Model selection is the problem of choosing one from among a set of candidate models.
It is common to choose a model that performs the best on a hold-out test dataset or to estimate model performance using a resampling technique, such as k-fold cross-validation.
An alternative approach to model selection involves using probabilistic statistical measures that attempt to quantify both the model performance on the training dataset and the complexity of the model. Examples include the Akaike and Bayesian Information Criterion and the Minimum Description Length.
The benefit of these information criterion statistics is that they do not require a hold-out test set, although a limitation is that they do not take the uncertainty of the models into account and may end-up selecting models that are too simple.
In this post, you will discover probabilistic statistics for machine learning model selection.
After reading this post, you will know:
- Model selection is the challenge of choosing one among a set of candidate models.
- Akaike and Bayesian Information Criterion are two ways of scoring a model based on its log-likelihood and complexity.
- Minimum Description Length provides another scoring method from information theory that can be shown to be equivalent to BIC.
Kick-start your project with my new book Probability for Machine Learning, including step-by-step tutorials and the Python source code files for all examples.
Let’s get started.
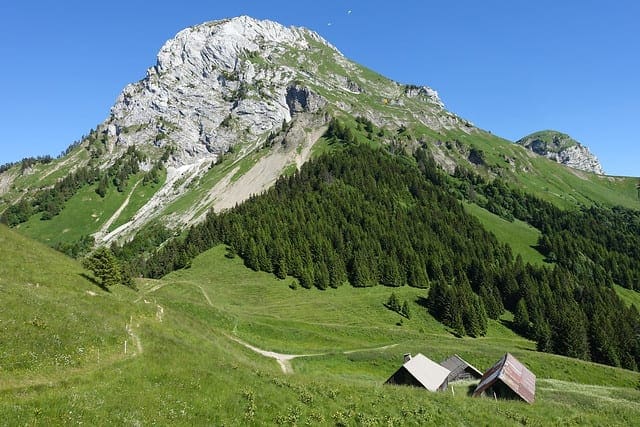
Probabilistic Model Selection Measures AIC, BIC, and MDL
Photo by Guilhem Vellut, some rights reserved.
Overview
This tutorial is divided into five parts; they are:
- The Challenge of Model Selection
- Probabilistic Model Selection
- Akaike Information Criterion
- Bayesian Information Criterion
- Minimum Description Length
The Challenge of Model Selection
Model selection is the process of fitting multiple models on a given dataset and choosing one over all others.
Model selection: estimating the performance of different models in order to choose the best one.
— Page 222, The Elements of Statistical Learning, 2016.
This may apply in unsupervised learning, e.g. choosing a clustering model, or supervised learning, e.g. choosing a predictive model for a regression or classification task. It may also be a sub-task of modeling, such as feature selection for a given model.
There are many common approaches that may be used for model selection. For example, in the case of supervised learning, the three most common approaches are:
- Train, Validation, and Test datasets.
- Resampling Methods.
- Probabilistic Statistics.
The simplest reliable method of model selection involves fitting candidate models on a training set, tuning them on the validation dataset, and selecting a model that performs the best on the test dataset according to a chosen metric, such as accuracy or error. A problem with this approach is that it requires a lot of data.
Resampling techniques attempt to achieve the same as the train/val/test approach to model selection, although using a small dataset. An example is k-fold cross-validation where a training set is split into many train/test pairs and a model is fit and evaluated on each. This is repeated for each model and a model is selected with the best average score across the k-folds. A problem with this and the prior approach is that only model performance is assessed, regardless of model complexity.
A third approach to model selection attempts to combine the complexity of the model with the performance of the model into a score, then select the model that minimizes or maximizes the score.
We can refer to this approach as statistical or probabilistic model selection as the scoring method uses a probabilistic framework.
Want to Learn Probability for Machine Learning
Take my free 7-day email crash course now (with sample code).
Click to sign-up and also get a free PDF Ebook version of the course.
Probabilistic Model Selection
Probabilistic model selection (or “information criteria”) provides an analytical technique for scoring and choosing among candidate models.
Models are scored both on their performance on the training dataset and based on the complexity of the model.
- Model Performance. How well a candidate model has performed on the training dataset.
- Model Complexity. How complicated the trained candidate model is after training.
Model performance may be evaluated using a probabilistic framework, such as log-likelihood under the framework of maximum likelihood estimation. Model complexity may be evaluated as the number of degrees of freedom or parameters in the model.
