Machine learning predictive modeling performance is only as good as your data, and your data is only as good as the way you prepare it for modeling.
The most common approach to data preparation is to study a dataset and review the expectations of a machine learning algorithm, then carefully choose the most appropriate data preparation techniques to transform the raw data to best meet the expectations of the algorithm. This is slow, expensive, and requires a vast amount of expertise.
An alternative approach to data preparation is to apply a suite of common and commonly useful data preparation techniques to the raw data in parallel and combine the results of all of the transforms together into a single large dataset from which a model can be fit and evaluated.
This is an alternative philosophy for data preparation that treats data transforms as an approach to extract salient features from raw data to expose the structure of the problem to the learning algorithms. It requires learning algorithms that are scalable of weight input features and using those input features that are most relevant to the target that is being predicted.
This approach requires less expertise, is computationally effective compared to a full grid search of data preparation methods, and can aid in the discovery of unintuitive data preparation solutions that achieve good or best performance for a given predictive modeling problem.
In this tutorial, you will discover how to use feature extraction for data preparation with tabular data.
After completing this tutorial, you will know:
- Feature extraction provides an alternate approach to data preparation for tabular data, where all data transforms are applied in parallel to raw input data and combined together to create one large dataset.
- How to use the feature extraction method for data preparation to improve model performance over a baseline for a standard classification dataset.
- How to add feature selection to the feature extraction modeling pipeline to give a further lift in modeling performance on a standard dataset.
Kick-start your project with my new book Data Preparation for Machine Learning, including step-by-step tutorials and the Python source code files for all examples.
Let’s get started.
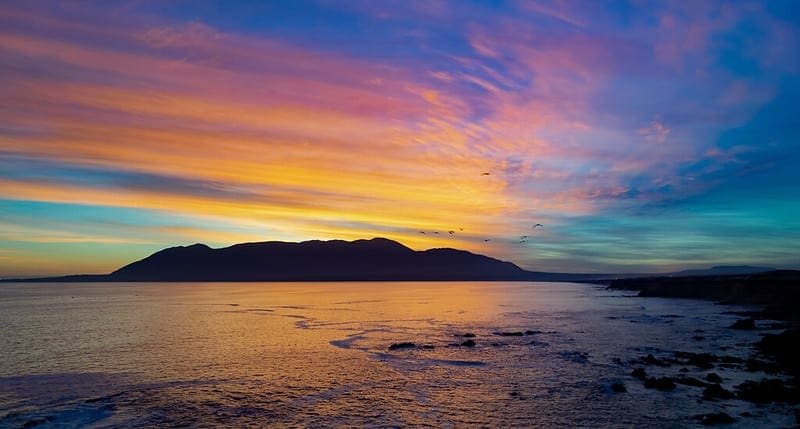
How to Use Feature Extraction on Tabular Data for Data Preparation
Photo by Nicolas Valdes, some rights reserved.
Tutorial Overview
This tutorial is divided into three parts; they are:
- Feature Extraction Technique for Data Preparation
- Dataset and Performance Baseline
- Wine Classification Dataset
- Baseline Model Performance
- Feature Extraction Approach to Data Preparation
Feature Extraction Technique for Data Preparation
Data preparation can be challenging.
The approach that is most often prescribed and followed is to analyze the dataset, review the requirements of the algorithms, and transform the raw data to best meet the expectations of the algorithms.
This can be effective, but is also slow and can require deep expertise both with data analysis and machine learning algorithms.
An alternative approach is to treat the preparation of input variables as a hyperparameter of the modeling pipeline and to tune it along with the choice of algorithm and algorithm configuration.
This too can be an effective approach exposing unintuitive solutions and requiring very little expertise, although it can be computationally expensive.
An approach that seeks a middle ground between these two approaches to data preparation is to treat the transformation of input data as a feature engineering or feature extraction procedure. This involves applying a suite of common or commonly useful data preparation techniques to the raw data, then aggregating all features together to create one large dataset, then fit and evaluate a model on this data.
