Recurrent neural networks can also be used as generative models.
This means that in addition to being used for predictive models (making predictions), they can learn the sequences of a problem and then generate entirely new plausible sequences for the problem domain.
Generative models like this are useful not only to study how well a model has learned a problem but also to learn more about the problem domain itself.
In this post, you will discover how to create a generative model for text, character-by-character using LSTM recurrent neural networks in Python with Keras.
After reading this post, you will know:
- Where to download a free corpus of text that you can use to train text generative models
- How to frame the problem of text sequences to a recurrent neural network generative model
- How to develop an LSTM to generate plausible text sequences for a given problem
Kick-start your project with my new book Deep Learning for Natural Language Processing, including step-by-step tutorials and the Python source code files for all examples.
Let’s get started.
Note: LSTM recurrent neural networks can be slow to train, and it is highly recommended that you train them on GPU hardware. You can access GPU hardware in the cloud very cheaply using Amazon Web Services. See the tutorial here.
- Aug/2016: First published
- Update Oct/2016: Fixed a few minor comment typos in the code
- Update Mar/2017: Updated for Keras 2.0.2, TensorFlow 1.0.1 and Theano 0.9.0
- Update Sep/2019: Updated for Keras 2.2.5 API
- Update Jul/2022: Updated for TensorFlow 2.x API
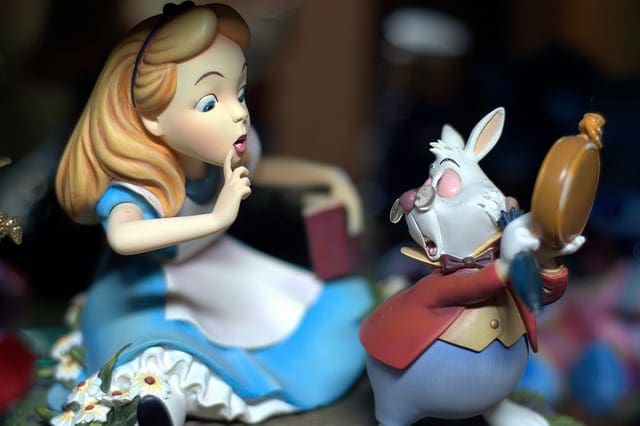
Text generation with LSTM recurrent neural networks in Python with Keras
Photo by Russ Sanderlin, some rights reserved.
Problem Description: Project Gutenberg
Many of the classical texts are no longer protected under copyright.
This means you can download all the text for these books for free and use them in experiments, like creating generative models. Perhaps the best place to get access to free books that are no longer protected by copyright is Project Gutenberg.
In this tutorial, you will use a favorite book from childhood as the dataset: Alice’s Adventures in Wonderland by Lewis Carroll.
You will learn the dependencies between characters and the conditional probabilities of characters in sequences so that you can, in turn, generate wholly new and original sequences of characters.
This is a lot of fun, and repeating these experiments with other books from Project Gutenberg is recommended. Here is a list of the most popular books on the site.
These experiments are not limited to text; you can also experiment with other ASCII data, such as computer source code, marked-up documents in LaTeX, HTML or Markdown, and more.
You can download the complete text in ASCII format (Plain Text UTF-8) for this book for free and place it in your working directory with the filename wonderland.txt.
Now, you need to prepare the dataset ready for modeling.
Project Gutenberg adds a standard header and footer to each book, which is not part of the original text. Open the file in a text editor and delete the header and footer.
The header is obvious and ends with the text:
1 |
*** START OF THIS PROJECT GUTENBERG EBOOK ALICE'S ADVENTURES IN WONDERLAND *** |
The footer is all the text after the line of text that says:
1 |
THE END |
You should be left with a text file that has about 3,330 lines of text.
Need help with LSTMs for Sequence Prediction?
Take my free 7-day email course and discover 6 different LSTM architectures (with code).
Click to sign-up and also get a free PDF Ebook version of the course.
Develop a Small LSTM Recurrent Neural Network
In this section, you will develop a simple LSTM network to learn sequences of characters from Alice in Wonderland. In the next section, you will use this model to generate new sequences of characters.
Let’s start by importing the classes and functions you will use to train your model.
1 2 3 4 5 6 7 8 9 |
import numpy as np import tensorflow as tf from tensorflow.keras.models import Sequential from tensorflow.keras.layers import Dense from tensorflow.keras.layers import Dropout from tensorflow.keras.layers import LSTM from tensorflow.keras.callbacks import ModelCheckpoint from tensorflow.keras.utils import to_categorical ... |
Next, you need to load the ASCII text for the book into memory and convert all of the characters to lowercase to reduce the vocabulary the network must learn.
1 2 3 4 5 |
... # load ascii text and covert to lowercase filename = "wonderland.txt" raw_text = open(filename, 'r', encoding='utf-8').read() raw_text = raw_text.lower() |
Now that the book is loaded, you must prepare the data for modeling by the neural network. You cannot model the characters directly; instead, you must convert the characters to integers.
You can do this easily by first creating a set of all of the distinct characters in the book, then creating a map of each character to a unique integer.
1 2 3 4 |
... # create mapping of unique chars to integers chars = sorted(list(set(raw_text))) char_to_int = dict((c, i) for i, c in enumerate(chars)) |
For example, the list of unique sorted lowercase characters in the book is as follows:
1 |
['\n', '\r', ' ', '!', '"', "'", '(', ')', '*', ',', '-', '.', ':', ';', '?', '[', ']', '_', 'a', 'b', 'c', 'd', 'e', 'f', 'g', 'h', 'i', 'j', 'k', 'l', 'm', 'n', 'o', 'p', 'q', 'r', 's', 't', 'u', 'v', 'w', 'x', 'y', 'z', '\xbb', '\xbf', '\xef'] |
You can see that there may be some characters that we could remove to further clean up the dataset to reduce the vocabulary, which may improve the modeling process.
Now that the book has been loaded and the mapping prepared, you can summarize the dataset.
1 2 3 4 5 |
... n_chars = len(raw_text) n_vocab = len(chars) print "Total Characters: ", n_chars print "Total Vocab: ", n_vocab |
Running the code to this point produces the following output.
1 2 |
Total Characters: 147674 Total Vocab: 47 |
You can see the book has just under 150,000 characters, and when converted to lowercase, there are only 47 distinct characters in the vocabulary for the network to learn—much more than the 26 in the alphabet.
You now need to define the training data for the network. There is a lot of flexibility in how you choose to break up the text and expose it to the network during training.
In this tutorial, you will split the book text up into subsequences with a fixed length of 100 characters, an arbitrary length. You could just as easily split the data by sentences, padding the shorter sequences and truncating the longer ones.
Each training pattern of the network comprises 100 time steps of one character (X) followed by one character output (y). When creating these sequences, you slide this window along the whole book one character at a time, allowing each character a chance to be learned from the 100 characters that preceded it (except the first 100 characters, of course).
For example, if the sequence length is 5 (for simplicity), then the first two training patterns would be as follows:
1 2 |
CHAPT -> E HAPTE -> R |
As you split the book into these sequences, you convert the characters to integers using the lookup table you prepared earlier.
1 2 3 4 5 6 7 8 9 10 11 12 |
... # prepare the dataset of input to output pairs encoded as integers seq_length = 100 dataX = [] dataY = [] for i in range(0, n_chars - seq_length, 1): seq_in = raw_text[i:i + seq_length] seq_out = raw_text[i + seq_length] dataX.append([char_to_int[char] for char in seq_in]) dataY.append(char_to_int[seq_out]) n_patterns = len(dataX) print "Total Patterns: ", n_patterns |
Running the code to this point shows that when you split up the dataset into training data for the network to learn that you have just under 150,000 training patterns. This makes sense as, excluding the first 100 characters, you have one training pattern to predict each of the remaining characters.
1 |
Total Patterns: Â 147574 |
Now that you have prepared your training data, you need to transform it to be suitable for use with Keras.
First, you must transform the list of input sequences into the form [samples, time steps, features] expected by an LSTM network.
Next, you need to rescale the integers to the range 0-to-1 to make the patterns easier to learn by the LSTM network using the sigmoid activation function by default.
Finally, you need to convert the output patterns (single characters converted to integers) into a one-hot encoding. This is so that you can configure the network to predict the probability of each of the 47 different characters in the vocabulary (an easier representation) rather than trying to force it to predict precisely the next character. Each y value is converted into a sparse vector with a length of 47, full of zeros, except with a 1 in the column for the letter (integer) that the pattern represents.
For example, when “n” (integer value 31) is one-hot encoded, it looks as follows:
1 2 3 |
[ 0. 0. 0. 0. 0. 0. 0. 0. 0. 0. 0. 0. 0. 0. 0. 0. 0. 0. 0. 0. 0. 0. 0. 0. 0. 0. 0. 0. 0. 0. 0. 1. 0. 0. 0. 0. 0. 0. 0. 0. 0. 0. 0. 0.] |
You can implement these steps as below:
1 2 3 4 5 6 7 |
... # reshape X to be [samples, time steps, features] X = np.reshape(dataX, (n_patterns, seq_length, 1)) # normalize X = X / float(n_vocab) # one hot encode the output variable y = to_categorical(dataY) |
You can now define your LSTM model. Here, you define a single hidden LSTM layer with 256 memory units. The network uses dropout with a probability of 20. The output layer is a Dense layer using the softmax activation function to output a probability prediction for each of the 47 characters between 0 and 1.
The problem is really a single character classification problem with 47 classes and, as such, is defined as optimizing the log loss (cross entropy) using the ADAM optimization algorithm for speed.
1 2 3 4 5 6 7 |
... # define the LSTM model model = Sequential() model.add(LSTM(256, input_shape=(X.shape[1], X.shape[2]))) model.add(Dropout(0.2)) model.add(Dense(y.shape[1], activation='softmax')) model.compile(loss='categorical_crossentropy', optimizer='adam') |
There is no test dataset. You are modeling the entire training dataset to learn the probability of each character in a sequence.
You are not interested in the most accurate (classification accuracy) model of the training dataset. This would be a model that predicts each character in the training dataset perfectly. Instead, you are interested in a generalization of the dataset that minimizes the chosen loss function. You are seeking a balance between generalization and overfitting but short of memorization.
The network is slow to train (about 300 seconds per epoch on an Nvidia K520 GPU). Because of the slowness and because of the optimization requirements, use model checkpointing to record all the network weights to file each time an improvement in loss is observed at the end of the epoch. You will use the best set of weights (lowest loss) to instantiate your generative model in the next section.
1 2 3 4 5 |
... # define the checkpoint filepath="weights-improvement-{epoch:02d}-{loss:.4f}.hdf5" checkpoint = ModelCheckpoint(filepath, monitor='loss', verbose=1, save_best_only=True, mode='min') callbacks_list = [checkpoint] |
You can now fit your model to the data. Here, you use a modest number of 20 epochs and a large batch size of 128 patterns.
1 |
model.fit(X, y, epochs=20, batch_size=128, callbacks=callbacks_list) |
The full code listing is provided below for completeness.
1 2 3 4 5 6 7 8 9 10 11 12 13 14 15 16 17 18 19 20 21 22 23 24 25 26 27 28 29 30 31 32 33 34 35 36 37 38 39 40 41 42 43 44 45 46 47 48 49 |
# Small LSTM Network to Generate Text for Alice in Wonderland import numpy as np from tensorflow.keras.models import Sequential from tensorflow.keras.layers import Dense from tensorflow.keras.layers import Dropout from tensorflow.keras.layers import LSTM from tensorflow.keras.callbacks import ModelCheckpoint from tensorflow.keras.utils import to_categorical # load ascii text and covert to lowercase filename = "wonderland.txt" raw_text = open(filename, 'r', encoding='utf-8').read() raw_text = raw_text.lower() # create mapping of unique chars to integers chars = sorted(list(set(raw_text))) char_to_int = dict((c, i) for i, c in enumerate(chars)) # summarize the loaded data n_chars = len(raw_text) n_vocab = len(chars) print("Total Characters: ", n_chars) print("Total Vocab: ", n_vocab) # prepare the dataset of input to output pairs encoded as integers seq_length = 100 dataX = [] dataY = [] for i in range(0, n_chars - seq_length, 1): seq_in = raw_text[i:i + seq_length] seq_out = raw_text[i + seq_length] dataX.append([char_to_int[char] for char in seq_in]) dataY.append(char_to_int[seq_out]) n_patterns = len(dataX) print("Total Patterns: ", n_patterns) # reshape X to be [samples, time steps, features] X = np.reshape(dataX, (n_patterns, seq_length, 1)) # normalize X = X / float(n_vocab) # one hot encode the output variable y = to_categorical(dataY) # define the LSTM model model = Sequential() model.add(LSTM(256, input_shape=(X.shape[1], X.shape[2]))) model.add(Dropout(0.2)) model.add(Dense(y.shape[1], activation='softmax')) model.compile(loss='categorical_crossentropy', optimizer='adam') # define the checkpoint filepath="weights-improvement-{epoch:02d}-{loss:.4f}.hdf5" checkpoint = ModelCheckpoint(filepath, monitor='loss', verbose=1, save_best_only=True, mode='min') callbacks_list = [checkpoint] # fit the model model.fit(X, y, epochs=20, batch_size=128, callbacks=callbacks_list) |
Note: Your results may vary given the stochastic nature of the algorithm or evaluation procedure, or differences in numerical precision. Consider running the example a few times and compare the average outcome.
After running the example, you should have a number of weight checkpoint files in the local directory.
You can delete them all except the one with the smallest loss value. For example, when this example was run, you can see below the checkpoint with the smallest loss that was achieved.
1 |
weights-improvement-19-1.9435.hdf5 |
The network loss decreased almost every epoch, so the network could likely benefit from training for many more epochs.
In the next section, you will look at using this model to generate new text sequences.
Generating Text with an LSTM Network
Generating text using the trained LSTM network is relatively straightforward.
First, you will load the data and define the network in exactly the same way, except the network weights are loaded from a checkpoint file, and the network does not need to be trained.
1 2 3 4 5 |
... # load the network weights filename = "weights-improvement-19-1.9435.hdf5" model.load_weights(filename) model.compile(loss='categorical_crossentropy', optimizer='adam') |
Also, when preparing the mapping of unique characters to integers, you must also create a reverse mapping that you can use to convert the integers back to characters so that you can understand the predictions.
1 2 |
... int_to_char = dict((i, c) for i, c in enumerate(chars)) |
Finally, you need to actually make predictions.
The simplest way to use the Keras LSTM model to make predictions is to first start with a seed sequence as input, generate the next character, then update the seed sequence to add the generated character on the end and trim off the first character. This process is repeated for as long as you want to predict new characters (e.g., a sequence of 1,000 characters in length).
You can pick a random input pattern as your seed sequence, then print generated characters as you generate them.
1 2 3 4 5 6 7 8 9 10 11 12 13 14 15 16 17 18 |
... # pick a random seed start = np.random.randint(0, len(dataX)-1) pattern = dataX[start] print("Seed:") print("\"", ''.join([int_to_char[value] for value in pattern]), "\"") # generate characters for i in range(1000): x = np.reshape(pattern, (1, len(pattern), 1)) x = x / float(n_vocab) prediction = model.predict(x, verbose=0) index = np.argmax(prediction) result = int_to_char[index] seq_in = [int_to_char[value] for value in pattern] sys.stdout.write(result) pattern.append(index) pattern = pattern[1:len(pattern)] print("\nDone.") |
The full code example for generating text using the loaded LSTM model is listed below for completeness.
