What To Do If Model Test Results Are Worse than Training.
The procedure when evaluating machine learning models is to fit and evaluate them on training data, then verify that the model has good skill on a held-back test dataset.
Often, you will get a very promising performance when evaluating the model on the training dataset and poor performance when evaluating the model on the test set.
In this post, you will discover techniques and issues to consider when you encounter this common problem.
After reading this post, you will know:
- The problem of model performance mismatch that may occur when evaluating machine learning algorithms.
- The causes of overfitting, under-representative data samples, and stochastic algorithms.
- Ways to harden your test harness to avoid the problem in the first place.
This post was based on a reader question; thanks! Keep the questions coming!
Let’s get started.
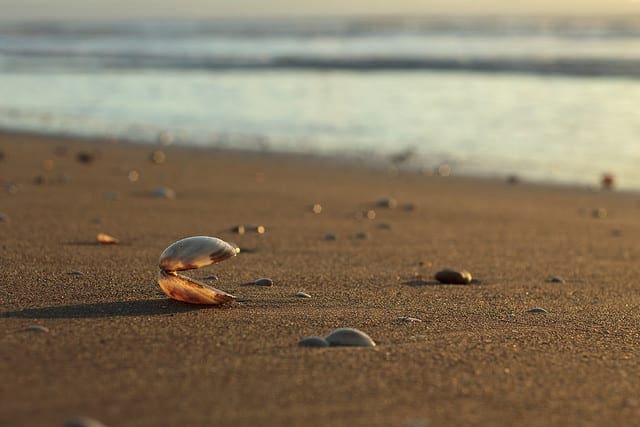
The Model Performance Mismatch Problem (and what to do about it)
Photo by Arapaoa Moffat, some rights reserved.
Overview
This post is divided into 4 parts; they are:
- Model Evaluation
- Model Performance Mismatch
- Possible Causes and Remedies
- More Robust Test Harness
Model Evaluation
When developing a model for a predictive modeling problem, you need a test harness.
The test harness defines how the sample of data from the domain will be used to evaluate and compare candidate models for your predictive modeling problem.
There are many ways to structure a test harness, and no single best way for all projects.
One popular approach is to use a portion of data for fitting and tuning the model and a portion for providing an objective estimate of the skill of the tuned model on out-of-sample data.
The data sample is split into a training and test dataset. The model is evaluated on the training dataset using a resampling method such as k-fold cross-validation, and the set itself may be further divided into a validation dataset used to tune the hyperparameters of the model.
The test set is held back and used to evaluate and compare tuned models.
For more on training, validation, and test sets, see the post:
Model Performance Mismatch
The resampling method will give you an estimate of the skill of your model on unseen data by using the training dataset.
The test dataset provides a second data point and ideally an objective idea of how well the model is expected to perform, corroborating the estimated model skill.
What if the estimate of model skill on the training dataset does not match the skill of the model on the test dataset?
The scores will not match in general. We do expect some differences because some small overfitting of the training dataset is inevitable given hyperparameter tuning, making the training scores optimistic.
But what if the difference is worryingly large?
- Which score do you trust?
- Can you still compare models using the test dataset?
- Is the model tuning process invalidated?
It is a challenging and very common situation in applied machine learning.
We can call this concern the “model performance mismatch” problem.
Note: ideas of “large differences” in model performance are relative to your chosen performance measures, datasets, and models. We cannot talk objectively about differences in general, only relative differences that you must interpret yourself.
Possible Causes and Remedies
There are many possible causes for the model performance mismatch problem.
Ultimately, your goal is to have a test harness that you know allows you to make good decisions regarding which model and model configuration to use as a final model.
In this section, we will look at some possible causes, diagnostics, and techniques you can use to investigate the problem.
Let’s look at three main areas: model overfitting, the quality of the data sample, and the stochastic nature of the learning algorithm.
1. Model Overfitting
Perhaps the most common cause is that you have overfit the training data.
You have hit upon a model, a set of model hyperparameters, a view of the data, or a combination of these elements and more that just so happens to give a good skill estimate on the training dataset.
The use of k-fold cross-validation will help to some degree. The use of tuning models with a separate dataset too will help. Nevertheless, it is possible to keep pushing and overfit on the training dataset.