The philosophy of the approach treats each data preparation technique as a transform that extracts salient features from raw data to be presented to the learning algorithm. Ideally, such transforms untangle complex relationships and compound input variables, in turn allowing the use of simpler modeling algorithms, such as linear machine learning techniques.
For lack of a better name, we will refer to this as the “Feature Engineering Method” or the “Feature Extraction Method” for configuring data preparation for a predictive modeling project.
It allows data analysis and algorithm expertise to be used in the selection of data preparation methods and allows unintuitive solutions to be found but at a much lower computational cost.
The exclusion in the number of input features can also be explicitly addressed through the use of feature selection techniques that attempt to rank order the importance or value of the vast number of extracted features and only select a small subset of the most relevant to predicting the target variable.
We can explore this approach to data preparation with a worked example.
Before we dive into a worked example, let’s first select a standard dataset and develop a baseline in performance.
Want to Get Started With Data Preparation?
Take my free 7-day email crash course now (with sample code).
Click to sign-up and also get a free PDF Ebook version of the course.
Dataset and Performance Baseline
In this section, we will first select a standard machine learning dataset and establish a baseline in performance on this dataset. This will provide the context for exploring the feature extraction method of data preparation in the next section.
Wine Classification Dataset
We will use the wine classification dataset.
This dataset has 13 input variables that describe the chemical composition of samples of wine and requires that the wine be classified as one of three types.
You can learn more about the dataset here:
No need to download the dataset as we will download it automatically as part of our worked examples.
Open the dataset and review the raw data. The first few rows of data are listed below.
We can see that it is a multi-class classification predictive modeling problem with numerical input variables, each of which has different scales.
1 2 3 4 5 6 |
14.23,1.71,2.43,15.6,127,2.8,3.06,.28,2.29,5.64,1.04,3.92,1065,1 13.2,1.78,2.14,11.2,100,2.65,2.76,.26,1.28,4.38,1.05,3.4,1050,1 13.16,2.36,2.67,18.6,101,2.8,3.24,.3,2.81,5.68,1.03,3.17,1185,1 14.37,1.95,2.5,16.8,113,3.85,3.49,.24,2.18,7.8,.86,3.45,1480,1 13.24,2.59,2.87,21,118,2.8,2.69,.39,1.82,4.32,1.04,2.93,735,1 ... |
The example loads the dataset and splits it into the input and output columns, then summarizes the data arrays.
1 2 3 4 5 6 7 8 9 10 11 12 |
# example of loading and summarizing the wine dataset from pandas import read_csv # define the location of the dataset url = 'https://raw.githubusercontent.com/jbrownlee/Datasets/master/wine.csv' # load the dataset as a data frame df = read_csv(url, header=None) # retrieve the numpy array data = df.values # split the columns into input and output variables X, y = data[:, :-1], data[:, -1] # summarize the shape of the loaded data print(X.shape, y.shape) |
Running the example, we can see that the dataset was loaded correctly and that there are 179 rows of data with 13 input variables and a single target variable.
1 |
(178, 13) (178,) |
Next, let’s evaluate a model on this dataset and establish a baseline in performance.
Baseline Model Performance
We can establish a baseline in performance on the wine classification task by evaluating a model on the raw input data.
In this case, we will evaluate a logistic regression model.
First, we can perform minimum data preparation by ensuring the input variables are numeric and that the target variable is label encoded, as expected by the scikit-learn library.
1 2 3 4 |
... # minimally prepare dataset X = X.astype('float') y = LabelEncoder().fit_transform(y.astype('str')) |
Next, we can define our predictive model.
1 2 3 |
... # define the model model = LogisticRegression(solver='liblinear') |
We will evaluate the model using the gold standard of repeated stratified k-fold cross-validation with 10 folds and three repeats.
Model performance will be evaluated using classification accuracy.
1 2 3 4 5 6 |
... model = LogisticRegression(solver='liblinear') # define the cross-validation procedure cv = RepeatedStratifiedKFold(n_splits=10, n_repeats=3, random_state=1) # evaluate model scores = cross_val_score(model, X, y, scoring='accuracy', cv=cv, n_jobs=-1) |
At the end of the run, we will report the mean and standard deviation of the accuracy scores collected across all repeats and evaluation folds.
