Hyperparameter optimization refers to performing a search in order to discover the set of specific model configuration arguments that result in the best performance of the model on a specific dataset.
There are many ways to perform hyperparameter optimization, although modern methods, such as Bayesian Optimization, are fast and effective. The Scikit-Optimize library is an open-source Python library that provides an implementation of Bayesian Optimization that can be used to tune the hyperparameters of machine learning models from the scikit-Learn Python library.
You can easily use the Scikit-Optimize library to tune the models on your next machine learning project.
In this tutorial, you will discover how to use the Scikit-Optimize library to use Bayesian Optimization for hyperparameter tuning.
After completing this tutorial, you will know:
- Scikit-Optimize provides a general toolkit for Bayesian Optimization that can be used for hyperparameter tuning.
- How to manually use the Scikit-Optimize library to tune the hyperparameters of a machine learning model.
- How to use the built-in BayesSearchCV class to perform model hyperparameter tuning.
Let’s get started.
- Update Nov/2020: Updated broken API links because the skopt website changed.
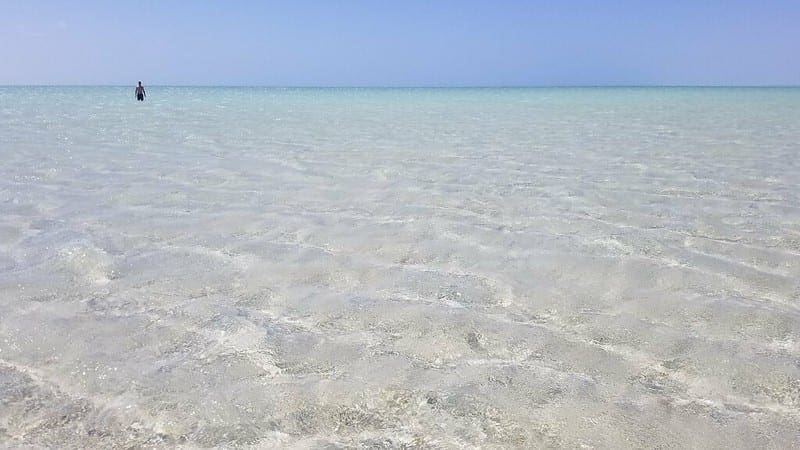
Scikit-Optimize for Hyperparameter Tuning in Machine Learning
Photo by Dan Nevill, some rights reserved.
Tutorial Overview
This tutorial is divided into four parts; they are:
- Scikit-Optimize
- Machine Learning Dataset and Model
- Manually Tune Algorithm Hyperparameters
- Automatically Tune Algorithm Hyperparameters
Scikit-Optimize
Scikit-Optimize, or skopt for short, is an open-source Python library for performing optimization tasks.
It offers efficient optimization algorithms, such as Bayesian Optimization, and can be used to find the minimum or maximum of arbitrary cost functions.
Bayesian Optimization provides a principled technique based on Bayes Theorem to direct a search of a global optimization problem that is efficient and effective. It works by building a probabilistic model of the objective function, called the surrogate function, that is then searched efficiently with an acquisition function before candidate samples are chosen for evaluation on the real objective function.
For more on the topic of Bayesian Optimization, see the tutorial:
Importantly, the library provides support for tuning the hyperparameters of machine learning algorithms offered by the scikit-learn library, so-called hyperparameter optimization. As such, it offers an efficient alternative to less efficient hyperparameter optimization procedures such as grid search and random search.
The scikit-optimize library can be installed using pip, as follows:
1 |
sudo pip install scikit-optimize |
Once installed, we can import the library and print the version number to confirm the library was installed successfully and can be accessed.
The complete example is listed below.
1 2 3 |
# report scikit-optimize version number import skopt print('skopt %s' % skopt.__version__) |
Running the example reports the currently installed version number of scikit-optimize.
Your version number should be the same or higher.
1 |
skopt 0.7.2 |
For more installation instructions, see the documentation:
Now that we are familiar with what Scikit-Optimize is and how to install it, let’s explore how we can use it to tune the hyperparameters of a machine learning model.
Machine Learning Dataset and Model
First, let’s select a standard dataset and a model to address it.
We will use the ionosphere machine learning dataset. This is a standard machine learning dataset comprising 351 rows of data with three numerical input variables and a target variable with two class values, e.g. binary classification.