1 2 3 4 5 6 7 8 9 10 11 12 13 14 15 16 17 18 19 20 21 22 23 24 25 26 27 28 29 30 31 32 33 34 35 36 37 38 39 40 41 42 43 44 45 46 47 48 49 50 51 52 53 54 55 56 57 58 59 60 61 62 63 64 65 |
# Load LSTM network and generate text import sys import numpy as np from tensorflow.keras.models import Sequential from tensorflow.keras.layers import Dense from tensorflow.keras.layers import Dropout from tensorflow.keras.layers import LSTM from tensorflow.keras.callbacks import ModelCheckpoint from tensorflow.keras.utils import to_categorical # load ascii text and covert to lowercase filename = "wonderland.txt" raw_text = open(filename, 'r', encoding='utf-8').read() raw_text = raw_text.lower() # create mapping of unique chars to integers, and a reverse mapping chars = sorted(list(set(raw_text))) char_to_int = dict((c, i) for i, c in enumerate(chars)) int_to_char = dict((i, c) for i, c in enumerate(chars)) # summarize the loaded data n_chars = len(raw_text) n_vocab = len(chars) print("Total Characters: ", n_chars) print("Total Vocab: ", n_vocab) # prepare the dataset of input to output pairs encoded as integers seq_length = 100 dataX = [] dataY = [] for i in range(0, n_chars - seq_length, 1): seq_in = raw_text[i:i + seq_length] seq_out = raw_text[i + seq_length] dataX.append([char_to_int[char] for char in seq_in]) dataY.append(char_to_int[seq_out]) n_patterns = len(dataX) print("Total Patterns: ", n_patterns) # reshape X to be [samples, time steps, features] X = np.reshape(dataX, (n_patterns, seq_length, 1)) # normalize X = X / float(n_vocab) # one hot encode the output variable y = to_categorical(dataY) # define the LSTM model model = Sequential() model.add(LSTM(256, input_shape=(X.shape[1], X.shape[2]))) model.add(Dropout(0.2)) model.add(Dense(y.shape[1], activation='softmax')) # load the network weights filename = "weights-improvement-19-1.9435.hdf5" model.load_weights(filename) model.compile(loss='categorical_crossentropy', optimizer='adam') # pick a random seed start = np.random.randint(0, len(dataX)-1) pattern = dataX[start] print("Seed:") print("\"", ''.join([int_to_char[value] for value in pattern]), "\"") # generate characters for i in range(1000): x = np.reshape(pattern, (1, len(pattern), 1)) x = x / float(n_vocab) prediction = model.predict(x, verbose=0) index = np.argmax(prediction) result = int_to_char[index] seq_in = [int_to_char[value] for value in pattern] sys.stdout.write(result) pattern.append(index) pattern = pattern[1:len(pattern)] print("\nDone.") |
Running this example first outputs the selected random seed, then each character as it is generated.
Note: Your results may vary given the stochastic nature of the algorithm or evaluation procedure, or differences in numerical precision. Consider running the example a few times and compare the average outcome.
For example, below are the results from one run of this text generator. The random seed was:
1 2 |
be no mistake about it: it was neither more nor less than a pig, and she felt that it would be quit |
The generated text with the random seed (cleaned up for presentation) was:
1 2 3 4 5 6 7 8 9 10 11 12 13 14 15 16 |
be no mistake about it: it was neither more nor less than a pig, and she felt that it would be quit e aelin that she was a little want oe toiet ano a grtpersent to the tas a little war th tee the tase oa teettee the had been tinhgtt a little toiee at the cadl in a long tuiee aedun thet sheer was a little tare gereen to be a gentle of the tabdit soenee the gad ouw ie the tay a tirt of toiet at the was a little anonersen, and thiu had been woite io a lott of tueh a tiie and taede bot her aeain she cere thth the bene tith the tere bane to tee toaete to tee the harter was a little tire the same oare cade an anl ano the garee and the was so seat the was a little gareen and the sabdit, and the white rabbit wese tilel an the caoe and the sabbit se teeteer, and the white rabbit wese tilel an the cade in a lonk tfne the sabdi ano aroing to tea the was sf teet whitg the was a little tane oo thete the sabeit she was a little tartig to the tar tf tee the tame of the cagd, and the white rabbit was a little toiee to be anle tite thete ofs and the tabdit was the wiite rabbit, and |
Let’s note some observations about the generated text.
- It generally conforms to the line format observed in the original text of fewer than 80 characters before a new line.
- The characters are separated into word-like groups, and most groups are actual English words (e.g., “the,” “little,” and “was”), but many are not (e.g., “lott,” “tiie,” and “taede”).
- Some of the words in sequence make sense(e.g., “and the white rabbit“), but many do not (e.g., “wese tilel“).
The fact that this character-based model of the book produces output like this is very impressive. It gives you a sense of the learning capabilities of LSTM networks.
However, the results are not perfect.
In the next section, you will look at improving the quality of results by developing a much larger LSTM network.
Larger LSTM Recurrent Neural Network
You got results, but not excellent results in the previous section. Now, you can try to improve the quality of the generated text by creating a much larger network.
You will keep the number of memory units the same at 256 but add a second layer.
1 2 3 4 5 6 7 8 |
... model = Sequential() model.add(LSTM(256, input_shape=(X.shape[1], X.shape[2]), return_sequences=True)) model.add(Dropout(0.2)) model.add(LSTM(256)) model.add(Dropout(0.2)) model.add(Dense(y.shape[1], activation='softmax')) model.compile(loss='categorical_crossentropy', optimizer='adam') |
You will also change the filename of the checkpointed weights so that you can tell the difference between weights for this network and the previous (by appending the word “bigger” in the filename).
1 |
filepath="weights-improvement-{epoch:02d}-{loss:.4f}-bigger.hdf5" |
Finally, you will increase the number of training epochs from 20 to 50 and decrease the batch size from 128 to 64 to give the network more of an opportunity to be updated and learn.
The full code listing is presented below for completeness.
1 2 3 4 5 6 7 8 9 10 11 12 13 14 15 16 17 18 19 20 21 22 23 24 25 26 27 28 29 30 31 32 33 34 35 36 37 38 39 40 41 42 43 44 45 46 47 48 49 50 51 |
# Larger LSTM Network to Generate Text for Alice in Wonderland import numpy as np from tensorflow.keras.models import Sequential from tensorflow.keras.layers import Dense from tensorflow.keras.layers import Dropout from tensorflow.keras.layers import LSTM from tensorflow.keras.callbacks import ModelCheckpoint from tensorflow.keras.utils import to_categorical # load ascii text and covert to lowercase filename = "wonderland.txt" raw_text = open(filename, 'r', encoding='utf-8').read() raw_text = raw_text.lower() # create mapping of unique chars to integers chars = sorted(list(set(raw_text))) char_to_int = dict((c, i) for i, c in enumerate(chars)) # summarize the loaded data n_chars = len(raw_text) n_vocab = len(chars) print("Total Characters: ", n_chars) print("Total Vocab: ", n_vocab) # prepare the dataset of input to output pairs encoded as integers seq_length = 100 dataX = [] dataY = [] for i in range(0, n_chars - seq_length, 1): seq_in = raw_text[i:i + seq_length] seq_out = raw_text[i + seq_length] dataX.append([char_to_int[char] for char in seq_in]) dataY.append(char_to_int[seq_out]) n_patterns = len(dataX) print("Total Patterns: ", n_patterns) # reshape X to be [samples, time steps, features] X = np.reshape(dataX, (n_patterns, seq_length, 1)) # normalize X = X / float(n_vocab) # one hot encode the output variable y = to_categorical(dataY) # define the LSTM model model = Sequential() model.add(LSTM(256, input_shape=(X.shape[1], X.shape[2]), return_sequences=True)) model.add(Dropout(0.2)) model.add(LSTM(256)) model.add(Dropout(0.2)) model.add(Dense(y.shape[1], activation='softmax')) model.compile(loss='categorical_crossentropy', optimizer='adam') # define the checkpoint filepath = "weights-improvement-{epoch:02d}-{loss:.4f}-bigger.hdf5" checkpoint = ModelCheckpoint(filepath, monitor='loss', verbose=1, save_best_only=True, mode='min') callbacks_list = [checkpoint] # fit the model model.fit(X, y, epochs=50, batch_size=64, callbacks=callbacks_list) |
Running this example takes some time, at least 700 seconds per epoch.
Note: Your results may vary given the stochastic nature of the algorithm or evaluation procedure, or differences in numerical precision. Consider running the example a few times and compare the average outcome.
After running this example, you may achieve a loss of about 1.2. For example, the best result achieved from running this model was stored in a checkpoint file with the name:
1 |
weights-improvement-47-1.2219-bigger.hdf5 |
This achieved a loss of 1.2219 at epoch 47.
As in the previous section, you can use this best model from the run to generate text.
The only change you need to make to the text generation script from the previous section is in the specification of the network topology and from which file to seed the network weights.
The full code listing is provided below for completeness.
1 2 3 4 5 6 7 8 9 10 11 12 13 14 15 16 17 18 19 20 21 22 23 24 25 26 27 28 29 30 31 32 33 34 35 36 37 38 39 40 41 42 43 44 45 46 47 48 49 50 51 52 53 54 55 56 57 58 59 60 61 62 63 64 65 66 67 |
# Load Larger LSTM network and generate text import sys import numpy as np from tensorflow.keras.models import Sequential from tensorflow.keras.layers import Dense from tensorflow.keras.layers import Dropout from tensorflow.keras.layers import LSTM from tensorflow.keras.callbacks import ModelCheckpoint from tensorflow.keras.utils import to_categorical # load ascii text and covert to lowercase filename = "wonderland.txt" raw_text = open(filename, 'r', encoding='utf-8').read() raw_text = raw_text.lower() # create mapping of unique chars to integers, and a reverse mapping chars = sorted(list(set(raw_text))) char_to_int = dict((c, i) for i, c in enumerate(chars)) int_to_char = dict((i, c) for i, c in enumerate(chars)) # summarize the loaded data n_chars = len(raw_text) n_vocab = len(chars) print("Total Characters: ", n_chars) print("Total Vocab: ", n_vocab) # prepare the dataset of input to output pairs encoded as integers seq_length = 100 dataX = [] dataY = [] for i in range(0, n_chars - seq_length, 1): seq_in = raw_text[i:i + seq_length] seq_out = raw_text[i + seq_length] dataX.append([char_to_int[char] for char in seq_in]) dataY.append(char_to_int[seq_out]) n_patterns = len(dataX) print("Total Patterns: ", n_patterns) # reshape X to be [samples, time steps, features] X = np.reshape(dataX, (n_patterns, seq_length, 1)) # normalize X = X / float(n_vocab) # one hot encode the output variable y = to_categorical(dataY) # define the LSTM model model = Sequential() model.add(LSTM(256, input_shape=(X.shape[1], X.shape[2]), return_sequences=True)) model.add(Dropout(0.2)) model.add(LSTM(256)) model.add(Dropout(0.2)) model.add(Dense(y.shape[1], activation='softmax')) # load the network weights filename = "weights-improvement-47-1.2219-bigger.hdf5" model.load_weights(filename) model.compile(loss='categorical_crossentropy', optimizer='adam') # pick a random seed start = np.random.randint(0, len(dataX)-1) pattern = dataX[start] print("Seed:") print("\"", ''.join([int_to_char[value] for value in pattern]), "\"") # generate characters for i in range(1000): x = np.reshape(pattern, (1, len(pattern), 1)) x = x / float(n_vocab) prediction = model.predict(x, verbose=0) index = np.argmax(prediction) result = int_to_char[index] seq_in = [int_to_char[value] for value in pattern] sys.stdout.write(result) pattern.append(index) pattern = pattern[1:len(pattern)] print("\nDone.") |
One example of running this text generation script produces the output below.
Note: Your results may vary given the stochastic nature of the algorithm or evaluation procedure, or differences in numerical precision. Consider running the example a few times and compare the average outcome.
The randomly chosen seed text was:
1 2 |
d herself lying on the bank, with her head in the lap of her sister, who was gently brushing away s |
The generated text with the seed (cleaned up for presentation) was :
1 2 3 4 5 6 7 8 9 10 11 12 13 14 15 16 |
herself lying on the bank, with her head in the lap of her sister, who was gently brushing away so siee, and she sabbit said to herself and the sabbit said to herself and the sood way of the was a little that she was a little lad good to the garden, and the sood of the mock turtle said to herself, 'it was a little that the mock turtle said to see it said to sea it said to sea it say it the marge hard sat hn a little that she was so sereated to herself, and she sabbit said to herself, 'it was a little little shated of the sooe of the coomouse it was a little lad good to the little gooder head. and said to herself, 'it was a little little shated of the mouse of the good of the courte, and it was a little little shated in a little that the was a little little shated of the thmee said to see it was a little book of the was a little that she was so sereated to hare a little the began sitee of the was of the was a little that she was so seally and the sabbit was a little lad good to the little gooder head of the gad seared to see it was a little lad good to the little good |
You can see that there are generally fewer spelling mistakes, and the text looks more realistic but is still quite nonsensical.
For example, the same phrases get repeated again and again, like “said to herself” and “little.” Quotes are opened but not closed.
These are better results, but there is still a lot of room for improvement.
10 Extension Ideas to Improve the Model
Below are ten ideas that may further improve the model that you could experiment with are:
- Predict fewer than 1,000 characters as output for a given seed
- Remove all punctuation from the source text and, therefore, from the models’ vocabulary
- Try a one-hot encoding for the input sequences
- Train the model on padded sentences rather than random sequences of characters
- Increase the number of training epochs to 100 or many hundreds
- Add dropout to the visible input layer and consider tuning the dropout percentage
- Tune the batch size; try a batch size of 1 as a (very slow) baseline and larger sizes from there
- Add more memory units to the layers and/or more layers
- Experiment with scale factors (temperature) when interpreting the prediction probabilities
- Change the LSTM layers to be “stateful” to maintain state across batches
Did you try any of these extensions? Share your results in the comments.
Resources
This character text model is a popular way of generating text using recurrent neural networks.
Below are some more resources and tutorials on the topic if you are interested in going deeper. Perhaps the most popular is the tutorial by Andrej Karpathy titled “The Unreasonable Effectiveness of Recurrent Neural Networks.”
- Generating Text with Recurrent Neural Networks [pdf], 2011
- Keras code example of LSTM for text generation
- Lasagne code example of LSTM for text generation
- MXNet tutorial for using an LSTM for text generation
- Auto-Generating Clickbait With Recurrent Neural Networks
Summary
In this post, you discovered how you can develop an LSTM recurrent neural network for text generation in Python with the Keras deep learning library.
After reading this post, you know:
- Where to download the ASCII text for classical books for free that you can use for training
- How to train an LSTM network on text sequences and how to use the trained network to generate new sequences
- How to develop stacked LSTM networks and lift the performance of the model
Do you have any questions about text generation with LSTM networks or this post? Ask your questions in the comments below, and I will do my best to answer them.
Great post. Thanks!
You’re welcome Avi.
Do the loss we get here is equal to number of bits per charecter ????
The loss represents the average difference between the expected and predicted probability distribution.
Hi,
when i try to run the codes, I get an error with the weights file.
ValueError: Dimension 1 in both shapes must be equal, but are 52 and 44 for Assign_13 with input shapes [256,52], [256,44].
Can you please let me know what is happening
Confirm that you have copied and run all of the code and that your environment and libraries are all up to date.
Hi Jason,
Just updated all libraries. Still getting the same error.
Thanks!
–
Sorry, it is not clear to me what your fault could be.
Jason,
Thanks. I got it to work now!!! But I am getting random results will work on it further. But i feel like it is a good start. Thanks for the codes!