1 2 3 |
... # report performance print('Accuracy: %.3f (%.3f)' % (mean(scores), std(scores))) |
Tying this together, the complete example of evaluating a logistic regression model on the raw wine classification dataset is listed below.
1 2 3 4 5 6 7 8 9 10 11 12 13 14 15 16 17 18 19 20 21 22 23 24 |
# baseline model performance on the wine dataset from numpy import mean from numpy import std from pandas import read_csv from sklearn.preprocessing import LabelEncoder from sklearn.model_selection import RepeatedStratifiedKFold from sklearn.model_selection import cross_val_score from sklearn.linear_model import LogisticRegression # load the dataset url = 'https://raw.githubusercontent.com/jbrownlee/Datasets/master/wine.csv' df = read_csv(url, header=None) data = df.values X, y = data[:, :-1], data[:, -1] # minimally prepare dataset X = X.astype('float') y = LabelEncoder().fit_transform(y.astype('str')) # define the model model = LogisticRegression(solver='liblinear') # define the cross-validation procedure cv = RepeatedStratifiedKFold(n_splits=10, n_repeats=3, random_state=1) # evaluate model scores = cross_val_score(model, X, y, scoring='accuracy', cv=cv, n_jobs=-1) # report performance print('Accuracy: %.3f (%.3f)' % (mean(scores), std(scores))) |
Running the example evaluates the model performance and reports the mean and standard deviation classification accuracy.
Note: Your results may vary given the stochastic nature of the algorithm or evaluation procedure, or differences in numerical precision. Consider running the example a few times and compare the average outcome.
In this case, we can see that the logistic regression model fit on the raw input data achieved the average classification accuracy of about 95.3 percent, providing a baseline in performance.
1 |
Accuracy: 0.953 (0.048) |
Next, let’s explore whether we can improve the performance using the feature extraction based approach to data preparation.
Feature Extraction Approach to Data Preparation
In this section, we can explore whether we can improve performance using the feature extraction approach to data preparation.
The first step is to select a suite of common and commonly useful data preparation techniques.
In this case, given that the input variables are numeric, we will use a range of transforms to change the scale of the input variables such as MinMaxScaler, StandardScaler, and RobustScaler, as well as transforms for chaining the distribution of the input variables such as QuantileTransformer and KBinsDiscretizer. Finally, we will also use transforms that remove linear dependencies between the input variables such as PCA and TruncatedSVD.
The FeatureUnion class can be used to define a list of transforms to perform, the results of which will be aggregated together, i.e. unioned. This will create a new dataset that has a vast number of columns.
An estimate of the number of columns would be 13 input variables times five transforms or 65 plus the 14 columns output from the PCA and SVD dimensionality reduction methods, to give a total of about 79 features.
1 2 3 4 5 6 7 8 9 10 11 12 |
... # transforms for the feature union transforms = list() transforms.append(('mms', MinMaxScaler())) transforms.append(('ss', StandardScaler())) transforms.append(('rs', RobustScaler())) transforms.append(('qt', QuantileTransformer(n_quantiles=100, output_distribution='normal'))) transforms.append(('kbd', KBinsDiscretizer(n_bins=10, encode='ordinal', strategy='uniform'))) transforms.append(('pca', PCA(n_components=7))) transforms.append(('svd', TruncatedSVD(n_components=7))) # create the feature union fu = FeatureUnion(transforms) |
We can then create a modeling Pipeline with the FeatureUnion as the first step and the logistic regression model as the final step.
1 2 3 4 5 6 7 8 |
... # define the model model = LogisticRegression(solver='liblinear') # define the pipeline steps = list() steps.append(('fu', fu)) steps.append(('m', model)) pipeline = Pipeline(steps=steps) |
The pipeline can then be evaluated using repeated stratified k-fold cross-validation as before.
Tying this together, the complete example is listed below.
