence or paragraph, this is the spatial structure.
For sequence to sequence mining ,which neural networks is better for good performance?
LSTMs.
i have the read about sequence to sequence learning in neural networks,we need to LSTMS layers for it,first one is for input sequence and second is for output sequnce,here we have to send our input sequnce vector in a reverse order to LSTM layers.
what my doubt ,is LSTM layer will take the input in a reverse order or we have to give input in reverse order
Yes, you can reverse the order with the go_backwards argument to the LSTM layer:
https://keras.io/layers/recurrent/#lstm
For sequence to sequence regression model ,output node i have to give one or i have to give maximum variable length of output vectors,.
finally we will get output vectors,how we have to convert to this output vectors to text ,is there any method is available in Keras ,like in embedding layer we are doing strings to vectors conversion,like vectors to integers conversion.
To output text, you use a softmax to output the prob of each char or word, then take the argmax to get an integer and map the integer back to a value in your vocabulary.
I will have examples of how to do this on the blog soon.
problem statement: my model should be generate the script file according to given instrctions using sequence to sequence modelling using keras..
examples:input: take two intergers from console,add two integers,print the addition of two integers on console.
output: like python script file for above input instructions.
Please give me any point of contact for this problem,how can i go further to solve this problem.
Here is an example:
https://machinelearningmastery.com/learn-add-numbers-seq2seq-recurrent-neural-networks/
Is it possible to use machine learning to translate natural language into a programming language, say, C, PHP, or Python? please suggsest me any libraries available to do this task.
Perhaps.
You could look into using LSTMs as text generator for sequence to sequence learning. Start here:
https://machinelearningmastery.com/start-here/#lstm
Dr. Brownlee, I can’t tell you how much I value the content on your site! So accessible, to the point, and enriching. You’re changing the world. Thank you.
Thanks Tamir!
Great tutorial!
But how can i use this network to classify several different classes? For instance 14 classes.
Am i correct – that i just need to change – model.add(Dense(1, activation=’sigmoid’))
to model.add(Dense(13, activation=’sigmoid’))
or i need use Conv2D?
And how can i transform my text data to word embedding (such as IMDB uses).
To change the example to work for a multi-class classification problem, change the output layer to have one neuron per class, and use the categorical_crossentropy loss function.
Thanks for your great example!
I got some troubles with overfitting my model –
For the training i am using, text data in Russian language (language essentially doesn’t matter,because text contains a lot of special professional terms, and sadly to employ existing word2vec won’t be an option.)
I have such parameters of training data – Maximum lengths of an article – 969 words Size of vocabulary – 53886 Amount of labels – 12 (sadly they are distributed quite unevenly, for instance i have first label – and have around 5000 examples of this, and second contains only 1500 examples.)
Amount of training data set – Only 9876 entries. I’ts the biggest problem, because sadly i can’t increase size of the training set by any means (only way out to wait another year☻, but even it will only make twice the size of training date, and even double amount is’not enough)
Here is my code –
x, x_test, y, y_test = train_test_split(x_, y_, test_size=0.1)
x_train, x_dev, y_train, y_dev = train_test_split(x, y, test_size=0.1)
embedding_vecor_length = 100
model = Sequential()
model.add(Embedding(top_words, embedding_vecor_length, input_length=max_review_length))
model.add(Conv1D(filters=32, kernel_size=3, padding=’same’, activation=’relu’))
model.add(MaxPooling1D(pool_size=2))
model.add(keras.layers.Dropout(0.3))
model.add(Conv1D(filters=32, kernel_size=4, padding=’same’, activation=’relu’))
model.add(MaxPooling1D(pool_size=2))
model.add(keras.layers.Dropout(0.3))
model.add(Conv1D(filters=32, kernel_size=5, padding=’same’, activation=’relu’))
model.add(MaxPooling1D(pool_size=2))
model.add(keras.layers.Dropout(0.3))
model.add(Conv1D(filters=32, kernel_size=7, padding=’same’, activation=’relu’))
model.add(MaxPooling1D(pool_size=2))
model.add(keras.layers.Dropout(0.3))
model.add(Conv1D(filters=32, kernel_size=9, padding=’same’, activation=’relu’))
model.add(MaxPooling1D(pool_size=2))
model.add(keras.layers.Dropout(0.3))
model.add(Conv1D(filters=32, kernel_size=12, padding=’same’, activation=’relu’))
model.add(MaxPooling1D(pool_size=2))
model.add(keras.layers.Dropout(0.3))
model.add(Conv1D(filters=32, kernel_size=15, padding=’same’, activation=’relu’))
model.add(MaxPooling1D(pool_size=2))
model.add(keras.layers.Dropout(0.3))
model.add(LSTM(200,dropout=0.3, recurrent_dropout=0.3))
model.add(Dense(labels_count, activation=’softmax’))
model.compile(loss=’categorical_crossentropy’, optimizer=’adam’, metrics=[‘accuracy’])
print(model.summary())
model.fit(x_train, y_train, epochs=25, batch_size=30)
scores = model.evaluate(x_, y_)
I tried different parameters and it gets really high accuracy in training (up to 98%) But i really performs badly on test set. Maximum that i managed to achieve was around 74%, usual result something around 64% And the best result was achieved with small embedding_vecor_length and small batch_size.
I know – that my test set is only 10 percent of training test, and overall data-set is the biggest problem, but i want to find a way around this problem.
So my questions are – 1) Is it correctly builded model for text classification purpose? (it works) Do i need to use simultaneous convolution an merge results instead? I just don’t get how the text information doesn’t get lost in the process of convolution with different filter sized (like in my example) Can you explain hot the convolution works with text data? There are mainly articles about image recognition..
2)i obliviously got a problem with overfitting my model. How can i make the performance better? I have already added Dropout layers. What can i do next?
3)May be i need something different? I mean pure RNN without convolution?
Perhaps try using cross-validation to get a more robust estimate of model skill.
Perhaps explore simpler CNN based approaches, here is a good start:
https://machinelearningmastery.com/develop-word-embedding-model-predicting-movie-review-sentiment/
Perhaps explore general deep learning tuning methods:
https://machinelearningmastery.com/improve-deep-learning-performance/
I hope that helps as a start.
How would you do sequence classification if there were no words involved? For example, I want to classify a sequence that looks like [0, 0, 0.4, 0.5, 0.9, 0, 0.4] either to be a 0 or a 1, but I don’t know what format to get my data in to feed into an LSTM.
Perhaps start here Alex:
https://machinelearningmastery.com/start-here/#lstm
Hi,
What if we need to classify a sequence of numbers, is this example applicable and do i need the embedding layer? and can you refer to an example that you have on the blog or on other places so i can understand more? Thanks
An embedding layer would not be required.
Hi.
Nice tutorial buddy. Please can you show how to use this LSTM network with a Binary classification problem (like your tutorial on neural networks – prima indian diabetics).
Please can you help me..
It would not be a fit for that dataset as there is no sequence information.
You can get started with LSTMs here:
https://machinelearningmastery.com/start-here/#lstm
Thanks Jason
Hi,
I tried sequence classification, but I am not able to add LSTM layer on top of embedded layer.
Did you faced a similar issue ?
Here is the problem that I am facing : https://stackoverflow.com/questions/47464256/unable-to-add-lstm-layer-on-top-of-embedded-layer-on-gpu-keras-with-tensorflow
Here’s an example with the functional API:
Taken from here:
https://machinelearningmastery.com/develop-a-caption-generation-model-in-keras/
Hi Jason,
Thanks for the tutorial. Can you clarify however, when you say:
“We can see that we achieve similar results to the first example although with less weights and faster training time.”
When you mean less weights, what are you referring to exactly? cause when you run model.summary the model with Convolution layer has 216k parameters vs. 213k parameters in the original model, technically there are more parameters to train.
Do you mean to say that with the convolution + pooling layers the input into the LTSM layer is from 250 hidden layer nodes vs 500 in the original model? I’m guessing the LTSM layer is harder to train which leads to the reduced fitting time?
Thanks
Hi
I tried text classification. I have data sets of tweets and I have to train a model to determine the writer was happy or sad. I used your “Simple LSTM for Sequence Classification” code . but the thing is that I want to know before using your code what should I replace with words .
previously I used ” sequences = tokenizer.texts_to_sequences(tweets_dict[“train”])” to convert text to vector and after that I used your code . Is it correct?
See this example:
https://machinelearningmastery.com/develop-word-embedding-model-predicting-movie-review-sentiment/
Real informative and fantastic anatomical structure of subject matter,
now that’s user friendly (:.
Thanks!
Do you mind if I quote a few of your posts as long as I provide
credit and sources back to your website? My blog site is in the exact same area of interest as yours and my users would really benefit
from a lot of the information you provide here.
Please let me know if this okay with you. Many thanks!
Sure, as long as you do not copy posts verbatim (e.g. just small quotes) and you credit the source clearly.
Very nice article. Can you tell me how to make single prediction ? Like for a given text we have to make prediction.
e.g. “Very nice movie” as single input to give “positive” output.
Yes, see this post:
https://machinelearningmastery.com/make-predictions-long-short-term-memory-models-keras/
Hi Jason,
In my problem I have made an one hot encoding with a vector size of 256 for each sample (10000 samples). The embedding layer is necessary? What I have done as the first layer:
model.add(LSTM(256, input_shape=(10000, 256), activation = ‘relu’))
You did model.add(LSTM(100)) too. It has any relation with the embedding_vecor_length? It has to be greater than embedding_vecor_length = 32? I am using 256 but without any idea. Thank you.
Perhaps try your model with and without the embedding to see how it impacts model skill.
Thank you sir, for providing the very nice tutorial. I am working on sequence classification. My data set contains 41 features, each of them are float and Y is 5 class .
Q.1 Do i need embedding ?
Q.2 I have normalized the data , so do i need top_words ?
Q.3 What could be embedding vector length?
Q.4 What could be the maximum review length ?
Q.5 All example contains 41 features, do i need padding ?
I am not very clear about the embedding layer. Your suggestions would be great for me.
Too many questions for one comment!
Generally, I cannot tell you what will work best for your problem, you must experiment to discover what works best. See this post:
https://machinelearningmastery.com/applied-machine-learning-as-a-search-problem/
Hello, suman , I got same situation just like you
My data set have 8 features and 100,000 obs, I have to classify these sequence data
into 4 different class.
But I have no idea with embedding ‘Vector Length’, ‘Maximum review length ‘ etc.
If you fixed your problem, could you tell me how you solved ?
Any comments or advices would be appreciated .
Here are some approaches for working with very long sequences:
https://machinelearningmastery.com/handle-long-sequences-long-short-term-memory-recurrent-neural-networks/
I have one small doubt. You are using the IMDB data set. If i want to use a different data set then how to pre-process the data set for preparing the word integer matrix to execute the following:
# load the dataset but only keep the top n words, zero the rest
top_words = 5000
(X_train, y_train), (X_test, y_test) = imdb.load_data(num_words=top_words)
# truncate and pad input sequences
max_review_length = 500
X_train = sequence.pad_sequences(X_train, maxlen=max_review_length)
X_test = sequence.pad_sequences(X_test, maxlen=max_review_length)
My data (two columns in .csv format: tweet and CLASS/manual annotation) looks like this:
president obama says the us needs to do more to help stop the ebola outbreak from becoming a global crisis actdont talk RISK
i was upset and angry that thomasericduncan lied about his exposure to ebola and put us all at risk for catching this deadly disease RISK
ebola is transmitted through blood and saliva so i better stop punching my haters so hard and smooching all these gorgeous b TRANSMISSION
he got the best treatment availablebetter than liberia and i am still not convinced he didnt know he had ebolarace card again TREATMENT
obama and cdc said they will fight ebola in africa news today ebola deaths rise sharply when exactly will they fight it tcot TREATMENT
fuck this is really tough dont know if i have the mind and guts to deal with death and ebola every day of work RISK
something more serious needs to be done about this ebola shit the airport and the town he was in needs to be quarantined im sick of being PREVENTION
if you have ebola symptoms or know someone who does please hug and kiss mr obama show him respect he appreciates tcot SYMPTOM
u can only get it if u have frequent contact with bodily fluids of someone who has ebola and is showing symptoms TRANSMISSION
See an example here:
https://machinelearningmastery.com/develop-word-embedding-model-predicting-movie-review-sentiment/
Hi Jason, I would like to know after building a model using ML or DL how to use that model which can automatically classify the untagged corpus? is there any example?
Regards
Yes, learn more about a final model here:
https://machinelearningmastery.com/train-final-machine-learning-model/
Learn how to save and load a DL model here:
https://machinelearningmastery.com/save-load-keras-deep-learning-models/
Hi Jason,
Thank you for your great effort,
I am trying to use Keras LSTM, but I dont know the data format.