Thanks!
Well done on your progress, hang in there!
You have to make the shape equal, either [256,44], [256,44] (or) [256,52], [256,52].
This was caused by the weights.hdf5 file being incompatible with the new data in the repository. I have updated the repo and it should work now.
I have the same issue. Where to download the hdf5 file. Thank youl
pls how did you update the repo
I’m excited to try combining this with nltk… it’s probably going to be long and frustrating, but I’ll try to let you know my results.
Thanks for sharing!
Good luck Max, report back and let us know how you go.
Hi Max,
Did you get anywhere with combining NLTK with this?
Just curious…
I really like your article, thank you for sharing. I can launch your code but I have a crash after finalization of 1st epoch:
—————————————————
Traceback (most recent call last):
File “train.py”, line 61, in
model.fit(X, y, nb_epoch=20, batch_size=128, callbacks=callbacks_list)
File “/afs/in2p3.fr/home/s/sbilokin/.local/lib/python2.7/site-packages/keras/models.py”, line 620, in fit
sample_weight=sample_weight)
File “/afs/in2p3.fr/home/s/sbilokin/.local/lib/python2.7/site-packages/keras/engine/training.py”, line 1104, in fit
callback_metrics=callback_metrics)
File “/afs/in2p3.fr/home/s/sbilokin/.local/lib/python2.7/site-packages/keras/engine/training.py”, line 842, in _fit_loop
callbacks.on_epoch_end(epoch, epoch_logs)
File “/afs/in2p3.fr/home/s/sbilokin/.local/lib/python2.7/site-packages/keras/callbacks.py”, line 40, in on_epoch_end
callback.on_epoch_end(epoch, logs)
File “/afs/in2p3.fr/home/s/sbilokin/.local/lib/python2.7/site-packages/keras/callbacks.py”, line 296, in on_epoch_end
self.model.save(filepath, overwrite=True)
File “/afs/in2p3.fr/home/s/sbilokin/.local/lib/python2.7/site-packages/keras/engine/topology.py”, line 2427, in save
save_model(self, filepath, overwrite)
File “/afs/in2p3.fr/home/s/sbilokin/.local/lib/python2.7/site-packages/keras/models.py”, line 56, in save_model
model.save_weights_to_hdf5_group(model_weights_group)
File “/afs/in2p3.fr/home/s/sbilokin/.local/lib/python2.7/site-packages/keras/engine/topology.py”, line 2476, in save_weights_to_hdf5_group
dtype=val.dtype)
File “/afs/in2p3.fr/home/s/sbilokin/.local/lib/python2.7/site-packages/h5py/_hl/group.py”, line 108, in create_dataset
self[name] = dset
File “_objects.pyx”, line 54, in h5py._objects.with_phil.wrapper (/scratch/pip_build_sbilokin/h5py/h5py/_objects.c:2513)
File “_objects.pyx”, line 55, in h5py._objects.with_phil.wrapper (/scratch/pip_build_sbilokin/h5py/h5py/_objects.c:2466)
File “/afs/in2p3.fr/home/s/sbilokin/.local/lib/python2.7/site-packages/h5py/_hl/group.py”, line 277, in __setitem__
h5o.link(obj.id, self.id, name, lcpl=lcpl, lapl=self._lapl)
File “_objects.pyx”, line 54, in h5py._objects.with_phil.wrapper (/scratch/pip_build_sbilokin/h5py/h5py/_objects.c:2513)
File “_objects.pyx”, line 55, in h5py._objects.with_phil.wrapper (/scratch/pip_build_sbilokin/h5py/h5py/_objects.c:2466)
File “h5o.pyx”, line 202, in h5py.h5o.link (/scratch/pip_build_sbilokin/h5py/h5py/h5o.c:3726)
RuntimeError: Unable to create link (Name already exists)
—————————————————–
The file names are unique, probably there is a collision of names in keras or h5py for me.
Could you help me please?
Found my mistake, I didn’t edit topology.py in keras correctly to fix another problem.
This is my second attempt to study NN, but I always have problems with versions, errors, dependencies and this scares me away.
For example now I have a problem to load the weights, using the example above on python 3 with intermediate weight files:
Traceback (most recent call last):
File “bot.py”, line 49, in
model.load_weights(filename)
File “.local/lib/python3.3/site-packages/keras/engine/topology.py”, line 2490, in load_weights
self.load_weights_from_hdf5_group(f)
File “.local/lib/python3.3/site-packages/keras/engine/topology.py”, line 2533, in load_weights_from_hdf5_group
weight_names = [n.decode(‘utf8’) for n in g.attrs[‘weight_names’]]
File “h5py/_objects.pyx”, line 54, in h5py._objects.with_phil.wrapper (/scratch/pip_build_/h5py/h5py/_objects.c:2691)
File “h5py/_objects.pyx”, line 55, in h5py._objects.with_phil.wrapper (/scratch/pip_build_/h5py/h5py/_objects.c:2649)
File “/.local/lib/python3.3/site-packages/h5py/_hl/attrs.py”, line 58, in __getitem__
attr = h5a.open(self._id, self._e(name))
File “h5py/_objects.pyx”, line 54, in h5py._objects.with_phil.wrapper (/scratch/pip_build_/h5py/h5py/_objects.c:2691)
File “h5py/_objects.pyx”, line 55, in h5py._objects.with_phil.wrapper (/scratch/pip_build_/h5py/h5py/_objects.c:2649)
File “h5py/h5a.pyx”, line 77, in h5py.h5a.open (/scratch/pip_build_/h5py/h5py/h5a.c:2179)
KeyError: “Can’t open attribute (Can’t locate attribute)”
I don’t think I can give you good advice if you are modifying the Keras framework files.
Good luck!
Hi Jason,
I followed your tutorial and then built my own sequence-to-sequence model, trained at word level.
Might soon share results and code, but wanted first to thank you for the great post, helped me a lot getting started with Keras.
Keep up the amazing work!
Great, well done Alex. It would be cool if you can post or link to your code.
hi
I know it is an old post but did you Alex ever shared your code for word level training?
thanks
Cristiano
I have a few examples on the blog:
https://machinelearningmastery.com/?s=language+model&submit=Search
When I try to get the unique set of characters:
I get the following:
[‘\n’, ‘ ‘, ‘!’, ‘”‘, “‘”, ‘(‘, ‘)’, ‘*’, ‘,’, ‘-‘, ‘.’, ‘:’, ‘;’, ‘?’, ‘[‘, ‘]’, ‘_’, ‘a’, ‘b’, ‘c’, ‘d’, ‘e’, ‘f’, ‘g’, ‘h’, ‘i’, ‘j’, ‘k’, ‘l’, ‘m’, ‘n’, ‘o’, ‘p’, ‘q’, ‘r’, ‘s’, ‘t’, ‘u’, ‘v’, ‘w’, ‘x’, ‘y’, ‘z’, ‘\xbb’, ‘\xbf’, ‘\xef’]
Note, that the ‘\r’ is missing, why ?
Thank you
It is not needed on some platforms, like Unix and friends. Only windows uses CRLF.
Thank you for the reply.
I am using windows.
I am running the fitting as I type.
🙂
Great!
Thank you for your reply, Jason.
Question:
Can I generate a text book the size of Alice in Wonderland using the same technique ?
And if so, how ?
Do I generate 50,000 characters for example ?
And how do I use a “SEED” to actually generate such a text ?
In the example you are using a 100 characters as a way to breakup the text and expose it to the network. DOES increasing the characters help with producing a more meaningful text ?
And another question ?
How many epochs should I run the fitting ? 100, 200 ? Because the loss keeps decreasing, but if it gets close to zero is that a good thing ?
Sorry for so many questions.
Thank you VERY VERY MUCH 🙂
Great questions, but these are research questions.
You would have to experiment and see.
I did a similar project, and I removed non ASCII characters using:
ascii_values = ascii_values[np.where(ascii_values < 128)]
Great tip!
Hey, nice article. Can you explain why you are using 256 as your output dimension for LSTM? Does the reasoning for 256 come from other parameters?
The network only outputs a single character.
There are 256 nodes in the LSTM layers, I chose a large number to increase the representational capacity of the network. I chose it arbitrarily. You could experiment with different values.
awesome explanation. Thanks for sharing
I’m glad you found it useful Lino.
Thanks for the nice article. Why do we have to specify the number of times-teps beforehand in LSTM ( in input_shape)? What if different data points have different number of sequence lengths? How can we make keras lstm work in that case?
Hi Shamit,
The network needs to know the size of data so that it can prepare efficient data structures (backend computation) for the model.
If you have variable length sequences, you will need to pad them with zeros. Keras offers a padding function for this purpose:
https://keras.io/preprocessing/sequence/
Hi Jason
Thanks a lot for the code and the easy explanations.
Only a short notice: For the model checkpoints you will need the h5py module which was not preinstalled with my python. I obviously only noticed it after the first epoch, when keras tried to import it. Might be a good idea for people to check before they waste time running the code on slow hardware like I did 🙂
Nice one, thanks Julian!
Hi Jason
I ran the exact same code of your small LSTM network on the same book(wonderland.txt) for 20 epochs. But the generated text in my case is not making any sense. A snippet of it is as below:
Seed:
” e chin.
æiÆve a right to think,Æ said alice sharply, for she was beginning to
feel a little worried ”
up!uif!tbccju!xp!cf!b!mpsu!pg!uif!tbbufs!bos!uif!ebsufs-!boe!uifo!tif
xbt!bom!uif!ebuufs!xiui!uif!sbtu!po!uif!!boe!uif!xbt!bpmjoh!up!uif!
bpvme-
uif!nbuuf!xbt!b!mpsumf!ujfff!up!cf!b!mpsu!pg!uif!tbbufs!bos!uif!ibouf
uif!tbt!pg!uif!xbt!tp!tff!pg!uif!xbt!pp!bo!bo!pg!tifof!!bou!uif!xbt!bom
!tif!ibe!tv!co!bo!uif!sbccju!xpvme!cf!bspmpvt!up!tffff!
uif!ibe!tp!cfe
Could you give some insights on why this is happening and how to fix it?
Ouch, something is going on there Bruce.
Perhaps confirm Keras 1.1.0 and TensorFlow 0.10.
It looks like maybe the network was not trained for long enough or the character conversion from ints is not working out.
Also, conform that there were no copy-paste errors.
Hey Bruce, any updates on this? I tried using one-hot vectors as inputs instead of the ones mentioned in the post. I’m getting a similar output to yours. Something along the lines of this:
xxw’?,p?9l5),d-?l?sxwx?fbb?flw?g5ps-up ?’xx?,)lqc?lrex?fqp,)xw?gfu-fwf ,,x?up ?bxvcxexw?)fwgxg?,pq54d-;wp9?ql5),[?p ,?p;?,)x?;pwwfs)-lq?bp’x?l’
How to take the seed from user instead of program generating the random text ?
You can read from sys.stdin, for example:
For user input you can use userinput = stdin.readline()
For ex the input is:
userinput = “Alice was beginning to get very tired of sitting by her sister on the bank, and of having nothing to”
sort_sen = sorted(list(p))
pattern = [char_to_int[value.lower()] for value in sort_sen]
This is how you can deal with user input. Hope this helps.
Thanks for sharing!
Hi Jason,
Thank you so much for the great post!
Can you please explain the need to reshape the X ?
What is wrong with the initial shape of list of lists?
Thank you!
Hi Victor, great question.
All LSTM input must be in the form [samples, timesteps, features]. The data was loaded as [samples, timesteps]. Rather than timesteps, think of sequence – it’s the same thing.
I hope that helps.
Using a similar approach: Can one generate numeric sequences from time series data, much like sentences? I don’t see a reason why we can’t. Any input is appreciated, Jason. thanks.
For sure, change the output from one node to n-nodes.
For sequence output, you’ll need a lot more data and a lot more training.
Thank you, but why should we change to n-nodes? Considering you generated a sequence of text, can’t I get a numeric sequence with the same 1 node setup?
Also, I don’t understand “index = numpy.argmax(prediction)
result = int_to_char[index]” part of the code. Can you please explain why its necessary?
I’m new to your website….Keep up the great work! Looking to hear from you.
Hi ATM,
Yes, there are a few ways to frame a sequence prediction problem. You can use a one-step prediction model many times. Another approach is to predict part or the entire sequence at once, and all the levels in between.
The line:
Selects the node with the largest output value. The index of the node is the same as the index of the class to predict.
I hope that helps.
Thanks for the clarification, Jason: I got the code running with decent predictions for time series data.
I think you might want to add in the tutorial that prediction usually works well for only somewhat statistically stationary datasets, regardless of training size?
I’ve tried it on both stationary and non-stationary, and I’ve come to this conclusion (which makes sense). Very often, time series collected from both financial and scientific datasets are not stationary, so LSTM has to be used very conservatively.
Thanks ATM! I agree, I’ll be going into a lot more details on stationary time series and making non-stationary data stationary in coming blog posts.
Hi jason,
I have been running this. But thebloss instead of decreasing is always increasing. So far, i ran it for 20 epochs. Do you have any idea what might be the case. I didn’t change anything in the program, though.
Best,
Sban
Maybe your network is too small? Maybe overlearning?
hello,
how would you handle a much bigger dataset ? I scraped all public declarations of the French goverment for the past few years so my corpus is Total Characters: 465163150
Total Vocab: 146
the dataX and dataY will likely crash due to size constraints, how could I circumvent that issue and use the full dataset ?
Perhaps read the data in batches from disk – I believe Keras has this capability for images (data generator), perhaps for text too? I’m not sure off hand.
Hi Jason,
Thanks for the example. I wonder about the loss function: categorical cross-entropy. I tried to find the source code but was not successful with it. Do you know what a loss of 1.2 actually means? Is there a unit to this number?
From my understanding, the goal is to somehow get the network to learn a probability distribution for the predictions as similar as possible to the one of the training data. But how similar is 1.2?
Obviously a loss of 0 would mean that the network could accurately predict the target to any given sample with 100% accuracy which is already quite difficult to imagine as the output to this network is not binary but rather a softmax over an array with values for all characters in the vocabulary.
Thanks again.
Hi Julian,
Great question. I need to dedicate a post to this question, thanks for the prompt.
Cross entropy is also called log loss in your googling, the math is here:
https://en.wikipedia.org/wiki/Cross_entropy
I’ll work on a write-up ASAP.
Hey, thanks for the post, will this work on Theano instead of tensorflow? I understand Keras can work with both, and I am having difficulties installing tensorflow on my mac
Yes, the code will work on either backend.
Thanks, TensorFlow worked after all. I am running the training on a big data like the book with these messages:
Total Characters: 237084
Total Vocab: 50
Total Patterns: 236984
on Epoche 13, loss is 1.5. I had to stop it there since it took my MacBook Pro 20 hours to create 13 epoches. Anyways, this is my result, notice it starts to repeat itself at a certain point:
is all the love and the sain on my baby
bnd i love you to be a line to be a line
to be a line to me, i’m she way the lov
es me down and i do and i do and i do an
d i do and i do and i do and i do and i
do and i do and i do and i do and i do a
nd i do and i do and i do and i do and i
do and i do and i do and i do and i do
and i do and i do and i do and i do and
This is another result from another run of the program:
oe mine i want you to ban tee the sain o
f move and i do and i do and i love you
to be a line to me, i’m she way the way
the way the way the way the way the way
the way the way the way the way the way
the way the way the way the way the way
the way the way the way the way the way
the way the way the way the way the way
This is pretty frustrating (while still incredibly awesome), do you have an idea why this should happen?