1 2 3 4 5 6 7 8 9 10 11 12 13 14 15 16 17 18 19 20 21 22 23 24 25 26 27 28 29 30 31 32 33 34 35 36 37 38 39 40 41 42 43 44 45 46 47 48 49 |
# data preparation as feature engineering for wine dataset from numpy import mean from numpy import std from pandas import read_csv from sklearn.model_selection import RepeatedStratifiedKFold from sklearn.model_selection import cross_val_score from sklearn.linear_model import LogisticRegression from sklearn.pipeline import Pipeline from sklearn.pipeline import FeatureUnion from sklearn.preprocessing import LabelEncoder from sklearn.preprocessing import MinMaxScaler from sklearn.preprocessing import StandardScaler from sklearn.preprocessing import RobustScaler from sklearn.preprocessing import QuantileTransformer from sklearn.preprocessing import KBinsDiscretizer from sklearn.decomposition import PCA from sklearn.decomposition import TruncatedSVD # load the dataset url = 'https://raw.githubusercontent.com/jbrownlee/Datasets/master/wine.csv' df = read_csv(url, header=None) data = df.values X, y = data[:, :-1], data[:, -1] # minimally prepare dataset X = X.astype('float') y = LabelEncoder().fit_transform(y.astype('str')) # transforms for the feature union transforms = list() transforms.append(('mms', MinMaxScaler())) transforms.append(('ss', StandardScaler())) transforms.append(('rs', RobustScaler())) transforms.append(('qt', QuantileTransformer(n_quantiles=100, output_distribution='normal'))) transforms.append(('kbd', KBinsDiscretizer(n_bins=10, encode='ordinal', strategy='uniform'))) transforms.append(('pca', PCA(n_components=7))) transforms.append(('svd', TruncatedSVD(n_components=7))) # create the feature union fu = FeatureUnion(transforms) # define the model model = LogisticRegression(solver='liblinear') # define the pipeline steps = list() steps.append(('fu', fu)) steps.append(('m', model)) pipeline = Pipeline(steps=steps) # define the cross-validation procedure cv = RepeatedStratifiedKFold(n_splits=10, n_repeats=3, random_state=1) # evaluate model scores = cross_val_score(pipeline, X, y, scoring='accuracy', cv=cv, n_jobs=-1) # report performance print('Accuracy: %.3f (%.3f)' % (mean(scores), std(scores))) |
Running the example evaluates the model performance and reports the mean and standard deviation classification accuracy.
Note: Your results may vary given the stochastic nature of the algorithm or evaluation procedure, or differences in numerical precision. Consider running the example a few times and compare the average outcome.
In this case, we can see a lift in performance over the baseline performance, achieving a mean classification accuracy of about 96.8 percent as compared to 95.3 percent in the previous section.
1 |
Accuracy: 0.968 (0.037) |
Try adding more data preparation methods to the FeatureUnion to see if you can improve the performance.
Can you get better results?
Let me know what you discover in the comments below.
We can also use feature selection to reduce the approximately 80 extracted features down to a subset of those that are most relevant to the model. In addition to reducing the complexity of the model, it can also result in a lift in performance by removing irrelevant and redundant input features.
In this case, we will use the Recursive Feature Elimination, or RFE, technique for feature selection and configure it to select the 15 most relevant features.
1 2 3 |
... # define the feature selection rfe = RFE(estimator=LogisticRegression(solver='liblinear'), n_features_to_select=15) |
We can then add the RFE feature selection to the modeling pipeline after the FeatureUnion and before the LogisticRegression algorithm.
1 2 3 4 5 6 7 |
... # define the pipeline steps = list() steps.append(('fu', fu)) steps.append(('rfe', rfe)) steps.append(('m', model)) pipeline = Pipeline(steps=steps) |
Tying this together, the complete example of the feature selection data preparation method with feature selection is listed below.