Historically various ‘information criteria’ have been proposed that attempt to correct for the bias of maximum likelihood by the addition of a penalty term to compensate for the over-fitting of more complex models.
— Page 33, Pattern Recognition and Machine Learning, 2006.
A benefit of probabilistic model selection methods is that a test dataset is not required, meaning that all of the data can be used to fit the model, and the final model that will be used for prediction in the domain can be scored directly.
A limitation of probabilistic model selection methods is that the same general statistic cannot be calculated across a range of different types of models. Instead, the metric must be carefully derived for each model.
It should be noted that the AIC statistic is designed for preplanned comparisons between models (as opposed to comparisons of many models during automated searches).
— Page 493, Applied Predictive Modeling, 2013.
A further limitation of these selection methods is that they do not take the uncertainty of the model into account.
Such criteria do not take account of the uncertainty in the model parameters, however, and in practice they tend to favour overly simple models.
— Page 33, Pattern Recognition and Machine Learning, 2006.
There are three statistical approaches to estimating how well a given model fits a dataset and how complex the model is. And each can be shown to be equivalent or proportional to each other, although each was derived from a different framing or field of study.
They are:
- Akaike Information Criterion (AIC). Derived from frequentist probability.
- Bayesian Information Criterion (BIC). Derived from Bayesian probability.
- Minimum Description Length (MDL). Derived from information theory.
Each statistic can be calculated using the log-likelihood for a model and the data. Log-likelihood comes from Maximum Likelihood Estimation, a technique for finding or optimizing the parameters of a model in response to a training dataset.
In Maximum Likelihood Estimation, we wish to maximize the conditional probability of observing the data (X) given a specific probability distribution and its parameters (theta), stated formally as:
- P(X ; theta)
Where X is, in fact, the joint probability distribution of all observations from the problem domain from 1 to n.
- P(x1, x2, x3, …, xn ; theta)
The joint probability distribution can be restated as the multiplication of the conditional probability for observing each example given the distribution parameters. Multiplying many small probabilities together can be unstable; as such, it is common to restate this problem as the sum of the natural log conditional probability.
- sum i to n log(P(xi ; theta))
Given the frequent use of log in the likelihood function, it is commonly referred to as a log-likelihood function.
The log-likelihood function for common predictive modeling problems include the mean squared error for regression (e.g. linear regression) and log loss (binary cross-entropy) for binary classification (e.g. logistic regression).
We will take a closer look at each of the three statistics, AIC, BIC, and MDL, in the following sections.
Akaike Information Criterion
The Akaike Information Criterion, or AIC for short, is a method for scoring and selecting a model.
It is named for the developer of the method, Hirotugu Akaike, and may be shown to have a basis in information theory and frequentist-based inference.
This is derived from a frequentist framework, and cannot be interpreted as an approximation to the marginal likelihood.
— Page 162, Machine Learning: A Probabilistic Perspective, 2012.
The AIC statistic is defined for logistic regression as follows (taken from “The Elements of Statistical Learning“):
- AIC = -2/N * LL + 2 * k/N
Where N is the number of examples in the training dataset, LL is the log-likelihood of the model on the training dataset, and k is the number of parameters in the model.
The score, as defined above, is minimized, e.g. the model with the lowest AIC is selected.
To use AIC for model selection, we simply choose the model giving smallest AIC over the set of models considered.
— Page 231, The Elements of Statistical Learning, 2016.
Compared to the BIC method (below), the AIC statistic penalizes complex models less, meaning that it may put more emphasis on model performance on the training dataset, and, in turn, select more complex models.
We see that the penalty for AIC is less than for BIC. This causes AIC to pick more complex models.
— Page 162, Machine Learning: A Probabilistic Perspective, 2012.
Bayesian Information Criterion
The Bayesian Information Criterion, or BIC for short, is a method for scoring and selecting a model.
It is named for the field of study from which it was derived: Bayesian probability and inference. Like AIC, it is appropriate for models fit under the maximum likelihood estimation framework.
The BIC statistic is calculated for logistic regression as follows (taken from “The Elements of Statistical Learning“):
- BIC = -2 * LL + log(N) * k
Where log() has the base-e called the natural logarithm, LL is the log-likelihood of the model, N is the number of examples in the training dataset, and k is the number of parameters in the model.
The score as defined above is minimized, e.g. the model with the lowest BIC is selected.
