The Fashion-MNIST clothing classification problem is a new standard dataset used in computer vision and deep learning.
Although the dataset is relatively simple, it can be used as the basis for learning and practicing how to develop, evaluate, and use deep convolutional neural networks for image classification from scratch. This includes how to develop a robust test harness for estimating the performance of the model, how to explore improvements to the model, and how to save the model and later load it to make predictions on new data.
In this tutorial, you will discover how to develop a convolutional neural network for clothing classification from scratch.
After completing this tutorial, you will know:
- How to develop a test harness to develop a robust evaluation of a model and establish a baseline of performance for a classification task.
- How to explore extensions to a baseline model to improve learning and model capacity.
- How to develop a finalized model, evaluate the performance of the final model, and use it to make predictions on new images.
Kick-start your project with my new book Deep Learning for Computer Vision, including step-by-step tutorials and the Python source code files for all examples.
Let’s get started.
- Update Jun/2019: Fixed minor bug where the model was defined outside of the CV loop. Updated results (thanks Aditya).
- Updated Oct/2019: Updated for Keras 2.3 and TensorFlow 2.0.
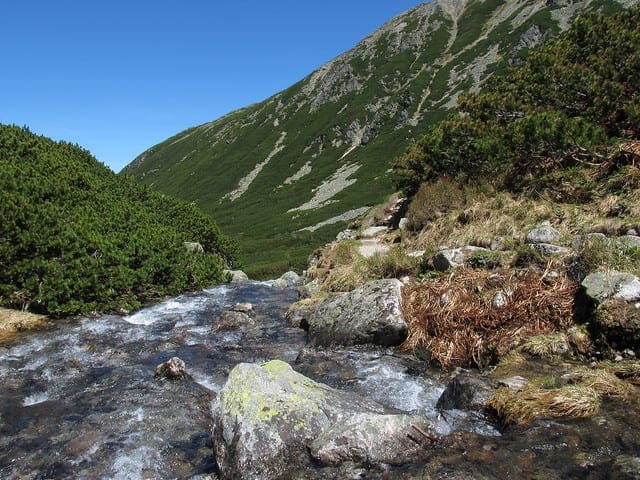
How to Develop a Deep Convolutional Neural Network From Scratch for Fashion MNIST Clothing Classification
Photo by Zdrovit Skurcz, some rights reserved.
Tutorial Overview
This tutorial is divided into five parts; they are:
- Fashion MNIST Clothing Classification
- Model Evaluation Methodology
- How to Develop a Baseline Model
- How to Develop an Improved Model
- How to Finalize the Model and Make Predictions
Want Results with Deep Learning for Computer Vision?
Take my free 7-day email crash course now (with sample code).
Click to sign-up and also get a free PDF Ebook version of the course.
Fashion MNIST Clothing Classification
The Fashion-MNIST dataset is proposed as a more challenging replacement dataset for the MNIST dataset.
It is a dataset comprised of 60,000 small square 28×28 pixel grayscale images of items of 10 types of clothing, such as shoes, t-shirts, dresses, and more. The mapping of all 0-9 integers to class labels is listed below.
- 0: T-shirt/top
- 1: Trouser
- 2: Pullover
- 3: Dress
- 4: Coat
- 5: Sandal
- 6: Shirt
- 7: Sneaker
- 8: Bag
- 9: Ankle boot
It is a more challenging classification problem than MNIST and top results are achieved by deep learning convolutional neural networks with a classification accuracy of about 90% to 95% on the hold out test dataset.
The example below loads the Fashion-MNIST dataset using the Keras API and creates a plot of the first nine images in the training dataset.
1 2 3 4 5 6 7 8 9 10 11 12 13 14 15 16 |
# example of loading the fashion mnist dataset from matplotlib import pyplot from keras.datasets import fashion_mnist # load dataset (trainX, trainy), (testX, testy) = fashion_mnist.load_data() # summarize loaded dataset print('Train: X=%s, y=%s' % (trainX.shape, trainy.shape)) print('Test: X=%s, y=%s' % (testX.shape, testy.shape)) # plot first few images for i in range(9): # define subplot pyplot.subplot(330 + 1 + i) # plot raw pixel data pyplot.imshow(trainX[i], cmap=pyplot.get_cmap('gray')) # show the figure pyplot.show() |
Running the example loads the Fashion-MNIST train and test dataset and prints their shape.
We can see that there are 60,000 examples in the training dataset and 10,000 in the test dataset and that images are indeed square with 28×28 pixels.
1 2 |
Train: X=(60000, 28, 28), y=(60000,) Test: X=(10000, 28, 28), y=(10000,) |
A plot of the first nine images in the dataset is also created showing that indeed the images are grayscale photographs of items of clothing.
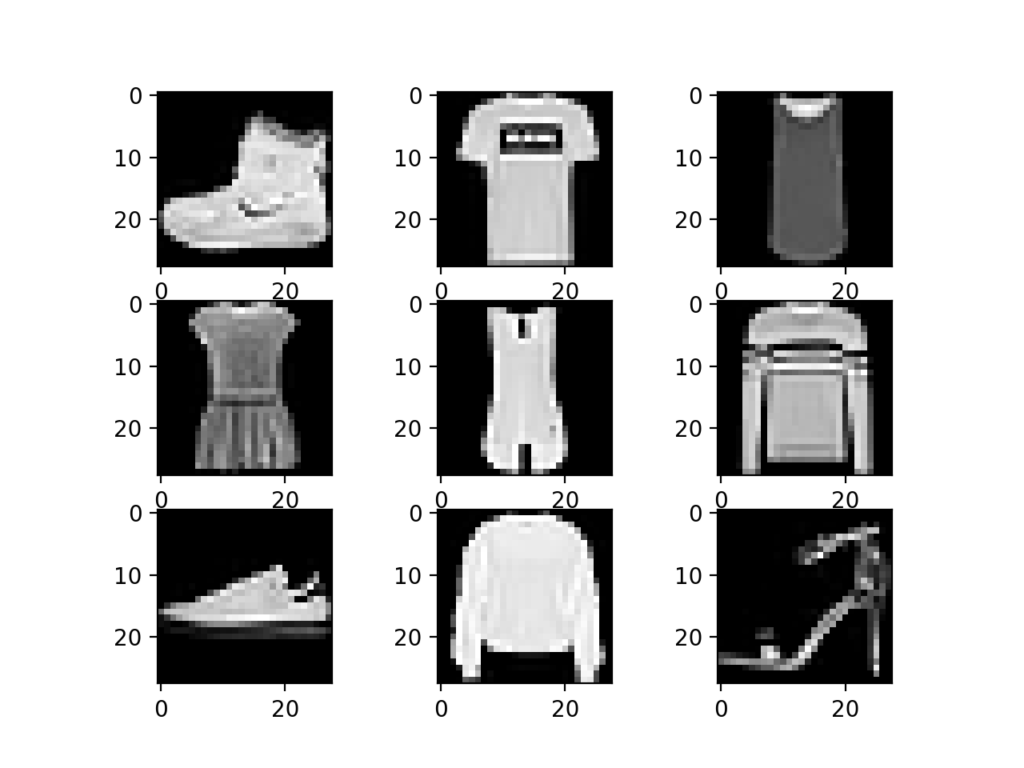
Plot of a Subset of Images From the Fashion-MNIST Dataset
Model Evaluation Methodology
The Fashion MNIST dataset was developed as a response to the wide use of the MNIST dataset, that has been effectively “solved” given the use of modern convolutional neural networks.
Fashion-MNIST was proposed to be a replacement for MNIST, and although it has not been solved, it is possible to routinely achieve error rates of 10% or less. Like MNIST, it can be a useful starting point for developing and practicing a methodology for solving image classification using convolutional neural networks.
Instead of reviewing the literature on well-performing models on the dataset, we can develop a new model from scratch.
The dataset already has a well-defined train and test dataset that we can use.
In order to estimate the performance of a model for a given training run, we can further split the training set into a train and validation dataset. Performance on the train and validation dataset over each run can then be plotted to provide learning curves and insight into how well a model is learning the problem.
The Keras API supports this by specifying the “validation_data” argument to the model.fit() function when training the model, that will, in turn, return an object that describes model performance for the chosen loss and metrics on each training epoch.
1 2 |
# record model performance on a validation dataset during training history = model.fit(..., validation_data=(valX, valY)) |
In order to estimate the performance of a model on the problem in general, we can use k-fold cross-validation, perhaps 5-fold cross-validation. This will give some account of the model’s variance with both respect to differences in the training and test datasets and the stochastic nature of the learning algorithm. The performance of a model can be taken as the mean performance across k-folds, given with the standard deviation, that could be used to estimate a confidence interval if desired.
We can use the KFold class from the scikit-learn API to implement the k-fold cross-validation evaluation of a given neural network model. There are many ways to achieve this, although we can choose a flexible approach where the KFold is only used to specify the row indexes used for each split.
1 2 3 4 5 6 7 8 |
# example of k-fold cv for a neural net data = ... # prepare cross validation kfold = KFold(5, shuffle=True, random_state=1) # enumerate splits for train_ix, test_ix in kfold.split(data): model = ... ... |
We will hold back the actual test dataset and use it as an evaluation of our final model.
How to Develop a Baseline Model
The first step is to develop a baseline model.
This is critical as it both involves developing the infrastructure for the test harness so that any model we design can be evaluated on the dataset, and it establishes a baseline in model performance on the problem, by which all improvements can be compared.
The design of the test harness is modular, and we can develop a separate function for each piece. This allows a given aspect of the test harness to be modified or inter-changed, if we desire, separately from the rest.
We can develop this test harness with five key elements. They are the loading of the dataset, the preparation of the dataset, the definition of the model, the evaluation of the model, and the presentation of results.
Load Dataset
We know some things about the dataset.