Using a test harness of repeated stratified 10-fold cross-validation with three repeats, a naive model can achieve an accuracy of about 64 percent. A top performing model can achieve accuracy on this same test harness of about 94 percent. This provides the bounds of expected performance on this dataset.
The dataset involves predicting whether measurements of the ionosphere indicate a specific structure or not.
You can learn more about the dataset here:
No need to download the dataset; we will download it automatically as part of our worked examples.
The example below downloads the dataset and summarizes its shape.
1 2 3 4 5 6 7 8 9 |
# summarize the ionosphere dataset from pandas import read_csv # load dataset url = 'https://raw.githubusercontent.com/jbrownlee/Datasets/master/ionosphere.csv' dataframe = read_csv(url, header=None) # split into input and output elements data = dataframe.values X, y = data[:, :-1], data[:, -1] print(X.shape, y.shape) |
Running the example downloads the dataset and splits it into input and output elements. As expected, we can see that there are 351 rows of data with 34 input variables.
1 |
(351, 34) (351,) |
We can evaluate a support vector machine (SVM) model on this dataset using repeated stratified cross-validation.
We can report the mean model performance on the dataset averaged over all folds and repeats, which will provide a reference for model hyperparameter tuning performed in later sections.
The complete example is listed below.
1 2 3 4 5 6 7 8 9 10 11 12 13 14 15 16 17 18 19 20 21 |
# evaluate an svm for the ionosphere dataset from numpy import mean from numpy import std from pandas import read_csv from sklearn.model_selection import cross_val_score from sklearn.model_selection import RepeatedStratifiedKFold from sklearn.svm import SVC # load dataset url = 'https://raw.githubusercontent.com/jbrownlee/Datasets/master/ionosphere.csv' dataframe = read_csv(url, header=None) # split into input and output elements data = dataframe.values X, y = data[:, :-1], data[:, -1] print(X.shape, y.shape) # define model model model = SVC() # define test harness cv = RepeatedStratifiedKFold(n_splits=10, n_repeats=3, random_state=1) # evaluate model m_scores = cross_val_score(model, X, y, scoring='accuracy', cv=cv, n_jobs=-1, error_score='raise') print('Accuracy: %.3f (%.3f)' % (mean(m_scores), std(m_scores))) |
Running the example first loads and prepares the dataset, then evaluates the SVM model on the dataset.
Note: Your results may vary given the stochastic nature of the algorithm or evaluation procedure, or differences in numerical precision. Consider running the example a few times and compare the average outcome.
In this case, we can see that the SVM with default hyperparameters achieved a mean classification accuracy of about 93.7 percent, which is skillful and close to the top performance on the problem of 94 percent.
1 2 |
(351, 34) (351,) Accuracy: 0.937 (0.038) |
Next, let’s see if we can improve performance by tuning the model hyperparameters using the scikit-optimize library.
Manually Tune Algorithm Hyperparameters
The Scikit-Optimize library can be used to tune the hyperparameters of a machine learning model.
We can achieve this manually by using the Bayesian Optimization capabilities of the library.
This requires that we first define a search space. In this case, this will be the hyperparameters of the model that we wish to tune, and the scope or range of each hyperparameter.
We will tune the following hyperparameters of the SVM model:
- C, the regularization parameter.
- kernel, the type of kernel used in the model.
- degree, used for the polynomial kernel.
- gamma, used in most other kernels.
For the numeric hyperparameters C and gamma, we will define a log scale to search between a small value of 1e-6 and 100. Degree is an integer and we will search values between 1 and 5. Finally, the kernel is a categorical variable with specific named values.
We can define the search space for these four hyperparameters, a list of data types from the skopt library, as follows:
1 2 3 4 5 6 7 |
... # define the space of hyperparameters to search search_space = list() search_space.append(Real(1e-6, 100.0, 'log-uniform', name='C')) search_space.append(Categorical(['linear', 'poly', 'rbf', 'sigmoid'], name='kernel')) search_space.append(Integer(1, 5, name='degree')) search_space.append(Real(1e-6, 100.0, 'log-uniform', name='gamma')) |
Note the data type, the range, and the name of the hyperparameter specified for each.
We can then define a function that will be called by the search procedure. This is a function expected by the optimization procedure later and takes a model and set of specific hyperparameters for the model, evaluates it, and returns a score for the set of hyperparameters.
In our case, we want to evaluate the model using repeated stratified 10-fold cross-validation on our ionosphere dataset. We want to maximize classification accuracy, e.g. find the set of model hyperparameters that give the best accuracy. By default, the process minimizes the score returned from this function, therefore, we will return one minus the accuracy, e.g. perfect skill will be (1 – accuracy) or 0.0, and the worst skill will be 1.0.