I have an FAQ list, the questions are considered samples and the answers are considered classes. So how can I use the lstm classifier for this dataset.
thanks in advance
See this post:
https://machinelearningmastery.com/reshape-input-data-long-short-term-memory-networks-keras/
Hi Jason,
I have a classification problem that has two types of input.
The first input is the sequence of online activities, which I can use the above mentioned models to deal with.
The second input is a vector of the time difference (minute) between each activity and last activity. In this case, I want my model consider the time impact of the decision as well.
My question is what is the best way to merge the second input to the above models?
What I have done is use a LSTM layer on the second input as well and merge the output with the above one. But it seems not right, because the second input is continuous value rather than the discrete index.
So what kind of layer should I use to apply on these real value vectors?
Perhaps try a suite of models and see what works best.
Perhaps a multi-headed model might be a good approach.
Hi Jason,
How to take two types of inputs in this model?
One is a sequence of online activities, the second input is the time different between each activity and last activity.
Should I use a multimodal layer to merge them?
Should I process the second input with LSTM layer as well? (It seems not right as the element of this vector is the continuous value)
Cheers,
R
See this post for examples:
https://machinelearningmastery.com/keras-functional-api-deep-learning/
Thanks for your response. I understand how to merge two layers, but my question is, in which layer shall I merge the online activities with their recency scores?
For example I can apply a lstm layer on the online activities, and then concatenate the output of lstm layer (the last hidden state output) with the sequence of their recency scores. But it doesn’t make sense.
Or I can multiply the embedding output with the sequence of their recency scores, then put the output into the lstm layer. But I don’t know whether this right or not.
Would please give me some suggestion?
Thanks,
Ray
My intuitions might lead you down a false path. Perhaps try a few designs and see what works best for your specific problem.
There is more art than science in this at the moment.
Fair enough. But thanks a lot. I will use this as the excuse when I have to talk with my professor about progress 😀
Hi,
I can implemented a LSTM to generate labels from videos? for example use youtube2text?
thanks
Sure.
Can I use this to for Lip Reading? I’m thinking of classifying a sequence of frames to a particular word. Like the entire video will be classified as hello, how, etc.
Can you tell me how to go about it?
Sounds great. Sorry, I don’t have any examples of lip reading models.
Hi Jason: Your teaching skills far exceed many ‘big’ teaching names.
As an experiment, I added one line to the model in your “simple” LSTM example.
model.layers[0].trainable = True # to train (back-prop) thru the embedding layer
While the trainable parameter count went up significantly (from 53,301 to 1,660,501), the accuracy did not change.
Would like your thoughts on the experiment.
The layer is trainable by default. The assignment should have had no effect. I’m surprised.
Jason,
Thanks for you excellent explanation.
I’ve done some modification on your codes in oder to get higher accuracy on the test data, finally, I could get accuracy 88.60% on test dataset.
My question is, besides what I’ve done on changing thoese hyper parameters (just like a blind man touching an elephant), what else we could to do improve the prediction accuracy on the test data? Or how to conquer the overfitting to get higher prediction accuray on test data? I found it’s very easy to get higher prediction accuracy on training data, but it’s astonishingly hard to make the same result happen on the test dataset(or validation dataset). The codes I modified is as following if anyone else need them as reference:
Thanks!
Clock ZHONG
Well done, here are some more ideas:
https://machinelearningmastery.com/improve-deep-learning-performance/
Thanks, Jason, that article you wrote, I already carefully read it half year ago. It’s also perfect, but I still feel we have no a clear guide on how to impove the prediction accuracy on test dataset.
We always say:
1. more training and testing data could get better performance, but it’s not always.
2. more deeper layers in the neural network could get better performance, but it’s still not always;
3. Fine tune hyper parameters could get better performance, yes ,it is, but let alone the time comsumption, this kind of work could only imporve the performance very very little (according to my experience.)
4. Try more other architecture neural network algorithms. Yes, sometimes this could work, but soon we’ll get to the upper-limit again. and face the same problem at once: how to impove it then?.
Conquering overfitting is really an interesting but difficult work in neural network, I feel we could find some better working ways to fix this problem in the future.
I still appreciate your articles and reply. Have a happy weekend.
Thanks
Clock ZHONG
Yes, it is hard and empirical. That is the nature of the job.
There are no clear answers and no one can tell you how to get the best result for a given dataset. You must discover it.
Thanks a lot Jason for your great post. I have difficulty of understanding how LSTM can remember long-term dependencies. Or maybe, I misunderstood the meaning of “remembering dependencies”. Does it remember different parts within a specific training data or among different training data?
For example, if we have 100 training data, does it learn from 81st data by remembering previous training data?
Thanks a lot for your time and help in advance,
I have an example that makes it clear here:
https://machinelearningmastery.com/memory-in-a-long-short-term-memory-network/
I’ll read it, thanks a lot.
Jason:
Great article! It helps me a lot.
However, I don’t understand why dropout is considered to play a positive role while reducing the accuracy rate.
It can help in general, this this post we are demonstrating how to implement it.
hello,
Thanks for the article.could you provide an idea on how to apply LSTM for handwriten images recognition.I have a dataset of handwriten alphabets as images of size 50*50.
It would also be helpful if i could know how Lstm helps handwriten text recognition
Thank you,
Sure, see this post:
https://machinelearningmastery.com/handwritten-digit-recognition-using-convolutional-neural-networks-python-keras/
Thanks for the help
1.The code uses convolutional neural network.what changes should I make to use recurrent neural network(LSTM).
2.How to load custom datatset of images for training and testing instead of mnist data set.
LSTM could be used for a sequence of images, but a CNN would still be used on the front end.
See this post for a CNN LSTM:
https://machinelearningmastery.com/cnn-long-short-term-memory-networks/
Thank you for this great work! Can we apply it for french language?
Sure.
Hi,
great article. I have a rather fundamental question. As I understand it, each sample is here a sequence of the lenght of “max_review_length”. However, if I have a one dimensional sequence, each sample is part of the sequence. My question is basically, how to tell the algorithm which dimension the sequence takes place.
Here, we feed in samples which are not part of the sequence themselves but they contain the sequence. But in other use cases it seems like we feed in samples in a sequence, and the samples themselves form the sequence. And we can even feed in a sequence of multiple dimensions, like multiple paralell time series, which is only a sequence in the first dimension.
I am a bit confused about this, in my mind the algorithm should only recognize the sequence along one dimension, would be great if you could clarify.
Thanks
Not sure I follow.
Perhaps this post will make inputs to the LSTM more clear:
https://machinelearningmastery.com/reshape-input-data-long-short-term-memory-networks-keras/
Ok, I will try to clarify. Say we have a sequence of 5 values. We can pass the sequence in one by one, shape (5,1,1) or all 5 points in one go (1,5,1) as a vector of lenght 5. However, are both of these considered a sequence?
In my mind, the first one is a sequence of 5, while the second is 5 parallel sequences of lenght 1. This is relevant because in the example of sentiment, we have N samples of lenght “max_lenght”, ie shape (N, max_lenght, 1). Or maybe (N, max_lenght, embedding_dim) if we use embeddings.
If the sequence is in the first dimension, ie that of N, then LSTM doesnt make sence because there should be no sequential relationship between different reviews.
Thanks
No, the first is 5 sequence the second is 1 sequence. Regardless, LSTMs process only one time step of data as input at a time.
One batch is comprised of 1 or more sequences (samples, first dimension).
Weight updates occur at the end of each batch at which time internal state is cleared. This means, there is knowledge across sequences. Or can be if that is desired.
Ok, I get it. Thanks for clarifying. Keep up the good work.
No problem.
Hi Jason,
I just start learning ML and trying some sample projects on keras. This post is a really good example to follow.
I have a question about the classification problem. Right now, I am trying a two-class sequence classification problem. I followed this tutorial to build a model with loss function as binary cross entropy. Then I change the output layer to have 2 units, change the loss function to categorical cross entropy and change the y_training to one hot encoding. I expect these two methods give me the same accuracy but actually, the categorical one seems to be more accurate. Do you have any idea of why this happens? From my understanding, binary cross entropy is the same with 2-class categorical cross entropy so these two methods should give me the same result.
Another problem. I read another post on your website and change the input layer to LSTM. Then I truncate the training data. I use the full training data to do validation. The truncated training data gives me a higher accuracy when validating than the model using the full training data. I use binary cross entropy method here. This is not what I expect. I am also wondering how to decide the type of the input layer?
I really appreciate it if you could spend any time answering my question.
It might allow the model to be more expressive (e.g. more weights in the calculation of the output).
Not sure I understand the second question, perhaps you can give a very short example?
Would this model is good for predicting that user has perfom this activity or not.? because i want to develope a model that predict that user has performed this activity or not.i want to train the model on user activity like jumping and test whether the user jumping or not.can this model help me out or do you have any code for this.?thanks seeking for help.regards sardar khan.
Perhaps try it and see.
Can you give me an example of this.
Sorry, I do not have a worked example of your problem.
I am not able to clearly understand how exactly is binary classification happening here? The following is the questions that I am trying to figure out:
For classification, is the final output by the final word in LSTM being given to the single neuron dense layer ? If so, in another one of your post related to “Text generation using LSTM” you seem to be creating an output dense layer with number of neurons equal to the number of words in the vocabulary. But in case of text generation you need the output such that a given memory unit predict the next appropriate word. Then how is a dense layer exactly being connected to the LSTM layer and how exactly is it working(since the LSTM layer seems to give only the final output of final word)?? Please help me both these question
Yes Jason, this is a question that even I am troubled with. Can you please explain how the dense layer is “CONNECTED” with the LSTM layer in these two different situtations(“Sequence classification” and “Text generation”).
Thank you in advance
Ankita
This example is classifying sequences of words as a sentiment good/bad.
It is different from generating text (outputting a sequence of words).
Does that help?
Thank you Jason for your reply.
But can you explain how exactly the connection between the LSTM layer and the dense layer differs in both the situations ??
How do you mean exactly?
Hi ..
nice work .. but how could we enter single review and get its prediction ?
You must prepare the single input as you would any training data.
Here’s some pseudocode that will help:
Hi Jason,
Thanks for the great post. I’m trying to implement a classifier like yours, but training on different data (logfiles) with another input shape. I got several lines of data, each with 9 features, each padded to a MAX_FEATURE_LEN. This works fine for LSTM layers, but as soon as i add the Embedding or the Dense layer, i get an error like:
Error when checking target: expected dense_1 to have 2 dimensions, but got array with shape (2000, 9, 256)
My current model:
features = 9
MAX_FEATURE_LEN = 256
model = Sequential()
model.add(Embedding(file_len(TRAIN_PATH), features, input_length=MAX_FEATURE_LEN))
model.add(Dropout(0.2))
model.add(LSTM(100, return_sequences=True))
model.add(Dropout(0.2))
model.add(Dense(1, activation='sigmoid'))
model.compile(loss='binary_crossentropy', optimizer='adam', metrics=['accuracy'])
I’ve tried several things and it works for LSTMs, so i don’t get what distinguishes them from Dense layers input_shape-wise
Thank you in advance
Adrian
Perhaps take a step back and skill up on LSTMs for NLP:
https://machinelearningmastery.com/start-here/#nlp
Great post and a very readable guide on LSTM-CNN using Keras.
Recently I’m working on a binary classification task which takes real numbers data from multi sensors. I was inspired by your post and wonder if it is possible that I arrange these data into a image-like matrix, which each row is a vector from one sensor and several rows for data from different sensors and then using model like LSTM or CNN or LSTM+CNN from your post to classify the data.
Do you think it is feasible for model to learn or ? Thanks for your post again~
Perhaps multiple 1-d CNNs would make more sense?
I would recommend trying it rather than thinking too much about whether it is feasible, e.g. Keras is so easy that you could prototype it in a few minutes.
Nice tutorial, Jason. It got me started with using LSTMs in Keras!
Are there any thumb rules for how many LSTM units to use for a classification problem? Does the length of the input sequence have any bearing number on this number?
Good question.
No good heuristics for configuring the number of units or layers. No relationship between input length and number of units in the hidden layer.
I recommend careful and systematic experimentation to see what works best for your specific dataset.
nb_words has been replaced by num_words
Thanks, fixed.
also nb_epoch was replaced by epochs
Thanks, fixed.
Rookie Query:Can this model predict certain pattern of the sequence like x,x^2,x^3,sin(x),etc all the combination of these sequene?
A model could perhaps be trained to learn those sequences.
Dear Jason,
Kindly can you help me in how to “upload my own dataset in keras” because I want to work on my own dataset. Thanks for your time.
This post will show you how to load your own CSV data into Python:
https://machinelearningmastery.com/load-machine-learning-data-python/
Dear Jason,
The keras contain predefined datasets like “imdb”,”cifar” etc.I want to know can I include my own dataset into keras dataset.