Regardless, this is such a great post that gives access to RNN and LSTM in a great nature!
maybe it is overfitting?
Well done!
The network may require greater representational capacity. Try more layers and/or more neurons per layer.
Also try running on AWS to give your poor laptop a break:
https://machinelearningmastery.com/develop-evaluate-large-deep-learning-models-keras-amazon-web-services/
Thanks! i will also try breaking into words instead of letters. Will update 🙂
Would we get better result if we trained the network at the word level instead of character? We would split the dataset into words, index them, the same way we do with the characters, and use them as input. Wouldn’t that at least eliminate the made up words in the generated text making it seem more plausible?
Yep. Try it out Jack! I’d love to see the result.
I agree that the word level modeling would be much more intuitive and sentences might show some meaningful results.
One issue is that you have 47 features to choose from if you go character level. However, the number of unique words is orders of magnitude larger than unique characters. Therefore, your network becomes much larger. You can add an embedding to reduce input dimensionality, however, the output softmax will still be large.
Is there any benchmark dataset for this task, to actually evaluate the model ?
Not as far as I’m aware Fateh.
Hi Jason amazing post! I have a doubt. I tried the following: instead of training with Alice’s Adventures, I train with this a list of barcodes. Unique barcodes in a plain text (example, 123455, 143223, etc etc) and they can be of different lengths. Is this approach still valid? What i want to do is to input a potentially barcode with errors (maybe a character is missing) and the LSTM returns me “the correct one”. Any suggestion? Many thanks in advance!
Nice idea Don.
LSTMs can indeed correct sequences, but they would need to memorize all of the barcodes. You would then have to train it to fill in the gaps (0) with the missing values.
Very nice idea!
You could also try uses an autoencoder to do the same trick.
I’d love to hear how you go.
Thanks Jason! For sure i will come back 🙂
Do you have any suggested read for either LSTM as a “word corrector” or an autoencoder for that task?
Best!
Sorry I don’t Don. Good luck with your project!
Hi Jason, thank you very much for your post.
I was thinking about changing the input and output to be a coordinate, which is 2D vector (x and y position) to predict movement.
I understood that we can change the input to be a vector instead of scalar. For example using one hot vector like you’re suggesting.
However, I don’t understand what to do when we want the output to be a 2D vector. Does this mean that I don’t need to use softmax layer as the output?
Thanks for your help.
Hi Alex, interesting idea – movement generator.
You can output a vector by changing the number of neurons in the output layer.
Hi Jason,
The features in the exercise are characters which are mapped to integers. It’s like replacing a nominal vector by a continuous variable. Isn’t that an issue?
Also, would you consider using Embedding as the first layer in this model – why or why not.
Thank you, your posts are immensely helpful.
Hi Ashok,
The input data is ordinal, either as chars or ints. The neural net expects to work with arrays of numbers instead of chars, so we use ints here.
An embedding layer would create a projection for the chars into a higher dimensional space. That would not be useful here as we are trying to learn and generalize the way sequences of chars are put together.
I could be wrong though, try it and see if you can make it work – perhaps with projections of word sequences?
Thanks Jason. This is helpful.
On the embedding layer, I have another question – how are the projections learnt? Is it a simple method like multiplying with a known matrix or are they learnt iteratively? If iteratively, then are they learnt a) first before the other weights or b) are the embedding projection weights learnt along with the other weights.
Ashok
Great question Ashok.
The embedding layer is not really described well in the Keras doc:
https://keras.io/layers/embeddings/
This SO post helps:
http://stackoverflow.com/questions/38189713/what-is-an-embedding-in-keras
Jason:
The below code for text generation is treating chars as nominal variables and hence giving assigning a separate dimension for each. Since this is a more complex approach, I will check if this leads to an improvement.
Again, thanks for your response. It only added to my curiosity .
https://github.com/fchollet/keras/blob/master/examples/lstm_text_generation.py
I’d love to hear how the representational approaches compare in practice Ashok.
Jason, could you explain please how the input data is ordinal? Instead of one-hot encoding we simply enumerate characters.
I have the same question. Have you found any answer?
If your input is text, you can use:
– an integer encoding of the sequence
– a bag of word encoding (e.g. counts of tf-idf)
– one hot encoding of integer encoding
– word embedding (word2vec, glove or learned)
Does that help?
Hello Jason!
Nice work. But I don’t really understand what is the point of applying RNN for this particular task. If one wants to predict the next character of a 100-long sequence, this is seems me achievable with any other common regression task where you feed in the training sequences and the following single character as output while training. What is the additional feat of your approach?
Yes, here are many ways to approach this problem.
LSTMs are supposed to particularly good at modeling the underlying PDF of char or words in a text corpus.
The example doesn’t run anymore under TensorFlow 1.0.0. (previous versions are OK, at least with 0.10.0rc0).
What error do you see Hendrik?
Great post Jason, I was trying to do almost the same thing and your post gave a lot of help. As some of the comments suggest, the RNN seems to achieve a loop quite quickly. I was wondering what you meant with “Add dropout to the visible input layer and consider tuning the dropout percentage.”, seems it appears to attack this problem.
You can apply regularization to the LSTMs on recurrent and input connections.
I have found adding a drop out of 40% or so on input connections for LSTMs very useful on other projects.
More details here:
https://keras.io/layers/recurrent/#lstm
Actually Jason,
I advanced my experiments and found some interesting things, I will probably do a Medium about it.
For the loop problem, as mentioned in http://karpathy.github.io/2015/05/21/rnn-effectiveness/, makes no sense to always use the argmax in the generation. Since the network output a list of probabilities is easy to randomically sample letter using this distribution:
def sample_prediction(char_map, prediction):
rnd_idx = np.random.choice(len(prediction), p=prediction)
return char_map[rnd_idx]
That simple change made the network to avoid loops and be much more diverse. (Using
temperature as you mentioned will be even better)
Here is a sample of a one-layer network (same as yours), trained with Cervante’s Dom
Quixote, for 20 epochs:
=====
from its beginning to its end, and above all manner; as so able to be almost unable to repeat the, which he had protected him to leave it. As now, for her face because I will be, that he ow her its missing their resckes my eyes, proved
for the descance in the mouth as saying, to eat for a
shepherdess and this knight ais, they
did so that was rockly than to possess the new lose of, that in a sword of now another
golden without a change on which not this; if shore of the Micomicona his dame, ‘To know some little why as capacquery; I will make any ammorimence is seen in their
countless
soicour and the adventure of her damsel a pearls that shows and vonquished callshind him, away itself, her fever and evil comrisoness by where they
will show not in time in which all the chain
himself of the
solmings of the hores of this
your nyiugh and it,
should have punisented, not to portion for, as it
just be-with his sort rich as the shaken of the
sun to
three lady male love?”
Am I he will not believe. I will disreel her more so knight, for
=====
Isn’t that cool? I hope that helps other people.
Very cool Gustavo, thanks for sharing!
First of all big thanks to Jason for such a valuable write up.
I have 1 question :
To Gustavo Führ and Jason Brownlee
Could you please explain in a little more detail what you did there. i didn’t understand, how you got such a good output on a single layer.
I ran my training on this book as my data -> http://www.gutenberg.org/cache/epub/5200/pg5200.txt
See below the output i am getting..
Seed :
see it coming. we can’t all work as hard as we have to and then come hometo be tortured like this, we can’t endure it. i can’t endure it anymore.” and she broke out so heavily in tears that they flo
Prediction :
led to het hemd and she was so the door and she was so the door and she was so the door and she was so the door and she was so the door and she was so the door and she was so the door and she was so the door and she was so the door and she was so the door and she was so the door and she was so the door and she was so the door and
As you can see here a text is repeating itself. This the result of 20th epoch out of 50 epochs in a dual layer LSTM with 256 cells and a sequence of 200 characters.
This is a result pattern i am seeing in all my trainings and i believe i am doing something wrong here. Could you please help out?
According to code above it repeats because of the MOST probable (argmax) on the line:
index = numpy.argmax(prediction)
and it should rather be:
index = numpy.random.choice(len(prediction[0]),p=prediction[0])
Now this works but it is giving gobeldegook instead of semi-english repeating words. Perhaps I need to train for hours longer and have loss <2.5?
I had a very similar experience in my own experimentation. This comment verified what I had found — thank you for sharing Jatin!
what is the need for normalization
# normalize
X = X / float(n_vocab)
This normalizes the integer values by the largest integer value. All values are rescaled to 0-1.
Is this because it’s using a relu activation function? Or because generally you need input values to be between 0-1?
LSTMs prefer normalized inputs – confirm this with some experiments (I have).
Hello~ I am your one of fans.
I have a question about lstm settings that I may apply your text generation model in a different way.
Your model generated texts by adding(updating) a character.
Do you think it is possible to generate texts by adding a word rather than a character.
If it is possible, adding word_embedding layer is effective for a performance of text generation??
Yes, I expect it may even work better June. Let me know how you go.
Thanks, Jason.
I will try it~!
You know, I am a doctoral student majored in management information system.
My research interest include medical informatics and healthcare business analytics.
Your excellent blog posts have helped me a lot.
I appreciated it so much!
I’m really glad to hear that. Thanks for your support and your kind words, I really appreciate it.
Hi,
Very interesting example – can’t wait to try training on other texts!
I have a question regarding the training of this, or in fact any neural network, on a GPU – I have a couple of CNNs written but that I can’t execute :(.
Suppose I am using either Amazon EC2 or Google Cloud, I can successfully log into the instance and run a simple ANN using just a CPU but I am totally confused as to how to get the GPU working. I am accessing the instance from Windows 10. Can you tell me the exact steps I need to do – presumably I need to get CUDA and CUDNN somehow? Then is there any other stuff I need to do or can I just pip install the necessary packages and then execute my code?
Thank you so much.
My best advice would be to use an AMI that is already set up to use the GPU.
I have step by step instructions here:
https://machinelearningmastery.com/develop-evaluate-large-deep-learning-models-keras-amazon-web-services/
nice post!
two questions,
1) what is the role of “seq_in = [int_to_char[value] for value in pattern]” in line 63 (doesn’t seem to be used in the loop) and,
2) could you expand on where and how Gustavo’s fix is needed? (I also get repeated predictions using argmax)
Does your Deep Learning book expand on (2) in regards to LSTM’s?
It seems problematic to output the same predictions over different inputs.
seq_in is the input sequence used in prediction. Agreed it is not needed in the latter part of the example.
His fix is not needed, it can just reduce the size of the universe of possible values. My book does not go into more detail on this.
I am working on a new book dedicated to LSTMs that may be of interest when it is released (next month).
Here is my implementation of Gustavo’s suggestions so that the predicted output tends to avoid repeated patterns
def sample_prediction(prediction):
“””Get rand index from preds based on its prob distribution.
Params
——
prediction (array (array)): array of length 1 containing array of probs that sums to 1
Returns
——-
rnd_idx (int): random index from prediction[0]
Notes
—–
Helps to solve problem of repeated outputs.
len(prediction) = 1
len(prediction[0]) >> 1
“””
X = prediction[0] # sum(X) is approx 1
rnd_idx = np.random.choice(len(X), p=X)
return rnd_idx
for i in range(num_outputs):
x = np.reshape(pattern, (1, len(pattern), 1))
x = x / float(n_vocab)
prediction = model.predict(x, verbose=0)
#index = numpy.argmax(prediction)
# per Gustavo’s suggestion, we should not use argmax here
index = sample_prediction(prediction)
result = int_to_char[index]
#seq_in = [int_to_char[value] for value in pattern]
# not sure why seq_in was here
sys.stdout.write(result)
pattern.append(index)
pattern = pattern[1:len(pattern)]
print “\nDone.”
Nice!
Hi Jason, great post.
I wanted to ask you if there is a way to train this system or use the current setup with dynamic input length.
The reason for that is if one to use this for real text generation, given a random seed of variable length say.. “I have a dream” or “To be or not to be” would it be possible to still generate coherent sentences if we train using dynamic length?
I tried with pad sequence in the predict stage (not the train stage) to match the input length for shorter sentences, but that doesn’t seem to work.
Regards
Yes, you are on the right track.
I would recommend zero-padding all sequences to be the same length and see how the model fairs.
You could try Masking the input layer and see what impact that has.
You could also try truncating sequences.
I have a post scheduled that gives many ways to handle input sequences of different lengths, perhaps in a few weeks.
That would be much appreciated. Looking forward to it.
Hi, thank you for the post. I was wondering if the subsequent post (discussed in this question thread) about implementing dynamically sized user input was ever posted? If so, which article is it? Thank you!
You can use padding or truncation:
https://machinelearningmastery.com/data-preparation-variable-length-input-sequences-sequence-prediction/
Hi,
may I ask about these two lines
seq_in = raw_text[i:i + seq_length]
seq_out = raw_text[i + seq_length]
should model predict next one abc -> d instead of abc -> c
Hi,
I need to thank you for all the good work on Keras, wounderful and awesome package.
However, i got a floating point exception (core dumped) running the code. Please, i need your advise to resolve the issues. I have upgrade the my keras from 1.0.8 to 2.0.1 and the issues is still the same.
88/200 [============>……………..] – ETA: 27s – loss: 11.3334 – acc: 0.0971{‘acc’: array(0.10470587760210037, dtype=float32), ‘loss’: array(9.283703804016113, dtype=float32), ‘batch’: 88, ‘size’: 17}
89/200 [============>……………..] – ETA: 26s – loss: 11.3103 – acc: 0.0972Floating point exception (core dumped)
Warm Regards
I’m sorry to hear that.
Perhaps you could try a different backend (theano or tensorflow)?
Perhaps you could try posting to stackoverflow or the keras user group?
Thank you Jason.
Hi guys,
With Tensorflow backend, i got different error messages. After about 89 batch from the second epochs, the loss become nan and the accuracy also the same. Any suggestion or advices
87/200 [============>……………..] – ETA: 113s – loss: 9.4303 – acc: 0.0947{‘acc’: 0.10666667, ‘loss’: 9.2033749, ‘batch’: 87, ‘size’: 32}
88/200 [============>……………..] – ETA: 112s – loss: 9.4277 – acc: 0.0949{‘acc’: 0.10862745, ‘loss’: 9.2667055, ‘batch’: 88, ‘size’: 17}
89/200 [============>……………..] – ETA: 110s – loss: 9.4259 – acc: 0.0950{‘acc’: nan, ‘loss’: nan, ‘batch’: 89, ‘size’: 0}
90/200 [============>……………..] – ETA: 108s – loss: nan – acc: nan {‘acc’: nan, ‘loss’: nan, ‘batch’: 90, ‘size’: 0}
91/200 [============>……………..] – ETA: 106s – loss: nan – acc: nan{‘acc’: nan, ‘loss’: nan, ‘batch’: 91, ‘size’: 0}
92/200 [============>……………..] – ETA: 105s – loss: nan – acc: nan{‘acc’: nan, ‘loss’: nan, ‘batch’: 92, ‘size’: 0}
This can happen and is caused by exploding or vanishing gradients.
Try using a clipnorm on the optimization algorithm:
https://keras.io/optimizers/
Thanks for the example. Piush.
You’re welcome.
Hi Jason,
I tried your suggestion and the problem of nan for loss and accuracy still the same after second epoch while the batch size is 39/50.
I have also try all the activation function and regularization but still the same problems. Too bad!
Hi Jason,
The problem of loss and accuracy becoming nan after few epoch as to do with the batch generator. I fix it now.
Thank you so much.
Glad to hear it.