For example, we know that the images are all pre-segmented (e.g. each image contains a single item of clothing), that the images all have the same square size of 28×28 pixels, and that the images are grayscale. Therefore, we can load the images and reshape the data arrays to have a single color channel.
1 2 3 4 5 |
# load dataset (trainX, trainY), (testX, testY) = fashion_mnist.load_data() # reshape dataset to have a single channel trainX = trainX.reshape((trainX.shape[0], 28, 28, 1)) testX = testX.reshape((testX.shape[0], 28, 28, 1)) |
We also know that there are 10 classes and that classes are represented as unique integers.
We can, therefore, use a one hot encoding for the class element of each sample, transforming the integer into a 10 element binary vector with a 1 for the index of the class value. We can achieve this with the to_categorical() utility function.
1 2 3 |
# one hot encode target values trainY = to_categorical(trainY) testY = to_categorical(testY) |
The load_dataset() function implements these behaviors and can be used to load the dataset.
1 2 3 4 5 6 7 8 9 10 11 |
# load train and test dataset def load_dataset(): # load dataset (trainX, trainY), (testX, testY) = fashion_mnist.load_data() # reshape dataset to have a single channel trainX = trainX.reshape((trainX.shape[0], 28, 28, 1)) testX = testX.reshape((testX.shape[0], 28, 28, 1)) # one hot encode target values trainY = to_categorical(trainY) testY = to_categorical(testY) return trainX, trainY, testX, testY |
Prepare Pixel Data
We know that the pixel values for each image in the dataset are unsigned integers in the range between black and white, or 0 and 255.
We do not know the best way to scale the pixel values for modeling, but we know that some scaling will be required.
A good starting point is to normalize the pixel values of grayscale images, e.g. rescale them to the range [0,1]. This involves first converting the data type from unsigned integers to floats, then dividing the pixel values by the maximum value.
1 2 3 4 5 6 |
# convert from integers to floats train_norm = train.astype('float32') test_norm = test.astype('float32') # normalize to range 0-1 train_norm = train_norm / 255.0 test_norm = test_norm / 255.0 |
The prep_pixels() function below implement these behaviors and is provided with the pixel values for both the train and test datasets that will need to be scaled.
1 2 3 4 5 6 7 8 9 10 |
# scale pixels def prep_pixels(train, test): # convert from integers to floats train_norm = train.astype('float32') test_norm = test.astype('float32') # normalize to range 0-1 train_norm = train_norm / 255.0 test_norm = test_norm / 255.0 # return normalized images return train_norm, test_norm |
This function must be called to prepare the pixel values prior to any modeling.
Define Model
Next, we need to define a baseline convolutional neural network model for the problem.
The model has two main aspects: the feature extraction front end comprised of convolutional and pooling layers, and the classifier backend that will make a prediction.
For the convolutional front-end, we can start with a single convolutional layer with a small filter size (3,3) and a modest number of filters (32) followed by a max pooling layer. The filter maps can then be flattened to provide features to the classifier.
Given that the problem is a multi-class classification, we know that we will require an output layer with 10 nodes in order to predict the probability distribution of an image belonging to each of the 10 classes. This will also require the use of a softmax activation function. Between the feature extractor and the output layer, we can add a dense layer to interpret the features, in this case with 100 nodes.
All layers will use the ReLU activation function and the He weight initialization scheme, both best practices.
We will use a conservative configuration for the stochastic gradient descent optimizer with a learning rate of 0.01 and a momentum of 0.9. The categorical cross-entropy loss function will be optimized, suitable for multi-class classification, and we will monitor the classification accuracy metric, which is appropriate given we have the same number of examples in each of the 10 classes.
The define_model() function below will define and return this model.
1 2 3 4 5 6 7 8 9 10 11 12 |
# define cnn model def define_model(): model = Sequential() model.add(Conv2D(32, (3, 3), activation='relu', kernel_initializer='he_uniform', input_shape=(28, 28, 1))) model.add(MaxPooling2D((2, 2))) model.add(Flatten()) model.add(Dense(100, activation='relu', kernel_initializer='he_uniform')) model.add(Dense(10, activation='softmax')) # compile model opt = SGD(lr=0.01, momentum=0.9) model.compile(optimizer=opt, loss='categorical_crossentropy', metrics=['accuracy']) return model |
Evaluate Model
After the model is defined, we need to evaluate it.
The model will be evaluated using 5-fold cross-validation. The value of k=5 was chosen to provide a baseline for both repeated evaluation and to not be too large as to require a long running time. Each test set will be 20% of the training dataset, or about 12,000 examples, close to the size of the actual test set for this problem.
The training dataset is shuffled prior to being split and the sample shuffling is performed each time so that any model we evaluate will have the same train and test datasets in each fold, providing an apples-to-apples comparison.
We will train the baseline model for a modest 10 training epochs with a default batch size of 32 examples. The test set for each fold will be used to evaluate the model both during each epoch of the training run, so we can later create learning curves, and at the end of the run, so we can estimate the performance of the model. As such, we will keep track of the resulting history from each run, as well as the classification accuracy of the fold.
The evaluate_model() function below implements these behaviors, taking the training dataset as arguments and returning a list of accuracy scores and training histories that can be later summarized.
1 2 3 4 5 6 7 8 9 10 11 12 13 14 15 16 17 18 19 20 |
# evaluate a model using k-fold cross-validation def evaluate_model(dataX, dataY, n_folds=5): scores, histories = list(), list() # prepare cross validation kfold = KFold(n_folds, shuffle=True, random_state=1) # enumerate splits for train_ix, test_ix in kfold.split(dataX): # define model model = define_model() # select rows for train and test trainX, trainY, testX, testY = dataX[train_ix], dataY[train_ix], dataX[test_ix], dataY[test_ix] # fit model history = model.fit(trainX, trainY, epochs=10, batch_size=32, validation_data=(testX, testY), verbose=0) # evaluate model _, acc = model.evaluate(testX, testY, verbose=0) print('> %.3f' % (acc * 100.0)) # append scores scores.append(acc) histories.append(history) return scores, histories |
Present Results
Once the model has been evaluated, we can present the results.
There are two key aspects to present: the diagnostics of the learning behavior of the model during training and the estimation of the model performance. These can be implemented using separate functions.
First, the diagnostics involve creating a line plot showing model performance on the train and test set during each fold of the k-fold cross-validation. These plots are valuable for getting an idea of whether a model is overfitting, underfitting, or has a good fit for the dataset.
We will create a single figure with two subplots, one for loss and one for accuracy. Blue lines will indicate model performance on the training dataset and orange lines will indicate performance on the hold out test dataset. The summarize_diagnostics() function below creates and shows this plot given the collected training histories.
1 2 3 4 5 6 7 8 9 10 11 12 13 14 |
# plot diagnostic learning curves def summarize_diagnostics(histories): for i in range(len(histories)): # plot loss pyplot.subplot(211) pyplot.title('Cross Entropy Loss') pyplot.plot(histories[i].history['loss'], color='blue', label='train') pyplot.plot(histories[i].history['val_loss'], color='orange', label='test') # plot accuracy pyplot.subplot(212) pyplot.title('Classification Accuracy') pyplot.plot(histories[i].history['accuracy'], color='blue', label='train') pyplot.plot(histories[i].history['val_accuracy'], color='orange', label='test') pyplot.show() |
Next, the classification accuracy scores collected during each fold can be summarized by calculating the mean and standard deviation. This provides an estimate of the average expected performance of the model trained on this dataset, with an estimate of the average variance in the mean. We will also summarize the distribution of scores by creating and showing a box and whisker plot.
The summarize_performance() function below implements this for a given list of scores collected during model evaluation.
1 2 3 4 5 6 7 |
# summarize model performance def summarize_performance(scores): # print summary print('Accuracy: mean=%.3f std=%.3f, n=%d' % (mean(scores)*100, std(scores)*100, len(scores))) # box and whisker plots of results pyplot.boxplot(scores) pyplot.show() |
Complete Example
We need a function that will drive the test harness.
This involves calling all of the define functions.
1 2 3 4 5 6 7 8 9 10 11 12 |
# run the test harness for evaluating a model def run_test_harness(): # load dataset trainX, trainY, testX, testY = load_dataset() # prepare pixel data trainX, testX = prep_pixels(trainX, testX) # evaluate model scores, histories = evaluate_model(trainX, trainY) # learning curves summarize_diagnostics(histories) # summarize estimated performance summarize_performance(scores) |
We now have everything we need; the complete code example for a baseline convolutional neural network model on the MNIST dataset is listed below.