If this is the case, the test skill may be more representative of the true skill of the chosen model and configuration.
One simple (but not easy) way to diagnose whether you have overfit the training dataset, is to get another data point on model skill. Evaluate the chosen model on another set of data. For example, some ideas to try include:
- Try a k-fold cross-validation evaluation of the model on the test dataset.
- Try a fit of the model on the training dataset and an evaluation on the test and a new data sample.
If you’re overfit, you have options.
- Perhaps you can scrap your current training dataset and collect a new training dataset.
- Perhaps you can re-split your sample into train/test in a softer approach to getting a new training dataset.
I would suggest that the results that you have obtained to-date are suspect and should be re-considered. Especially those where you may have spent too long tuning.
Overfitting may be the ultimate cause for the discrepancy in model scores, though it may not be the area to attack first.
2. Unrepresentative Data Sample
It is possible that your training or test datasets are an unrepresentative sample of data from the domain.
This means that the sample size is too small or the examples in the sample do not effectively “cover” the cases observed in the broader domain.
This can be obvious to spot if you see noisy model performance results. For example:
- A large variance on cross-validation scores.
- A large variance on similar model types on the test dataset.
In addition, you will see the discrepancy between train and test scores.
Another good second test is to check summary statistics for each variable on the train and test sets, and ideally on the cross-validation folds. You are looking for a large variance in sample means and standard deviation.
The remedy is often to get a larger and more representative sample of data from the domain. Alternately, to use more discriminating methods in preparing the data sample and splits. Think stratified k-fold cross validation, but applied to input variables in an attempt to maintain population means and standard deviations for real-valued variables in addition to the distribution of categorical variables.
Often when I see overfitting on a project, it is because the test harness is not as robust as it should be, not because of hill climbing the test dataset.
3. Stochastic Algorithm
It is possible that you are seeing a discrepancy in model scores because of the stochastic nature of the algorithm.
Many machine learning algorithms involve a stochastic component. For example, the random initial weights in a neural network, the shuffling of data and in turn the gradient updates in stochastic gradient descent, and much more.
This means, that each time the same algorithm is run on the same data, different sequences of random numbers are used and, in turn, a different model with different skill will result.
You can learn more about this in the post:
This issue can be seen by the variance in model skill scores from cross-validation, much like having an unrepresentative data sample.
The difference here is that the variance can be cleared up by repeating the model evaluation process, e.g. cross-validation, in order to control for the randomness in training the model.
This is often called the multiple repeats k-fold cross-validation and is used for neural networks and stochastic optimization algorithms, when resources permit.
I have more on this approach to evaluating models in the post:
More Robust Test Harness
A lot of these problems can be addressed early by designing a robust test harness and then gathering evidence to demonstrate that indeed your test harness is robust.
This might include running experiments before you get started evaluating models for real. Experiments such:
- A sensitivity analysis of train/test splits.
- A sensitivity analysis of k values for cross-validation.
- A sensitivity analysis of a given model’s behavior.
- A sensitivity analysis on the number of repeats.
On this last point, see the post:
You are looking for:
- Low variance and consistent mean in evaluation scores between tests in a cross-validation.
- Correlated population means between model scores on train and test sets.
Use statistical tools like standard error and significance tests if needed.
Use a modern and un-tuned model that performs well in general for such testing, such as random forest.
- If you discover a difference in skill scores between training and test sets, and it is consistent, that may be fine. You know what to expect.
- If you measure a variance in mean skill scores within a given test, you have error bars you can use to interpret the results.
I would go so far as to say that without a robust test harness, the results you achieve will be a mess. You will not be able to effectively interpret them. There will be an element of risk (or fraud, if you’re an academic) in the presentation of the outcomes from a fragile test harness. And reproducibility/robustness is a massive problem in numerical fields like applied machine learning.
Finally, avoid using the test dataset too much. Once you have strong evidence that your harness is robust, do not touch the test dataset until it comes time for final model selection.
Further Reading
This section provides more resources on the topic if you are looking to go deeper.
- What is the Difference Between Test and Validation Datasets?