Hello Jason,
I was wondering how I could add more hidden layers. I was wondering if this could help generate text with more efficiency
I tried using model.add(LSTM(some_number)) again but it failed and gave me the error:
“Input 0 is incompatible with layer lstm_3: expected ndim=3, found ndim=2”
Hello Jason,
Thanks for your great post it helped me a lot. Since I finished reading your post, I was thinking of how to implement it in a word level instead of character level. I am just confused of how to implement it because with characters we only have few characters but with words we might have say 10000 or even more. Would you please share your thoughts. I am really excited to see the results in a words-level and make further enhancements.
Great idea.
I would recommend ranking all words by frequency the assign integers to each word based on rank. New words not in the corpus can then also be assigned integers later.
hi jason , thanks for such an awesome post
I keep getting the error :
ImportError:
load_weights
requires h5py.even if i have installed h5py, did anyone else face this error, please help
Perhaps your environment cannot see the h5py library?
Confirm you installed it the correct way for your environment.
Try important the library itself and refine env until it works.
Let me know how you go.
It was solved, I just restarted python after installing h5py.
when I predicted using the model, it through the characters like this, whats up with the 1s why are they coming?
t1
h1
e1
1
s1
e1
r1
e1
1
t1
o1
1
t1
e1
e1
1
w1
e1
r1
1
i1
n1
1
a1
n1
d1
I don’t know. Confirm that you have a copy of the code from the tutorial without modification.
can we use print() in place of sys.stdout.write()
Sure.
Hi Jason,
Please, I need your help. I try to validate my test data using the already training result from the model but I got the following error:
##################
AttributeError Traceback (most recent call last)
in ()
1 # load weights into new model
—-> 2 model_info.load_weights(save_best_weights)
3 predictions = model.predict([test_q1, test_q2, test_q1, test_q2,test_q1, test_q2], verbose = True)
AttributeError: ‘History’ object has no attribute ‘load_weights’
In [ ]:
#####################
Below is the snippets of my code:
###Fitting model
save the best weights for predicting the test question pairs
save_best_weights = “weights-pairs1.h5”
checkpoint = ModelCheckpoint(filepath, monitor=’loss’, verbose=1, save_best_only=True, mode=’min’)
callbacks = [ModelCheckpoint(save_best_weights, monitor=’val_loss’, save_best_only=True),
EarlyStopping(monitor=’val_loss’, patience=5, verbose=1, mode=’auto’)]
start = time.time()
model_info=merged_model.fit([x1, x2, x1, x2, x1, x2], y=y, batch_size=64, epochs=3, verbose=True,
validation_split=0.33, shuffle=True, callbacks=callbacks)
end = time.time()
print(“Minutes elapsed: %f” % ((start – end) / 60.))
#####evaluting
#load weights into a new model
model_info.load_weights(save_best_weights)
predictions = model.predict([test_q1, test_q2, test_q1, test_q2,test_q1, test_q2], verbose = True)
Rgeards
See this tutorial on how to load a saved model:
https://machinelearningmastery.com/save-load-keras-deep-learning-models/
Hello Jason,
Great tutorial. I have just one doubt though, in np.reshape command what does feature mean?
and why is it set to 1?
The raw data are sequences of integers. There is only one observation (feature) per time step and it is an integer. That is why the first reshape specifies one feature.
Excellent tutorial!
I have one question. I need predict some words inside text and I currently use LSTM based on your code and binary coding. Is a good practice to use n-words behind and k-words ahead of word which I want to predict?
It’s good to train model on data like this or we must rather use only data behind our prediction?
Try a few method and see what works best on your problem.
Thanks, This can make a good reference for me
I’m glad to hear it.
Why not one hot encode the numbers?
Sure, try it.
Also try an encoding layer.
There are many ways to improve the example Joe, let me know how you go.
Jason,
Great post. Thanks much. I am very new to LSTM. I am trying to recreate the codes here. How to increase the number of epochs?
Thanks
Change the value after “epochs=”
Hi Jason,
Thank you so much for these guides and tutorials! I’m finding them to be very helpful.
I’m glad to hear that Greg.
Hi Jason,
Thanks for your great post, and all the other great post as a matter of fact. I’m interested to train the model on padded sentences rather than random sequences of characters, but it’s not clear to me how to implement it. Can you please elaborate about it and give an example?
Many thanks!
Don
Keras has a great pad function for this:
https://keras.io/preprocessing/sequence/
Does that help?
Thanks again for the post and all the info! I have another question. What should I do if I want to predict by a inserting a string shorter than 100 characters?
Many thanks again!
Use padding:
https://machinelearningmastery.com/data-preparation-variable-length-input-sequences-sequence-prediction/
Thanks!
I have a question regarding the number of parameter in the model and the amount of data. When I look at the summary of the simplest model, I get: Total params: 275,757.0, Trainable params: 275,757.0, and Non-trainable params: 0.0 (for some reason I didn’t succeed to sent a reply with the whole summary).
The number of characters in the Alice book is about 150,000. Thus, isn’t the number of parameter larger than the number of characters (data)?
Thanks again!
Yes.
Isn’t that over-fitting? I ask because you suggested to improve the quality of results by developing an even much larger LSTM network. But if in the simple LSTM network you already have more parameters than data, shouldn’t you simplify the network even more?
Thanks a lot for all the tips!
A simpler model is preferred, but overfitting is only the case when skill on test/validation data is worse than train data.
Thanks Dr.Jason,
What if I want to output the probability of a sequence under a trained model rather than finding the most probable next charterer.
The use case that I have in mind is to feed the model test sentence and it prints the probability of this sentence being true under the model
Much thanks in advance
That would be a different framing of the problem, or perhaps you can collect the probabilities of each char step by step.
So when I train, Xs is similarly he sequence, but what would the Ys be ?
Thanks!
The next char or word in the sequence.
If we do this then how it will differ from the original framing of the problem? I mean the resulted probability will measure the how likely it is a character X will be the next rather than the probability of the seen sequence under the trained model
e.g. if we feed the model with “I am” and the next word either [egg or Sam]. Following the above-reply the output will be the probability of egg or Sam to be the next. Rather, I need to find the probability of the entire segment ” I am Sam” and “I am egg” to be able to tell which one makes more sense
Yes, it is the same framing. The difference is how you handle the probabilities. Sorry, I should have been clearer.
E.g. you can beam search the probabilities across each word/char, or output the probabilities for a specific output sequence, or list the top n most likely output sequences.
Does that make sense?
Hi Jason,
Thanks for the tutorial. When I am using one-hot encoded input, my accuracy and loss is not improving and becomes stagnant
function:
def one_hot_encode(sequences, next_chars, char_to_idx):
X = np.zeros((n_patterns, seq_length, N_CHARS),dtype=float)
y = np.zeros((n_patterns, N_CHARS),dtype=float)
for i, sequence in enumerate(sequences):
for t, char in enumerate(sequence):
X[i, t, char] = 1
#print(i,t,char)
y[i,[next_chars[i]]] = 1
return X, y
X, y = one_hot_encode(dataX, dataY, char_to_int)
Is it the layer size or a bug in one_hot_encoding?
The fault is not obvious to me, sorry.
Perhaps this list of ideas may give you ways to help lift model skill:
https://machinelearningmastery.com/improve-deep-learning-performance/
Hi, thanks for the great tutorial!
If I begin with some random character and use the trained model to predict the next one, how can the network generate different sentences using the same first character?
It will predict probabilities across all output characters and you can use a beam search through those probabilities to get multiple different output sequences.
Hello,
I’m attempting to run the lastf ull code example for generating text using the loaded LSTM model.
However, line 59 simply produces the same number (effectively a space character) each time across the whole 1000 range.
Not sure what I’m doing wrong ?
Sorry to hear that Sam.
Have you tried to run the example a few times?
Have you confirmed that your environment is up to date?
I did the experiment with a different corpus of text; Shakespeare’s sonnets, two LSTM layers of 256, and 20 epochs (probably needs longer). I found that the method in your post where you simply choose the next character from the maximum output, quickly produces very repetitive text – the same two lines repeat after a while, like this:
Seed:
” raise is crowned,
but those same tongues that give thee so thine own,
in other accents do this p ”
oor so me
the world with the shee the world with thee,
the world with the shee the world wour self stolne,
the world with the shee the world with thee shee steel.
12
the world with the the world with the dearty see,
the world with the shee the world with thee wour self,
the world with the shee the world with thee shee shee,
the world with the shee the world wour self so bear,
the world with the shee the world with thee shee shee,
the world with the shee the world wour self so bear,
the world with the shee the world with thee shee shee,
the world with the shee the world wour self so bear,
the world with the shee the world with thee shee shee,
the world with the shee the world wour self so bear,
the world with the shee the world with thee shee shee,
the world with the shee the world wour self so bear,
the world with the shee the world with thee shee shee,
the world with the shee the world wour self so bear,
the world with t
However, if you introduce some randomness by sampling the prediction probability distribution randomly, you get much more interesting results – although it’s gibberish, there are many gibberish words that are pronounceable – ie not just randomly selected, and the overall effect looks like it might be middle English, or even german in places. The randomness means that sometimes it doesn’t get the line breaks in approximately the right place. Nonetheless a very interesting result, and it doesn’t loop.
I love the way it puts the sonnet number in the right place!
Seed:
” nds to the course of alt’ring things:
alas why fearing of time’s tyranny,
might i not then say ‘ ”
to sof bn bde,
the iroelge gatesds bever neagne brmions
ph puspper stais, delcs tien love ahena,
thich thi derter worndsm hin fafn’sianlu thee:
shese blunq so me for they fadr mnhit creet.
64
cscv thy swbiss io ht the yanjes sast,
mftt pwr thet thai, io mengdvr to mehpt
nadengoes’dflret, acseriog of shein puonl.
to slobn of wourt)s fron shy fort siou mavt dr chotert fold (mn heart oo shoedh stcete,
that hil bu toun fass rrunhng of aeteinen
rie trilg mf prinlenlss, and ge voids,
batse mi tey betine out le il your wou,
these own taref in formuiers wien,io hise)
h eaar nonlhng uhe wari her bfsriite,
ming oat,s al she wantero wo me.
96
theteeo i fave shee wout fnler fiselgct
sreanind,
whthsu doom that brss nn len; a
tekl of me
tr pfngslcs,tien gojeses tore dothen,
o beau aal wierefe, oo ttomnaei ofs.
au in doace fiasss eireen thae despered,
thv urut ninyak wtaprr’ and thereoo dlg hns
cooh,
afaonyt o
PS this is just to test it out – I really want to generate synthetic time-series data, but I found that just predicting the next value then running generatively always produced a decay to a constant value.
PS – having cut pasted it the pasting on this blog puts the sonnet number in the wrong place at the beginning of the line. In the generated text, the sonnet number is centred, ie preceded by a number of spaces, as in the original.
Very nice, thanks for sharing.
Perhaps you can use a beam search to better sample the output probabilities and get a sequence that maximizes the likelihood.
Sounds like a good idea, and also applicable to my goal – to generate synthetic sensor data.
Glad to hear it Iain.
Hey Jason,
Thanks for such a nice effort in deep learning stuff.
I am trying to run your code on my machine and it throws me this hdf5 error:
File “C:/Users/CPL-Admin/PycharmProjects/Tensor/KerasCNN.py”, line 59, in
model.load_weights(filename)
File “C:\Users\CPL-Admin\AppData\Local\Programs\Python\Python36\lib\site-packages\keras\models.py”, line 707, in load_weights
f = h5py.File(filepath, mode=’r’)
File “C:\Users\CPL-Admin\AppData\Local\Programs\Python\Python36\lib\site-packages\h5py\_hl\files.py”, line 269, in __init__
fid = make_fid(name, mode, userblock_size, fapl, swmr=swmr)
File “C:\Users\CPL-Admin\AppData\Local\Programs\Python\Python36\lib\site-packages\h5py\_hl\files.py”, line 99, in make_fid
fid = h5f.open(name, flags, fapl=fapl)
File “h5py\_objects.pyx”, line 54, in h5py._objects.with_phil.wrapper
File “h5py\_objects.pyx”, line 55, in h5py._objects.with_phil.wrapper
File “h5py\h5f.pyx”, line 78, in h5py.h5f.open
OSError: Unable to open file (unable to open file: name = ‘weights-improvement-47-1.2219-bigger.hdf5’, errno = 2, error message = ‘No such file or directory’, flags = 0, o_flags = 0)
It looks like the file or path does not exist.
Perhaps check that the file with that name exists in the current working directory.
Thanks for the interesting post. I am getting the exact same errors as Vibhu. When you say working directory do you mean the one in which the code resides? In packages?
The directory where you are working, where you have saved your code file.
Hello.
I’m currently trying to convert your process, using characters as an unit of information, to a system where I’m using the words as an unit of information. Currently dabbling in the domain of NN, I’m trying to figure out why I would get a repeated sequence of words without any change, and I may have some clues:
– Too much classes? With letters we have arond 30 or so classes (as we have the alphabet + the residual tick/backtick). Does it have really an impact during learning so generation can give this kind of repetitive result?
+ A few notions from the Markov chain is staying with me and I have a supposition why the system repeats in such cases : maybe the link between words isn’t pronounced enough fror the generator to choose anything else than what was provided to it?
– Wrong parameters? I’m trying multiple settings for the learning process, such as changing seq_length to 10 or 50 or something else and/or changing the batch size?
– Corpus too small? Should I grab other books?
– Training too short? Is 60 epochs a relatively small or important processus?
– Note : I’m currently using a set to remove repeated sequences once the text tokenized . It really helps is seq_len is small, I think it could also help for character-based processing. That’s additional init overhead, but I think it might worth it.
I copied the code into this gist to avoid cluttering any further the comment log : https://gist.github.com/anonymous/28f30611bb0849ef0d99fd341e6e1d7b
Thanks for the article, it was pretty interesting and made me learn quite a bit on notions I forgot or didn’t understand well. I look forward to learn more and understand how to progress!
Have a nice day!
Great work, I recommend testing each hypothesis.
Instead of letters, can we map each individual word to a number? Then we can use a few words worth of context to predict the next word.
So we could have [“I”,”am”,”a”] as the input and have [“human”] as the output.
I’m still learning a few concepts, so I might be wrong.
Absolutely!
book text link is dead. new location seems to be: http://www.gutenberg.org/files/11/11-0.txt
nevermind, i’m totally wrong. please delete 🙂
You may have to visit the link twice to set/use the cookie.
Just wanted to say you are an amazing human who is enabling tons of people to learn complex material that otherwise might not be able to. I’m also astounded with your dedication in responding to literally every single comment, and within a short time too. You’re making the world a better place.
Thanks Ari, I appreciate your support and recognition!
Hi,Jason, Thank you so much for the great post!
I ran the exact same code of your small LSTM network on the same book(wonderland.txt)
for 20 epochs.
But I get an error with this line:
“model.add(LSTM(256, input_shape=(X.shape[1], X.shape[2]))) ”
The error:
“TypeError: Expected int32, got list containing Tensors of type ‘_Message’ instead.”
Could you please give me some insights on why this is happening and how to fix it?
Thank you very much!
Tensorflow: 0.10.0rc0
Keras: 2.1.1
Sorry, I have not seen this error before.
Perhaps double check that you have copied all of the code from the example exactly?
Hello Jason,
I have been looking at examples of LSTM text generation for some time and I have the following question:
Once the network has been trained and all the weights have been fixed at some acceptable values, would the network generate different text every time it is fed the same input seed text (“What would happen” , for example), or would the output text sequence always be the same for that exact input seed because the next character with the highest probability after the sequence “What would happen” would always be predicted to be the same on each run due to the network’s fixed weights, and then the next one after appending the previously predicted character to the input as well, etc.?