The evaluate_model() function below implements this and takes a specific set of hyperparameters.
1 2 3 4 5 6 7 8 9 10 11 12 13 14 |
# define the function used to evaluate a given configuration @use_named_args(search_space) def evaluate_model(**params): # configure the model with specific hyperparameters model = SVC() model.set_params(**params) # define test harness cv = RepeatedStratifiedKFold(n_splits=10, n_repeats=3, random_state=1) # calculate 5-fold cross validation result = cross_val_score(model, X, y, cv=cv, n_jobs=-1, scoring='accuracy') # calculate the mean of the scores estimate = mean(result) # convert from a maximizing score to a minimizing score return 1.0 - estimate |
Next, we can execute the search by calling the gp_minimize() function and passing the name of the function to call to evaluate each model and the search space to optimize.
1 2 3 |
... # perform optimization result = gp_minimize(evaluate_model, search_space) |
The procedure will run until it converges and returns a result.
The result object contains lots of details, but importantly, we can access the score of the best performing configuration and the hyperparameters used by the best forming model.
1 2 3 4 |
... # summarizing finding: print('Best Accuracy: %.3f' % (1.0 - result.fun)) print('Best Parameters: %s' % (result.x)) |
Tying this together, the complete example of manually tuning the hyperparameters of an SVM on the ionosphere dataset is listed below.
1 2 3 4 5 6 7 8 9 10 11 12 13 14 15 16 17 18 19 20 21 22 23 24 25 26 27 28 29 30 31 32 33 34 35 36 37 38 39 40 41 42 43 44 45 46 |
# manually tune svm model hyperparameters using skopt on the ionosphere dataset from numpy import mean from pandas import read_csv from sklearn.model_selection import cross_val_score from sklearn.model_selection import RepeatedStratifiedKFold from sklearn.svm import SVC from skopt.space import Integer from skopt.space import Real from skopt.space import Categorical from skopt.utils import use_named_args from skopt import gp_minimize # define the space of hyperparameters to search search_space = list() search_space.append(Real(1e-6, 100.0, 'log-uniform', name='C')) search_space.append(Categorical(['linear', 'poly', 'rbf', 'sigmoid'], name='kernel')) search_space.append(Integer(1, 5, name='degree')) search_space.append(Real(1e-6, 100.0, 'log-uniform', name='gamma')) # define the function used to evaluate a given configuration @use_named_args(search_space) def evaluate_model(**params): # configure the model with specific hyperparameters model = SVC() model.set_params(**params) # define test harness cv = RepeatedStratifiedKFold(n_splits=10, n_repeats=3, random_state=1) # calculate 5-fold cross validation result = cross_val_score(model, X, y, cv=cv, n_jobs=-1, scoring='accuracy') # calculate the mean of the scores estimate = mean(result) # convert from a maximizing score to a minimizing score return 1.0 - estimate # load dataset url = 'https://raw.githubusercontent.com/jbrownlee/Datasets/master/ionosphere.csv' dataframe = read_csv(url, header=None) # split into input and output elements data = dataframe.values X, y = data[:, :-1], data[:, -1] print(X.shape, y.shape) # perform optimization result = gp_minimize(evaluate_model, search_space) # summarizing finding: print('Best Accuracy: %.3f' % (1.0 - result.fun)) print('Best Parameters: %s' % (result.x)) |
Running the example may take a few moments, depending on the speed of your machine.
You may see some warning messages that you can safely ignore, such as:
1 |
UserWarning: The objective has been evaluated at this point before. |
At the end of the run, the best-performing configuration is reported.
Note: Your results may vary given the stochastic nature of the algorithm or evaluation procedure, or differences in numerical precision. Consider running the example a few times and compare the average outcome.
In this case, we can see that configuration, reported in order of the search space list, was a modest C value, a RBF kernel, a degree of 2 (ignored by the RBF kernel), and a modest gamma value.
Importantly, we can see that the skill of this model was approximately 94.7 percent, which is a top-performing model
1 2 3 |
(351, 34) (351,) Best Accuracy: 0.948 Best Parameters: [1.2852670137769258, 'rbf', 2, 0.18178016885627174] |
This is not the only way to use the Scikit-Optimize library for hyperparameter tuning. In the next section, we can see a more automated approach.