1 2 3 4 5 6 7 8 9 10 11 12 13 14 15 16 17 18 19 20 21 22 23 24 25 26 27 28 29 30 31 32 33 34 35 36 37 38 39 40 41 42 43 44 45 46 47 48 49 50 51 52 53 54 55 56 57 58 59 60 61 62 63 64 65 66 67 68 69 70 71 72 73 74 75 76 77 78 79 80 81 82 83 84 85 86 87 88 89 90 91 92 93 94 95 96 97 98 99 100 101 102 103 104 105 106 107 108 109 |
# baseline cnn model for fashion mnist from numpy import mean from numpy import std from matplotlib import pyplot from sklearn.model_selection import KFold from keras.datasets import fashion_mnist from keras.utils import to_categorical from keras.models import Sequential from keras.layers import Conv2D from keras.layers import MaxPooling2D from keras.layers import Dense from keras.layers import Flatten from keras.optimizers import SGD # load train and test dataset def load_dataset(): # load dataset (trainX, trainY), (testX, testY) = fashion_mnist.load_data() # reshape dataset to have a single channel trainX = trainX.reshape((trainX.shape[0], 28, 28, 1)) testX = testX.reshape((testX.shape[0], 28, 28, 1)) # one hot encode target values trainY = to_categorical(trainY) testY = to_categorical(testY) return trainX, trainY, testX, testY # scale pixels def prep_pixels(train, test): # convert from integers to floats train_norm = train.astype('float32') test_norm = test.astype('float32') # normalize to range 0-1 train_norm = train_norm / 255.0 test_norm = test_norm / 255.0 # return normalized images return train_norm, test_norm # define cnn model def define_model(): model = Sequential() model.add(Conv2D(32, (3, 3), activation='relu', kernel_initializer='he_uniform', input_shape=(28, 28, 1))) model.add(MaxPooling2D((2, 2))) model.add(Flatten()) model.add(Dense(100, activation='relu', kernel_initializer='he_uniform')) model.add(Dense(10, activation='softmax')) # compile model opt = SGD(lr=0.01, momentum=0.9) model.compile(optimizer=opt, loss='categorical_crossentropy', metrics=['accuracy']) return model # evaluate a model using k-fold cross-validation def evaluate_model(dataX, dataY, n_folds=5): scores, histories = list(), list() # prepare cross validation kfold = KFold(n_folds, shuffle=True, random_state=1) # enumerate splits for train_ix, test_ix in kfold.split(dataX): # define model model = define_model() # select rows for train and test trainX, trainY, testX, testY = dataX[train_ix], dataY[train_ix], dataX[test_ix], dataY[test_ix] # fit model history = model.fit(trainX, trainY, epochs=10, batch_size=32, validation_data=(testX, testY), verbose=0) # evaluate model _, acc = model.evaluate(testX, testY, verbose=0) print('> %.3f' % (acc * 100.0)) # append scores scores.append(acc) histories.append(history) return scores, histories # plot diagnostic learning curves def summarize_diagnostics(histories): for i in range(len(histories)): # plot loss pyplot.subplot(211) pyplot.title('Cross Entropy Loss') pyplot.plot(histories[i].history['loss'], color='blue', label='train') pyplot.plot(histories[i].history['val_loss'], color='orange', label='test') # plot accuracy pyplot.subplot(212) pyplot.title('Classification Accuracy') pyplot.plot(histories[i].history['accuracy'], color='blue', label='train') pyplot.plot(histories[i].history['val_accuracy'], color='orange', label='test') pyplot.show() # summarize model performance def summarize_performance(scores): # print summary print('Accuracy: mean=%.3f std=%.3f, n=%d' % (mean(scores)*100, std(scores)*100, len(scores))) # box and whisker plots of results pyplot.boxplot(scores) pyplot.show() # run the test harness for evaluating a model def run_test_harness(): # load dataset trainX, trainY, testX, testY = load_dataset() # prepare pixel data trainX, testX = prep_pixels(trainX, testX) # evaluate model scores, histories = evaluate_model(trainX, trainY) # learning curves summarize_diagnostics(histories) # summarize estimated performance summarize_performance(scores) # entry point, run the test harness run_test_harness() |
Running the example prints the classification accuracy for each fold of the cross-validation process. This is helpful to get an idea that the model evaluation is progressing.
Note: Your results may vary given the stochastic nature of the algorithm or evaluation procedure, or differences in numerical precision. Consider running the example a few times and compare the average outcome.
We can see that for each fold, the baseline model achieved an error rate below 10%, and in two cases 98% and 99% accuracy. These are good results.
1 2 3 4 5 |
> 91.200 > 91.217 > 90.958 > 91.242 > 91.317 |
Next, a diagnostic plot is shown, giving insight into the learning behavior of the model across each fold.
In this case, we can see that the model generally achieves a good fit, with train and test learning curves converging. There may be some signs of slight overfitting.
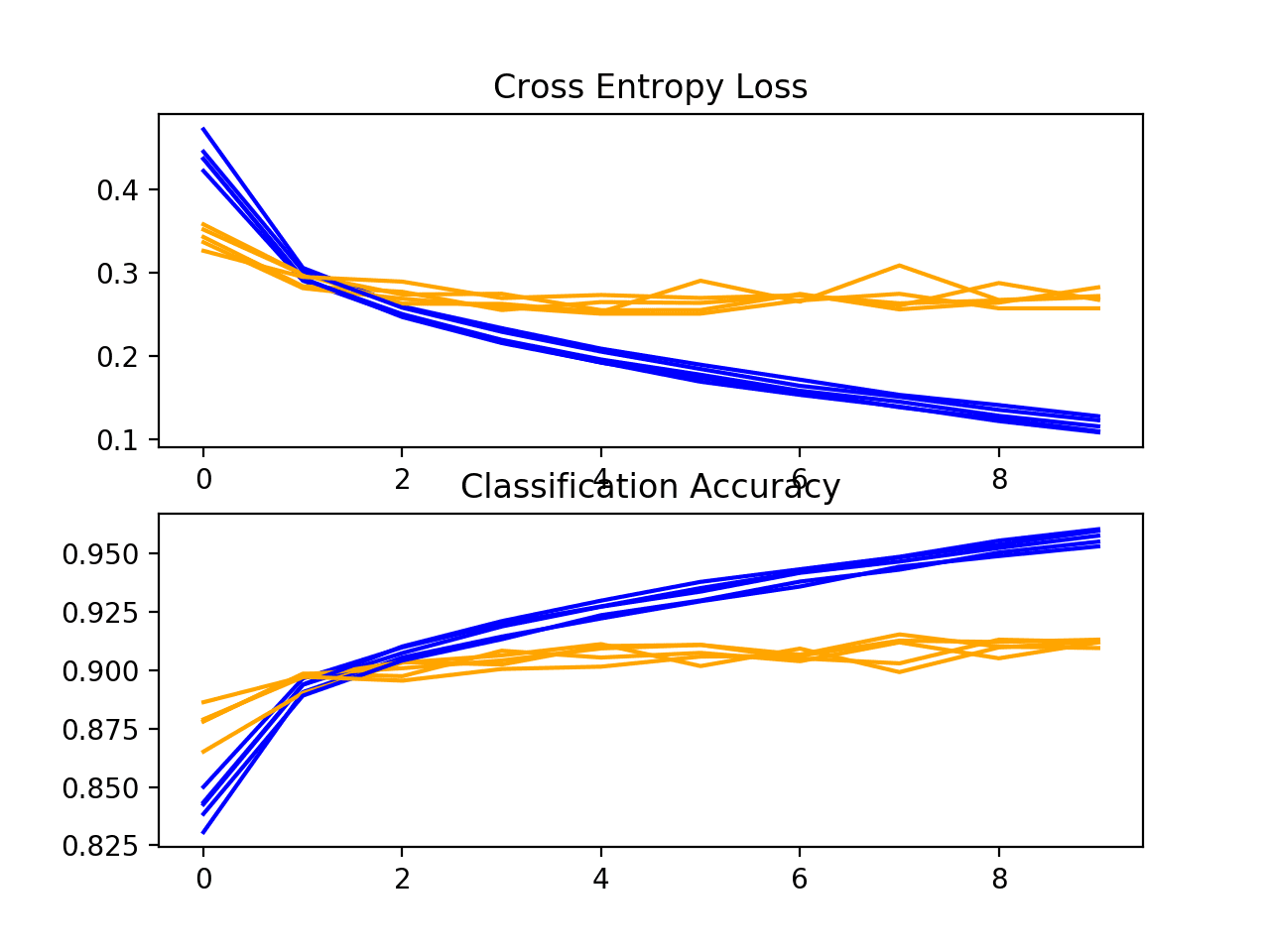
Loss-and-Accuracy-Learning-Curves-for-the-Baseline-Model-on-the-Fashion-MNIST-Dataset-During-k-Fold-Cross-Validation
Next, the summary of the model performance is calculated. We can see in this case, the model has an estimated skill of about 96%, which is impressive.
1 |
Accuracy: mean=91.187 std=0.121, n=5 |
Finally, a box and whisker plot is created to summarize the distribution of accuracy scores.
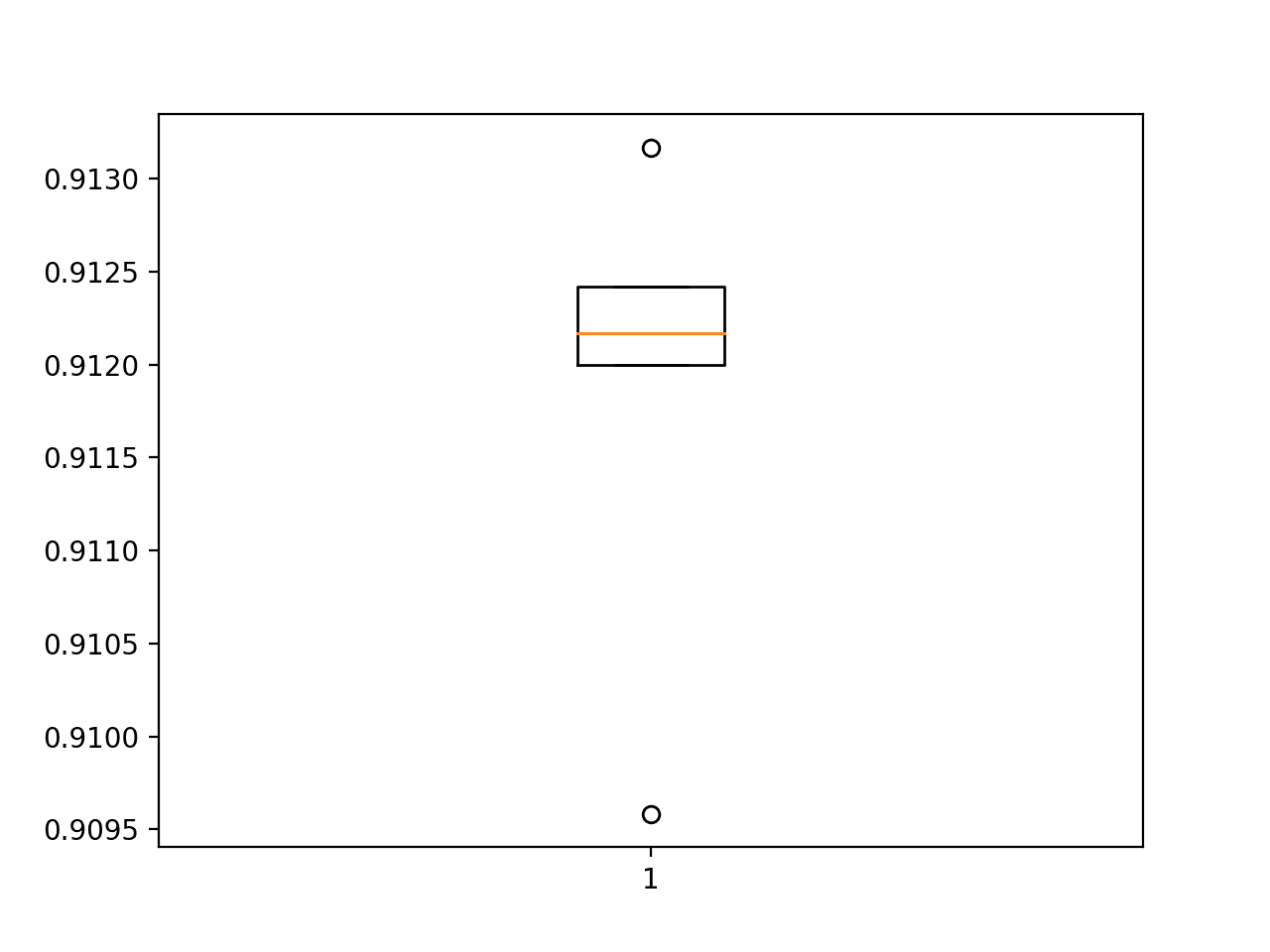
Box-and-Whisker-Plot-of-Accuracy-Scores-for-the-Baseline-Model-on-the-Fashion-MNIST-Dataset-Evaluated-Using-k-Fold-Cross-Validation
As we would expect, the distribution spread across the low-nineties.