You can load your data into numpy arrays and start using it with Keras.
I have many examples of this on the blog for CSV data and text data.
I am a bit confused of how the LSTM is trained.
What is the input to the LSTM at each timestamp, is it the whole review (a 500 x 32 matrix?) or a word ( 32 dimension vector)?
What does a LSTM do in each epoch?
And how is the 100 neurons in the LSTM used? Can we use only 1 neuron for the job since it is recurrent?
Many thanks!
The LSTM takes a training dataset of samples comprised of time steps and features or [samples, timesteps, features]
Does that help?
I have the same doubt.. can you please elaborate?
You can learn more about how to prepare data for LSTMs here:
https://machinelearningmastery.com/faq/single-faq/how-do-i-prepare-my-data-for-an-lstm
i have a data set of 25000 length and i choose top 2500 length and consider it as x_train but i am confused with embedding layer:argument – vocab size should be what .. if i choose 2500 then remaining vocab are not including in this and giving the error
”
InvalidArgumentError: indices[23,2433] = 80188 is not in [0, 80000)
[[Node: embedding_59/embedding_lookup = GatherV2[Taxis=DT_INT32, Tindices=DT_INT32, Tparams=DT_FLOAT, _class=[“loc:@training_42/Adam/Assign_2″], _device=”/job:localhost/replica:0/task:0/device:CPU:0″](embedding_59/embeddings/read, embedding_59/Cast, training_42/Adam/gradients/embedding_59/embedding_lookup_grad/concat/axis)]]”
and
cannot download the data by this code line :
(X_train, y_train), (X_test, y_test) = imdb.load_data(num_words=top_words)
error showing name or service is not known .
please help asap
Perhaps try posting your code and error to stackoverflow?
Hi Jason,
I have 32 sentence blocks of 500 words each to pass to an LSTM after using a pretrained word2vec model to get embeddings of 400 words each. How can I achieve this so that the 32 features are learnt simultaneously?
Thanks!
Namrata
The number of units in the first hidden layer is unrelated to the size of your data.
You can learn more about how to prepare data for LSTMs here:
https://machinelearningmastery.com/faq/single-faq/how-do-i-prepare-my-data-for-an-lstm
Hi,
In pad_sequences, dtype of output is int32 by default. Shouldn’t we change it to float32 if we are feeding in word vectors?
Thanks
No, feeding the int mapping of words to the mapping is what we want, unless I misunderstand your question.
after finishing the model testing it gave an 84% accuracy
however when i tried to predict sentences using this code :
text = ‘It is a bad movie to watch’
text = preprocessing.text.one_hot(text, 5000, lower=True, split=’ ‘)
text = [text]
text = preprocessing.sequence.pad_sequences(text, 500)
predictions = model.predict(text)
print(predictions)
the result was 0.90528411
and when i change the sentence to ‘It is really a good movie to watch’
the prediction was 0.88954359
so is there`s a problem with code of prediction or i missed up the training
It is important that the new text is prepared in the same way as the text used to fit the model.
We don’t have information on how the imdb dataset was prepared.
I’d recommend this tutorial instead:
https://machinelearningmastery.com/develop-word-embedding-model-predicting-movie-review-sentiment/
thank you for replying and thank you for those great tutorials they are really useful and informative it really helped me a lot
Hi Jason,
Great work and splendid efforts! Really appreciate.
I am interested in sequence classification to analyse malwares using rnn-lstm and Tensorflow. While there are a couple of sources, I always find your blogs very readable and easily comprehensible. Hence, request you to come up with a blog on ‘Sequence Classification using RNN-LSTM in Tensorflow.’
Thanks Matt.
Dear Jason,
I want to know that in deep learning( RNNLSTM) models what should be the difference between training and testing accuracy in order to develop a good fit model.
and kindly tell me that my model is a good fit or not.
In [10]: model.fit(X_train, Y_train, epochs = 7, batch_size=batch_size, verbose = 2)
In [11]: score,acc = model.evaluate(X_test, Y_test, verbose = 2, batch_size = batch_size)
print(“score: %.2f” % (score))
print(“acc: %.2f” % (acc))
Epoch 1/7
1109s – loss: 0.6918 – acc: 0.5056
Epoch 2/7
971s – loss: 0.6269 – acc: 0.7041
Epoch 3/7
693s – loss: 0.3696 – acc: 0.8639
Epoch 4/7
594s – loss: 0.1743 – acc: 0.9388
Epoch 5/7
534s – loss: 0.0699 – acc: 0.9800
Epoch 6/7
473s – loss: 0.0276 – acc: 0.9950
Epoch 7/7
472s – loss: 0.0148 – acc: 0.9963
Out[10]:
score: 0.62
acc: 0.82
Thanx for your help.
I explain how to determine if a model is a good fit or not here:
https://machinelearningmastery.com/faq/single-faq/how-to-know-if-a-model-has-good-performance
Dear Jason,
I have a query that this accuracy
# Final evaluation of the model
scores = model.evaluate(X_test, y_test, verbose=0)
print(“Accuracy: %.2f%%” % (scores[1]*100))
is the predicted accuracy of the model?
Yes.
HI Jason,
Thanks for the tutorial it was really helpful.
I have question,for example I am dealing in total with 500 messages.These messages are grouped into certain patterns.some times 6 messages make one pattern A.And some times next 3 messages make one Pattern B.I need to classify the patterns in that 500 messages.
I trained model with LSTM given input shape of pattern containing highest number of messages and padded other patterns.I used sliding window concept and used multi label classification.
While testing when i give a file with 150 messages,During sliding the window ,some time non of the patterns may occur in that window but lstm model is classifying it as some known pattern.So how to overcome this issue.
Thanks in advance.
Perhaps you can have a “no pattern” output for those cases and train the model on them?
Appreciate your reply Jason . There are so many unknown patterns when compared to known patterns if i have to train with unknown class too.So it is facing class imbalance problem and always giving output as unknown class.
We only train the model on data where we know the output.
Dear Jason
Thanks for the tutorial do you have other example of tutorial that use Convolution lstm on time series dataset?
Thanks
I have a number of posts scheduled. Until then, perhaps this will help:
https://machinelearningmastery.com/cnn-long-short-term-memory-networks/
Nice explanation!
How do I construct a vocabulary just as like as imdb dataset format.
Can you give some form of pseudo code?
Thanks
Perhaps this will help:
https://machinelearningmastery.com/prepare-text-data-deep-learning-keras/
Dear Sir,
I want to know that what are the parameters or factors of the CNN model that allows the CNN+LSTM architecture to produces an accuracy of 86.36%.In other words, factors affecting the accuracy of the model when using the CNN model. Thanks…
Many many things, this may help:
https://machinelearningmastery.com/improve-deep-learning-performance/
Dear Jason
First thanks a lot for your effort. I just start learning different algorithms and your post helps me a lot.
I follow your LSTM post where I tried y_pred = model.predict(X_test) .
But it gives me continuous vale rather 0 or 1. Where do i need to change for binary output. Thanks
I wish you a happy time.
Best
Rashid
Perhaps your model is configured to predict a continuous value?
Thanks for your reply. Yeah I think so. I just copy and paste your code as well as the data you also used. I’m just learning. I didn’t change anything of your code. Could you give me the idea where I have to change for binary result.
Sure, this will help:
https://machinelearningmastery.com/faq/single-faq/how-can-i-change-a-neural-network-from-regression-to-classification
Sorry Im new to NN but can I use this to identify if a sentence is lewd or non-lewd (gut says yes) just need confirmation
Start by collecting a dataset with sentences where you know their label.
Awesome content, thanks for sharing!
Should this be used for, let’s say, classifying weather patterns of historical data (not for prediction; e.g. classified as ‘rain’ based on a labeled training set etc.) due to the sequential nature of such data, or would you think simpler support vector classification methods can still model sequential data to an extent?
I recommend testing a suite of methods in order to discover what works best for your specific problem.
Hi Jason, thanks for the great article! I am not too sure I understand why we need the embedding layer? What if we simple feed the network with the original matrix (padded):
[0 0 0 … 12 33 421]
[0 0 0 … 1 654 211]
Why does the embedding help?
You can learn more about the benefit of embedding layers here:
https://machinelearningmastery.com/what-are-word-embeddings/
Thanks! Actually, it would make no sense to feed the original matrix, where from what I understand, the order of the words matters. If we use another approach, such as CountVectorizer (from sci-kit learn), can we avoid the embedding layer and directly starts with the LSTM layer?
Sure, you can feed sequences of integers (tokenized words) directly to the LSTM.
Hi Jason,
I have learned a lot from the post.
Regarding the LSTM layer, I am having hard time understanding the dimensionality of input vs output. I read a lot about the unit layers and how they work and I understand the math, but on the higher level I am getting confused.
The input for the LSTM is 500 by 32 after embedding. What exactly is the output of each LSTM unit, if we receive an output of a vector in size of n units (100)?
I had the wrong impression earlier that each unit produce a vector of 32 in this case, and then you end up with a matrix of 32 by 100.
Can you ease explain the LSTM dynamics that generates this output?
An LSTM takes a sequence as input and produces a single value as output.
If you have a layer of 100 nodes, each will receive the entire sequence as input and output one value, therefore a vector of length 100.
Does that help?
Hi,
Thanks for the quick reply 🙂
In many places I see that the nodes output a vector (usually called h(t)). This is what I don’t understand.
Yes, LSTMs output a vector with one value for each node at the end of the sequence. The refer to this as h or hidden state.
Hello,
thanks again for your blog. I am guessing why you are using binary crossentropy. Is it not supossed that this dataset is laballed with star reviews from 1 to 10?
Any post of a text classsifier using categorical crossentropy?
Thanks a lot.
Kind regards
Yes, I’m sure I have a few. It only suitable for multi-class classification, for example:
https://machinelearningmastery.com/multi-class-classification-tutorial-keras-deep-learning-library/
Hi Jason! Can you explain why you have not used you Series to Supervised function here? I thought for all sequential problems you need to convert to that format, or is that only for time series, i.e. weather prediction?
This is a text classification problem where the data was already prepared.
I was working on same kind data set where I converted my text data to vectors using Bag of words . Can I use same model??
Perhaps try it and see?
Nice tutorial! Does the embedding preserve the order of the words?
so the sentence “don’t I like bikes” will not be the same as “I don’t like bikes”.
The nature of the embedding can capture the similarity between “bike” and “bikes”, if your training data contains usage of both.
nice post! I’m still a little confused about using metrics=[“accuracy”] though and wondering if you could help. Suppose we have an LSTM with prediction problem being single-label multi-class, several time steps, and each LSTM layer has return_sequences=True. Then the “predictions” are one class for each time step, i.e. each prediction is a list where len(list) = len(time_steps). In this case, what does “accuracy” mean? Is it the binary accuracy of getting *each* time step prediction *entirely* correct? For example, if the true label is [1, 3, 2, 1] and the predicted label is [1, 3, 2, 2] would the error be equal to 1 since the prediction is not exactly equal to the true label?
It would be accuracy for each output timestep which might not be appropriate. You might want to manually evaluate the performance of the predictions.
Hello Jason,
Thank you for this tutorial.
I am trying to use the trained network to predict the sentiment of one imdv review.
so I tried
prediction = model.predict(x_test[0])
I was expecting to get len(prediction) = 1
but I get len(prediction) = 80
80 is the maxlen used to pad the input sequence.
So I am confused.
I would greatly appreciate some insight on this.
Thank you very much Jason
I think the shape of the one sample was not what the model expected. Perhaps reshape it?
Hi
I’m trying to obtain pure CNN model, but seems, lack of expertise beats me. Using your blog I’ve constructed model like this:
top_words = 5000
max_review_length = 500
embedding_vecor_length = 32
model = Sequential()
model.add(Embedding(top_words, embedding_vecor_length, input_length=max_review_length))
model.add(Conv1D(filters=32, kernel_size=3, padding=’same’, activation=’relu’))
model.add(MaxPooling1D(pool_size=2))
model.add(Flatten())
#model.add(Dense(32, activation=’relu’))
model.add(Dense(1, activation=’softmax’))
model.compile(loss=’binary_crossentropy’, optimizer=’adam’, metrics=[‘accuracy’])
model.fit(X_train, y_train, epochs=3, batch_size=64)
But I’m getting 50% accuracy:
25000/25000 [==============================] – 5s 190us/step – loss: 7.9712 – acc: 0.5000
Accuracy: 50.00%
Please direct me, and show my errors.
With respect,
Igor
Perhaps the model requires tuning to the problem?
Thanks. It was very helpful.
Just a question:
As far as I know, the validation set should be differ from the test set.