Automatically Tune Algorithm Hyperparameters
The Scikit-Learn machine learning library provides tools for tuning model hyperparameters.
Specifically, it provides the GridSearchCV and RandomizedSearchCV classes that take a model, a search space, and a cross-validation configuration.
The benefit of these classes is that the search procedure is performed automatically, requiring minimal configuration.
Similarly, the Scikit-Optimize library provides a similar interface for performing a Bayesian Optimization of model hyperparameters via the BayesSearchCV class.
This class can be used in the same way as the Scikit-Learn equivalents.
First, the search space must be defined as a dictionary with hyperparameter names used as the key and the scope of the variable as the value.
1 2 3 4 5 6 7 |
... # define search space params = dict() params['C'] = (1e-6, 100.0, 'log-uniform') params['gamma'] = (1e-6, 100.0, 'log-uniform') params['degree'] = (1,5) params['kernel'] = ['linear', 'poly', 'rbf', 'sigmoid'] |
We can then define the BayesSearchCV configuration taking the model we wish to evaluate, the hyperparameter search space, and the cross-validation configuration.
1 2 3 4 5 |
... # define evaluation cv = RepeatedStratifiedKFold(n_splits=10, n_repeats=3, random_state=1) # define the search search = BayesSearchCV(estimator=SVC(), search_spaces=params, n_jobs=-1, cv=cv) |
We can then execute the search and report the best result and configuration at the end.
1 2 3 4 5 6 |
... # perform the search search.fit(X, y) # report the best result print(search.best_score_) print(search.best_params_) |
Tying this together, the complete example of automatically tuning SVM hyperparameters using the BayesSearchCV class on the ionosphere dataset is listed below.
1 2 3 4 5 6 7 8 9 10 11 12 13 14 15 16 17 18 19 20 21 22 23 24 25 26 27 28 |
# automatic svm hyperparameter tuning using skopt for the ionosphere dataset from pandas import read_csv from sklearn.model_selection import cross_val_score from sklearn.svm import SVC from sklearn.model_selection import RepeatedStratifiedKFold from skopt import BayesSearchCV # load dataset url = 'https://raw.githubusercontent.com/jbrownlee/Datasets/master/ionosphere.csv' dataframe = read_csv(url, header=None) # split into input and output elements data = dataframe.values X, y = data[:, :-1], data[:, -1] print(X.shape, y.shape) # define search space params = dict() params['C'] = (1e-6, 100.0, 'log-uniform') params['gamma'] = (1e-6, 100.0, 'log-uniform') params['degree'] = (1,5) params['kernel'] = ['linear', 'poly', 'rbf', 'sigmoid'] # define evaluation cv = RepeatedStratifiedKFold(n_splits=10, n_repeats=3, random_state=1) # define the search search = BayesSearchCV(estimator=SVC(), search_spaces=params, n_jobs=-1, cv=cv) # perform the search search.fit(X, y) # report the best result print(search.best_score_) print(search.best_params_) |
Running the example may take a few moments, depending on the speed of your machine.
You may see some warning messages that you can safely ignore, such as:
1 |
UserWarning: The objective has been evaluated at this point before. |
At the end of the run, the best-performing configuration is reported.
Note: Your results may vary given the stochastic nature of the algorithm or evaluation procedure, or differences in numerical precision. Consider running the example a few times and compare the average outcome.
In this case, we can see that the model performed above top-performing models achieving a mean classification accuracy of about 95.2 percent.
The search discovered a large C value, an RBF kernel, and a small gamma value.
1 2 3 |
(351, 34) (351,) 0.9525166191832859 OrderedDict([('C', 4.8722263953328735), ('degree', 4), ('gamma', 0.09805881007239009), ('kernel', 'rbf')]) |
This provides a template that you can use to tune the hyperparameters on your machine learning project.
Further Reading
This section provides more resources on the topic if you are looking to go deeper.
Related Tutorials
- Results for Standard Classification and Regression Machine Learning Datasets
- How to Implement Bayesian Optimization From Scratch in Python
APIs
Summary
In this tutorial, you discovered how to use the Scikit-Optimize library to use Bayesian Optimization for hyperparameter tuning.
Specifically, you learned:
- Scikit-Optimize provides a general toolkit for Bayesian Optimization that can be used for hyperparameter tuning.
- How to manually use the Scikit-Optimize library to tune the hyperparameters of a machine learning model.
- How to use the built-in BayesSearchCV class to perform model hyperparameter tuning.
Do you have any questions?