- Embrace Randomness in Machine Learning
- Estimate the Number of Experiment Repeats for Stochastic Machine Learning Algorithms
- How to Evaluate the Skill of Deep Learning Models
Summary
In this post, you discovered the model performance mismatch problem where model performance differs greatly between training and test sets, and techniques to diagnose and address the issue.
Specifically, you learned:
- The problem of model performance mismatch that may occur when evaluating machine learning algorithms.
- The causes of overfitting, under-representative data samples, and stochastic algorithms.
- Ways to harden your test harness to avoid the problem in the first place.
Do you have any questions?
Ask your questions in the comments below and I will do my best to answer.
Thanks again for the nice post! I learn new things whenever I read your blogs.
I have basic question here – Is there a way to check 100% coverage of training data before stratified k-fold cross validation? 100% coverage means training sample/data that will have all possible combinations/data/values of features to be considered for model, or is it something domain expert should confirm?
Normally we do not seek 100% coverage, instead we seek a statistically representative sample.
We can explore whether we achieve this using summary statistics.
Thanks for the nice post. Can I have a question please:
I am developing my model prediction using both plsr package (partial least square) and h2o package (deep machine learning). From the original data set, I split 80% for model training through 10-fold CV and retain 20% for testing. I repeat this 10 time (i.e. outer loop CV) and each time the data is shuffled before split into training (80%) and testing (20%). My observation is that the Rcv reported by the pls model is always higher than that of machine learning. However, on the outer loop, when I use the best model obtained during cross validation to predict the test data, the thing is opposite: the r-squared of machine learning is better than that of pls for all 10 repeats. Can I conclude that the CV of pls possibly overfits the data?
Thanks, Phuong
I would change one thing.
Discard all models trained during cross validation, then train a model on all training data and evaluate it on the hold out test set.
Hi
I trained a model and scores were consistent with train test and validation set but it did not give expected out put on new dataset.
Is this commonly happens ?
is consistent scores between train test and validation is not validation process?
What could the reason for this type behavior?
Regards
Anil kumar
Yes, very common.
Perhaps the model or data prep was not appropriate.
Perhaps the dataset is too small or the test set is too small.
Hey Jason,
I made a model with some 15 features and when made their dummies as their many features with categorical variables after which my model before training took input of around 680 features,
When I tested on a few new data points to predict the rate from my model it showed an error of
“Number of features of the model must match the input. Model n_features is 680 and input n_features is 41”
What should I do in this situation?
Before I tried managing my variables with one hot encoding, I got the result but they were absurd as they manage variables and number them alphabetically.
Can you suggest me a way out of it?
The input to the model at inference time must undergo the same preparation as the training data.
Hi Jason,
I developed nlp classification model,
But I found issue:
First of all I run the training process on all data and got model and probabilities per object,
after that I took one object from base data and execute the model on it,
I expected to get the same probabilities outputs but there are difference,
Note –
That for each run I got the same results every time,
The difference is between training and useing with model,
Thanks in advance 🙂
Perhaps your model has overfit the training data?
Perhaps some of these methods will help:
https://machinelearningmastery.com/introduction-to-regularization-to-reduce-overfitting-and-improve-generalization-error/
Thank you for your response,
If the model is overfitting, Is it can to be that we will get other probabilities for same data?
What do you mean exactly, can you elaborate?
Thank you again,
I mean to ask , that in case that my model is overfitting, One of symptom is the situation what I described, that I got other probabilities for same data
When I build model and when I am using with this?
Sorry, I don’t follow, can you elaborate please?
Note- that the data is same ,nothing change it
Hi Jason,
Thank you a lot that you try to help,
I explain you again my issue:
I developed NLP ML algorithim and got strange situation :
I build model with all data,
Then take one of records (the data wasnt changed) and run the model on it, and got different results,
Can you know something about this situation?
Have you ever encountered such a thing?
Thank you!!
What do you mean you took one record and ran the model on it?
Retrained the model?
Made a prediction?
Made a prediction,for test the model
Thank you
Perhaps the model was a poor fit or overfit?
Hi Jason,
I would really appreciate if you can provide some insights on out of time validations.
I have built a model to predict the users that will make a purchase in future using the historical data. My training and test data have the same time period and i want to make predictions for the future time. I am getting an accuracy of around 80 percent on test data but the real time performance is really poor i.e. around 50 percent.