But in: model.fit(X_train, y_train, validation_data=(X_test, y_test), epochs=3, batch_size=64)
It seems you showed the test set as validation set!
Would you please explain?
Yes, I reused the test set to keep the example simple.
Hi Jason,
I have a dataset which has time(Unix timestamp) and few device level features to predict a specific status of the device, can I use these features directly to make a prediction using LSTM, or is there an alternative way to weigh time?
I would recommend getting familiar with time series forecasting here first:
https://machinelearningmastery.com/start-here/#timeseries
Hi Jason, can you please post a picture of the network ?
I explain how to create a plot of a network here:
https://machinelearningmastery.com/visualize-deep-learning-neural-network-model-keras/
Hi Jason,
I am doing a 3-way classification using a LSTM model. One of the categories appears at the end of the sequence for every sample, while the other two categories appear at the beginning and the middle of the sequence.My LSTM is failing to detect the third category even though it is available consistently towards the end of the sequence.
On the other hand, if my data is such that the three categories are evenly distributed across the timesteps( all three categories are available at the beginning, middle and end of the dataset), the 3-way classification is working fine. This shows that my model is fine.
Do you have some idea, why the classification is shutting off one category, when it appears solely at the end of the dataset?
Regards,
Rick
Just wanted to add, I am using a timedistributed LSTM model
Not off hand, perhaps design some careful experiments with contrived data to help expose what exactly is going on.
hi jason
kindly share a link for forecast with CNN-LSTM
I show how in this post:
https://machinelearningmastery.com/how-to-develop-lstm-models-for-time-series-forecasting/
I also have others, try the search box.
HI Jason ,
What if i want to use LSTM with Conv2d layer, Would it be same or i shall try different approach like adding TimeDistributed layer?
Please let me know
Thanks in advance!!
It depends on the specific of your problem and model, e.g. what are the inputs and outputs.
Jason, thanks so much for this – super clear and helpful, well explained tutorial !
I get an error when trying to run the code (using up to date Pycharm CE on MacBook Pro,
– code is as pasted from your first complete code listing initially, with 29 lines.
Error I get is below – any ideas to get this running appreciated!
Using TensorFlow backend.
Traceback (most recent call last):
File “/Users/charlie.roberts/PycharmProjects/test_new_mac_dec_18/venv/LSTM brownlee_cr expts.py”, line 28, in
model.add(LSTM(100))
File “/Users/charlie.roberts/PycharmProjects/test_new_mac_dec_18/venv/lib/python2.7/site-packages/keras/engine/sequential.py”, line 181, in add
output_tensor = layer(self.outputs[0])
File “/Users/charlie.roberts/PycharmProjects/test_new_mac_dec_18/venv/lib/python2.7/site-packages/keras/layers/recurrent.py”, line 532, in __call__
return super(RNN, self).__call__(inputs, **kwargs)
File “/Users/charlie.roberts/PycharmProjects/test_new_mac_dec_18/venv/lib/python2.7/site-packages/keras/engine/base_layer.py”, line 457, in __call__
output = self.call(inputs, **kwargs)
File “/Users/charlie.roberts/PycharmProjects/test_new_mac_dec_18/venv/lib/python2.7/site-packages/keras/layers/recurrent.py”, line 2194, in call
initial_state=initial_state)
File “/Users/charlie.roberts/PycharmProjects/test_new_mac_dec_18/venv/lib/python2.7/site-packages/keras/layers/recurrent.py”, line 649, in call
input_length=timesteps)
File “/Users/charlie.roberts/PycharmProjects/test_new_mac_dec_18/venv/lib/python2.7/site-packages/keras/backend/tensorflow_backend.py”, line 3011, in rnn
maximum_iterations=input_length)
TypeError: while_loop() got an unexpected keyword argument ‘maximum_iterations’
Sorry to hear that, I have some suggestions here:
https://machinelearningmastery.com/faq/single-faq/why-does-the-code-in-the-tutorial-not-work-for-me
Jason – huge apologies just re-searched and found TF needed updating again which seems to have fixed. Lesson learned, will double check that first in future (and try to post more interesting questions than bug fixes!). Charlie
Nice work Charlie!
This is really first rate. Thorough, lucid, a nice long walk up to a summit with a great view.
Thanks.
Hi Jason,
Thanks for your sharing. Have a few questions below:
1. When we use LSTM with a post padded sequence in Keras, and when set return_sequences = False, will the LSTM be able to return the activation for the timestep that is from last one before padding starts? or LSTM will always return the activation from the last timestep including padding?
2. If it is returning the activation from last time step including padding, how do we go around about it? We need to track the sequence length, and set return_sequences = True for the LSTM and pick the correct activation based on input sequence length? is there any easier way?
3. Generally for sentiment analysis, do you think that will make a big difference?
Many thanks for your time!
Good questions.
LSTMs always return the accumulated activation (called hidden state or h) from the final tie step, but the padded inputs are ignored if you use a masking layer.
The final activation has all info about the entire sequence – it is a summary. If you need activations from each input time step to make a decision, then return_sequences=True and interpret it with another LSTM or some other model.
It is problem dependent. Generally CNNs seem to work better for sentiment analysis anyway:
https://machinelearningmastery.com/best-practices-document-classification-deep-learning/
When doing LSTM analysis, do you play with forget and remember gates? For example, in above LSTM model used for IMDB data, how did you integrate those in your analysis? I assume Keras default values are used but how can we change that if we wanted? Thanks
Not really. You can try and see if it makes a difference.
Hi Jason thank you a lot for this tutorial.
I cannot see where the seed initialized with numpy.random.seed(7) is used after ? For Dropout layer ?
For the first LSTM model, why do I get different training accuracies while using the same seed (7) ?
Getting reproducible results in Keras is challenging, more here:
https://machinelearningmastery.com/reproducible-results-neural-networks-keras/
Nice tutorial, you did a brilliant job. Can you make a tutorial on speech recognition using spectrogram or mfcc and neural network?
Thanks for the suggestion.
hi jason,
thank you for your post, it’s very helpful. I have a dataset is such of paragraph, with each paragraph is combine of multi sentence. And I want each sentence go through lstm layer, and output of all sentence in paragraph combine and go through final lstm layer.
like image in this link :
https://drive.google.com/open?id=1E9naIUKybZjlpraidKe_3J5AXJ42ZET_
can you let me know how we can build this architecture in keras.
thanks you.
I don’t understand the architecture in the picture, sorry.
My best advice on getting started with LSTMs is here:
https://machinelearningmastery.com/start-here/#lstm
Hello,
Firstly, thank you so much for this post.
I want to use a dataset containing sequences of words, how can I change this part of code:
#top_words = 5000
#(X_train, y_train), (X_test, y_test) = imdb.load_data(num_words=top_words)
???
Thank you soo much.
I recommend starting here when working with text:
https://machinelearningmastery.com/start-here/#nlp
Hello,
Thanks for this post.
I tried to do the LSTM sequential for numerical classification problem.
My data set includes 36 features of float values(X) and classification attribute(Y) (lables: 0,1,2,3)
And my data set includes 7537 records of csv file.
Can you please tell how to reshape the dataset array for LSTM model, since LSTM expects input_shape in 3-d format.
Great question, this will help you reshape your data:
https://machinelearningmastery.com/faq/single-faq/what-is-the-difference-between-samples-timesteps-and-features-for-lstm-input
Hello
,
I’m asking if I want to do sentiment analysis for brand data following the same approach in this tutorial. Brand data is the data that submitted by customer on social media like twitter or News expressing their opinion toward that brand. Is there a ground truth related to brand data so I can definitely train the model based on that ground truth or training data?!
Thanks you
No, or I doubt it. You must establish a metric that helps you to define what a good result looks like.
This is not unique, most problems have this issue, and you can approach it by comparing your metric to the results of a naive method – e.g. performance/skill is relative.
Can I do future prediction using LSTM and keras?
Yes, you can get started here:
https://machinelearningmastery.com/start-here/#deep_learning_time_series
Hi Jason,
Thank you for the tutorial. Instead of using top n words. Can we choose the words in our model according to, say Word Count x IDF ?
Sure.
Mr Jason
The code gives the following error
Object arrays cannot be loaded when allow_pickle=False
Could you please help in rectifying it
Thanks
Sorry to hear that, I have some suggestions here:
https://machinelearningmastery.com/faq/single-faq/why-does-the-code-in-the-tutorial-not-work-for-me
I encountered the exact same error but the solution here seemed to fix it:
https://stackoverflow.com/questions/55890813/how-to-fix-object-arrays-cannot-be-loaded-when-allow-pickle-false-for-imdb-loa
Essentially, downgrade numpy to 1.16.2
That’s a shame.
I have another fix, add these lines to the start of the code example:
Based on:
https://stackoverflow.com/questions/55890813/how-to-fix-object-arrays-cannot-be-loaded-when-allow-pickle-false-for-imdb-loa
Hi, I’m doing a classification study for Turkish texts using cnn and lstm. Is there any Turkish word dictionary to vectorize Turkish texts ? Or, why does python do this vector operation?
You must vectorize the texts, I give examples.
Perhaps start here:
https://machinelearningmastery.com/start-here/#nlp
This tutorial specifically will be helpful:
https://machinelearningmastery.com/develop-word-embedding-model-predicting-movie-review-sentiment/
Hi Jason,
I am getting the following error while implementing LSTM:
ValueError: Input 0 is incompatible with layer lstm_1: expected ndim=3, found ndim=2
Here’s the code I have used:
model.add(LSTM(100, input_shape=(32652,21767,16),return_sequences=False) )
model.add(Dense(22, activation=’relu”))
model.compile(loss=’binary_crossentropy’, optimizer=’adam’, metrics=[‘accuracy’])
model.fit(X[train], Y[train], epochs=100, batch_size=10)
I have seen by changing the parameters of input_shape and omitting return_sequences also. But the program is not running.
Please help me to solve the issue:
Hi Jason,
as always I’ve got to tell you how much I appreciate your website. I’ve already learnt tons of stuff about neural network coding and it somehow appears that you’ve covered each kind of topic. Probably also my request, though I wasn’t able to find it.
Following issue: I’ve got Time Series data with mixed categorical and numerical entries that shall be analyzed via a LSTM. Imagine it like this, but huge (too huge for one-hot-encoding):
2000|20|West|0.2|Yes
2001|21|East|0.4|Yes
2002|22|East|0.9|Maybe
2003|23|North|1.0|No
2000|11|South|0.9|No
2001|12|West|1.1|Maybe
2002|13|West|3.1|Maybe
2003|14|South|0.8|Yes
First line is the time stamp (i.e. year). For a mere LSTM a 3D-reshape would suffice (in this case 2,4,5) to feed it in.
The categorical data require some preprocessing, which can be achieved via LabelEncoder and Embedding layer.
However, I’m quite unsure, how to fuse correctly the Embedding layer and the LSTM when I’ve got several categories of different internal extent.
Is there any easy and brief example you might give based on your experiences with Embeddings?
Lots of Thanks in advance,
Tobias
Thanks Tobias!
You can try integer encode, one hot encode and embedding for each categorical variable and see what works best.
The embedding layer is concatenated with the other inputs for each time step, probably via a multi-input model.
I can see how this would be easy for tabular data without time steps, it is a straight multi-input model. For time steps of categorical, you may need Embedding-LSTM for each categorical var and then merge each model input.
Some experimentation may be required, this may help as a first step:
https://machinelearningmastery.com/keras-functional-api-deep-learning/
Thanks for your reply, Jason!
However, this somehow not helping me out with my dimensional struggles between Embeddings that have to be concatenated and the LSTM.
For a mere LSTM (I already used some) a 3D-reshape would suffice (in this case 2,4,5) to feed it into a model like this:
def createLSTMModel():
model = Sequential()
model.add(Bidirectional(LSTM(250, return_sequences=True),input_shape=(Train_Num,1)))
model.add(Dropout(.65))
model.add(Bidirectional(LSTM(25, return_sequences=True)))
model.add(Dropout(.65))
#model.add(Flatten())
model.add(Dense(1))
return model
However, with Embeddings of different embedding sizes together with a different number of continiuous and categorical data, I’m not sure how to do this, especially when I wanna stick with the Sequential model structure. Is there a possibility how to this neatly with an embedding layer like this
model.add(Embedding(input_dim=num_words,
input_length = training_length,
output_dim=dim_length,
#weights=[embedding_matrix],
trainable=True,#mask_zero=True)))
Sorry if this might seem simplistic to you, but sometimes it’s a little mental border that has to be overcome and neat little examples often do the trick. Is it possible to stick with the Sequential layer cake strucutre in this case?
Start with an integer encoding as a baseline, very easy
Then try a one hot encoding, this will dramatically increase the number of features, but still very easy.