We now have a robust test harness and a well-performing baseline model.
How to Develop an Improved Model
There are many ways that we might explore improvements to the baseline model.
We will look at areas that often result in an improvement, so-called low-hanging fruit. The first will be a change to the convolutional operation to add padding and the second will build on this to increase the number of filters.
Padding Convolutions
Adding padding to the convolutional operation can often result in better model performance, as more of the input image of feature maps are given an opportunity to participate or contribute to the output
By default, the convolutional operation uses ‘valid‘ padding, which means that convolutions are only applied where possible. This can be changed to ‘same‘ padding so that zero values are added around the input such that the output has the same size as the input.
1 2 |
... model.add(Conv2D(32, (3, 3), padding='same', activation='relu', kernel_initializer='he_uniform', input_shape=(28, 28, 1))) |
The full code listing including the change to padding is provided below for completeness.
1 2 3 4 5 6 7 8 9 10 11 12 13 14 15 16 17 18 19 20 21 22 23 24 25 26 27 28 29 30 31 32 33 34 35 36 37 38 39 40 41 42 43 44 45 46 47 48 49 50 51 52 53 54 55 56 57 58 59 60 61 62 63 64 65 66 67 68 69 70 71 72 73 74 75 76 77 78 79 80 81 82 83 84 85 86 87 88 89 90 91 92 93 94 95 96 97 98 99 100 101 102 103 104 105 106 107 108 109 |
# model with padded convolutions for the fashion mnist dataset from numpy import mean from numpy import std from matplotlib import pyplot from sklearn.model_selection import KFold from keras.datasets import fashion_mnist from keras.utils import to_categorical from keras.models import Sequential from keras.layers import Conv2D from keras.layers import MaxPooling2D from keras.layers import Dense from keras.layers import Flatten from keras.optimizers import SGD # load train and test dataset def load_dataset(): # load dataset (trainX, trainY), (testX, testY) = fashion_mnist.load_data() # reshape dataset to have a single channel trainX = trainX.reshape((trainX.shape[0], 28, 28, 1)) testX = testX.reshape((testX.shape[0], 28, 28, 1)) # one hot encode target values trainY = to_categorical(trainY) testY = to_categorical(testY) return trainX, trainY, testX, testY # scale pixels def prep_pixels(train, test): # convert from integers to floats train_norm = train.astype('float32') test_norm = test.astype('float32') # normalize to range 0-1 train_norm = train_norm / 255.0 test_norm = test_norm / 255.0 # return normalized images return train_norm, test_norm # define cnn model def define_model(): model = Sequential() model.add(Conv2D(32, (3, 3), padding='same', activation='relu', kernel_initializer='he_uniform', input_shape=(28, 28, 1))) model.add(MaxPooling2D((2, 2))) model.add(Flatten()) model.add(Dense(100, activation='relu', kernel_initializer='he_uniform')) model.add(Dense(10, activation='softmax')) # compile model opt = SGD(lr=0.01, momentum=0.9) model.compile(optimizer=opt, loss='categorical_crossentropy', metrics=['accuracy']) return model # evaluate a model using k-fold cross-validation def evaluate_model(dataX, dataY, n_folds=5): scores, histories = list(), list() # prepare cross validation kfold = KFold(n_folds, shuffle=True, random_state=1) # enumerate splits for train_ix, test_ix in kfold.split(dataX): # define model model = define_model() # select rows for train and test trainX, trainY, testX, testY = dataX[train_ix], dataY[train_ix], dataX[test_ix], dataY[test_ix] # fit model history = model.fit(trainX, trainY, epochs=10, batch_size=32, validation_data=(testX, testY), verbose=0) # evaluate model _, acc = model.evaluate(testX, testY, verbose=0) print('> %.3f' % (acc * 100.0)) # append scores scores.append(acc) histories.append(history) return scores, histories # plot diagnostic learning curves def summarize_diagnostics(histories): for i in range(len(histories)): # plot loss pyplot.subplot(211) pyplot.title('Cross Entropy Loss') pyplot.plot(histories[i].history['loss'], color='blue', label='train') pyplot.plot(histories[i].history['val_loss'], color='orange', label='test') # plot accuracy pyplot.subplot(212) pyplot.title('Classification Accuracy') pyplot.plot(histories[i].history['accuracy'], color='blue', label='train') pyplot.plot(histories[i].history['val_accuracy'], color='orange', label='test') pyplot.show() # summarize model performance def summarize_performance(scores): # print summary print('Accuracy: mean=%.3f std=%.3f, n=%d' % (mean(scores)*100, std(scores)*100, len(scores))) # box and whisker plots of results pyplot.boxplot(scores) pyplot.show() # run the test harness for evaluating a model def run_test_harness(): # load dataset trainX, trainY, testX, testY = load_dataset() # prepare pixel data trainX, testX = prep_pixels(trainX, testX) # evaluate model scores, histories = evaluate_model(trainX, trainY) # learning curves summarize_diagnostics(histories) # summarize estimated performance summarize_performance(scores) # entry point, run the test harness run_test_harness() |
Running the example again reports model performance for each fold of the cross-validation process.
Note: Your results may vary given the stochastic nature of the algorithm or evaluation procedure, or differences in numerical precision. Consider running the example a few times and compare the average outcome.
We can see perhaps a small improvement in model performance as compared to the baseline across the cross-validation folds.
1 2 3 4 5 |
> 90.875 > 91.442 > 91.242 > 91.275 > 91.450 |
A plot of the learning curves is created. As with the baseline model, we may see some slight overfitting. This could be addressed perhaps with the use of regularization or the training for fewer epochs.
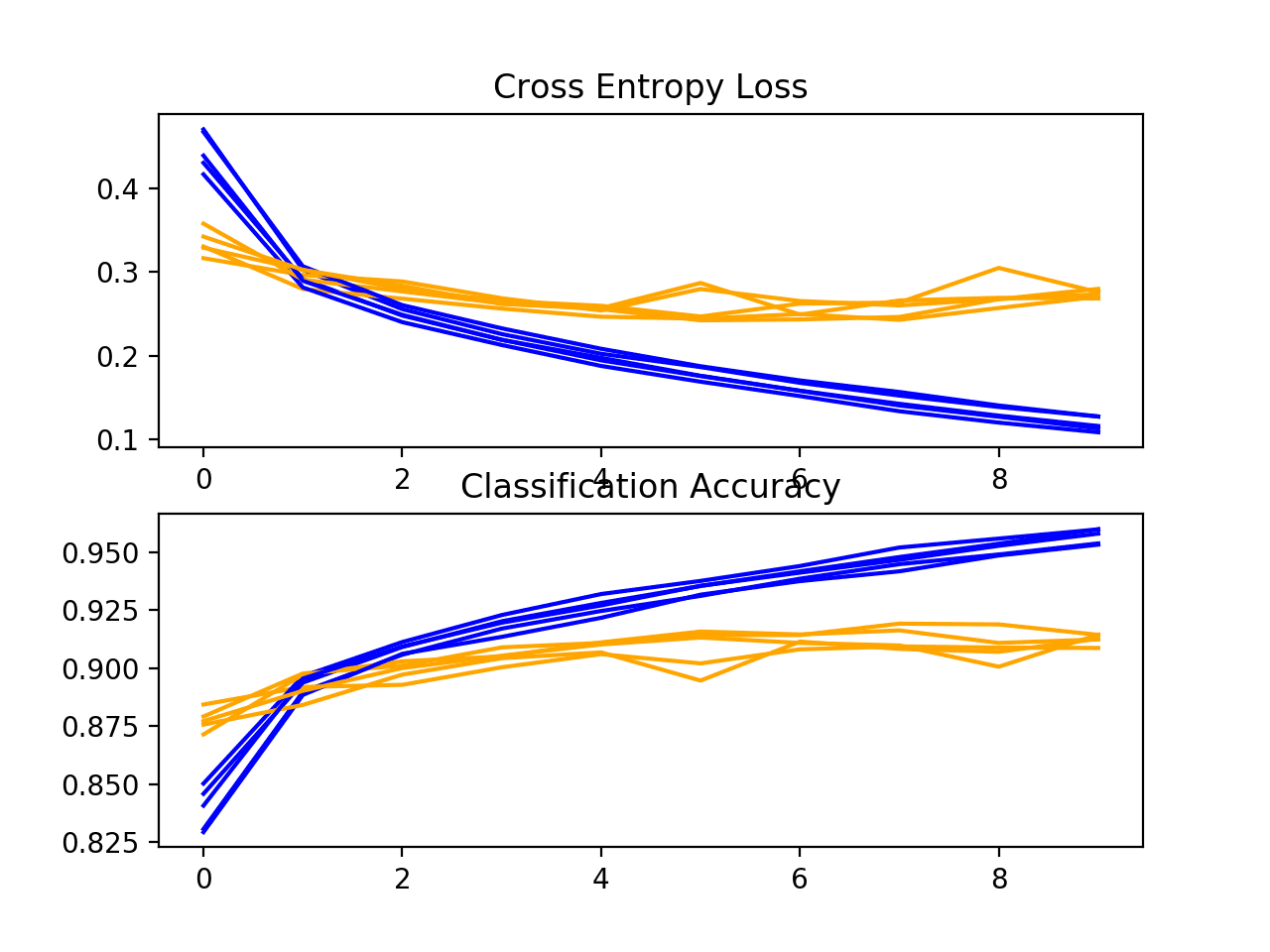
Loss-and-Accuracy-Learning-Curves-for-the-Same-Padding-on-the-Fashion-MNIST-Dataset-During-k-Fold-Cross-Validation
Next, the estimated performance of the model is presented, showing performance with a very slight decrease in the mean accuracy of the model, 91.257% as compared to 91.187% with the baseline model.