My intuition says that train, validation and test data should have different time period to get the good predictions.
Perhaps use the models to make a forecast on out of sample data where the real values are available or can be acquired.
Then compare each to see what type of errors the model is making.
Hello and thank you for your posts, they really help in understanding machine learning.
Please i have a question:
i am trying to compare different machine learning techniques: ANN, KNN, Random Forest
in my study i am doing two phases: the training phase and the prediction phase
in the training phase,i am using a dataset, but in the prediction phase i use a different dataset. both datasets are the same in term of features, but different in term of instances.
in the training phase, i apply 10 fold cross validation using ANN,KNN, random forest, then i extract the confusion matrix to calculate the Accuracy, Sensitivity and Specificity, and i save the model.
in the prediction phase, i use the second dataset, but instead of applying one of the techniques, i apply the saved model on the dataset, and then i calculate the same performance measures.
i found that, the results of the prediction phase are higher than the training phase.
for example: for random forest:
in the training phase i found an accuracy of 74.41%, sensitivity of 72.26%, specificity of 76.25%
in he prediction phase, i found an accuracy of 99.30%, sensitivity of 98.33%, specificity of 100%
is this normal ? i couldn’t interpret the results, my supervisor told me that the prediction phase should be lower than the training phase, and i am getting confused.
Thank you very much
Yes, you should expect some variance in the result.
I recommend using repeated k-fold cross validation for estimating model performance on a dataset and instead of reporting one number, report a mean and standard deviation – e.g. summarize the distribution of expected accuracy scores.
Thank you sir for your answer. but i am not quite sure that i understand it very well. do you mean by yes that my results are correct or not correct. i used 10 folds cross validation to estimate the model performance. I would be grateful if you provide additional explanations or a link to some posts in your website or keywords to look after to address my problem.
Sorry, I was confirming your finding. It is to be expected.
The solution is to report the distribution of results, not a point value.
This might help to make the ideas more concrete:
https://machinelearningmastery.com/evaluate-skill-deep-learning-models/
And this:
https://machinelearningmastery.com/randomness-in-machine-learning/
Thank you very much sir for your help.
You’re welcome.
Hi Jason,
I have a scenario where target variable is biased (0 – 90%, 1 – 10%). I used ADASYN & SMOTE to make balance target variable. I build model and confusion matrices values are coming like this:
Precison – 96%
Recall – 97%
F1-Score – 97%
Accuracy – 97%
When I used model on live data, Recall is coming 25% (72% reduction).
What can be the possible reason for this?
Any other method that can be used on imbalanced data?
Any possible solution so that model can work on live data.
Perhaps pick one metric to focus on, e.g. F1 or Fbeta.
Perhaps explore cost-sensitive models?
hello,
i trained SVM, Tree, ensemble tree and ANN models for both classification and regression in this i got ANN and SVM performs well but ANN performs bit better than SVM. is it any reasons for this and how can i justify ANN is better rather than error evaluation method. any specific reasons why ANN performs well.
We don’t have good theories about why one algorithm performs better than another on a given dataset.
The best we can do is use controlled experiments to collect statistical evidence.
Thanks for your response, i followed the instruction
Well done.
Is it possible to get any examples for Hello,
controlled experiments to collect statistical evidence to find model performance. Because I am not getting about this.
Please do the needful.
Yes, there are hundreds of examples of controlled experiments on this blog, use the search feature.
Hi Jason, I got a question here. Suppose due to lack of Data, my training/test set is not a good representative of my future implementation set. Is it wise to align the training/test Data such that it looks like the implementation set by over/under-sampling?
For example, in my implementation set, I know I am dealing with 80% dogs and 20% cats, but I don’t have the adequate training Data (say 15% dogs, 80% cats and 5% birds)?
Can you please provide me any reference of a scholarly article which deals with such cases.
If you have few examples of some classes, perhaps try using data oversampling on the training dataset from a fair split of the original dataset.
Hi Sir, I got a question. How can I be sure that the procedure that I am following is the correct one? Following the steps for building a machine learning model, we start by analyzing the data and then by prepare that data.