Ask your questions in the comments below and I will do my best to answer.
Wonderful Jason, it seems promising!
Thank you again for sharing!
You’re welcome!
That’s some real secret sauce. Thanks for the clear explanation and example code. I like that it runs multi-threaded. I converged to .955 accuracy in just under a minute.
Thanks, I hope it helps on you next project!
Thanks! amazing explanation!!
You’re welcome.
Thank you Jason for this wonderful content.
I’m learning the cores through your books.
Really it is amazing.
Thanks!
Hey Jason,
I have found an error in your text.
At the end of section “Machine Learning Dataset and Model” you write:
“In this case, we can see that the SVM with default hyperparameters achieved a mean classification accuracy of about 83.7 percent, which is skillful and close to the top performance on the problem of 94 percent.”
However, the performance is actually 0.937 and not 0.837.
I also verified this by implementing your code by myself.
Therefore the performance of the out-of-the-box SVM is 93.7 not 83.7.
Cheers
Tom
Thanks Tom, looks like a typo. Fixed.
What a great secret!
Thanks for sharing
Thanks.
Dear Dr Jason,
This is a question on relying on the optimized parameters when wanting to fix the parameters on a later use of the data..
SKIP the background information.
My questions are about running the programs a few times – (i) do I use the lowest or highest accuracy and associated parameters and (ii) if I have similar accuracies, do I use say high C or a low C?
Background information
I ran the second model twice
You mention regularly the ‘stochastic nature’ of the results.
Knowing the fined-tuned parameters to set to which of the parameters to set? Do I use the model’s parameters associated with the accuracy of 0.866 or the parameters associated with the accuracy of 0.951.
YET, even with an accuracy of 0.951, the associated parameters are different than your models.
To illustrate
That is even with very similar accuracy, your model had C=4.87 compared to my C at 26. Similarly your model was degree 4 and my model was degree 5. Your gamma was over twice my gamma.
Questions:
In sum: which model parameters do I use? the one associated with the highest or lowest accuracy?
Suppose I have models that produce similar accuracies. Which of the associated parameters do I use – highest varlue of C or lowest value of C?
Thank you,
Anthony of Sydney
It is a good idea to report the distribution of the performance (e.g. mean and stdev) of a repeated experiment.
Choose a final model that is fit using a stochastic learning algorithm is challenging. I recommend fitting multiple final models and using them together in an ensemble:
https://machinelearningmastery.com/how-to-reduce-model-variance/
Dear Dr Jason,
I noticed that you used two different approaches to defining the search space for four hyperparmeters.
Under the subheading “Manually Tune Algorithm Parameters”, your search space for the hyperparameters were set up for the SVC in lines 13-19 as a list().
In contrast under the subheading “Automatically Tune Algorithm Hyperparameters” your search space hyperparameters were set up as a dictionary in lines 14-19.
I find the setting of the parameters search under the dictionary method less complicated than the list() approach.
I am trying to work out how to use dictionary method instead of the list method for the lines 22-33 of the code under “Manually Tune Algorithm Hyperparameters”
Merely replacing a list() with a dict() gives you the error message
Why can’t I use the dict() approach. It is easier because you don’t need to concern yourself of using the Real, Categorical, Integer and Real settings as described at https://scikit-optimize.github.io/stable/modules/generated/skopt.Space.html.
Thank you,
Anthony of Sydney
Both approaches use two different APIs and are not directly compatible.
Dear Dr Jason,
Thank you for for that.
Anthony of Sydney
You’re welcome.
Dear Dr Jason,
I have a question that deals with functions having hyperparameters.
In the example you used the SVC() function which had a number of hyperparameters.
You set up the hyperparameters search list as:
Using the inspect method, I was able to find out the parameters of SVC().
Question: I would like to know how to relate the signature of SVC to the search_list. That is if I know the signature, how do I relate the signature with setting up the search space? I will ask ONE example and hope to find the pattern.
Example.
In SVC()’s signature:
Where does one get the information to set up the parameters 1e-6, 100, ‘log-uniform’ associated with C:
What about instead of ‘log-uniform’ say ‘binary’ or ‘blahblahblah-distribution’?
In other words, if I find functions with parameters, how to find which are valid/invalid parameters and the range of the parameters for a given functions’s signature.
Thank you,
Anthony of Sydney
Dear Dr Jason,
I wish to amend the question with inspect.signature(SVC):
Again the question is if I have a function which has a lot of parameters to tune such as SVC(), how do I relate the signature of the function to setting up the search_space for hyperparameter tuning?