This may or may not be a real effect as it is within the bounds of the standard deviation. Perhaps more repeats of the experiment could tease out this fact.
1 |
Accuracy: mean=91.257 std=0.209, n=5 |
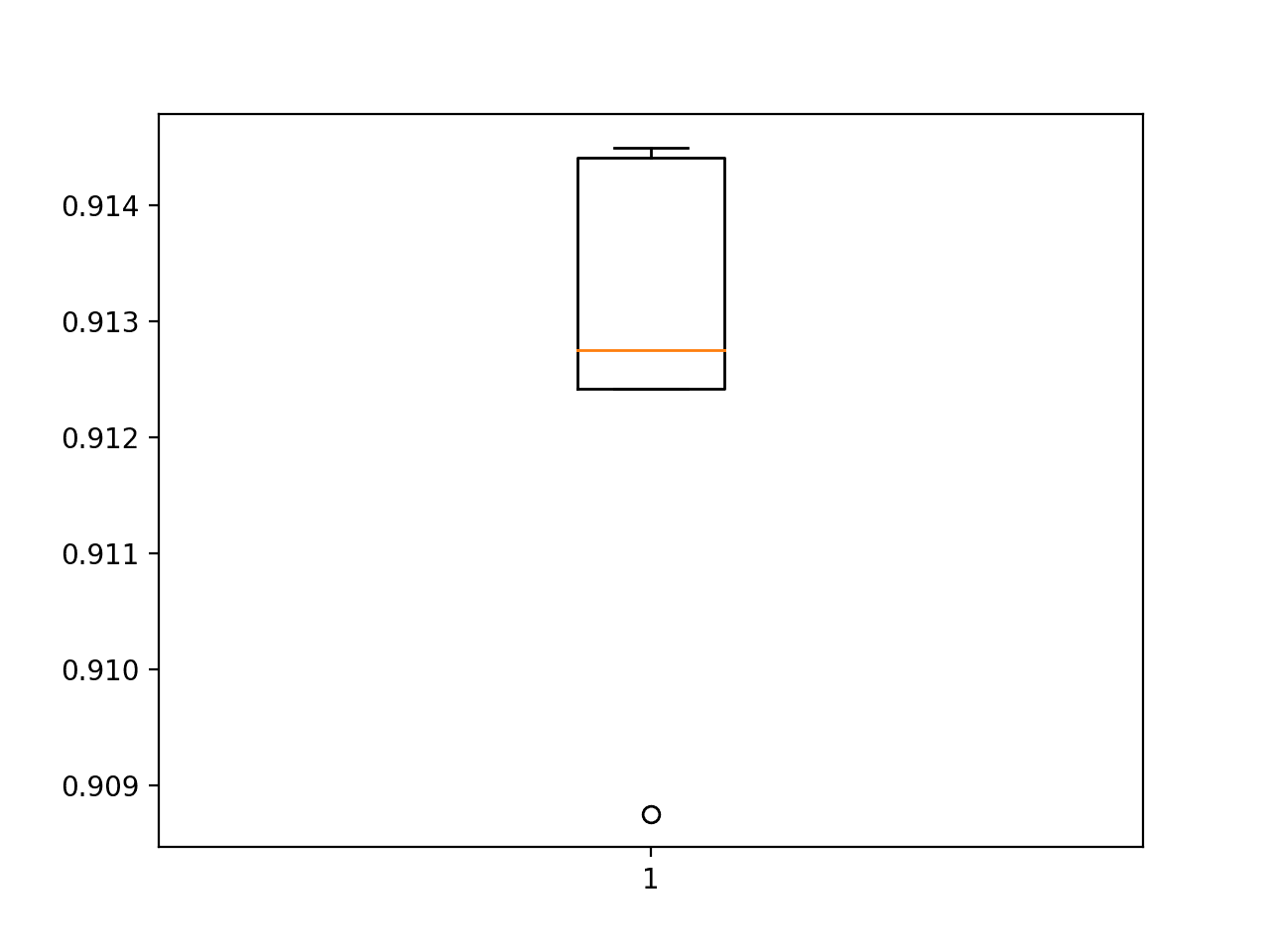
Box-and-Whisker-Plot-of-Accuracy-Scores-for-Same-Padding-on-the-Fashion-MNIST-Dataset-Evaluated-Using-k-Fold-Cross-Validation
Increasing Filters
An increase in the number of filters used in the convolutional layer can often improve performance, as it can provide more opportunity for extracting simple features from the input images.
This is especially relevant when very small filters are used, such as 3×3 pixels.
In this change, we can increase the number of filters in the convolutional layer from 32 to double that at 64. We will also build upon the possible improvement offered by using ‘same‘ padding.
1 2 |
... model.add(Conv2D(64, (3, 3), padding='same', activation='relu', kernel_initializer='he_uniform', input_shape=(28, 28, 1))) |
The full code listing including the change to padding is provided below for completeness.
1 2 3 4 5 6 7 8 9 10 11 12 13 14 15 16 17 18 19 20 21 22 23 24 25 26 27 28 29 30 31 32 33 34 35 36 37 38 39 40 41 42 43 44 45 46 47 48 49 50 51 52 53 54 55 56 57 58 59 60 61 62 63 64 65 66 67 68 69 70 71 72 73 74 75 76 77 78 79 80 81 82 83 84 85 86 87 88 89 90 91 92 93 94 95 96 97 98 99 100 101 102 103 104 105 106 107 108 109 |
# model with double the filters for the fashion mnist dataset from numpy import mean from numpy import std from matplotlib import pyplot from sklearn.model_selection import KFold from keras.datasets import fashion_mnist from keras.utils import to_categorical from keras.models import Sequential from keras.layers import Conv2D from keras.layers import MaxPooling2D from keras.layers import Dense from keras.layers import Flatten from keras.optimizers import SGD # load train and test dataset def load_dataset(): # load dataset (trainX, trainY), (testX, testY) = fashion_mnist.load_data() # reshape dataset to have a single channel trainX = trainX.reshape((trainX.shape[0], 28, 28, 1)) testX = testX.reshape((testX.shape[0], 28, 28, 1)) # one hot encode target values trainY = to_categorical(trainY) testY = to_categorical(testY) return trainX, trainY, testX, testY # scale pixels def prep_pixels(train, test): # convert from integers to floats train_norm = train.astype('float32') test_norm = test.astype('float32') # normalize to range 0-1 train_norm = train_norm / 255.0 test_norm = test_norm / 255.0 # return normalized images return train_norm, test_norm # define cnn model def define_model(): model = Sequential() model.add(Conv2D(64, (3, 3), padding='same', activation='relu', kernel_initializer='he_uniform', input_shape=(28, 28, 1))) model.add(MaxPooling2D((2, 2))) model.add(Flatten()) model.add(Dense(100, activation='relu', kernel_initializer='he_uniform')) model.add(Dense(10, activation='softmax')) # compile model opt = SGD(lr=0.01, momentum=0.9) model.compile(optimizer=opt, loss='categorical_crossentropy', metrics=['accuracy']) return model # evaluate a model using k-fold cross-validation def evaluate_model(dataX, dataY, n_folds=5): scores, histories = list(), list() # prepare cross validation kfold = KFold(n_folds, shuffle=True, random_state=1) # enumerate splits for train_ix, test_ix in kfold.split(dataX): # define model model = define_model() # select rows for train and test trainX, trainY, testX, testY = dataX[train_ix], dataY[train_ix], dataX[test_ix], dataY[test_ix] # fit model history = model.fit(trainX, trainY, epochs=10, batch_size=32, validation_data=(testX, testY), verbose=0) # evaluate model _, acc = model.evaluate(testX, testY, verbose=0) print('> %.3f' % (acc * 100.0)) # append scores scores.append(acc) histories.append(history) return scores, histories # plot diagnostic learning curves def summarize_diagnostics(histories): for i in range(len(histories)): # plot loss pyplot.subplot(211) pyplot.title('Cross Entropy Loss') pyplot.plot(histories[i].history['loss'], color='blue', label='train') pyplot.plot(histories[i].history['val_loss'], color='orange', label='test') # plot accuracy pyplot.subplot(212) pyplot.title('Classification Accuracy') pyplot.plot(histories[i].history['accuracy'], color='blue', label='train') pyplot.plot(histories[i].history['val_accuracy'], color='orange', label='test') pyplot.show() # summarize model performance def summarize_performance(scores): # print summary print('Accuracy: mean=%.3f std=%.3f, n=%d' % (mean(scores)*100, std(scores)*100, len(scores))) # box and whisker plots of results pyplot.boxplot(scores) pyplot.show() # run the test harness for evaluating a model def run_test_harness(): # load dataset trainX, trainY, testX, testY = load_dataset() # prepare pixel data trainX, testX = prep_pixels(trainX, testX) # evaluate model scores, histories = evaluate_model(trainX, trainY) # learning curves summarize_diagnostics(histories) # summarize estimated performance summarize_performance(scores) # entry point, run the test harness run_test_harness() |
Running the example reports model performance for each fold of the cross-validation process.