In the data preparation, we have various options. For example, if the data have missing values, I can choose to remove those values or replace them (among other techniques). How can I be sure what is the “correct” technique to follow? I mean I can always use an algorithm for getting an initial accuracy and then I can compare the changes that I will do to that accuracy. However, can I rely on that accuracy? The same happens with feature selection. I cannot be sure among all the techniques witch one is the best.
The question is the same if we talk about choosing a model and then make the hyper parametrization of that model. How can I be sure that the model that I choose is the correct one? Because without the hyperparameters tunning can be better for one specific model but after the hyperparameters tunning(in all of them) I can have better accuracy in another one. For example, between SVM and KNN without tunning the accuracy is better in SVM, so I will choose that one and then make the tunning. However, if I try tunning on both models maybe KNN will be better than SVM.
In short, my question is, they’re a lot of possible combinations between all the steps to build a machine learning model. I know its practically impossible to test all these combinations (computation power, and so on), however, it is correct to rely on an “ephemeral” accuracy? Or there a “correct” procedure to follow?
Thanks in advance, and sorry for my English because it’s not my maternal language
You cannot be sure.
But you can collect evidence that the results are “good” or “good enough” relative to a naive approach.
My best advice is to test many different data preparation methods, and many different modeling methods.
This might help:
https://machinelearningmastery.com/faq/single-faq/how-to-know-if-a-model-has-good-performance
Hi Jason,
This post was great! I have a model performance mismatch, and this post helped me to understand what can I do.
This is very important for me because this is my first model for work.
I found that:
– I overfitted the decision tree based training model, so I realized I had to prune it
– I need more data, because the test data seem to be in a different distribution than the training data. When I plot a 2D principal components of both training and test data, they look in different and separate clouds of data points
I expect to have more data soon to confirm this issue.
Thank you very much
Thanks!
Nice work.
Hello,
Sorry for the late response.
For my earlier query tried to find controlled experiments to collect statistical evidence.
But I am facing difficulties to find a suggestion about controlled experiments to collect statistical evidence. Please provide any of your tutorials which supports my question to get a solution.
Please help me how to do controlled experiments to collect statistical evidence to find model performance,
You’re question is very broad, I don’t know how to answer it.
Hi jason , Thanks for the nice post .
Please i have a question:
I trained light-gbm for classification (150 observations) , I split 80% for model training and hypeparameters tunnig using 5-fold CV and retained 20% for testing.
I repeated (100 times ) this procedure , i got different results (every time i change the split for training(hyperparameters tuning ) , testing ) . Should i take , the model who gave me the best score (AUC) on test data , or the mean .
Algo :
repeat {1,…100} :
X_train,X_test,y_train,y_test = train_test_split(X,y,test_size = 0.2)
————————————————————————————–
parameters tuning on (X_train,y_train) using 5 cv
—————————————————————————————
Train the model using the best hyperparameters on 80% (X_train,Y_train)
—————————————————————————————–
evaluate on 20% (X_test,y_test).
——————————————————————————————-
100 score (Auc) [ standard deviation : 0.12]
Should i take the mean of the scores with this high standard deviation ,or the best one ?
No.
You are estimating the average performance of the model when used to make predictions on new data.
You use the estimate to select which modeling procedure to use, then you fit on all available data and use it to start making predictions.
Thank you for your answer , in general i think you inderstand what i did , but i am not quite sure that i understand very well the second point .
Can you please suggest me , how can i take the final score , actually for different test data (unssen ), i got different results ) , should i take only one mixture ( not 100 ) but in this case which one would be correct .
Thank you very much for your help.
I’m sorry, I don’t understand your question. Perhaps you can rephrase it?
Indeed, it s not so easy to be understood in machine mearnin:)
Just to understand your points, the second one “You use the estimate to select which modeling procedure to use, then you fit on all available data and use it to start making predictions.” Is it what you suggest doing? Or can you please rephrase it?
So far I did a procedure I should not have, it was like a double cross-validation. But now I want to know what I should do.
My fundamental question is : when I test the new data (20% left a part from the start) after all the CV and hyperparameters choice (max of mean CV). So if I repeat the test 100 times with different hyperparameters and with the same 20% test data, I want to stabilize the final result. But is it correct to do in such a way? Is it how it is done in machine elearning competitions? Or should I use only the best hyperparameters chosen the training CV phase of 80%?