Then try one embedding per categorical var, each on a separate head. It will require the functional API. Start with just one categorical var, e.g. 2 heads, and use a concatenate merge layer. Get that working, then scale up to the rest.
Does that help?
Hello jason Brownlee,
I have a doubt in unknown symbols at test time.
unknown symbols at test time. example: unique names in sentences. For example a sentence in training data might be ‘Thank you John’ and in test data be ‘Thank you Mary’.
please share your views.
Unknown symbols can be mapped to 0.
Hi Jason,
I am learning Machine learning under your guidance through your website and materials.I am working on multi class/multi label classifier using RNN(LSTM) .It is emotion classification of tweets which are categorically divided into anger, disgust, fear, guilt, joy, sadness, shame . I was your example here for binary classification , 0 for negative and – for positive, I am unable to put things together for my assignment,Please guide me for solving this problem of multi class/multi label classification?.I have followed similar way what you have done here, I have used label encoding for seven labels and they are converted into numbers 0 to 6 , The model accuracy is decreasing and loss is increasing while training the model.Please advise me. Thanks in advance and i always appreciate your helping nature and encouraging the people to learn things.
I have some suggestions here that might help as a first step:
https://machinelearningmastery.com/improve-deep-learning-performance/
Thank you very much, I was doing the things in wrong way. I got the things right to some extent.I need to work on improving the accuracy of the model.
I will appreciate your help.
Here are many suggestions:
https://machinelearningmastery.com/start-here/#better
Hi Jason,
I want to train network to predict TV channel by given time, the data is in date and time with tv channel columns. can you give me suggestion how to train it. Thanks in advance
Start with a strong definition of the problem, use this framework:
https://machinelearningmastery.com/how-to-define-your-machine-learning-problem/
Hi Jason, For LSTM 100 units, where exactly do these 100 units reside in a LSTM network? (as per the Colab’s famous description with the various input/forget gates)
What do you mean exactly? They are numbers in a program.
I mean visually speaking as LSTM is not a classical neural network. The way I understand a traditional LSTM is it is 4 gates that interact with one another but as per Keras and your description above LSTM(100) is a 100 neurons. I just wasn’t sure how these 100 neurons into the LSTM network dealing with the 4 gates
Each of the 100 units has 4 gates. Each unit receives all input and creates an output.
Does that help?
Hi Jason,
Thanks for these examples. By the way, in statement “The problem is to determine whether a given movie review has a positive or negative sentiment.”, where is the part of the code that addresses this?
Is that what you mean to the answer in your Problem statement?
I mean what are the context of inputs and outputs?
y=model.predict(x)
where x = is a string sequence of a movie review, Y = whether positive or negative?
Thanks
Yes, the model learns the relationship with text input to sentiment class output.
The key part is the model and what it learns.
Hello,
Very useful blog and examples! I spend some time each morning learning some more on ML with your materials!
I have a question on the choice of data set for this LSTM classification post.
If I understand correctly, LSTM NN are somehow dedicated to time series. In this example we use movie reviews, which are rather not of this kind. So, for this classification a simpler, classic Multi-Layer perceptron could be sufficient, right? Or there is other specific reason for this choice?
Best regards!
No, generally LSTMs are suited for sequence prediction, not specifically tie series.
In fact, LSTMs work better with text data than with time series data.
Hi Jason. Thanks a lot for the great great article. Honestly, I have become a fan of your articles now 🙂
One quick question. I have a dataset as follows and would like to apply the techniques you have mentioned above.
The details of my dataset is as follows:
I have about 1000 nodes dataset where each node has 4 time-series. Each time series is exactly 6 length long.The label is 0 or 1 (i.e. binary classification).
More precisely my dataset looks as follows.
node, time-series1, time_series2, time_series_3, time_series4, Label
n1, [1.2, 2.5, 3.7, 4.2, 5.6, 8.8], [6.2, 5.5, 4.7, 3.2, 2.6, 1.8], …, 1
n2, [5.2, 4.5, 3.7, 2.2, 1.6, 0.8], [8.2, 7.5, 6.7, 5.2, 4.6, 1.8], …, 0
and so on.
However, since the setting of my data is bit different, I am not sure how I can transform it in a way that is suitable to apply these techniques.
My question are;
1. Do you think that 1000 nodes is sufficient for deep learning (e.g., about 800 for training and 200 for testing)? If it is not sufficient I would like to look for options to increase my dataset.
2. Since I have 4 seperate short sequences (time-series) for each node, how can I use it for classification?
Please kindly let me know your thoughts.
Looking forward to hearing from you. Thank you 🙂
It really depends. Perhaps test and discover how sensitive your mode/problem is to the amount of data?
You can use time series classification with MLP, CNN and LSTM, I give an examples here:
https://machinelearningmastery.com/start-here/#deep_learning_time_series
Hi Jason, Thanks a lot. Sure I will have a look 🙂
BTW I also came across a model named as autoencoder. Can we use autoencoders as well? What is the difference of autoencoders with the reamaining models like CNN, RNN and LSTM? 🙂
An autoencoder is an architecture and can be constructed with different network types. It can be used as a feature extraction model, e.g. a front end to another classifier model.
Hi Jason,
Thanks for your terrific example. I got the gist of it, but when I try running it I get a whole bunch of output, I suspect connected with data loading, and then the error message below. The problem seems to start at line 12 of the program. Is there a way I can put my own data there instead of loading IMDB.
Thank you,
akhil
all last):
File “M:/Akhil/Research/Neural-Networks/LSTM-TF3.py”, line 12, in
(X_train, y_train), (X_test, y_test) = imdb.load_data(num_words=top_words)
File “C:\Users\axk41\AppData\Local\Programs\Python\Python36\lib\site-packages\keras\datasets\imdb.py”, line 57, in load_data
file_hash=’599dadb1135973df5b59232a0e9a887c’)
File “C:\Users\axk41\AppData\Local\Programs\Python\Python36\lib\site-packages\keras\utils\data_utils.py”, line 222, in get_file
urlretrieve(origin, fpath, dl_progress)
File “C:\Users\axk41\AppData\Local\Programs\Python\Python36\lib\urllib\request.py”, line 277, in urlretrieve
block = fp.read(bs)
File “C:\Users\axk41\AppData\Local\Programs\Python\Python36\lib\http\client.py”, line 449, in read
n = self.readinto(b)
File “C:\Users\axk41\AppData\Local\Programs\Python\Python36\lib\http\client.py”, line 493, in readinto
n = self.fp.readinto(b)
File “C:\Users\axk41\AppData\Local\Programs\Python\Python36\lib\socket.py”, line 586, in readinto
return self._sock.recv_into(b)
File “C:\Users\axk41\AppData\Local\Programs\Python\Python36\lib\ssl.py”, line 1009, in recv_into
return self.read(nbytes, buffer)
File “C:\Users\axk41\AppData\Local\Programs\Python\Python36\lib\ssl.py”, line 871, in read
return self._sslobj.read(len, buffer)
File “C:\Users\axk41\AppData\Local\Programs\Python\Python36\lib\ssl.py”, line 631, in read
v = self._sslobj.read(len, buffer)
ConnectionResetError: [WinError 10054] An existing connection was forcibly closed by the remote host
>>>
Sorry to hear that, it looks like you have an error reading a remote file.
Hi Jason,
I used a pre-trained doc2vec model to get embedding for the input sequence. Then removed the Embedding layer.
My x_train shape is (num_training_data, 300) where 300 is doc2vec embedding size.
I faced an error that the LSTM layer was expecting dim 3, but received 2.
I corrected the error by adding number of time-steps as 1 by re-shaping x_train.
My question is: Is this approach acceptable? Or am I doing something wrong?
Code snippet:
# Convert string labels to integers
le = preprocessing.LabelEncoder()
le.fit(y_train)
y_train = le.transform(y_train)
# Convert x_train to 3 dimensions – middle dimension is number of time steps
x_train = np.reshape(x_train, (x_train.shape[0], x_train.shape[1], 1))
# Define the model
self.model = Sequential()
self.model.add(LSTM(100))
self.model.add(Dense(100, input_dim=300))
self.model.add(Dense(1, activation=’sigmoid’))
self.model.compile(loss=’binary_crossentropy’, optimizer=’adam’, metrics=[‘accuracy’])
# Train the model
self.model.fit(self._x_train, self._y_train, epochs=10000)
It is acceptable if the model gives better results than all other models you can think of/test.
Generally, it suggests that it is not getting the most out of the LSTM, and perhaps an MLP would be more suitable.
Thanks for this guide!
How can I use it to train multivariate to multiclass sequence?
I have 50000 sequences, each in the length of 100 timepoints.
At every time point, I have 3 features (So the width is 3).
I have 4 classes and I want to bulid a classifier to determine class for sequence.
What is the best way to do so?
Good question, you can get started right here:
https://machinelearningmastery.com/start-here/#deep_learning_time_series
Dear Jason, thank you for your post.
I have questions about fundamentals in LSTM (also in a way that Keras explains).
Suppose that I have 100 samples with each sample contains 7 rows and 17 columns. My code looks like this:
model.add(CuDNNLSTM(
input_shape=(7, 17),
units=100,
return_sequences=False))
and then followed by several fully connected layers.
My model works fine and I understand how to use it. Here are my questions:
1. In my case, how many LSTM cells do I have? and how many LSTM units do I have?
2. What is actually the difference between LSTM cell and LSTM unit? I tend to think LSTM unit equivalent to neurons in fully connected layer, but I am afraid that this is a faulty assumption.
3. In my understanding, LSTM works by processing each samples (call it X_t) with previous (or initial) hidden state through its gates and simple math with previous cell state, and then will output new hidden state and new cell state. Does X_t refer to first row of the sample (it would be only one row and 17 columns), or first sample from all of the samples (7 rows and 17 columns)?
Thank you very much for your attention and assistance, and sorry for this long questions. I want to understand my code so that I would not treat them as a black box.
You have 100 lstm units.
There are only lstm units. One unit is one cell.
Each time step is one example, the sequence is provided to each lstm unit.
Thank you for your reply.
So, does it works like this?
1. First lstm cell will get my first sample input (7 time steps)
2. After processing all of the time steps, the hidden state will be passed to the second lstm cell along with the first sample. (So hidden_state_1 and first sample -> second cell).
3. We will repeat all of these steps until all lstm cells processed the first sample.
4. After all cells processed the first sample (and reach the last layer), we pass the second sample to the first cell, repeating all the steps.
Am I correct, or did I miss something important?
Let me rephrase a bit.
– We have 100 LSTM cells, which will have my first sample as their input.
– After the first lstm cell processes the first sample, it will then pass the hidden state to the second lstm cell.
-The second cell will pass the hidden state after processing both hidden state from the first cell and the first sample to the third cell.
– Eventually, all the cells will process the same input sequentially, waiting for the hidden state from previous lstm cell, and the last cell will pass the value to the next layer.
Thank you
Sorry again, to clarify a bit about my last comment,
the first row in my first sample is the X_t-6 and the last row is X_t.
Thank you Jason.
No, each unit gets the sequence one time step at a time and does not interact with other units in the same layer.
Think of a layer as a collection of many separate “networks”.
Only layers interact, e.g. stacked LSTMs.
Sorry Jason, but I am still haven’t understood this part:
“… each unit gets the sequence one time step at a time and does not interact with other units in the same layer.”
In my case, does this mean:
– I have 100 LSTM units.
– For the first time, each unit will take my first sample as their input (7 rows, 17 columns).
– My first LSTM unit will process the first row of the sample, and then pass the hidden state to be processed along with the second row of the sample (still in the same unit).
– Each LSTM unit will process the same sample, but a unit does not interact with another unit in the same layer.
The illustration will be somewhat like this:
( [ X_t ] is an LSTM unit processing input X in timestep t )
we will have one hundred of this in one LSTM layer:
[ X_t-6 ] -> [ X_t-5 ] -> [ X_t-4 ] -> [ X_t-3 ] -> [ X_t-2 ] -> [ X_t-1 ] -> [ X_t ]
Please correct me if I am wrong.
Thank you very much.
Almost.
Consider just one unit. The unit gets a “sample”, but processes it one time step of input at a time with internal state updated for each time step. At the end of the sample, a new sample is started (second row) and the state is already primed from the end of the last sample, and the process repeats.
Does help?
Thank you for helping me Jason. Maybe I need to visualize things first.
In a hidden layer (dense layer), 100 units means there are 100 neurons inside a layer.
Does saying “100 LSTM unit in one LSTM layer” equivalent to saying 100 neuron in one dense layer?
Thank you
Yes.
Sorry again, did not mean to spam, I missed one information.
The [ X_t-6 ] -> [ X_t-5 ] means that my first row will be passed to the LSTM cell, and the hidden state from X_t-6 will be passed to be processed with the second row in [X_t-5 ]
Thank you very much Jason.