Note: Your results may vary given the stochastic nature of the algorithm or evaluation procedure, or differences in numerical precision. Consider running the example a few times and compare the average outcome.
The per-fold scores may suggest some further improvement over the baseline and using same padding alone.
1 2 3 4 5 |
> 90.917 > 90.908 > 90.175 > 91.158 > 91.408 |
A plot of the learning curves is created, in this case showing that the models still have a reasonable fit on the problem, with a small sign of some of the runs overfitting.
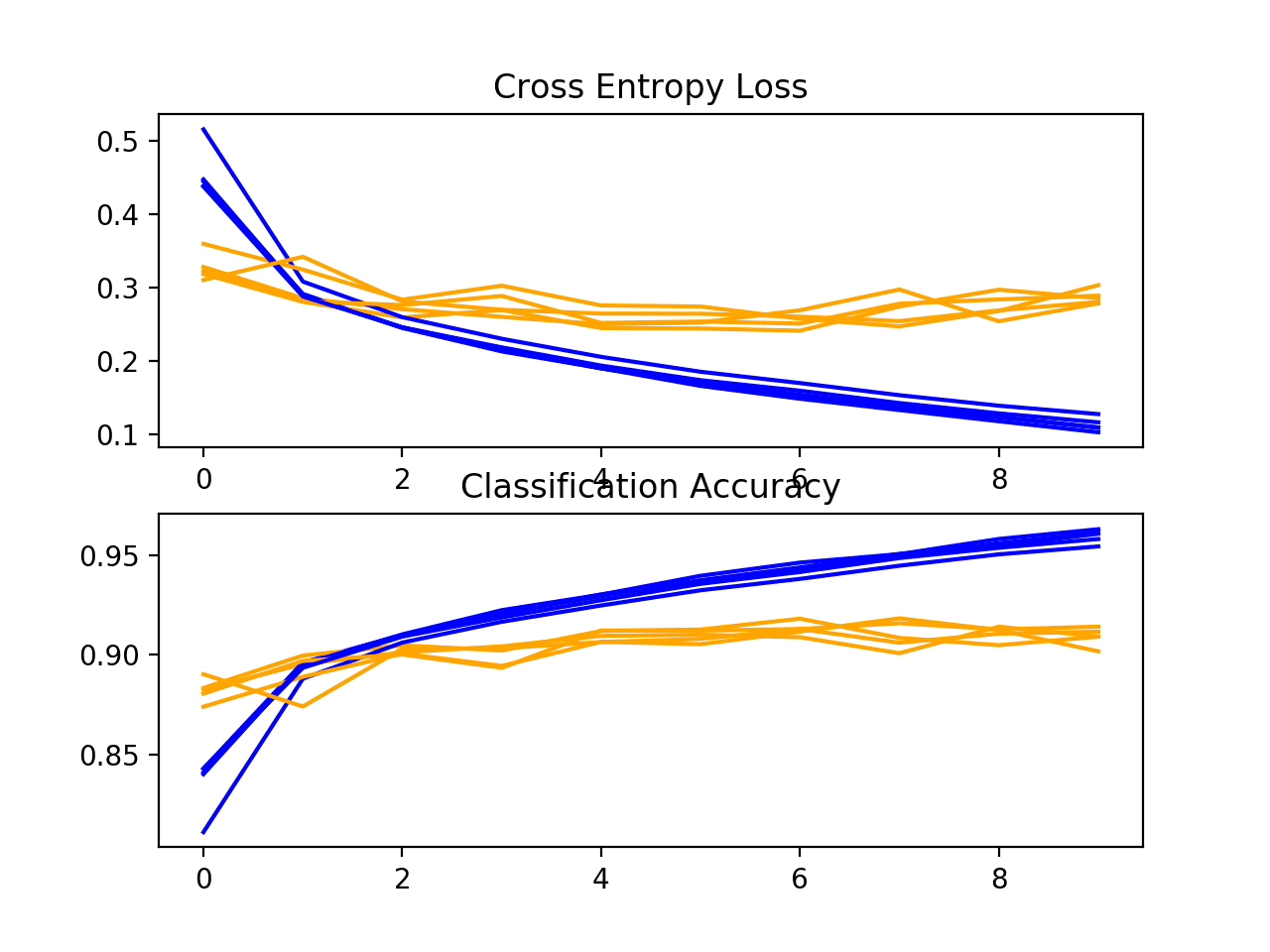
Loss-and-Accuracy-Learning-Curves-for-the-More-Filters-and-Padding-on-the-Fashion-MNIST-Dataset-During-k-Fold-Cross-Validation
Next, the estimated performance of the model is presented, showing a small improvement in performance as compared to the baseline from 90.913% to 91.257%.
Again, the change is still within the bounds of the standard deviation, and it is not clear whether the effect is real.
1 |
Accuracy: mean=90.913 std=0.412, n=5 |
How to Finalize the Model and Make Predictions
The process of model improvement may continue for as long as we have ideas and the time and resources to test them out.
At some point, a final model configuration must be chosen and adopted. In this case, we will keep things simple and use the baseline model as the final model.
First, we will finalize our model, but fitting a model on the entire training dataset and saving the model to file for later use. We will then load the model and evaluate its performance on the hold out test dataset, to get an idea of how well the chosen model actually performs in practice. Finally, we will use the saved model to make a prediction on a single image.
Save Final Model
A final model is typically fit on all available data, such as the combination of all train and test dataset.
In this tutorial, we are intentionally holding back a test dataset so that we can estimate the performance of the final model, which can be a good idea in practice. As such, we will fit our model on the training dataset only.
1 2 |
# fit model model.fit(trainX, trainY, epochs=10, batch_size=32, verbose=0) |
Once fit, we can save the final model to an h5 file by calling the save() function on the model and pass in the chosen filename.
1 2 |
# save model model.save('final_model.h5') |
Note: saving and loading a Keras model requires that the h5py library is installed on your workstation.
The complete example of fitting the final model on the training dataset and saving it to file is listed below.
1 2 3 4 5 6 7 8 9 10 11 12 13 14 15 16 17 18 19 20 21 22 23 24 25 26 27 28 29 30 31 32 33 34 35 36 37 38 39 40 41 42 43 44 45 46 47 48 49 50 51 52 53 54 55 56 57 58 59 60 61 |
# save the final model to file from keras.datasets import fashion_mnist from keras.utils import to_categorical from keras.models import Sequential from keras.layers import Conv2D from keras.layers import MaxPooling2D from keras.layers import Dense from keras.layers import Flatten from keras.optimizers import SGD # load train and test dataset def load_dataset(): # load dataset (trainX, trainY), (testX, testY) = fashion_mnist.load_data() # reshape dataset to have a single channel trainX = trainX.reshape((trainX.shape[0], 28, 28, 1)) testX = testX.reshape((testX.shape[0], 28, 28, 1)) # one hot encode target values trainY = to_categorical(trainY) testY = to_categorical(testY) return trainX, trainY, testX, testY # scale pixels def prep_pixels(train, test): # convert from integers to floats train_norm = train.astype('float32') test_norm = test.astype('float32') # normalize to range 0-1 train_norm = train_norm / 255.0 test_norm = test_norm / 255.0 # return normalized images return train_norm, test_norm # define cnn model def define_model(): model = Sequential() model.add(Conv2D(32, (3, 3), activation='relu', kernel_initializer='he_uniform', input_shape=(28, 28, 1))) model.add(MaxPooling2D((2, 2))) model.add(Flatten()) model.add(Dense(100, activation='relu', kernel_initializer='he_uniform')) model.add(Dense(10, activation='softmax')) # compile model opt = SGD(lr=0.01, momentum=0.9) model.compile(optimizer=opt, loss='categorical_crossentropy', metrics=['accuracy']) return model # run the test harness for evaluating a model def run_test_harness(): # load dataset trainX, trainY, testX, testY = load_dataset() # prepare pixel data trainX, testX = prep_pixels(trainX, testX) # define model model = define_model() # fit model model.fit(trainX, trainY, epochs=10, batch_size=32, verbose=0) # save model model.save('final_model.h5') # entry point, run the test harness run_test_harness() |
After running this example, you will now have a 1.2-megabyte file with the name ‘final_model.h5‘ in your current working directory.
Evaluate Final Model
We can now load the final model and evaluate it on the hold out test dataset.
This is something we might do if we were interested in presenting the performance of the chosen model to project stakeholders.
The model can be loaded via the load_model() function.
The complete example of loading the saved model and evaluating it on the test dataset is listed below.