On the side note, I actually wonder if I should reply a clinical question and machine learning separately, as I am working on clinical data of survival.
All CV models are discarded and you fit a final model on all available data.
Yes, you can learn more about choosing and fitting a final model here:
https://machinelearningmastery.com/train-final-machine-learning-model/
You can reduce the variance of the final model by fitting multiple final models with different seeds and ensemble them together:
https://machinelearningmastery.com/how-to-reduce-model-variance/
Dear Jason,
Thanks for this tutorial, it is very helpful. And I have a specific problem in terms of the uncertainty.
I build a LSTM model to predict human activity with different window length, and I use seed to make my results reproducible. Now the problem is every time wheh window is 1.25s, the performance is worse than other window.( I use window from 0.25 to 3.5 with a step 0.25s)
As I understand, this maybe due to the random initialization of weight, make the LSTM can not find a good optimum point. But I am not sure is there other reasons?
And from your tutorial, in this situation, I should set different seed number and run the model many times to get an average results? is there other method I should try?
Thanks
ldd
I recommend not fixing the seed and instead evaluate your model by repeating the evaluation many times and averaging the result:
https://machinelearningmastery.com/faq/single-faq/why-do-i-get-different-results-each-time-i-run-the-code
Hi Jason. Very informative tutorial! I am doing a tweet classification project. And the task here is to determine whether a tweet is traffic-related or not.
I have collected a huge number of tweets. To train a classifier, I first use keywords to get some candidate tweets. Then I manually review these tweets and check whether these tweets are traffic-related or not. I also construct another a dataset which are not traffic-related. Finally, I get 1000 traffic-related tweets and 2000 not traffic-related tweets.
Then I use the CNN-LSTM model to train a classifier based on the labeled data I have just created. Actually, the model performs quite well, reaching 0.99 F1 score on the test data. The confusion matrix about the performance of the model on the test data is:
[[384 3]
[ 2 223]]
However, when I use the trained classifier to make the prediction on all the tweets I have collected. The performance is really bad. I manually check the tweet which is labeled by ‘traffic_related’ by the classifier, and I find that most of the tweets are not traffic-related at all.
I am wondering which part I did wrong. For more information, please check this StackOverflow link I have posted: https://stackoverflow.com/questions/63087654/well-trained-classifier-does-not-perform-well-in-same-source-of-dataset
Thank you very much for your time!
Thanks!
Nice problem.
Perhaps use cross-validation to estimate model performance.
Perhaps explore different model types to confirm a neural net is required.
Perhaps inspect more data and confirm the training data is sensible.
Perhaps confirm your hold out dataset is sufficiently representative.
Let me know how you go.
Jason, can you provide any link for details discussion of more robust test harness procedure.
I recommend repeated stratified k-fold cross-validation:
https://machinelearningmastery.com/repeated-k-fold-cross-validation-with-python/
I also like nested CV:
https://machinelearningmastery.com/nested-cross-validation-for-machine-learning-with-python/
Hi, Jason, thanks for the article.
I think this problem is often happening to time series data, that’s bad the time series data cannot be shuffled, which results in the mismatch of training data and testing data. The trained LSTM model makes perfect predictions on training data, but very poor in testing data, do you have some idea how to solve this problem?
Thanks.
You’re welcome.
Yes, you must use walk-forward validation instead. You can learn about it here:
https://machinelearningmastery.com/backtest-machine-learning-models-time-series-forecasting/
Thank you for your nice article as always.
Please I want your advice about an issue I face in my segmentation model. I deal with microscopic images that are big in size so I train a U-net like model in a patch-wise manner. I split the patches using 5 cross-validation. When training the model I got very good results for the training and validation sets (patches), but when I tested the model on the test datasets (predicting the patches and stitching the results to form the whole image) I got very bad results, can you explain what may go wrong?
I have another issue, as the epochs pass, the segmentation prediction gradually becomes worse instead of being better?
Hi Samir…It may be helpful to know whether your models are “underfitting” or “overfitting”. The following resources will hopefully give you some ideas:
https://machinelearningmastery.com/diagnose-overfitting-underfitting-lstm-models/
https://machinelearningmastery.com/overfitting-and-underfitting-with-machine-learning-algorithms/