Hey Jason
Nice tutorial
1. can we use LSTM for multiclass classification?
2. I have a time series data and i want LSTM to classify it into multiple classes ? is it possible
Yes, but only if the inputs are sequences.
Yes. I give an example here:
https://machinelearningmastery.com/how-to-develop-rnn-models-for-human-activity-recognition-time-series-classification/
Hi Jason,
your job is awesome, I learnt a lot from your tutorials, thank you very much.
I have 2 doubts regarding scaling data for lstm models:
1. If a new min/max value is found when forecasting and the model uses on-line learning (it uses test data to get updated), how do I handle that new value? does the model have to update the hole scaled data?
2. When working with financial data, close past values have an increasing effect on actual value. Then suppose that a financial univariate time series distribution has some special values that affect the sequence behavior when the independent variable gets close to those values. For example, the raw interval real range is within [1,10] and, let’s say, the value of the dependent variable increases/decreases its variance or bounces more than usual when it approaches the value of 6.
a. does the model also learn the effects of those hot values?
b. I would like to make some kind of k-fold validation but without using future values to predict past values. I would like to split the distribution in n sets of equal length and with train-test parts
within (i.e. with 80%-20% train-test ratio for each set). Then the model is fit starting from set 0 and move forward up to set n. In that case, I must first scale the hole dataset at the beginning, right?
Thank you in advance
Seb
Great questions.
Scale data with the expectation of future values (e.g. using domain expertise or educated guesses), or clip values to the past min/max limits.
If it useful to learn such dependence, then it would be nice if your model did so. You can test this with scenarios.
You can use walk-forward validation:
https://machinelearningmastery.com/backtest-machine-learning-models-time-series-forecasting/
Please may tutorial on “RAY” python library. How to use and implement it in deep learning ?
What is the RAY library?
Hi Jason, I have a question about outliers.
I am trying to do sequence classification using LSTM (one layer LSTM followed with some Dense layers).
I noticed that 15 out of 17 features I used in my training set contain more than 10% outliers (measured by IQR).
I figured that I can use scikit-learn’s RobustScaler to scale because I don’t want to remove the outlier (most likely, those outliers are reasonable–not a measurement failure)
However, I seem not able to find any literature that talks about LSTM and outliers.
My questions are:
1. Does deep neural network (with LSTM) affected by outliers?
2. Is there any recommendation to handle this problem? I’m afraid that these outliers are the main reason I can’t achieve good accuracy, even on training set
3. Should I use another scaler? If yes, do I need to cap the value of the outliers? For example, outliers will be automatically have value “1” when scaled using StandardScaler with cap for values with z-score more than 3.
You have been helping me through my hard thesis since August 2019, I’m grateful that you created this blog. Thank you Jason.
Really nice work!
Yes, I would expect it is effected. Use controlled tests to conform the impact on model skill though, don’t guess.
Yes, try a suite of methods, e.g. smoothing, removing, imputing, do nothing, various scaling, review effects on skill using controlled experiments.
Yes, try them all, standardization, normalization, power transform, etc.
Also, do all of the above with an MLP and a CNN to compare. Sometimes LSTMs are a real pain and other models work better.
I hope that helps, I’m eager to hear how you go – what you discover about your data/model.
Ah I see, thanks! I guess I have to create small baseline model first.
If you don’t mind, I still want to ask about sequence classification (but not LSTM), is it possible to do sequence classification using Logistic Regression, Random Forest, and SVM? I found that they need 2D-array. So far, I reshaped the sequence from 3D numpy array to 2D numpy array in order to handle this problem, but I wonder if this is the correct step..
Sure, using the window method.
This will help:
https://machinelearningmastery.com/convert-time-series-supervised-learning-problem-python/
Hi Jason,
great tutorial, thanks a lot! How would you extract most predictive words (feature importances) from the LSTM network used for sentiment analysis? Thanks!
Best,
Nikolay
Hmmm, good question.
Off the cuff, you could design input sequences and perform a sensitivity analysis on a model.
But I think there are better approaches designed specifically for LSTMs. Perhaps look up some of the LSTM visualization methods.
what an amazing code it is!
Thanks!
Hi Jason,
Thank you for the article it was very helpful!
In my case, In my dataset the data is repeating at random intervals as in, the previous data is repeating as the future data and I want to classify the original data and the repeated data. Is it possible to do with LSTM.
Perhaps develop a prototype to test the model?
Hi
Why the final accuracy is higher than the train accuracy in some cases? I have this issue in the other sequence classification data set?
A standalone evaluation is more accurate than the accuracy seen during training, as the accuracy during training is averaged over batches.
Hi Jason, I have a question about the sensitivity of LSTM models in general.
I am currently developing a sequence classification LSTM model. In total I have 5 of sequences to classify, using softmax as the activation function for the last layer.
The model is able to accurately classify each sequence, meaning that for e.g. I have a sequence of [1,1,1,1,1,1], which the element 1 is used to denote that the element belongs to the class “1”, for the model to predict. The model can accurately classify the sequence into class 1 with value of 0.9. Likewise, for a sequence [2,2,2,2,2,2] the model is able to classify it into class ‘2’ with value of 0.9.
However, when I feed into a sequence that is “mixed”, e.g. [1,1,1,1,1,1, 2, 2, 2], the model still predicts class “1” with a value of 0.9, without a drop in value despite the inclusion of elements from class “2”. Is it natural for LSTM to not be sensitive to such “mixed” sequences? If not, are there any solutions to make the LSTM react and drop their value when they see such sequences?
I would love to hear your insights on this, thanks!
Perhaps you want to frame your problem as multi-label classification rather than multi-class classification. E.g. use sigmoids in the output layer and binary cross entropy loss.
Hi Jason, thanks for your reply.
However due to my research topic, I am to use a multi-class classification model in combination with an algorithm to split the sequences into their respective classes and a key part of the algorithm requires the LSTM to see a drop in value if sequence contains elements from two classes.
e.g [1,1,1,1,1,1] will be predicted as class ‘1″ with value of 0.9 while [1,1,1,1,1,1, 2, 2, 2] will be classified as class ‘1’ but with a value of 0.8. So the drop in value will signify that some elements in the sequence does not belong to class ‘1’.
My model architecture in keras goes something like this:
LSTM -> fully connected layer of 5 neurons with activation of softmax.
Loss : categorical_crossenthropy
As for your suggestion of sigmoids in the output layer, can I know what is the rationale behind it? Thanks!
Yes, if we were modeling the problem as multi-label classification, we use sigmoids in the activation layer.
Hi Jason, thanks for the reply.
Do you happen to know any tools that I can debug my model with? For e.g at each time step in the LSTM during prediction, I want to be able to see the values produced by the input gate and output gate etc.
You can access the weights of the model via keras.
If you want more detail, you might need to implement it yourself from scratch.
Hello Jason,
I just finished my undergraduate thesis defense about predictive maintenance using LSTM and I just want to thank you for always replying to my questions!
Thank you very much Jason.
Well done!
Hey Jason,
I want to classify many samples and judge whether they will have problems. The classification results are represented by 0,1. But there is a problem. Each sample of mine has the same characteristic number (20), but their time length is different, and the sample length I want to predict is also different. How can I solve this problem, or do you have any good articles recommended to me?
My simple sample is as follows:
1Sample number 2time 3temperature 4speed 5problem
1 2019-0101 25 1.8 0
1 2019-0102 23 1.7 0
1 2019-0103 26 1.5 0
1 2019-0104 28 1.9 0
2 2019-0105 26 1.7 1
2 2019-0106 28 2.0 1
3 2019-0107 30 2.4 0
3 2019-0108 29 2.1 0
3 2019-0109 26 1.8 0
This will give you ideas:
https://machinelearningmastery.com/data-preparation-variable-length-input-sequences-sequence-prediction/
Hi Jason,
A lot of thanks for your tutorial. I’m a newei with neural networks
I have been trying to aplayd the template to my classification problem, but it gives me very poor results (less than 50% of Accuracy).
I have a dataset composed of 10000 rows and 254 columns, each row is a generated sequense of 253 decimal numbers and the las columns is of labels (0s and 1s). There is my code:
path = “C:/Users/i_dra/Documents/Challenge Data/TrainMyriad.csv”
df = pd.read_csv(path)
y=df[“Class”]
X=df.drop(“Class”, axis = 1, inplace = False)
X_train, X_test, y_train, y_test = train_test_split(X, y, test_size=0.2, random_state=42)
X_train = X_train.values
y_train = y_train.values
X_test = X_test.values
y_test = y_test.values
model = Sequential()
model.add(Embedding(8000, 32, input_length=253))
model.add(Conv1D(filters=32, kernel_size=3, padding=’same’, activation=’relu’))
model.add(MaxPooling1D(pool_size=2))
model.add(LSTM(160))
model.add(Dense(1, activation=’sigmoid’))
model.compile(loss=’binary_crossentropy’, optimizer=’adam’, metrics=[‘accuracy’])
print(model.summary())
model.fit(X_train, y_train, epochs=3, batch_size=64)
It is correct to used the embedding layer with my decimal secuences? There is any other approach?
Yes, I recommend diagnosing issues with the model and experimenting with new configurations.
The suggestions here will help:
https://machinelearningmastery.com/start-here/#better
Hi Jason, an excellent blog for me to start my hands on RNN.
May I know what does it means for a 32 length real-value vector and how do you know the output dim of embedding is 32?
I am also thinking why do you know to use 100 smart neurons for LSTM?
I saw on the Keras website, they have an example of bidirectional RNN. In my course work, it was mentioned that bidirectional RNN may work well in understanding long term words relationship.
I think LSTM has also this effect since it helps to propagate down the earlier cell state to later states, so they are pretty similar. In this case, how would you suggest to use which?
The embedding size is specified as a parameter.
The number of nodes in a layer is arbitrary and found via trial and error:
https://machinelearningmastery.com/faq/single-faq/how-many-layers-and-nodes-do-i-need-in-my-neural-network
I recommend testing a suite of different models in order to discover what works well/best for a given dataset.
Hi Jason, I am new to DNN and recently trying to learn RNN. I must admit it is not easy to piece different info from the internet and make sense out of it. Your article helps alot.
Can i clarify the following so that i can better understand how it works.
1. Base on my understanding of RNN, it handles different length of input, in this case the reviews.
So why do you need to pad them to make it standard length?
2. Only 1 LSTM with 100 neurons included in model. Can i understand better, with 500 length, the RNN will unfold 500 LSTM to handle the 500 inputs per review right?
3. model.compile(loss=’binary_crossentropy’, optimizer=’adam’, metrics=[‘accuracy’])
binary_crossentropy is used as we are dealing with two classes right? What if we have more than 2 classes, what should be used? I was googling and seems like i can use categorical_crossentropy but i need to convert the 3 classes into a matrix of 1 and 0 with to_categorical from keras.
i.e. from tensorflow.keras.utils import to_categorical.
I am still finding out how is this differ from the standard crossentropy loss function.
Thanks in advanced!
Keras expects inputs to have a fixed length, therefore we pad.
Input length is unrelated to the number of nodes in the layer.
More than 2 classes you must use categorical_crossentropy and softmax activation function, one hot encoded inputs.
Hi Jason,
Firstly, thanks a lot for all the blogs that you have written!
Coming to my question,
I have a scenario where my dataset has sentences and some tabular features along with them. I want to use the tabular features along with the sentence itself for my classification task. Is there a way to feed the tabular features into the LSTM model?
Thanks!
Thanks!
You can use a multi-input model, with one input for text and one for static features.
This will help:
https://machinelearningmastery.com/keras-functional-api-deep-learning/
Thanks Jason! That helped 🙂
You’re welcome, I”m happy to hear that.
I am trying to use this LSTM for classification but I am getting an error as follows:
The added layer must be an instance of class Layer. Found:
Please what do I do?
Perhaps this will help:
https://machinelearningmastery.com/faq/single-faq/why-does-the-code-in-the-tutorial-not-work-for-me
I have noticed an unpleasant dependence on the “input_length” argument. If I set it to 5’000 in your example, the results are much worse. I would have hoped that the LSTM memory automatically realizes that looking at the past 5’000 words is ineffective. Why do you think this happens? And what would you do if you have longer texts to classify?
Thanks for your great tutorials!
LSTMs are typically limited to 200-400 time steps before they degrade.
Interesting, seems like most tutorials don’t discuss this. How would you go about classifying longer sequences? Break them up into different pieces and combine the prediction? Just cutting off the text seems like a waste of data, no?
My bad, just found this: https://machinelearningmastery.com/handle-long-sequences-long-short-term-memory-recurrent-neural-networks/
Thanks 🙂
Great.
Perhaps compare a few strategies and evaluate their results.
There are newer techniques that specifically address the need to output long sequences.