I am working on a similar LSTM network in tensorflow for a sequence-labeling problem, and so far it appears that my generated output sequence is always exactly the same for a fixed starting input. Is there a way to generate different output sequences for the same input seed?
Once the network is trained, it will make deterministic predictions.
Thank you for the answer. I suppose what I was describing was a stochastic Neural Network.
Hey Jason
May I ask why you used a normalized X vector as input and not just onehot-encoded input? Is this done by someone else as well? Just curious because I don’t really see an advantage over onehot encoding.
I would recommend one hot encoding instead these days.
Can you put your pretrained weights?
Yes, you can use pre-trained weights.
Shouldn’t we use word2vec instead of one-hot encoding?
You can, try it and see.
Hi Jason,
thanks for this great post. Do you have experience in using the LSTM as a model for Genetic Programming to further improve the output? Or have you ever heard about it? It would then be something like an EDA-GP. If you know anything about it, i’d be happy if you let me know!
Many thanks in advance!
David
I’ve not heard about using LSTMs with GPs. Interesting idea.
What makes you want to explore this combination?
Oh I was just wondering. I’ve recently seen some other text generation work, they implemented it quite similarly as you did but came to the conclusion that in future this could probably be improved by using the LSTM as a model for a genetic program, to add further constraints and thus to improve the output. I just wanted to know if something like this has already been implemented. Yesterday I came across a really interesting paper. (https://arxiv.org/pdf/1602.07776.pdf) They invented a new method: Recurrent Neural Network Grammars (RNNGs). They report major improvements both in language modeling as also in parsing. I will maybe try this out, as a topic for my master thesis.
Thanks for you prompt reply!
Best regards and happy new year!
David
Looks interesting, thanks for sharing.
Hi Jason, thank you for your great site and all the material that you offer.
I’ve been trying to modify the LSTM to predict words instead of letters. I stripped all special characters and numbers from the text and vectorized the words. It’s 26386 words in total and 2771 distinct words. The model could be trained, but it always and only predicts the word “the” as next word. I tried different sequence lengths with the simple and the complex model, but the result stays the same. Of “the” is the most frequent word with 1600 occurences, but shouldn’t the model still be able to predict something else? Or is the corpus just too small? What do you think?
This post has an example of a word-based language generator:
https://machinelearningmastery.com/develop-word-based-neural-language-models-python-keras/
Hi, Thanks for good contents, I have a question.
Is it possible to make similar sentence with RNN?
For example, seed sentence : “The rabbit-hole went straight on like a tunnel for some way”
generating several similar sentences :
” The rabbit is go straight on like a tunnel”
” The rabbit hole went straight”
“The rabbit straight for some way”
I don’t focus the semantic for sentences, just need to make training data with tagging to solve other text domain problem
Yes, generate multiple times, or generate once and use beam search to read off multiple output lines.
This tutorial was a great help to me. Thanks for this. But I have a question regarding deep learning. As Deep Neural Networks take large amounts of time to train how can I tune different hyper-parameters of the model easily?
You can use multiple computers in the cloud in parallel to perform parameter tuning.
You could also try tuning using a smaller amount of data that requires less time to train. But this may impact the quality of the results.
Thanks, Jason.
Hi Jason, Thank you so much for this blog. It was very easy to understand, and really helped consolidate the theoretical aspects. I’ve just recently gotten to RNN’s and quite surprised how effective they are. It seems they are used for musical note generations as well.
I was reading through your improvement section, and I don’t quite understand what you mean by this:
‘Train the model on padded sentences rather than random sequences of characters.’
Do you mean to split the text into sentences, and pad each sentence with zeros to match the max length sentence?
Yes, exactly.
As you have used 100 characters to predict the next one, I wanted to know is there any method that I can use to remove this restriction. I want the user to input any character length sentence and generate sentences using it. Thanks.
Is there any other way instead of padding the sentences? I really do not want to do this.
You can pad and then use a mask to ignore the padding.
You can also change the model to operate on one time step at a time and manually reset state.
Sure, you have configure it anyway you like. The model will need to tuned for your specific framing.
Hi, Jason. Thank you for your nice blog.
By the way, when I run this code, I got a ValueError message.
It says “ValueError: You are trying to load a weight file containing 3 layers into a model with 2 layers.”
And I thought that I run this code exactly same as yours, but I got a message like that.
Did I doing something wrong??
Never mind. I did it wrong. The problem is solved.
I’m glad to hear that.
Sorry to hear that, I have not seen this error. Perhaps the API has changed?
How did you fix it?
Hello Jason,
When you transform input sequences into the form [samples, time steps, features]
using X = numpy.reshape(dataX, (n_patterns, seq_length, 1)) , why is the features equal to 1? I was thinking it’d be equal to the num of chars
In that we are providing a sequence of integers.
When training on my own data, I notice after around 25 epochs the loss starts to increase. I’ve tried adding a batchnorm layer but it doesn’t do much. Any idea?
It could be one of 100 things. See this post for some ideas:
https://machinelearningmastery.com/improve-deep-learning-performance/
Hi Jason
Great article and I am a beginner in Neural Nets. I exactly implemented your code. Ran it for 20 epochs. However while printing the next predicted character it just repeats the same character again and again. I printed out the prediction matrix (before the argmax line) and checked and the prediction matrix is having the same order of values for every prediction. What do you think is going wrong
[[ 1.91119227e-08 1.16459273e-01 1.62738379e-05 2.84793477e-19
3.71392664e-26 1.29606026e-25 8.98123726e-28 1.22408485e-12
4.26335023e-27 1.49551446e-13 6.07275735e-24 7.06751049e-01
1.82651810e-10 4.56609821e-04 7.45972931e-19 1.12589063e-25
8.50236564e-26 4.02262855e-25 4.94167675e-24 2.13961749e-23
1.36306586e-25 2.24255431e-25 1.49555098e-25 7.40160183e-24
4.36129492e-25 2.62904668e-05 6.99173128e-08 1.21143455e-06
4.55503128e-20 1.59668721e-27 2.71070909e-24 9.95265099e-24
1.40039655e-11 2.50412119e-15 8.04228073e-11 5.80116819e-07
1.76183641e-01 7.31929057e-13 4.60703950e-06 1.45222771e-06
1.41010821e-06 8.60679574e-17 5.74646819e-09 3.02204597e-07
8.52235157e-11 7.89179467e-05 1.59914478e-07 3.00487274e-10
1.23463905e-19 5.40685824e-06 5.15879286e-08 5.95685590e-09
1.24319504e-05 5.76499569e-14 1.03425171e-14 1.20372456e-15
7.63502825e-08 6.09846451e-10]]
11
,[[ 1.80287671e-07 2.36380938e-02 2.51635304e-03 4.06853066e-14
1.85676736e-18 1.52850751e-17 6.71450574e-21 5.60100522e-10
1.99784797e-20 1.19577592e-09 7.35863182e-18 9.02304709e-01
4.51892888e-08 1.19447969e-02 2.06239065e-13 9.34988509e-19
2.99471357e-19 3.93370166e-18 9.95959604e-17 1.55780542e-16
3.49815963e-18 8.74736384e-18 1.30014977e-18 8.46453788e-17
5.59418112e-18 3.94404633e-03 1.59909483e-04 5.36000647e-04
8.32731372e-14 2.82467025e-20 2.60687211e-17 3.69919471e-17
2.15683293e-09 3.75411101e-13 1.86202476e-09 2.00714544e-06
5.37619703e-02 6.46848131e-10 4.58007389e-06 1.08297354e-05
1.26086352e-05 1.41199541e-12 3.86868749e-07 4.80629433e-06
1.67563452e-09 4.32701403e-04 3.21613561e-06 2.57872514e-08
1.72215827e-15 7.51974294e-05 1.74515321e-07 1.23122863e-08
6.45807479e-04 5.92429439e-11 6.11113677e-12 5.76062505e-12
8.02828595e-07 6.99018585e-07]]
11
The algorithm is stochastic so it may get different results each time it is run.
Perhaps try fitting the model a few times?
Hi Jason,
I executed the code. Building the model was not a problem. But using the model I hve an error:
ValueError: Dimension 1 in both shapes must be equal, but are 60 and 47 for ‘Assign_5’ (op: ‘Assign’) with input shapes: [256,60], [256,47].
The error is generated at the line of code:
# load the network weights
filename = “weights-improvement-20-1.9161.hdf5”
model.load_weights(filename)
model.compile(loss=’categorical_crossentropy’, optimizer=’adam’)
Of course weights-improvement-20-1.9161.hdf5 is my file.
Perhaps test making predictions prior to saving and then use the same code after loading so that you know it works.
This was caused by the weights.hdf5 file being incompatible with the new data in the repository. I have updated the repo and it should work now.
index = numpy.argmax(prediction) only output the maximum value 1. When it converts the index value to char ie.., int_to_char[index] in the dictionary int_to_char the key for 1 is the newline. How should I overcome this?
working on a smaller dataset cause all this problem.When I trained on larger dataset it actually starts to produce the text. Thanks, @Jason for this article 🙂
Nice work!
Perhaps the model requires further tuning on your problem?
Why didnt you assign batch size(64) same as sequence size(100)?
I still confuse how sequence and batch work when they dont match each other.
One batch is comprised of many sequences.
One sequence is one sample or list of time steps.
Does that help?
Your posts, and your attentive responses to comments are amazing. Thanks for that.
I’ve had some success training a model using words instead of characters. I think it would be interesting to augment each word with synthetic features (parts-of-speech, for instance). But, I can’t wrap my head around how to do this properly? In my mind, I feel that there would need to be a second sparse array with POS-tagging. And perhaps this variable is given a weight of some sort. Does this make sense? Is this possible with the Keras LSTM models?
Yes, that makes sense. The input would be a mess and hard to keep straight though.
It might be easier to separate the streams and have a multi-input model instead. Just and idea.
https://machinelearningmastery.com/keras-functional-api-deep-learning/
If you go down this road, I’d love to hear how you go.
Thanks for the response. I’ll work on this and will definitely share the results. I have some other speculative features that I want to experiment with as well.
Let me know how you go.
Hi Jason,
If we have 1 million words to predict, shall we still use one hot encoding and softmax in output layer? It might cause memory problem. Is there any way to solve this problem.
Thanks,
Ray
Good question.
I have seen some papers that look at splitting up large one hot encoded vectors into multiple pieces. Perhaps try searching on google scholar?
I am not sure how this code will work?
seq_length = 100
n_patterns = len(dataX)
print “Total Patterns: “, n_patterns
# reshape X to be [samples, time steps, features]
X = numpy.reshape(dataX, (n_patterns, seq_length, 1))
Should this not give an error?
You can learn more about lists and reshaping here:
https://machinelearningmastery.com/index-slice-reshape-numpy-arrays-machine-learning-python/
sir,thanks for the awesome tutorials,these tutorials are really helpful………
sir what is the procedure for generating a sentance from a set of keywords……..i am having the following data…..
“no_result, sorry, suggest”
from this i need to generate a sentence as following
“Hi…I checked a few options for you, and unfortunately, we do not currently have any trips that meet this criteria. Would you like to book an alternate travel option?”
thanks
Good question, I have not worked on this type of problem. Perhaps survey the literature to see what your options are?
How and where to change the “temperature”, i.e., scale factors?
What do you mean exactly?
Hello,
I am currently working on a project. The idea is to generate the description of a product for example from characteristics and keywords.
My learning base is a set of product descriptions.
I would like to give my model some characteristics, key-words and that it generates me a description from it.
For example :
Characteristics: “Fridge, Bosh, American, Stainless steel, 2 drawers, 531L, 2 vegetable trays”
Generation: “Our experts have selected the BOSH fridge for you: an American stainless steel fridge that keeps all its promises. This product has a total usable volume of 531L, which is very important. With 2 drawers and 2 vegetable trays, it will allow you to store a maximum of fresh produce.”
Wow, great problem!
The first step would be to prepare thousands of examples, somehow.
Hello!
I am trying to do something similar, did you find a way to do this?
Would it be possible to use a Seq2Seq model for this?
It is really great that you take time to reply to each question. Something extremely unusual in today’s world.
Thanks.
Hi Jason!
How come you did not use any validation or test set? Will it be a valid argument to not use any validation or test set as I only want to generate text based on the training data alone? say, i am trying to generate a text based on all harry potter books.. I only want to generate text, words, phrases, sentences based on what my model has learnt and known, since validation and test sets are just there to validate that your trained model can classify and predict what is unknown, it will be of no use already?
It is a challenge to test the generative model that is supposed to generate new/different but similar output sequences.
Awesome tutorials sir.Can I know what is x in making the prediction??.what do we input during prediction
It depends on how the model was defined, e.g. what the model expects as input.
In this tutorial, it expects a seed sequence of 100 words.
Nice tutorial. With one hot encoding for the input sequences, one can get a loss of 1.22 in less than 10 epochs.
Nice!
Btw, mainly for the other readers, code to do this is quite simple. just replace lines:
X = numpy.reshape(dataX, (n_patterns, seq_length, 1))
X = X / float(n_vocab)
with:
X = [e for lst in dataX for e in lst]
X = np_utils.to_categorical(X)
enc_length = len(X[0])
X = numpy.reshape(X, (n_patterns, seq_length, enc_length))
Don’t forget to also do this in the text generation part, using enc_length as the second parameter when generating one hot encodings for the seeds.
Also, it’s not compulsory to get seeds from the actual data. You can construct seed/pattern like this:
in_phrase = ‘her name was ‘
in_phrase = [char_to_int[c] for c in in_phrase]
pattern = list(np.ones(100 – len(in_phrase)).astype(int)) + in_phrase
Again, this was/is a really fun and straightforward example to work with. Thanks Jason.
Nice, thanks for sharing!
Thank you for this nice tutorial. I didn’t really get why you used one hot encoding only for the output character? why for example you didn’t use integer encoding for the output pattern, it will calculate also the output probability for it if it was encoded as the input.
An integer encoding was used for the inputs and passed directly to the LSTM.
I have another question, Can we use here also word2vec instead of converting the characters to integers and then scaling. Or it won’t be character to character model anymore ?
Perhaps, I have not seen embedding models for chars, but I bet it has been tried.
Hello Jason,
I have adapted your code in kaggle.com to try and fit project gutenberg txt files on Shakespeare’s plays mentioning you and this website.
Hanks, well done!
Hello Sir, thank you for the post!
I have created this model and trained it on a different data set. The results contains sequences repeating infinitely in a loop. Can you please share some insights?
Perhaps the model is over fit?
Perhaps try fitting the model again?
When I tried running the final complete code it shows an error saying im trying to load a weight file containing 2 layers into a model with 3 layers.
I have not seen this, are you sure you copied all of the code without modification?
Are you sure you have the lates version of the libraries installed?
int to char is trowing eerror
i have encoded file with utf 8
I have some suggestions here:
https://machinelearningmastery.com/faq/single-faq/why-does-the-code-in-the-tutorial-not-work-for-me
result = int_to_char[index] i am getting error
here when rinning final code in
i read file like this
raw_text = open(filename,encoding=’utf-8′).read()
Are you able to confirm that all of your libraries are up to date and that you copied all of the code from the tutorial?
Yes jason but errror at result line which converts to char 3425
Hello, Sir. This is not about this post, but your posting about RNN. I read your post about RNN and how the weight. So, how we can get the WHy matrix? for WXy matrik, we initiate it. But for WHy matrix i don’t understand. Can you help me, please?
What is “WXy?
How did you settle on a 256×256 hidden layer?