1 2 3 4 5 6 7 8 9 10 11 12 13 14 15 16 17 18 19 20 21 22 23 24 25 26 27 28 29 30 31 32 33 34 35 36 37 38 39 40 41 42 |
# evaluate the deep model on the test dataset from keras.datasets import fashion_mnist from keras.models import load_model from keras.utils import to_categorical # load train and test dataset def load_dataset(): # load dataset (trainX, trainY), (testX, testY) = fashion_mnist.load_data() # reshape dataset to have a single channel trainX = trainX.reshape((trainX.shape[0], 28, 28, 1)) testX = testX.reshape((testX.shape[0], 28, 28, 1)) # one hot encode target values trainY = to_categorical(trainY) testY = to_categorical(testY) return trainX, trainY, testX, testY # scale pixels def prep_pixels(train, test): # convert from integers to floats train_norm = train.astype('float32') test_norm = test.astype('float32') # normalize to range 0-1 train_norm = train_norm / 255.0 test_norm = test_norm / 255.0 # return normalized images return train_norm, test_norm # run the test harness for evaluating a model def run_test_harness(): # load dataset trainX, trainY, testX, testY = load_dataset() # prepare pixel data trainX, testX = prep_pixels(trainX, testX) # load model model = load_model('final_model.h5') # evaluate model on test dataset _, acc = model.evaluate(testX, testY, verbose=0) print('> %.3f' % (acc * 100.0)) # entry point, run the test harness run_test_harness() |
Running the example loads the saved model and evaluates the model on the hold out test dataset.
The classification accuracy for the model on the test dataset is calculated and printed.
Note: Your results may vary given the stochastic nature of the algorithm or evaluation procedure, or differences in numerical precision. Consider running the example a few times and compare the average outcome.
In this case, we can see that the model achieved an accuracy of 90.990%, or just less than 10% classification error, which is not bad.
1 |
> 90.990 |
Make Prediction
We can use our saved model to make a prediction on new images.
The model assumes that new images are grayscale, they have been segmented so that one image contains one centered piece of clothing on a black background, and that the size of the image is square with the size 28×28 pixels.
Below is an image extracted from the MNIST test dataset. You can save it in your current working directory with the filename ‘sample_image.png‘.
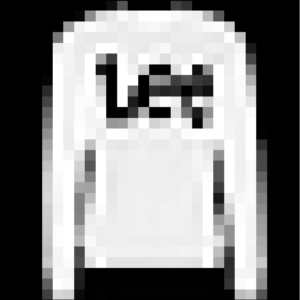
Sample Clothing (Pullover)
We will pretend this is an entirely new and unseen image, prepared in the required way, and see how we might use our saved model to predict the integer that the image represents. For this example, we expect class “2” for “Pullover” (also called a jumper).
First, we can load the image, force it to be grayscale format, and force the size to be 28×28 pixels. The loaded image can then be resized to have a single channel and represent a single sample in a dataset. The load_image() function implements this and will return the loaded image ready for classification.
Importantly, the pixel values are prepared in the same way as the pixel values were prepared for the training dataset when fitting the final model, in this case, normalized.
1 2 3 4 5 6 7 8 9 10 11 12 |
# load and prepare the image def load_image(filename): # load the image img = load_img(filename, grayscale=True, target_size=(28, 28)) # convert to array img = img_to_array(img) # reshape into a single sample with 1 channel img = img.reshape(1, 28, 28, 1) # prepare pixel data img = img.astype('float32') img = img / 255.0 return img |
Next, we can load the model as in the previous section and call the predict_classes() function to predict the clothing in the image.
1 2 |
# predict the class result = model.predict_classes(img) |
The complete example is listed below.
1 2 3 4 5 6 7 8 9 10 11 12 13 14 15 16 17 18 19 20 21 22 23 24 25 26 27 28 29 30 |
# make a prediction for a new image. from keras.preprocessing.image import load_img from keras.preprocessing.image import img_to_array from keras.models import load_model # load and prepare the image def load_image(filename): # load the image img = load_img(filename, grayscale=True, target_size=(28, 28)) # convert to array img = img_to_array(img) # reshape into a single sample with 1 channel img = img.reshape(1, 28, 28, 1) # prepare pixel data img = img.astype('float32') img = img / 255.0 return img # load an image and predict the class def run_example(): # load the image img = load_image('sample_image.png') # load model model = load_model('final_model.h5') # predict the class result = model.predict_classes(img) print(result[0]) # entry point, run the example run_example() |
Running the example first loads and prepares the image, loads the model, and then correctly predicts that the loaded image represents a pullover or class ‘2’.
1 |
2 |
Extensions
This section lists some ideas for extending the tutorial that you may wish to explore.
- Regularization. Explore how adding regularization impacts model performance as compared to the baseline model, such as weight decay, early stopping, and dropout.
- Tune the Learning Rate. Explore how different learning rates impact the model performance as compared to the baseline model, such as 0.001 and 0.0001.
- Tune Model Depth. Explore how adding more layers to the model impact the model performance as compared to the baseline model, such as another block of convolutional and pooling layers or another dense layer in the classifier part of the model.
If you explore any of these extensions, I’d love to know.
Post your findings in the comments below.
Further Reading
This section provides more resources on the topic if you are looking to go deeper.
APIs
Articles
Summary
In this tutorial, you discovered how to develop a convolutional neural network for clothing classification from scratch.
Specifically, you learned:
- How to develop a test harness to develop a robust evaluation of a model and establish a baseline of performance for a classification task.
- How to explore extensions to a baseline model to improve learning and model capacity.
- How to develop a finalized model, evaluate the performance of the final model, and use it to make predictions on new images.
Do you have any questions?
Ask your questions in the comments below and I will do my best to answer.
Jason, what a brilliant tutorial. I have followed all of it and a lot of magic is now become real knowledge about the machinery that makes all deep learning to work. Also, thank you very much for your courses in Kaggle also. You deserve my money so your books will be in my virtual shelves soon.
Do you have some license strategy for your code? I would love to use it as a starting point, so if I can reuse it with a Open Source license (MIT, Apache or GPL) I would start from it.
Thanks, I’m glad it helped.
Yes, you can use the code in your project, I explain more here:
https://machinelearningmastery.com/faq/single-faq/can-i-use-your-code-in-my-own-project
In the section where you build the baseline model, the evaluate_model() function takes the model as a parameter. Doing so causes the same model to be used for all the k-fold splits, each time training over the weights created from the previous iteration. This is also the reason why we see a steady increase in the accuracy of the model over the 5 iterations.
As per my understanding of k-fold cross validation, the model should be freshly trained each time with that iteration’s train and test. Otherwise, we risk over fitting as the model eventually ends up learning from all of the data provided and do no really train the model 5 times.
Ouch, that looks like a bug! Thanks.
I’ll schedule time to fix it up.
Understandably, fixing the bug will get rid of the 99%+ accuracies obtained on k-fold validation. However, the obtained accuracy is now in line with accuracy obtained on the test set.
As you had mentioned, I added another convolution layer, and added 40% dropout to the dense layer as well. Doing so pushed the accuracy to 92.3%.
Changing the padding from valid to same caused a slight dip in the accuracy as opposed to increasing it (90.81% -> 90.05%). While I did not try to reproduce this result by running it again, it seems to me that this does not appreciably change the outcome.
Nice work!
Update: I have corrected the bug in the tutorial and updated the results.
is there any link for full code ..Thanks
Yes, the full code is provided directly in the tutorial above.
What problem are you having exactly?
I have a huge problem recently.
I have CNN and MLP models, which start to overfit in strange way;
during the training, the validation loss increases but validation accuracy increases as well.
I end up with models that have better score than corresponding non-overfit models…
Is that desired behaviour ? In the end the prediction is important, not the loss function result…
That’s interesting.
This can happen if the dataset is small and not representative, or if the problem is trivial.
Maybe this post will help:
https://machinelearningmastery.com/learning-curves-for-diagnosing-machine-learning-model-performance/
Thanks, buddy for such a nice post.
I have a question, can you add some other models for the recommendation . like after classification using some customized algorithm for recommendations on using single input.
Thanks
Sorry, I don’t understand, can you please elaborate?
Hi
Can you merge this code to your model like for prediction, can you add knn, cosine or other modules.
Thanks
https://github.com/samgrassi01/Cosine-Similarity-Classifier/blob/master/Superior%20K-NN%20Classifier.ipynb
Sorry, I don’t have the capacity to merge code for you.
In the evaluate_model section, the statement ‘model = define_model()’ should be moved to where before ‘for train_ix, test_ix in kfold.split(dataX)’. Therefore, the model accuracy should be as:
> 90.842
> 94.500
> 97.117
> 99.317
> 99.908
No, the model must be re-defined (re-initialized) each time it is evaluated.
Otherwise the evaluation will continue on from the last set of weights.
Hi Jason, if you are redefining the model for each iteration, then which model is used on the final test data? I only see a model.fit for the whole training data but which model is this? should we not redefine the model, then do model.fit on the whole training data and then apply on the test data.
A final model can be fit on all data and used to make predictions on new data, more on final models here:
https://machinelearningmastery.com/train-final-machine-learning-model/
I am getting KeyError: ‘accuracy’
while executing summarize_diagnostics
I’m sorry to hear that, I have some suggestions here:
https://machinelearningmastery.com/faq/single-faq/why-does-the-code-in-the-tutorial-not-work-for-me
Hi Jason,
I think the bug is stemming from the fact that you’re using the key “accuracy” instead of “acc” in the following line of code in your ‘summarize_diagnostics()’function:
pyplot.plot(histories[i].history[‘accuracy’], color=’blue’, label=’train’)
In fact, it needs to be:
pyplot.plot(histories[i].history[‘acc’], color=’blue’, label=’train’)
Am I right ?
Because you have appended the key “acc” to your histories list in the previous function.
No, the API changed in Keras 2.3. I recommend updating to the latest version of Keras.
Hi Jason, it’s a great tutorial.I am new to machine learning but was able to get everything you described to work well. It took me 30 min to run # model with padded convolutions for the fashion mnist dataset or # model with double the filters for the fashion mnist dataset, but only a few seconds to run with final_model.h5. I am on Windows 10 and intel CPU 4 core. I tested some images from the mnist, all worked perfect. However, when I use my own images of similar items, given a black background, the accuracy is very low. Any suggestion? (I am new to machine learning as well as coding).
Thanks! Well done.