Hi Jason,
I have a small question normally when you train your model you are to see in the console the epoch, as well as the loss, accuracy and the time that is taking per epoch. I find strange that my model is not printing or showing each epoch.
#Building the LSTM
model = Sequential()
model.add(LSTM(100,activation=’sigmoid’, input_shape = (n_steps, n_features) ))
model.add(Dense(3, activation=’softmax’))
model.compile(loss=’categorical_crossentropy’, optimizer=’adam’, metrics=[‘accuracy’])
#history = model.fit(X, to_categorical(y), validation_split=0.2,epochs=2, verbose=0, batch_size = n_steps )
model.fit(X, to_categorical(y), validation_split=0.2,epochs=2, verbose=0, batch_size = n_steps )
scores = model.evaluate(X, to_categorical(y))
What do you think is the issue? Even though I have small amount epochs I should be able to visualize them right?
Change the value of “verbose” to 1 or 2.
Hey Jason,
thank you very much for the insightful articles. They are helping me a lot with my data analysis and I cited you in a scientific paper I wrote (that is under revision and will hopefully get published soon).
I have a few questions regarding my data and the LSTM:
I collected mouse usage data during a task and now want to test wether I can predict the condition (dichtomous classifiaction) or a self-reported value (regression) from the mouse data. I already tried some approaches (e.g. calculating features such as the mouse speed) and used that for classification/regression.
Thanks again and sorry for the noob questions!
The latest idea was to use the raw x-and y-coordinate values as the model input. To me, they represent a time-series of mouse movements and therefore, a sequence classification or regression using a LSTM might be a suitable approach. Would you agree or do you think that the data is not suited for this approach?
In my code so far, i followed this tutorial for the classification task, but wondered how I should change the LSTM model in the regression case. Do you have a suggestion? My humble approach would be to change the loss function to mean_squared_error and use the R² as the evalution metric.
Well done!
Perhaps try it and compare to other approaches.
Yes, my advice is to explore as many different framings of the problem and models you can think of in order to discover what works/works well for your specific dataset. Use results to drive decisions, not guesses.
Thank you very much for the answer. I agree, that trying out different things is the way to go! The problem in my case is that no model (regardless of the approach) is able to find meaningful patterns in the data and I guess it is because there are none in the specific use case (there is no relationship between the emotional state and mouse usage). The goal therefore is to report null findings, but also show different methods to analyze the data in other usage cases that might work better. That’s why I wanted to know if the time-series classification/regression approach makes sense or should rather not be suggested as an analysis approach.
Perhaps the target is not predictable from available data. Change the problem or get new/different data.
Hi ,
I want to know how to deal with signals in load them and decompose them to classify in 3×3 matrix
Sorry, I don’t understand your question, perhaps you can elaborate?
Dear Jason, thanks for the great tutorial.
I am going to run LSTM on imdb database to classify movies into attacked with malicious users or not. but I am going to use numerical rates of users. Can I use similar logic and consider each each movie’s ratings as sequence of rates and classify movies based on that?
You’re welcome!
Perhaps try it and see?
Hi,
Many thanks for the great tutorial! Can we infer the structural info the Convolution layer has found?
Would be nice to see what makes a good review 🙂
Best,
Attila
Sorry, I don’t understand your question, can you please elaborate?
Hello and thanks for your very informative article. I want to ask you if i can use this model for anomaly detection?
I have lots of time series labeled data from iot devices ( each timestamp have a label : 1 or 0 anomaly or not) and i want to know if your strategy could work in this case ?
Thanks a lot!
Yes, this will help:
https://machinelearningmastery.com/faq/single-faq/how-do-i-model-anomaly-detection
Thanks ! I’m checking the literature and i hope you gonna write more about it.
You’re welcome. Me too.
Hey i have observed one thing that is when we are adding dropout layers and recurrent dropout model tends to underfit as we can see train accuracy is less then test accuracy. please explain me why it is so???
You may need to increase learning rate and epochs.
Hello Sir,
First of all, thank you so much for creating such excellent website tutorials. It helps a lot in removing confusion regarding ML and DL. I always visit your website for clearing my doubts and before starting to work on any model.
My current project requires me to report UAR as a metric. I assume that I need to use “recall” as a metric for that, in model.compile().
It is a multiclass classification problem with 3 classes. I am performing one-hot encoding using LabelBinarizer since the original labels are integers.
My model is :
BiLSTM(128) -> BiLSTM(64) -> Activation(relu) -> Dense(16,tanh) -> Dense(3,softmax)
Input shape = (None, 300, 20)
Loss = Categorical Crossentropy
Optimiser = Adam
When the model is training, the recall score is very low, on both Train and Validation Set (sometimes around 0.0023).
But, when the model is passed the Train, Test and Val set separately, using model.predict(),
the recall score reported is much higher (around 0.3 to 0.4).
Q1) Why is this difference so high? Is there any mistake in the model?
Q2) Is there any way by which, for a 3-class problem, I use a single dense node at output and still use recall as metric
In Q2, whenevr I try to do that, I get error, saying that there are many classes to compare
You’re welcome.
I have not heard of UAR, what is it?
Perhaps just focus on manually calculated score on the test set.
No, multi-class classification should use a one output per class and softmax activation.
Thanks for your reply !
1) UAR means “Unweighted average recall”. It is often used when #samples per class are imbalanced.
UAR = the average of the recall for each class, as referred from literature
2) So, It’s OK to have that difference in recall score between model.fit() and model.predict().
3) Alright.
Keras has now included attention layer in its library. Is there any tutorial regarding usage of that with LSTM for sequence classification problem?
Thanks once again!
Thanks.
Yes, focus on the evaluation scores you calculate yourself. Anything else is just an estimate.
I hope to write a tutorial on the topic soon.
Great!, awaiting for the tutorial.
Hi Jason,
Thank you a lot for your great articles. It helps me so much in ML field.
Now I would like to apply the LSTM to classify my data, could you give me some advice, please?
The details of my data set are as follows: (https://drive.google.com/file/d/13TRMLw8YfHSaAbkT0yqp0nEKBXMD_DyU/view?usp=sharing)
I have 10 users, each user at the same time generates some traffic depends on different applications (we have 3 applications). My target is the detection/classification of which application appears in the timestamp.
The output indicates how many types of the application appears in the observation time.
More precisely my dataset looks as follows.
Time index | User ID | Variable 1 | Variable 2 | …. | Variable 7 |||| Output 1 | Output 2 | Output 3
1 1 267 839 2,7 1 0 0
2 3 18057 30525 6.1 1 1 0
……
20000
Since most of the classification problem is a binary classification. So if I have 3 output or more, could I use LSTM to solve my classification problem? Should I keep my data as mentioned above or I have to change it for the input of LSTM? (<— could you give me please a link refers to the data preparation for input LSTM)
Please kindly let me know your thoughts.
Looking forward to hearing from you. Thank you ????
Best regards
Sorry, I don’t have the capacity to review your data.
Perhaps the suggestions here will help, replace sites with users:
https://machinelearningmastery.com/faq/single-faq/how-to-develop-forecast-models-for-multiple-sites
Thank you Jason for your answer.
The idea of multiple sites is fitted with my data. The only thing different that I have one more dataset represents the correlation between each site at each observed time.
Here is my raw data look like:
| Date | UserID | Feat. 1 | Feat 2 | Feat 3 | AppType |
|————|———|——-|————-|———|———–|
| 01/01/2016 | 1 | 0 | 36 | 0 | 1 |
| 01/02/2016 | 1 | 10100 | 42 | 1 | 1 |
| …
| 12/31/2016 | 1 | 14300 | 39 | 1 | 1 |
| 01/01/2016 | 2 | 25000 | 46 | 1 | 3 |
| 01/02/2016 | 2 | 23700 | 43 | 1 | 3 |
| …
| 12/31/2016 | 2 | 20600 | 37 | 1 | 3 |
| …
| 12/31/2016 | 10 | 19800 | 52 | 1 | 2 |
I would like to simultaneously predict the AppType of each UserID at the same observation time.
The idea of developing one model per site is not my purpose because if I have several (>1000) sites, it seems not effective.
I aim to develop one model for all sites but I don’t’ know how to preprocess the input data for LSTM since the input_shape = [no of samples, timestep, no of features].
Do you have any examples related to multiple site LTSM model?
Looking forward to hearing from you. Thank you ????
Best regards
This will give you some ideas:
https://machinelearningmastery.com/faq/single-faq/how-to-develop-forecast-models-for-multiple-sites
Yes, but could you give me some advice about the preprocessing input data for LSTM (in case one model for all sites) please?
The preparation of the data will be based on the type of model/framing of the problem you choose.
Is there any way to avoid this or is it expected?
” UserWarning: Converting sparse IndexedSlices to a dense Tensor of unknown shape. This may consume a large amount of memory.
“Converting sparse IndexedSlices to a dense Tensor of unknown shape.”
Warning is thrown on the fit method.
Not sure off hand, perhaps check google/stackoverflow.
Hi Jason, thank you for your awesome work!!!
But there is always the Question of what is the benefit of using an Embedding-Layer or get the Embedding matrix of a pretrained Modell (Word2Vec) in a Textclassification, if the LSTM Network or the CNN is just learning that the Sequence Embedding1 to Embedding 10 is relevant for the Textclassification.
i think i would get the same results by using a normal Dictionary like:
{this: 1,
Movie : 2,
is: 3
great: 4 …}
since the Model is also getting for similar Words, still a little bit different vectors as Embeddings.
For Example: This Movie is great! –> 5 Stars
so the Network would learn the Sequence 1,2,3,4 –>5 Stars
or Embedding1,E2,E3,E4 –> 5 Stars
So the whole think of finding Similarities with the Embedding Layer is unnecessary.
Or did i missunderstood something?
You’re welcome.
Often an embedding trained as part of a model will result in a better overall model than a model that uses a standalone embedding. At least in my experience.
Models with an embedding perform better than models without, at least in general.
Hello Sir,
Again, formidable work.
I am a little lost in this topic, RNN.
I have some data depending on time, as example [0,1,2,3….300 s].
I would like to train a neural network which could predict in real time.
Is appropiate to use seq2seq?
I would like to put a example.
In real time, 20 seconds have passed, so the RNN uses them to predict the next 280 seconds, but at that time, the time is running. When 40 seconds have spent, the RNN should predict the next 260 s. How is this possible?
Thanks in advance,
Best wishes,
Perhaps try it and compare results to other methods such as classical time series methods, ml methods, mlp, cnn and hybrids.
This tutorial will help to get you started:
https://machinelearningmastery.com/how-to-develop-lstm-models-for-time-series-forecasting/
This will help you prepare your data:
https://machinelearningmastery.com/faq/single-faq/what-is-the-difference-between-samples-timesteps-and-features-for-lstm-input
Thank you very much Prof. Brownlee!
You’re welcome.
Hey. I am somehow perplexed. I wanna start learning NLP from the scratch with your website. (I have gotten familiar with it but not in a standard mode just from different websites) i’m compeletely familiar with coding and specially python. Would you please tell me from where section of your website shall i start learning nlp!?
Since i wanna persuade my education i wanna learn every ins and outs of it.
Thank you in advance
Yes, start here for NLP:
https://machinelearningmastery.com/start-here/#nlp
Hello, can you help me?
The question I have is:
Are sequence data still sequenced after quantitative ?
In other words, there is a sequence in final numerical data and can the LSTM be used?
Sorry, I don’t understands your question. Perhaps you can rephrase it?
The data I work on is gene expression sequence data that, after preprocessing and quantification, is numerical data. Now my question is, is there still sequence in the data?
If observations are ordered by space or time, then it is probably sequence data.
Hello Jason, thank you so much for your thorough explanations. I have an issue and I hope that you could help me.
In my case I have 2000 samples each with 800 time steps. In each sample there are some points of interest (signal goes up (on), signal goes down (off)). I would like to detect those points so I thought that MIMO multi-label classification with lstm could solve my problem. So, I want to classify each time step of each sample as 0 if it is not a point of interest, or 1 if it is. Eventually I’m interested only in ones so I’m not sure if it is the best method to follow.
Because more than 98% of the timesteps are zeroes the lstm keeps giving my only zeroes after the training. I have implemented a custom weighted binary cross entropy function and I give 0.97 weight to the ones and 0.03 to zeros, but the algorithm keeps giving me only zeros as a result for each prediciton.
Do you have any suggestions on how to solve this highly skewed problem? I read about over-sampling and under-sampling but this won’t help in my problem as it is natural to have this very little number of active points (ones).
Thanks a lot in advance
Perhaps you can model it as an imbalanced classification problem and use as cost-sensitive lstm?