I ask because I’m interested in not paring out caps, and the vocab in what I’m learning on has expanded to 132 characters. In doing so I’ve gotten the loss down to 1.3, and the generative text is still producing a *LOT* of typos.
If I added more neurons to the LSTM layers, could the bot improve? How many neurons?
Or would it be more beneficial to allow the network to train to an even lower loss on the current 256×256 network?
Trial and error.
I recommend testing a suite of configurations to see what works best for your specific problem, more here:
https://machinelearningmastery.com/faq/single-faq/how-many-layers-and-nodes-do-i-need-in-my-neural-network
Hi Jason,
As you mentioned, we can also experiment with other ASCII data, such as computer source code.
So I need to use this post to create a program repair model.
The input will be a buggy code and the output will be the fixed code.
I have some vulnerable/buggy examples and their fixes but I don’t know how to generate a dataset and make a train for that.
Please help.
Thanks
Sounds like a cool project.
Sorry, i don’t have examples of repairing bugging code with LSTMs, perhaps in the future.
Hi. Thanks for this post. I have one question. How come you aren’t providing the output labels of every timestep? For example when the input is ‘HelloWorl’, then the output is ‘elloWorld’, if we are using 9 timesteps. For your example, there is only one letter for each sample. How are you going to provide the corresponding outputs for the timesteps then during training?
Thanks
I’m not sure I follow, sorry?
hey jason, check out a blog post i made that leverages some of you methodology!
this post is awesome!
http://overslant.com/2018/07/22/deep-nba-nicknames/
Thanks.
Hi Jason,
Thank you for the nice article. It made many things easy for me… haha.
I have one question though. I don’t quite understand the necessity of line 65 in the full code listing of the “Generating text with and LSTM Network” section:
seq_in = [int_to_char[value] for value in pattern]
seq_in
is set but doesn’t seemed to be used.If it is not used, you can ignore it, delete the line.
HI…Jason
Please give me information a baut specification minimum ( ram, processor, etc) a laptop for running 3D-Unet-Pytorch for classification images 2D/3D.and can i try MINST data set for 3D images.
Thanks.
Regards,,
Verdy
You can train on your CPU or use AWS if you need to access GPUs.
I explain how here:
https://machinelearningmastery.com/develop-evaluate-large-deep-learning-models-keras-amazon-web-services/
With same as your code I trained model but during testings I got this…
ValueError: You are trying to load a weight file containing 1 layers into a model with 2 layers.
Any idea what’s the problem?
I have some suggestions here:
https://machinelearningmastery.com/faq/single-faq/why-does-the-code-in-the-tutorial-not-work-for-me
shoudnt the input sequnce and lstm size be tha same, eg. here since sample input is 100 characters lstm should be 100 as well instead of 256
The number of nodes in the first hidden layer is unrelated to the number of units (defined by input_shape).
how did you decide the number of hidden units? ,can we take 128 instead of 256
Trial and error.
Learn more here:
https://machinelearningmastery.com/faq/single-faq/how-many-layers-and-nodes-do-i-need-in-my-neural-network
Hello Jason, great tutorial, as always!!
I tried running this however on Kaggle, the training went well, got a good saved weights. However, during generation, I ran into the error and I can’t seem to identify what is causing it:
“—————————————————————————
KeyError Traceback (most recent call last)
in ()
79 prediction = model.predict(X, verbose=0)
80 index = numpy.argmax(prediction)
—> 81 result = int_to_char[index]
82 seq_in = [int_to_char[value] for value in pattern]
83 sys.stdout.write(result)
KeyError: 2463279”
Sorry to hear that, I have some suggestions here:
https://machinelearningmastery.com/faq/single-faq/why-does-the-code-in-the-tutorial-not-work-for-me
Hi Jason,
Great Post!
Just curious to know the possibilities of implementing Sequence mining use case here.
Can we use Generative LSTM Networks for sequence mining? This is to suppress the events.
I have a dataset with 2 million sequence of events, where we have root events and child events. We have to suppress all the child events based on root event presence. This pattern needs to captured by Generative LSTM model which is trained on 70% of the whole data. And then apply this trained model on test data (30%) to perform event suppression to dedue the whole number of events.
Thanks,
Uday Vakalapudi
Sorry, I don’t know what “sequence mining” is?
Great post. It inspire me to think about the application of the solution to my problem. So, let see what do you think about LSTM capabilities to predict next value in the array like this: [5.3, 2.2, 3.1, 8.33, 2.32, 2.01, 4, 12.2, 12.2, 4, 4, 3, 2.30, 13.30…]? It is like the sentences if you look from this perspective:
– first three numbers are connected like this: first number is the sum of second and third;
– forth number isn’t connected with the previous and it represented the sum of the next three numbers;
…
General conclusion about array: there is a groups of numbers they are connected with the mathematical operations of subtraction and additions but there is no connection between groups except that they represented the journal entries in a bookeeping.
Thank you on your help
It may be possible, perhaps try it and see.
I got this error when using your code, any help or advice you could give me?
https://i.gyazo.com/69b94f1f42990146b27050dd2459a3f3.png
You must change the filename to the file in the code that you saved.
I just might not have seen the part, but could you please tell me what file that would be? I didn’t see a part about saving a file.
Thank you, i accidentally missed a part, have a good day and thank you for the help
No problem.
I am new here,can i use this model to train a text time-based collection of documents and then predict the documents that will be generated at a future time point.
Perhaps, you may have to experiment a little to discover a suitable model.
Can you recommend some papers or documents that have done this to me ? Thank you Jason .
No sorry, perhaps searching scholar.google.com
can you recommend some papers about this that use LSTM and LDA to predict the Technology topic Trend, I’m stuck here.Thanks very much
Hi Jason, I follow your posts and they are absolutely great. But I am trying to generate text using tensorFlow.
I have trained my model using tensorflow’s original RNN code; https://www.tensorflow.org/tutorials/sequences/recurrent#tutorial_files; but not sure how to predict and test my model. Looking forward to hear from you.
Thanks for the great work!
Sorry, I don’t have TensorFlow tutorials, I focus on Keras that runs on top of TensorFlow.
Thanks!
You’re welcome.
Hi Jason thank you for this great tutorial.
You’re welcome, I’m glad it helped.
Hi,
In this tutorial, if you keep training until you get really good accuracy, say 99%, isn’t that just memorizing the data? The end goal is to generate something new right?
Yes, the idea is to have a dataset that is large enough or a model that is regularized enough that it cannot be memorized.
Hi Jason,
I have seen your tutorial and tried it.
I am now using a different project, where we want to launch an LSTM network in production.
In other words, we have a Time Series Prediction network, and we want to place it on AWS or Azure. We have also seen Tensorflow Serving as a way of putting these Networks online.
The only thing i’m afraid of is the performance of LSTM.
Let me elaborate:
When training an LSTM network, the Long- and Short-term memory is crucial. The weights get adapted as well, so they are important.
If we save the model – like you did in the tutorial – will we still get good results if we try to run a test-seed through it?
Some seed it has never seen before, like some kind of user-input? I’m afraid that it will not get great results, as the Hidden states are lost now, and the seed will make for new hidden states, that are completely different.
Or am I totally wrong with this?
Thank you in advance for taking your time to reply!
Perhaps try using an ensemble of final models to reduce the variance?
Perhaps design tests for the system before deploying it into production (e.g. good engineering practices).
Consider having a transform that converts a word (or a few words) to a binary vector, multi-hot.
Now, I want to convert back the binary vector to the original word (or words).
Can this be done using RNN/LSTM?
Of course, I can train the model using a train set (given the words and their corresponding binary vector), but, then, test it with a predicted binary vector, hopefully, to predict the correct words.
Yes, calculate the argmax() of each vector, then map the integer to the word in your vocab – a reverse lookup.
I believe I have examples in many tutorials of this.
Hi Jason ,
Is it possible to generate sequence using return_sequence=False ?
Meaning that only the last timestep is being predicted in train – how would a generation look like ?
Thanks
I’m not sure I understand your question, sorry, can you elaborate please?
You can frame any sequence prediction problem you wish and test whether an LSTM performs well or not.
In your example you use this line :
model.add(LSTM(256, input_shape=(X.shape[1], X.shape[2]), return_sequences=True))
As far as I understand , the return_sequences=True means that there will be an output in every item in the sequence (many-to-many) and Tx=Ty. and if return_sequences=False there will be only one output to the sequence (of the last) and only it will be accounted for in the Loss function.
So , can return_sequences=False be used in a generation-task such as this ?
Yes, but the results will be poor when making a prediction with the output of the LSTM layer directly. It is better to use one or more fully connected layers to interpret the output of the LSTM.
Hi jason,
Thank you for the informative tutorial.
I am on python 2.7, tensorflow 1.12, and keras 2.2.3
I am getting the following error:
maximum_iterations=input_length)
TypeError: while_loop() got an unexpected keyword argument ‘maximum_iterations’
ERROR:tensorflow:==================================
Object was never used (type ):
Sorry, I have not seen this error. Perhaps try searching/posting on stackoverflow?
Hi Jason,
Thank you for the tutorial.
I am repurposing this model to generate SMILES strings, which are character representations of drugs and molecules:
They look like this:
Nc1nc(Nc2ccc(cc2)S(N)(=O)=O)nn1C(=O)c1c(F)cccc1F
CO[C@H]1[C@H]
I have trained the model and saved the weights.
How do I now get the model to generate the SMILES strings with variable lengths.
Thanks,
John
Perhaps train the model on zero-padded but variable length content inputs that have a “end of string” char (e.g. like a period). Then generate new sequences and stop when that char is encountered.
Does that help?
Hi Jason,
Thank you for the advice, but what if it is important for the model to understand the end of the sequence or there is a specific pattern at the end.
Sorry, I don’t follow, what is the concern exactly?
Hey Jason Brownlee.
Thank you for the wonderful explanation about the text generation and also providing the code with point to point explanation.
You’re welcome, I’m glad it helped.
I’m running this model (the simple LSTM) but with what you’d call a huge dataset. A file of about 350 mb and this much info
muiruri_samuel@instance-1:~/rap-generator$ python new_model.py
Using TensorFlow backend.
(‘Total Characters: ‘, 307478020)
(‘Total Vocab: ‘, 177)
Killed
which btw I’m running on a google cloud instance with 120 GB of RAM but it exhausts it all.
Here’s the file so far https://bitbucket.org/muiruri_samuel/rap-generator/src/master/new_model.py
I’ve contemplated just training on a file with 1m lines of text and that’s become 10 smaller files of 30 mbs but I wonder is it possible to know how much the main file would need in RAM (lyrics.txt) and can I get it to work in a batch method either using the smaller files and a kind of fit_generator method.
You can estimate the RAM based on the number of chars and the choice of 8-bit or 16-bit encoding.
Perhaps progressive loading with a custom data generator would be the way to go?
Hi Jason,
This is a wonderful post, thanks for sharing. I have a different problem to solve and was wondering if it can be solved using your solution.
I have a corpus of numerical data (structured) and its corresponding article (readable text). i.e. A simple table with numbers in it and a paragraph explaining the table. How can this dataset be used to train the model and then used to generate paragraphs based on any input of the table form?
Sanjeev
Wow, great problem.
Try a model that might use an MLP, CNN or LSTM to read in the numbers, and then a decoder to output text – maybe one output for the table and one for the text.
Hello Jason,
Thank you for such an amazing post. I did a complete tensorflow version of the same.
It would be great if you can write some blogs on BERT and GPT.
Highly appreciate the work you’re doing for the AI community.
Regards,
LK
Great suggestions, thanks.
Hi Jason, Thanks for the tutorial.
Could you please shed me some light on how I should approach for the following problem:
I have a set of words. The word(s) might have spelling error(s).
From the set of words, I would like to generate a sentence.
The set of words might ( in most of the cases ) be in the order of the sentence formation, BUT there might be missing words also.
e.g: A dog is running behind a car. (ORIGINAL sentence)
word_set={‘a’, ‘dot’, ‘runni’, ‘ehin’, ‘ar’}
I am working on multiple NON English languages, where tools such as POS tagger, etc are NOT available.
For one language, I have some pretty good amount of corpus. And for one, very less amount of corpus.
The sentence that I have to generate using the set of words may or may not be present in the corpus.
(Any steps or tutorial guide will be helpful).
Thank you for your time.
I think some development and prototyping might be required – there’s no step-by-step tutorial for this.
Perhaps you can get some ideas from related text-correction papers?
I was think of spelling correction followed by text sequence generation. Anyway, I will look into it. Thanks!
Hello, I would like to know your opinion on why it is better to generate text predicting letter by letter and not word by word.
I’m trying to make a text generator in Spanish, with the little prince’s book, the results letter by letter and word by word are different but none I like.
Letter by letter I get sentences with more global sense, but with incorrect letters. But word by word I get incoherent sentences.
BR!
Great question.
I don’t know, perhaps you can design some experiments to help tease out the cause and effect.
Perhaps it has to do with the limited cardinality if the input/output (letters vs words).
hii great blog thanks for it
I have also implemented by referencing your blog. I had used shakespeare poem.
with 30 epochs 64 batch size I got “weights-improvement-30-1.4482.hdf5”
error loss 1.4482
I have one request can you write a blog on Recommendation system with RNN LSTM in keras
Once again thank you
Well done!
Thanks for the suggestion.
Hi Jason,
In all the LSTM text generative models I found online, there’s a text file and they’re predicting the next sequence. In my case, I’ve an input and output column in a csv file. For a particular input, I want the model to generate corresponding output. How do I approach this? and what are the pre-processing steps involved?
You can load the data into memory first, then work with it any way you wish.
I really didn’t get that. What I did was, I converted my input and output column to two individual text files, did character mapping for both of them individually, then taking X and y according to a sequence length. But couldn’t fit the model. I’m getting no.of input samples doesn’t match output samples.
Could you elaborate the steps that have to be done. Again, I have an input text column and dependent output text column. Based on an input sequence, the model has to generate output column’s text
Perhaps start with the code in the tutorial and slowly modify it work with your dataset?
Hi Jason. Nice post on text generation.
I have few queries regarding my ongoing project:
How do I parse/process the structured data(dataframe format) for text generation, where-in I can the output text in a form of sentences. Eg.: Lets say I have Financial data in csv/excel format and once I load it in NLP, I should get the insights of data in the form of narratives/sentences.
I know this is more of the NLG task, but I am not able to set a pipeline right from parsing the data to generating narratives.
Please help regarding this or else at least few tips will be okay as well to start with my project.
I’m not sure off hand, experimentation will be required.
Thanks Jason this is an awesome post! I modified my code to treat the characters as actual words as my base dataset is extremely small ~200 sentences. I plan on posting my example on Github. Is there a specific way you like to be cited?
Thanks!
I am interested to try this example out. You mentioned that with GPU it takes about 700 seconds per epoch for the 2-hidden-layered-LSTM. How many seconds (or hours) would it take for Intel i5 CPU, if you could give some raw estimate?
I don’t know, sorry. Perhaps test it and find out?
hey jason,
why are you using the seed sent (to test the model)extracted from the exact same text(corpus) that you have used to train/fit your model? isn’t it obvious that the model you have trained on the corpus will generate the same output during testing if it sees the same sequence of char in its training data!!! the real test should be using a sentence different from the corpus OR it does not matter that much?!?!?
It does not matter much.
thanks for this code sir
due to your tutorial i have learned many think for neural network
You’re welcome. I’m happy to hear that.
Thanks for the easy-to-understand post.
1 question though : One improvement suggested was add dropout to the visible input layer.
Does it mean the parameter dropout to LSTM function?
Before the first LSTM layer, e.g. dropout as the first hidden layer layer.