1 2 3 4 5 6 7 8 9 10 11 12 13 14 15 16 17 18 19 20 21 22 23 24 25 26 27 28 29 30 31 32 33 34 35 36 37 38 39 40 41 42 43 44 45 46 47 48 49 50 51 52 53 |
# data preparation as feature engineering with feature selection for wine dataset from numpy import mean from numpy import std from pandas import read_csv from sklearn.model_selection import RepeatedStratifiedKFold from sklearn.model_selection import cross_val_score from sklearn.linear_model import LogisticRegression from sklearn.pipeline import Pipeline from sklearn.pipeline import FeatureUnion from sklearn.preprocessing import LabelEncoder from sklearn.preprocessing import MinMaxScaler from sklearn.preprocessing import StandardScaler from sklearn.preprocessing import RobustScaler from sklearn.preprocessing import QuantileTransformer from sklearn.preprocessing import KBinsDiscretizer from sklearn.feature_selection import RFE from sklearn.decomposition import PCA from sklearn.decomposition import TruncatedSVD # load the dataset url = 'https://raw.githubusercontent.com/jbrownlee/Datasets/master/wine.csv' df = read_csv(url, header=None) data = df.values X, y = data[:, :-1], data[:, -1] # minimally prepare dataset X = X.astype('float') y = LabelEncoder().fit_transform(y.astype('str')) # transforms for the feature union transforms = list() transforms.append(('mms', MinMaxScaler())) transforms.append(('ss', StandardScaler())) transforms.append(('rs', RobustScaler())) transforms.append(('qt', QuantileTransformer(n_quantiles=100, output_distribution='normal'))) transforms.append(('kbd', KBinsDiscretizer(n_bins=10, encode='ordinal', strategy='uniform'))) transforms.append(('pca', PCA(n_components=7))) transforms.append(('svd', TruncatedSVD(n_components=7))) # create the feature union fu = FeatureUnion(transforms) # define the feature selection rfe = RFE(estimator=LogisticRegression(solver='liblinear'), n_features_to_select=15) # define the model model = LogisticRegression(solver='liblinear') # define the pipeline steps = list() steps.append(('fu', fu)) steps.append(('rfe', rfe)) steps.append(('m', model)) pipeline = Pipeline(steps=steps) # define the cross-validation procedure cv = RepeatedStratifiedKFold(n_splits=10, n_repeats=3, random_state=1) # evaluate model scores = cross_val_score(pipeline, X, y, scoring='accuracy', cv=cv, n_jobs=-1) # report performance print('Accuracy: %.3f (%.3f)' % (mean(scores), std(scores))) |
Running the example evaluates the model performance and reports the mean and standard deviation classification accuracy.
Note: Your results may vary given the stochastic nature of the algorithm or evaluation procedure, or differences in numerical precision. Consider running the example a few times and compare the average outcome.
Again, we can see a further lift in performance from 96.8 percent with all extracted features to about 98.9 with feature selection used prior to modeling.
1 |
Accuracy: 0.989 (0.022) |
Can you achieve better performance with a different feature selection technique or with more or fewer selected features?
Let me know what you discover in the comments below.
Further Reading
This section provides more resources on the topic if you are looking to go deeper.
Related Tutorials
Books
APIs
Summary
In this tutorial, you discovered how to use feature extraction for data preparation with tabular data.
Specifically, you learned:
- Feature extraction provides an alternate approach to data preparation for tabular data, where all data transforms are applied in parallel to raw input data and combined together to create one large dataset.
- How to use the feature extraction method for data preparation to improve model performance over a baseline for a standard classification dataset.
- How to add feature selection to the feature extraction modeling pipeline to give a further lift in modeling performance on a standard dataset.
Do you have any questions?
Ask your questions in the comments below and I will do my best to answer.
Hi Jason! Big fan of yours.
Thanks for the article. I learnt few new libraries through it. I have a question instead of doing both feature extraction and the selection, is there anyway through which we can understand what to use by looking at the data or some stats at the very beginning ?
And can we use feature selection separately or do we need to use it with the large dataset created by feature extraction methods ? How to understand that ?
Thanks!