Hello Jason again,
I found your article: cost-sensitive-neural-network-for-imbalanced-classification which was very interesting. The difference is that I’m working with lstm neural network and that I want to classify each timestep.
It was very interesting the comment about the weights. That Fractions that represent the same ratio do not have the same effect. I changed my weights from {0: 0.02, 1:0.98} to {0:2. , 1:98.} and now almost all my output labels are turning into ones!!
The loss function after one or two epochs stops stays constant. Do you have any suggestions on how to help my code finding correctly the labels? because before it was setting everythin to zero and now it sets almost all into ones. Would a sliding window help in that case?
Thanks a lot!
Perhaps you can explore different model configurations, data preparation and class weights and discover what works well or best for your dataset.
Thank you Jason, your example is simply put and well articulated. This was big help!
You’re welcome.
Hi thanks for the great tutorial
I have an assignment to classify the outcome of hospital Intensive care. I have 1000 patient records with a variable length of observation (time series on 4 different features values such as blood pressure ,temperature,…) taken every hour or such for each patient
Goal is to predict outcome (recovery or death) based on the 1000 patients treining set
How should I setup the Model? Is there a standard approach to to this’
thanks
You’re welcome.
I recommend comparing a suite of different data preparation methods, different models, and different model configurations in order to discover what works well or best for your dataset.
Hi Jason, thank you for the very helpful tutorials. I tried using the code from the example, however I seem to be having a couple of errors.
When loading the dataset, I’m getting VisibleDeprecationWarning . I believe the problem is due to newer versions of Numpy not liking lists of unequal length items. Apparently the solution is to use “dtype=object”, but I’m not sure where to put it in that line.
Also, when creating the model, specifically the line:
model.add(LSTM(100))
python returns the error:
NotImplementedError: Cannot convert a symbolic Tensor (lstm_2/strided_slice:0) to a numpy array. This error may indicate that you’re trying to pass a Tensor to a NumPy call, which is not supported
Any idea what is causing this, and how to remedy it?
You’re welcome.
Perhaps you can safely ignore the warning for now?
Regarding the error, perhaps these tips will help:
https://machinelearningmastery.com/faq/single-faq/why-does-the-code-in-the-tutorial-not-work-for-me
Nice tutorial, I have a question on masking: Correct me if I am wrong but the mask produced in the Embedding Layer will stop propagating through the network after the first LSTM layer because return_sequences is False by default.
That means that the mask is not propagated to the output and as a result it’s not propagating to the loss. Is that correct? And if so is it really an issue? I understand that LSTM layer knows how to handle the produced padding given the mask, but I was wondering whether the loss should be masked as well.
Yes, it is only handled up to the point of the last LSTM layer. This should be sufficient for most applications.
Perhaps compare the results with alternate approaches if you’re concerned.
Hello sir, you have published a great tutorial on sequence classification using LSTM. I also have the same type of problem to solve. However, I have to calculate the confusion matrix. Therefore, I calculate the confusion matrix on your code(first code segment, i.e. without using dropout) using the X_test dataset. But it is not classified accurately by the model. Hence the confusion matrix does not display a good result. I calculate the confusion matrix on your code as follows:
loss=model.evaluate(X_test, y_test, verbose=0)
print(“loss= “,loss)
predictions =np.argmax(model.predict(X_test), axis=-1)#(model1.predict(X_test) > 0.5).astype(“int32”)
print(“Prediction= “, predictions)
conf_mat=confusion_matrix(y_test,predictions)
print(“Confusion matrix=\n”,conf_mat)
It displays the following output:
loss= [0.29482781887054443, 0.8802400231361389]
Prediction= [0 0 0 … 0 0 0]
Confusion matrix=
[[12500 0]
[12500 0]]
Please tell me at which portion I made a mistake? How do I calculate the confusion matrix using X_test data on your model?
Perhaps this example will help you calculate a confusion matrix:
https://machinelearningmastery.com/how-to-calculate-precision-recall-f1-and-more-for-deep-learning-models/
I have a question regarding batch size:
since each sample is a complete “review”, when (or why) would you set the batch size to 1?
Based on my understanding of LSTMs and batch size, the model weights would be updated after each review (batch size=1) vs taking into account multiple reviews (batch size >1).
Thanks in advance!
Yes, it does not make sense to preserve state across reviews in a batch. It also does not appear to impact the model.
Interesting Jason. As I reason that out, I assume that the batch size doesn’t seem to matter because this is framed as a classification problem. So the network compares to “y” for each sample, even though the state is updated after samples. Because when state is reset, it is reset after trying to determine the right states for each of the samples in the batch to optimize classification accuracy. is that a correct way to rationalize this result?
I think state matters much less on many problems, it rarely seems to have an impact when I run experiments.
Hello Jason,
I have read your article and would like to use it on my project. I am doing a language classification task. When I try your code as it is, it works. However, when I try to replace the IMDB data with my dataset, it does not work. So, would you please help me how to feed my dataset on your code?
I don’t have the capacity to customize the example for you sorry.
This is the error message it returns:
~\Anaconda3\envs\myenv\lib\site-packages\tensorflow\python\framework\ops.py in __array__(self)
843
844 def __array__(self):
–> 845 raise NotImplementedError(
846 “Cannot convert a symbolic Tensor ({}) to a numpy array.”
847 ” This error may indicate that you’re trying to pass a Tensor to”
NotImplementedError: Cannot convert a symbolic Tensor (lstm_6/strided_slice:0) to a numpy array. This error may indicate that you’re trying to pass a Tensor to a NumPy call, which is not supported
Perhaps these tips will help:
https://machinelearningmastery.com/faq/single-faq/can-you-change-the-code-in-the-tutorial-to-___
Hello Jason,
I’m doing a little project of mine, a multivariate time series classification one.
My input data has 3 features, and 4000 timesteps, and the total number of samples is 500000
So my input shape should be (None, 4000, 3), if I am not wrong.
The output is in the range 0-1, which makes me think I should use softmax or sigmoid in the final Dense layer
But the model never achieves any more than 50% accuracy
I’ve tried many architectures, Stacked LSTM, GRUs, Conv1D, I played around with the number of filters/units and tried different activations (ReLU, PReLU, LeakyReLU, tanh, sigmoid), with no success
I used different learning rates on all of the architectures I tried, learning rate from 0.000001 to 10
Nothing seems to work. What do you advise?
If you get no more than 50% accuracy consistently, flipping around the result will give you always more than 50% accuracy. But that can be many reason you cannot improve the prediction. One example is that the input and classification are really unrelated.
first, i would like to thank you very much for this amazing tutorial, but i have a problem and i would really appreciate it if i get some help: when i run the code on Spyder it outputs the following error:
runfile(‘C:/Users/Amenii/Desktop/IGC/MLproject/imdb.py’, wdir=’C:/Users/Amenii/Desktop/IGC/MLproject’)
Traceback (most recent call last):
File “C:\Users\Amenii\Desktop\IGC\MLproject\imdb.py”, line 14, in
(x_train, y_train), (x_test, y_test) = imdb.load_data(num_words=top_words)
File “C:\Users\Amenii\anaconda3\lib\site-packages\tensorflow\python\keras\datasets\imdb.py”, line 116, in load_data
rng = np.random.RandomState(seed)
File “mtrand.pyx”, line 183, in numpy.random.mtrand.RandomState.__init__
File “_mt19937.pyx”, line 130, in numpy.random._mt19937.MT19937.__init__
File “C:\Users\Amenii\anaconda3\lib\contextlib.py”, line 74, in inner
with self._recreate_cm():
File “C:\Users\Amenii\anaconda3\lib\site-packages\numpy\core\_ufunc_config.py”, line 436, in __enter__
File “C:\Users\Amenii\anaconda3\lib\site-packages\numpy\core\_ufunc_config.py”, line 314, in seterrcall
raise ValueError(“Only callable can be used as callback”)
ValueError: Only callable can be used as callback
It seems like some version mismatch issue?
How to predict the new query given to us?
How will I vectorize the new query text with same indexes we used while loading the dataset (num_words = 5000)???
Please help asap.
Hi Ojjaswi…please rephrase or otherwise elaborate on your question so that we better assist you.
How to predict the new query given to us?
How will I vectorize the new query text with same indexes we used while loading the dataset (num_words = 5000)???
Please help with the procedure.
Please help asap.
Great explanation, the way you put everything is a masterpiece
Thank you for the kind words and feedback, Amit!
I am working in Windows environment and could advance sufficiently in installing the packages and running the scripts by following the steps. The website is very helpful.
However, when defining the LSTM model by using the command:
model = Sequential()
the following error message is received:
NameError: name ‘Sequential’ is not defined.
Please advice how to rectify the fault.
Thanks.
Hi Jain…Please confirm that you have properly installed in TensorFlow and properly added the Sequential to your model. See the final code listing in the tutorial.
Is the above model suitable for a classification that is not only binary? Using a different compile, for example:
model.compile(loss=’categorical_crossentropy’, optimizer=opt, metrics=[‘accuracy’])
Hi Rom…You may adjust the following code for this purpose:
for i in range(len(test)):
# make prediction
predictions.append(history[-1])
# observation
history.append(test[i])
Hi Jason
I really appreciate your great articles.
In pad_sequences I changed padding and truncating to ‘post’ instead of its default which is ‘pre’. The results became awful, the model was learning nothing. Do you have any idea why it happens?
Hi,
First of all I want to express my appreciation for your nice website and useful training. I am looking for a simple way to draw my deep learning general architecture of a hybrid model. Could you please offer me the best one you know?
Thanks in advance.
Mina
Hello Sir,
I really like your tutorial I am working on a project for Hyperspectral Image classification (HSI) data. these image dataset contain (145*145*200) shape (H, W, channel). I want to extract the information from the channel (200). my question is how can I use CONVLSTM3D or CONVLSTM2D layers with time sequence? is the time sequence represent 200 channels? if you give me any example or hint I shall be very thankful to you.
Hi Tahir…Our most comprehensive resource can be found here:
https://machinelearningmastery.com/deep-learning-for-time-series-forecasting/
There are many examples and full code listings to get you started on your own projects.
Hi,
I really like your posts – in regard to the above models, is it possible to add learning rate to the model build, or is it included in some other way? thankyou in advance!
Hi Signe…The following resource may be of interest to you:
https://datascience.stackexchange.com/questions/48736/how-to-see-change-learning-rate-in-keras-lstm
Hello ,
thank you Jason Brownlee so much for a useful document and explanation.
I am wondering about using LSTM for training a sequences of dataset that represents the distance (x,y,z) for 4 keypoints. so my dataset is with a shape of (50,12) , with 12 features .
I did the train of the LSTM model with different timestep , and found that the optimal timestep was on 100 ,athough my data was with 50 timesteps/frames. i used lstm for did a classification for 3 classes.
my lstm model is basic as bellow :
Model: “sequential_35”
_________________________________________________________________
Layer (type) Output Shape Param #
=================================================================
lstm_70 (LSTM) (None, 100, 30) 5160
lstm_71 (LSTM) (None, 10) 1640
dense_35 (Dense) (None, 3) 33
=================================================================
Total params: 6,833
Trainable params: 6,833
Non-trainable params: 0
_________________________________________________________________
the plot of accuracy shows that train accuracy is increased ,while the valid one kept constant.
the plot of losses shows that the train loss and the valid loss are decreased.
the average accuracy after doing 10 trials = 98.039%
but when test / predict the mode on some sequences , it did mistakes , it did not give accurate classes to some sequences .
what do you think ? and how can i improve my model to get more accurate results of prediction for new related dataset.
taking into considrateion that when predict the results , and used to padd my data of 50 frames and shape of (50,12) to become (1,100,12) , the code i used was bellow :
#if you have a LSTM with 100 timespets , and a input sequnce of shape of (50,12)
# Pad the sequence to (100, 12)
padded_sequence = pad_sequences([X_new], maxlen=100, padding=’post’, truncating=’post’, dtype=’float32′)
# Add an extra dimension to represent the batch size
reshaped_sequence = padded_sequence.reshape((1, 100, 12))
please give me an advice about that.
Hi Alaa…You may find the following resources of interest that investigate options for improving LSTM performance:
https://machinelearningmastery.com/tune-lstm-hyperparameters-keras-time-series-forecasting/
https://machinelearningmastery.com/use-dropout-lstm-networks-time-series-forecasting/
Thank you for sharing your insights on prepadding versus postpadding. Your explanation regarding the impact of word position on weight updates in recurrent neural networks like LSTMs is quite illuminating. Indeed, considering the chain rule, padding zeros at the beginning of a sequence allows the network to better focus on learning the content towards the end of the sequence, which may have a more significant influence on weight updates. This thoughtful approach to preprocessing sequences sheds light on the nuances of optimizing neural network architectures for various tasks.
Hi priya…you are very welcome! Let us know how you proceed with your models.