For example in the signature for SVC(), I have:
In the search space, you have the search space associated with ‘C’:
The page https://scikit-optimize.github.io/stable/modules/generated/skopt.Space.html barely relates the function’s signature of SVC() to organizing the search space.
Thank you,
Anthony of Sydney
C is a regularization hyperparameter, it is common to search regularization hyperparametres on a log-10 scale.
You can see the full list of hyperparameters supported by the SVC model here:
https://scikit-learn.org/stable/modules/generated/sklearn.svm.SVC.html
I chose specific ranges for each hyperparameter based on my knowledge of how the algorithm works / the role of the hyperparameter.
Yes, you can choose different hyperparameters to tune and different distributions. Go for it!
E.g. this tutorial has more information on the common hyperparameter to tune for machine learning algorithms:
https://machinelearningmastery.com/hyperparameters-for-classification-machine-learning-algorithms/
How can we use the automatic version that uses BayesSearchCV in order to optimize a model for ROC-AUC (maximization) or CrossEntropy (minimization)?
Specify the “scoring” argument to whatever metric you like.
More details here:
https://scikit-optimize.github.io/stable/modules/generated/skopt.BayesSearchCV.html
Hi, i always get error. it stated:
__init__() got an unexpected keyword argument ‘iid’
i searched for solution and found that iid parameter is deprecated in current version of sklearn. how to solve this.
thanks
Perhaps remove that argument from your example?
Perhaps ensure your libraries are up to date?
Hey Jason, How can I implement the tuning using skopt for regression problem ?
Perhaps you can try adapting the above example – if skopt supports regression, which I assume it does.
Hi Jason,
Many thanks for this tutorial. I did not know that such an easy to use module (skopt) was available, and this makes it simple to implement Bayesian optimization.
Now that scikit-learn v0.24 is out, it introduces an incompatibility with BayesSearchCV. The latter passes an iid=True parameter, by default, but scikit-learn no longer accepts it: https://github.com/scikit-optimize/scikit-optimize/issues/978
Until scikit-optimize is changed to be compatible, it will be necessary to downgrade scikit-learn to version 0.23.2.
Please consider adding a note to that effect in your description.
Thanks for letting me know.
Hi Jason,
Are these both methods (manual and automatic) doing the same thing at the end? Are they using the same algorithm under the hood with different apis? Can we expect same result set from both apis?
Regards,
Adarsh
Same general approach, different implementations.
Thanks for the interesting article, Jason. I am currently struggling with a problem where I need to tune hyperparameters of not only my classifier, but also of a second algorithm that runs before the classifier.
All scikit-learn approaches only seem to tune the parameters of the estimator only, as does BayesSearchCV used here. For other problems I could use transformers and chain them together with the estimator in a sklearn pipeline that I can feed into a standard CV algorithm.
BUT my problem is in the active-learning domain, where my first algorithm selects rows to train with, before the estimator is fit. But sklearn transformers cannot augment rows, as they only pass X to the estimator. But if I use only a subset of rows, I need to change y, too, which sklearn pipelines cannot.
forest_minimize() seems to be a candidate to solve this issue, as it seems to me that the objective function you call evaluate_model() could take any arbitrary script and tune all its parameters.
But this script works without cross validation, which would be important to have.
Do you see any ways I could wrap a (nested) cv around the manual approach you show above?
Briefly reading your description, it seems that you want to build a pipeline to connect everything you needed. Please checkout the pipeline function in scikit-learn. It should give you an insight.
Hi, Jason
Good post as always, how can i possibly implement similar hyperparameter optimization for SVR, if possible?
Theoretically it is possible. What are you intended to do here?
Thanks for this great tutorial! Your site is the second stackoverflow for me!
Can you please clear the “top performing” in the sentence:” A top performing model can achieve accuracy on this same test harness of about 94 percent.”. Is there any scoreboard for this or something else?
Thank you for the feedback Abdalsamad! You may find the following interesting:
https://machinelearningmastery.com/statistical-significance-tests-for-comparing-machine-learning-algorithms/
Hi Jason. Thanks for the tutorial. I have a question. What is the maximum number of hyperparameters that can be optimized using scikit optimize with a reasonable number of function calls, let’s say 10000?
Hi Lekha…You are very welcome! This is a great question! We will have to differ to the source documentation for such specifications:
https://scikit-optimize.github.io/dev//_downloads/scikit-optimize-docs.pdf