Yes, sometimes looking at the data can suggest methods to use, see this:
https://machinelearningmastery.com/what-is-data-preparation-in-machine-learning/
Yes, you can use feature selection separately:
https://machinelearningmastery.com/feature-selection-with-real-and-categorical-data/
Nice. Thank you Jason. Is there a function that also selects the interaction of columns as well? Or would you have to create all those new columns first? For instance generate interaction features by multiplying features together to get a new feature. Given how many possible relevent combinations there might be, it be would nice if there was a function that could automate that.
Nice explanation. We have been using Convolutional Nerual Network and Auto Encoder. Can we embed this feature extraction for deep learning.
Sure.
You would have to create integration features and then select, this can help you create them:
https://machinelearningmastery.com/polynomial-features-transforms-for-machine-learning/
Hi Jason, good work!
I am wonder what is your opinion about tsfresh module?
I have not used it, sorry.
Hatta, Thank you for the heads-up on the tsfresh module. Looks very useful.
Another great post – thank you very much. I would just add that it make sense to check if the selected features indeed makes sense for you. Sometimes it allows you to find a bug in you code and sometimes to learn something new about your data
Thanks.
Great tip!
Great article.
Let’s say I split data into train/test at the beggining.
How would you make a prediction on new data ?
How do you make a model using all those steps and pipeline?
Thanks a lot!!
You can fit the pipeline on all data and call pipleine.predict() to make a prediction on new data.
Jason, if you wanted to run more than one model, i.e logistic regression and Random Forest, using the same features and preprocessing steps, how would you do that ?
Do you have an example?
Thanks in advance,
Maybe voting:
https://machinelearningmastery.com/voting-ensembles-with-python/
Or, maybe a stacking model:
https://machinelearningmastery.com/stacking-ensemble-machine-learning-with-python/
Jason, sorry,
I meant to also try another classifier apart from logistic regression.
You made a CV of a RL, is it possible to add a few lines more and try some other models to see if they make better predictions ?
Not stacking them but checking wich one is the best.
Thanks
Yes, you can change the model to anything you like.
Good day, please I’m new to machine learning and I want to learn and have research interest. What are the requirements to start ? Thank you
No requirements other than being a developer, start here:
https://machinelearningmastery.com/start-here/#getstarted
I wonder why do we need to label encode the target variable which was already numeric (with value 1,2 and 3).
Good default practice, perhaps. Also, ensure the integer values start at zero in case they don’t.
Jason,
Great book, “Data Preparation For ML”. I read the entire book in a day – I could not put it down! Question, can you direct me to a simple example that utilizes FeatureUnion, ColumnTransformer, and the TransformedTargetRegressor together? I am assuming that the TransformedTargetRegressor only operates on the target variable(s) and not the features themselves so I would need to do that before the TransformedTargetRegressor? Is there a method to discover the final selected RFE?
Very impressive!
You can use RFE in isolation and report the features found by the method. It would be a mess though.
Un excellent post – merci beaucoup pour vos articles.
En fait je voulais savoir quel est l’intérêt d’utiliser deux techniques de normalisations “MinMaxScaler” et un “StandarScaler” sur un même ensemble de données ?
Thanks!
Good question, it is possible that vars with a gaussian variable may better respond to standardization than normalization, but you’re probably right that one scaling method would be sufficient.
Merci de votre réponse.
Dans ce cas serait-il pas plus rigoureux de faire un test d’hypothèse statistique sur les variables afin de déterminer les variables qui ont une distribution gaussienne et en appliqué une standardisation à ces variables.
Sometimes, and sometimes we get better results when we break the rules/expectations.
I think this tutorial might be interesting to you:
https://machinelearningmastery.com/selectively-scale-numerical-input-variables-for-machine-learning/
Merci
Je suis tout à fait d’accord avec vous , on obtient de meilleurs résultats en enfreignant les règles.
Ton article sur la “selectively scale” est Top!!! Merci encore Jason.
Thanks!
sir is we apply GAN based data augmentation at test time to pre-trained AlexNet
You can if you want.
which feature selection method is best for analysis weblog data
This is a common question that I answer here:
https://machinelearningmastery.com/faq/single-faq/what-feature-selection-method-should-i-use