Perhaps your images are too diffrent from those used during training?
Jason, thank you for the tutorial!
One question, is it possible to use this Fashion MNIST dataset to find objects (t-shirt/dress/shoe…) in other images? For example, if I have stock photos from apparel website, then is it possible to train a neural network to find shoe in those stock photos?
Not really.
I recommend using an object recognition algorithm:
https://machinelearningmastery.com/object-recognition-with-deep-learning/
Thanks, I will go over it.
You’re welcome.
Hello Jason,
I am making a visual search engine, so that given an image I need to find images which are visually similar. I have only used a pretrained model vgg16 to generate the image vectors, and nmslib to calculate scores. The images I have are very varied in nature, from art pieces and such to product images, comices etc. I am finding that when I search for a sneaker, something unrelated can come up. Does it make sense to retrain the existing vgg16 model with these fashion images, to increase the sensitivity to fashion items, because the vgg16 may not have had enough of those images. But I am realizing that the vector of a large sized image with a lot going on (eg. model running in sneakers) can be very different than that of a 28 px training image … or I am missing some basic concept here
From your answer above (to Tarandeep) it doesnt look like the approach to take, rather as you suggest I should perhaps look to see if there are sneakers in a picture, as well as seeing if the whole picture is of a sneaker.
If you cover related topics in another post or course, any links are helpful, or looks like I should just get your book.
Thanks for any input
Sounds like a great project!
It might be interesting to try a new model trained as an autoencoder, or a classification model.
It might also be interesting to try other pre-trained models.
thanks for the tip on the auto encoder, will be trying that out.
aside from the well known pre-trained models like the ones included in keras, do you know of others which could be worthwhile. I have found that the general model they have over at Clarifai.com is very good, but its an expensive API with our volume. I doubt if anything that good is available even on a commercial basis (aside from an API service)
more generally, how would you suggest I can combine the image vector data with some other meta data, when doing the nearest neighbor search. The meta data can be a user assigned category for the image, as well as the objects extracted. Do you cover something like this in your books?
That sounds like a good approach.
No, I don’t have this exact problem in any tutorials.
Fashion MNIST Cnn in pycharm?
I recommend not using an IDE, use a text editor instead, here’s why:
https://machinelearningmastery.com/faq/single-faq/why-dont-use-or-recommend-notebooks
I am trying to train it via Transfer Learing (VGG 16) , the input image size is 28×28, how can i change it to 224,224,64? or can i somehow change size of input layer of vgg16 model. I cannot use model.fit_generator_from directory as it needs path of the training image and test image…how ever i have directly accessed data from keras and with the help of load_data I have accessed it? Could you please help me how can i change the shape of input images or can modify input layer of vgg16?
Yes, you can change the input shape of a pre-trained vgg model, perhaps start here:
https://machinelearningmastery.com/how-to-use-transfer-learning-when-developing-convolutional-neural-network-models/
In this which CNN model are you using? (LeNet,ResNet,VGG Net…..)
A custom CNN model developed from scratch.
The model seems to be performing very bad on the images I download from the net which are not in grayscale format. They are coloured images and I need the model to be to classify those images..can you help me?
The model is designed for grayscale images.
If working with color images, you will need a different model, for example:
https://machinelearningmastery.com/how-to-develop-a-cnn-from-scratch-for-cifar-10-photo-classification/
Hi, Jason,
Thank you for your post!
There is probably a typo when you print the model accuracy: it is not in percentage, so no need to *100. Here is my modification of the function:
# summarize model performance
def summarize_performance(scores):
# print summary
print(f”Accuracy: mean={np.mean(scores): .3f} std={np.std(scores): .3f}, n={len(scores)}”)
# box and whisker plots of results
pyplot.boxplot(scores)
pyplot.title(“Model Accuracy on Validation Set”)
pyplot.ylabel(“Accuracy”)
pyplot.show()
We are printing classification accuracy which is a ratio and can be multiplied by 100 to give a percentage.
Why do you say there is a typo?
Great tutorial , as always from you Jason.
I had a problem with the load_img() function . I consistently got error message :’ ValueError: cannot reshape array of size 2352 into shape (28,28,1)’ , despite setting grayscale=’True’ and also using color_mode=’gray’ which seems to be the updated setting . This means , the image has a shape [28,28,3] when loading
Finally , I had to use img=img[:,:,0] after converting it into an array and it worked . Not sure where I had gone wrong and if any else faced the same issue.
Code reproduced below
new= img_to_array(new)
new=new[:,:,0]
new= new.reshape(28, 28, 1)
new= new.astype(‘float32’) / 255.0
Also , i tried external grayscale images picked up randomly from google images i.e pullovers , sneakers etc . The model failed miserably in predicting these images .
I’m sorry to hear that, this may help:
https://machinelearningmastery.com/faq/single-faq/why-does-the-code-in-the-tutorial-not-work-for-me
Great Tutorial,
Will you please help me to do this using RNN architecture.
RNN is in appropriate for this dataset, see this:
https://machinelearningmastery.com/when-to-use-mlp-cnn-and-rnn-neural-networks/
I’m working on a fashion dresses module, I would like to use the fashion mnist dataset weights in my module and use it with some architecture like resnet or inceptionV3. I’m wondering is there any way. If yes, please help me with a bit of code, I’m new to deep learning. Any help would be much appreciated!
It should be possible, although you will have to redefine the input layer.
This will help:
https://machinelearningmastery.com/how-to-use-transfer-learning-when-developing-convolutional-neural-network-models/
inception = InceptionV3(input_shape=IMAGE_SIZE + [3], weights=’fashion_mnist’, include_top=False), will it work if I do like this?
And also fashion_mnist images are in gray scale and my dataset images are colored, will it be a problem?
I’m not able to find fashion_mnist weights and import into my module, please help.
You will have to test to find out.
Hi, why you have used the same data for validation and testing during K-Fold cross validation? Is not a big mistake ? You didnt even use 10000 images from testing set. Im right?
This is a common question that I answer here:
https://machinelearningmastery.com/faq/single-faq/why-do-you-use-the-test-dataset-as-the-validation-dataset
Hi Jason,
Thank you so much for your nice article.
Could you please guide me regarding increasing Image Sizes.. Would it be great idea to increase image sizes if accuracy is increasing? The reported accuracy of the dataset is 95% but with original size of 32. What if i get the same accuracy with resizing the images? Does this approach worth? I am using transfer learning models and some models need minimum 96 image size, so that’s the reason to increase image size… How would you consider resizing images approach?
Thank you!
You’re welcome.
This will help with resizing images:
https://machinelearningmastery.com/how-to-load-and-manipulate-images-for-deep-learning-in-python-with-pil-pillow/
Do u have a flowchart for this?
No.
Thank you for this interesting article, I want to ask you something, I want to create a model with a computer vision, where the model starts by taking a picture from the user and analyzing it and the output is evaluating the user kit out of 100, and also is the color consistency appropriate or not, I want to create this The model is also in machine learning and deep learning
As I am a beginner, what would you advise me to learn to make this model?
Perhaps you can start with an existing model for object detection and adapt it for your needs.
The model in this article does not do this ??
By writing the following code: trainX, trainY, testX, testY = dataX[train_ix], dataY[train_ix], dataX[test_ix], dataY[test_ix], history = model.fit(trainX, trainY, epochs=10, batch_size=32, validation_data=(testX, testY), verbose=0) and _, acc = model.evaluate(testX, testY, verbose=0) we are using the same dataset for validation and evaluation, am I correct? I tried this code and when I run it, I obtain identical values, was this the aim of these lines of code? Or the two datasets should be different (as I suspect)?
Yes, you’re correct. That’s why people will use train-test-validation split in your case.
Hi, just stumbled across this tutorial, thank you!
I’m running into a problem when I try to follow the tutorial, with a syntax error on pyplot.show()
for i in range(9):
… pyplot.subplot(330 + 1 + i)
… pyplot.imshow(trainX[i], cmap=pyplot.get_cmap(‘gray’))
… pyplot.show()
File “”, line 4
pyplot.show()
^
SyntaxError: invalid syntax
I’ve even copy and pasted the code but the error remains the same.
What am I doing wrong?
Hi Trent…You are very welcome! I would recommend that you type the code to avoid copy and paste issues. Additionally, you may want to try typing the code into Google Colab as well. Let us know what you find!
Hello,
I am working on a school project that requires me to compare the results obtained when I use 1, 2 and 3 hidden layers in a MLP for the Fashion MNIST. From what I understand of your code, it would be simply a question of repeating the following line twice when defining the model:
model.add(Conv2D(64, (3, 3), padding = ‘same’,activation=’relu’, kernel_initializer=’he_uniform’, input_shape=(28, 28, 1)))
Am I correct?
Thanks for the help!
Hi Bruno…Please proceed with your idea and let us know what you find.
If I want to test with an image outside of this dataset, how should I do it
Hi no_name…Once a model is trained, you may validate it with a “validation” dataset:
https://machinelearningmastery.com/difference-test-validation-datasets/
Is there any way to classify an image that lies outside the Fashion MNIST dataset? I have tried with images in the dataset and obtained relatively good results. However, with images outside the dataset, it seems that the model does not perform effectively.
When I use this model to test images within the Fashion MNIST dataset, the results are relatively accurate. However, when I use images outside the dataset, it seems that the model cannot recognize the results. Is there any way to overcome this?
Hi Jason, Thanks for this clear and concise tutorial. It helped me get clear idea on CNN models. I am working on a CNN-SNN architecture where the CNN(with 3 CNN layers) is trained on a MNIST dataset and then the middle CNN layer is replaced with SNN layer and trained again by freezing the first layer. Do you have any tutorials or articles that could help on this as I am a newbie here and couldn’t get any relevant sources in this this type of problem.
Hi Maya…You are very welcome! The following resource provides great insight into the practical application of such networks.
https://www.frontiersin.org/articles/10.3389/fnins.2022.759900/full