Thanks Jason for your quick response. But I did not see any improvement on adding Dropout before LSTM layer.
In your code, input is a sliding window of 100 chars and output is 101st char.
Now for training the model on padded sentences, I have converted the input into padded sentences of 100 words. But how do I model the input and output? For each sentence of 100 words, the output is the101st word. Any better way?
You might have to get creative and explore a range of ideas to see what works well/best.
The trouble here is this : to explore even 1 idea, takes min 50 epochs to see its proof, with each epoch ~12mins. So was asking if you have any experience.
Thanks again for the great post and your prompt responses.
Opinions are not helpful because models and data can vary so widely, I would encourage you to experiment.
Perhaps you can reduce the size of the dataset or model to increase the rate of testing ideas?
sir in spyder it is showing that
Unable to open file (unable to open file: name = ‘weights-improvement-19-1.9435.hdf5’, errno = 2, error message = ‘No such file or directory’, flags = 0, o_flags = 0)
how to solve this problem
@jasonBrownlee can we ask the model to generate a sentence based on few input keywords given, instead of random seeded sentence it generated
That would be a different problem, e.g. generate a sequence from keywords.
It would be like the reverse of text summarization.
Jason Brother it is prinitng empty letters Any suggestions ?
Perhaps try fitting the model again?
Hi, very nice website!
I am trying to generate swim practices programs from learning all the swim practice that I did. I think this model would apply. Do you think It could be adapted? I have a basic setup now that is giving me some results, but I would like to add more data to each exercise, like time. Do you think I am in the right path ?
I don’t know sorry. Perhaps experiment and see what you can achieve?
Sir, how do I determine the correct batch size?
Great question, I recommend testing a range of different batch sizes to see what works well on your specific problem.
See this post:
https://machinelearningmastery.com/how-to-control-the-speed-and-stability-of-training-neural-networks-with-gradient-descent-batch-size/
Hi Jason,
I have tried to run the 2nd exercise using 50 epochs, but in my PC it simple does not finish, it crash after 2 hrs. Even I tried in a AWS machine (ml.m4.xlarge) but it hangs also.
Could you please help me with two things?
1.- In which type of machine I need to run the exercises? Is it mandatory to have one with a GPU?
2.- Could you please share the “weights-improvement-47-1.2219-bigger.hdf5” you obtained, then I can use it to generate text.
Thanks in advance and regards,
No GPU is required, but it can help.
Sorry, I cannot share trained models.
Hi Jason,
Could you please explain to me what weights are updated after the loss function is calculated? In MLP for example, the weights and bias of the Input layer were updated at the end network calculation, but in case of LSTM, which weights are re-calculated if we have “inner” cells.
Thanks in advance,
Yes, this is backpropagation through time. You can learn more about it in general here:
https://machinelearningmastery.com/gentle-introduction-backpropagation-time/
Thank you Jason,
Another question please 🙂
My laptop is not able to run all the epochs, so, is there a way to load the last hdf5 file and continue from there training the model? Like loading the file generated of epoch 10th of 20.
Thanks in advance,
Yes, you can save the model, then load it later and continue training.
This will help:
https://machinelearningmastery.com/save-load-keras-deep-learning-models/
can i use the same step and same code for another language other than English?
I don’t see why not.
Hi, i want to make Table-to-Text Generation by Seq2seq Learning, how can i represent the table numeric data with the sequences?
Perhaps row by row or column by column?
Perhaps brainstorm and test each framing of the problem?
Given data about the weather for example like temperature, humidity, wind speed, …..etc, and the corresponding texts that describes each instance.
To construct the model, text data is converted into list of input sequences with fixed length L and one word predictions.
Can we repeat the numeric data with corresponding sequences to represent input to the model?
For example the numeric data is 37, 40, 20, and the corresponding text is ‘hot and the humidity is moderate’ can be represented as:
X Y
37, 40, 20, hot ,-,- and
37, 40, 20, hot, and,- the
37, 40, 20, hot, and ,the humidity
37, 40, 20, and, the ,humidity is
37, 40, 20, the, humidity, is moderate
No, I recommend against this framing.
Hi Jason,
That’s a really interesting article. I read a few articles that use LSTM to predict punctuations. I am wondering how to do that? can you make a post about it
Thanks.
Perhaps a seq2seq, text in text with punctuation out.
Using TensorFlow backend.
Illegal instruction (core dumped)
Sounds like you might need to re-install tensorflow?
Hi Jason,
Interesting post, I tried your code but I have and error with the reshape of dataX
(ValueError: cannot reshape array of size 167418 into shape (167418,100,1))
the code as following,
import gzip
import urllib
dataurl=”http://www.gutenberg.org/cache/epub/11/pg11.txt”
urllib.request.urlretrieve(dataurl, “wonderland.txt.gz”)
with gzip.open(‘wonderland.txt.gz’) as f:
data=f.read()
chars = sorted(list(set(data)))
len_data = len(data)
print(‘Total Characters:’, len(data))
len_chars = len(chars)
print(‘Total Of Unique chars:’, len(chars))
#Converting the characters to integers
char_to_int = dict((c, i) for i, c in enumerate(chars))
SEQ_LEN = 100
STEP = 1
dataX = []
dataY = []
for i in range(0, len_data-SEQ_LEN, STEP):
input_chars = data[i:i+SEQ_LEN]
label_chars = data[i+SEQ_LEN]
dataX.append(char_to_int[char] for char in input_chars)
dataY.append(char_to_int[label_chars])
len_dataX = len(dataX)
print(‘Total Of dataX:’, len(dataX))
X = np.reshape(dataX, (len_dataX, SEQ_LEN, 1))
dataY = np_utils.to_categorical(dataY) # convert the target character into one hot encoding
I’m sorry to hear that, I have some suggestions here that might help:
https://machinelearningmastery.com/faq/single-faq/why-does-the-code-in-the-tutorial-not-work-for-me
i red your book and submit its codes and it is really amazing .now i want you to suggest me a book in deep learning which you think is useful in this field
Thanks.
You can see the full catalog of 17 books and book bundles here:
https://machinelearningmastery.com/products/
This is great! I’m working on a project with RNN (many to many) where input text sentence length is not equal to the output text length. Can you please help me with any examples on the same?
Thanks!
Yes, you can use an encoder-decoder model. I have many examples, start here:
https://machinelearningmastery.com/start-here/#nlp
Hi Jason. I followed your guide (the “Develop a Small LSTM Recurrent Neural Network” part) but instead of using single letters I used full words as my samples. I did that because I have also preparsed some test cases which I wanted to try out using model.predict(). The problem I have is that the network is always suggesting to put an interpunction (99% of the time). My training data are not bloated with interpunction only, however it’s true that interpunction appears often and after many different parts of speech which is not a surprise to us – humans. If I purposefully remove interpunction from my training data then the model will suggest me putting preposition in 95% cases. It looks just as the network was showing me the most used part of speech instead of guessing the correct one.
My training data sequence length is 10 (words) where 1-9 is a sample and 10th is output. I have roughly 500k patterns.
any idea what could be causing this?
Nice work.
Perhaps try some of the language modeling methods described here:
https://machinelearningmastery.com/start-here/#nlp
Hi Jason,
Thank you for
I have developed the same model (epoch=20) but model is prediction some repeated.
for example:
Input for the model is : “For some minutes the whole court was in confusion getting the Dormouse turned out”
Output from model is: “for some minutes the whole court was in confusion getting the dormouse turned off the terms the mock turtle said the mock turtle said the”
To overcome this-
Do i need to increase the data set and add mode layers and more neurons?
Please suggest.
I have tried increasing context window size but no luck.
Thanks,
Dinesh
This can happen.
Perhaps try fitting the model again?
Perhaps try tuning the model learning rate or capacity?
help me with this value error please
Input 0 is incompatible with layer lstm_13: expected ndim=3, found ndim=2
Perhaps post your code and error to stackoverflow.
Dear Jason,
This is the first time I have come to say to you how wonderful job you do for learners like us. The value of your work is beyond expression.
Coming to the point, i have started to work on seq2seq models. In this concern, i came across this post of yours like your other posts and books.
My question is, training an LSTM / GRU / any RNN, can be:
i) many to many : input sequence is S[ t : t+N] and output sequence is S[ t+1 : t+1+N], where S[ t+1+N ] is the new character predicted and produced at the last time step.
ii) many to one : exactly the one you mentioned in this post — input is S[ t : t+N ] and output is S[ t+N+1].
Regarding these two training procedures, i am quite confused about —
a) does the RNNs always pass on the hidden state to the next timestep of itself by default ?
b) what is the purpose of ‘stateful’ parameter in this context ?
c) is there any concept where i can update the initial state of an LSTM at every timestep ? If so, how do i code it ?
I am looking forward to your help.
Once again, thank you for all that you do.
Yes.
More on stateful:
https://machinelearningmastery.com/stateful-stateless-lstm-time-series-forecasting-python/
No need to update the state manually, the model takes care of it.
Hi Jason,
Looks like the link to text format of book “Alice in wonderland” is
http://www.gutenberg.org/files/11/11-0.txt
and not
http://www.gutenberg.org/cache/epub/11/pg11.txt
and thanks for the article 🙂
Regards
Amal
Thanks.
Someone, please tell me how to install NumPy. I tried everything!!!
This tutorial will show you how to setup your workstation, including installing numpy which is part of anaconda:
https://machinelearningmastery.com/setup-python-environment-machine-learning-deep-learning-anaconda/
(1) I wanted to know how we can extend this example to a many-to-many model or if you could link to any articles you have on this site that covers this.
(2)
In your full code for the first general model line on line 33 you have,
X = numpy.reshape(dataX, (n_patterns, seq_length, 1))
Does this 1 correspond to the number of characters you are predicting, i.e., does num_features = num_predictions as in CHAPT -> E?
What if we extended this amount to 2, i.e., CHAPT -> ER?
I tried to extend your code to adopt for these changes, such as this line.
seq_out = raw_text[i + seq_length: i + seq_length + 2]
But the problem is we can’t create categorical variables out of sequences because this results in “ValueError: setting an array element with a sequence.”
Source code: https://pastebin.com/dTu5GnZr
(3)
from keras.utils import to_categorical for the latest keras as of July 2020
The examples here might help:
https://machinelearningmastery.com/?s=language+model&post_type=post&submit=Search
The final dimension is the number of features, which is 1 because it is one sequence of characters. Learn more here:
https://machinelearningmastery.com/faq/single-faq/what-is-the-difference-between-samples-timesteps-and-features-for-lstm-input
Hi
Can I use LSTM for generating data curves for a specific material type? If yes. How ca I do this?
Perhaps, you may have to experiment to see if it is appropriate/viable.
Hi Mr. Brownlee,
Thanks for the great tutorial as always.
I’ve one question:
In your model, it learns one character given the input sequence:
CHAPTE->R
When I check the text generation tutorial in tensorflow documentation, there’s a mapping like below. Basically at each time step the input is the current character and the label is the next character. During prediction phase only the output of final time step is considered.
CHAPTE->HAPTER
Do you think one of them is better than other?
Yes, there are many different approaches.
Perhaps adopt the approach that best suits or works best for your specific application.
Thank u very much sir.
You’re welcome.
I learned a lot by looking at the material you posted.
But there is a problem.
In the last Larger LSTM Recurrent Neural Network part 0 due to variable shape (256, 58) and value shape (256, 44) are incompatible
It does not work because the data array does not match. Could you please let me know what data material is right for you or how to change it?
You can also reply by email to qwqw8919@gmail.com.
Perhaps these suggestions will help as a first step:
https://machinelearningmastery.com/faq/single-faq/what-is-the-difference-between-samples-timesteps-and-features-for-lstm-input
Hello Jason!
Amazing post!
Loved it!
I have been facing some issues while trying to execute it on my end.
I have a dataset of 9 GB. And while trying to run it on a super computer.
Python program in the shell runs out of memory.
Can you please suggest how I can use iterators / generators with this script to work with large datasets?
Thank you!
Perhaps try using progressive loading, e.g. don’t load all data into memory, just parts of it you need sequentially.
Please share the code for-
1.
How to use LSTM for morphological analysis
2. Word generation
Thanks for the suggestion. No existing code for these yet, however.
Hello Jason,
This was a useful post and I think it’s fairly easy to see in the other reviews, so this post is well written and useful. Keep up the good work.
Hi Jason, I was running the code provided here but got this error.
Unable to open file (unable to open file: name = ‘weights-improvement-47-1.2219-bigger.hdf5’, errno = 2, error message = ‘No such file or directory’, flags = 0, o_flags = 0).
What could be the issue ?. Sorry, If it’s too naive a question to ask but I am new to all this. Hoping for an early reply. Thank you.
Hi Adam…This error occurs when the filename path is not specified properly. When you download the text file, please take note where on your computer you saved it. The example below shows a path name being stored in the “filename” variable.
filename = “c:/temp/wonderland.txt”
raw_text = open(filename, ‘r’, encoding=’utf-8′).read()
raw_text = raw_text.lower()
Let me know if you have any further questions.
Regards,
this is very informative artical and comments also very interesting. thank you for this.
Thank you for the feedback and kind words. You are very welcome!
Hi Jason,
This code really helped me in my text generation project. However, I would like to save the generated output instead of printing it! Is there any method to save the generated text in another variable?
Hi Amulya…The following will hopefully be of interest to you:
https://www.geeksforgeeks.org/saving-text-json-and-csv-to-a-file-in-python/
https://en.wikibooks.org/wiki/Python_Programming/Variables_and_Strings
Hi and thank you very much for a super nice tutorial.
How can I change the Temperature of the Softmax activation function that you recommended as a possible extension?
Hi, thank you for the very clear and helpful tutorial.
I tried to make the same thing using an Italian text rather than English. I left all the hyperparameters like in your tutorial. Unexpectedly, the result was quite different: actually it produces a few almost correct words, and after that it repeats them untill the end of the generated text ( something like’ i don’t know i don’t know i don’t know i don’t know ….’). It is also strange that whatever seed I give, I was able to get just two of these obsessively sentences. I was able to mitigate this by using ‘temperature’ (dividing the input of softmax layer by T with T greater than one), but I wondered if this can be seen as a case of overfitting and also how I could solve it with better hyperparameters: may it help to add more units in LSTM layer?
Hi Guido…I would highly recommend adding more LSTMs units. Some other considerations are provided in the following resource:
https://www.analyticsvidhya.com/blog/2015/10/6-practices-enhance-performance-text-classification-model/
Hi Guido…Perhaps the following resource may help you decide among a few to try:
https://machinelearningmastery.com/impressive-applications-of-generative-adversarial-networks/
HI sir, I need to ask what exactly this RNN model doing, what is the main purpose of this model,
I cannot understand what makes difference in our final result, what we achieved in the end of this model.
Because i am training Swedish language sequence for training and testing through this model, but i am not happy with my results.
Hi Usama…You may find the following of interest:
https://www.techtarget.com/searchenterpriseai/definition/recurrent-neural-networks#:~:text=Recurrent%20neural%20networks%20recognize%20data's,activity%20in%20the%20human%20brain.
Hello, sorry if this has been asked before but…
Since there are checkpoints saved, I was wondering if there’s some way to stop the program and run it later starting where it was left the last time, because running the 20 epochs or more at once takes too many hours.
Thanks
Hi delgado…The following may be of interest to you:
https://machinelearningmastery.com/setting-breakpoints-and-exception-hooks-in-python/
Thank you very much James
Thank you so much for the article; it greatly helped with understanding the implementation of LSTM for text generation.
You are very welcome ChaLin! We appreciate your support!