The quantity calculated is different from AIC, although can be shown to be proportional to the AIC. Unlike the AIC, the BIC penalizes the model more for its complexity, meaning that more complex models will have a worse (larger) score and will, in turn, be less likely to be selected.
Note that, compared to AIC […], this penalizes model complexity more heavily.
— Page 217, Pattern Recognition and Machine Learning, 2006.
Importantly, the derivation of BIC under the Bayesian probability framework means that if a selection of candidate models includes a true model for the dataset, then the probability that BIC will select the true model increases with the size of the training dataset. This cannot be said for the AIC score.
… given a family of models, including the true model, the probability that BIC will select the correct model approaches one as the sample size N -> infinity.
— Page 235, The Elements of Statistical Learning, 2016.
A downside of BIC is that for smaller, less representative training datasets, it is more likely to choose models that are too simple.
Minimum Description Length
The Minimum Description Length, or MDL for short, is a method for scoring and selecting a model.
It is named for the field of study from which it was derived, namely information theory.
Information theory is concerned with the representation and transmission of information on a noisy channel, and as such, measures quantities like entropy, which is the average number of bits required to represent an event from a random variable or probability distribution.
From an information theory perspective, we may want to transmit both the predictions (or more precisely, their probability distributions) and the model used to generate them. Both the predicted target variable and the model can be described in terms of the number of bits required to transmit them on a noisy channel.
The Minimum Description Length is the minimum number of bits, or the minimum of the sum of the number of bits required to represent the data and the model.
The Minimum Description Length (MDL) principle recommends choosing the hypothesis that minimizes the sum of these two description lengths.
— Page 173, Machine Learning, 1997.
The MDL statistic is calculated as follows (taken from “Machine Learning“):
- MDL = L(h) + L(D | h)
Where h is the model, D is the predictions made by the model, L(h) is the number of bits required to represent the model, and L(D | h) is the number of bits required to represent the predictions from the model on the training dataset.
The score as defined above is minimized, e.g. the model with the lowest MDL is selected.
The number of bits required to encode (D | h) and the number of bits required to encode (h) can be calculated as the negative log-likelihood; for example (taken from “The Elements of Statistical Learning“):
- MDL = -log(P(theta)) – log(P(y | X, theta))
Or the negative log-likelihood of the model parameters (theta) and the negative log-likelihood of the target values (y) given the input values (X) and the model parameters (theta).
This desire to minimize the encoding of the model and its predictions is related to the notion of Occam’s Razor that seeks the simplest (least complex) explanation: in this context, the least complex model that predicts the target variable.
The MDL principle takes the stance that the best theory for a body of data is one that minimizes the size of the theory plus the amount of information necessary to specify the exceptions relative to the theory …
— Page 198, Data Mining: Practical Machine Learning Tools and Techniques, 4th edition, 2016.
The MDL calculation is very similar to BIC and can be shown to be equivalent in some situations.
Hence the BIC criterion, derived as approximation to log-posterior probability, can also be viewed as a device for (approximate) model choice by minimum description length.
— Page 236, The Elements of Statistical Learning, 2016.
Worked Example for Linear Regression
We can make the calculation of AIC and BIC concrete with a worked example.
In this section, we will use a test problem and fit a linear regression model, then evaluate the model using the AIC and BIC metrics.
Importantly, the specific functional form of AIC and BIC for a linear regression model has previously been derived, making the example relatively straightforward. In adapting these examples for your own algorithms, it is important to either find an appropriate derivation of the calculation for your model and prediction problem or look into deriving the calculation yourself.
In this example, we will use a test regression problem provided by the make_regression() scikit-learn function. The problem will have two input variables and require the prediction of a target numerical value.
1 2 3 4 |
... # generate dataset X, y = make_regression(n_samples=100, n_features=2, noise=0.1) # define and fit the model on all data |
We will fit a LinearRegression() model on the entire dataset directly.
1 2 3 4 |
... # define and fit the model on all data model = LinearRegression() model.fit(X, y) |
Once fit, we can report the number of parameters in the model, which, given the definition of the problem, we would expect to be three (two coefficients and one intercept).
1 2 3 4 |
... # number of parameters num_params = len(model.coef_) + 1 print('Number of parameters: %d' % (num_params)) |
The likelihood function for a linear regression model can be shown to be identical to the least squares function; therefore, we can estimate the maximum likelihood of the model via the mean squared error metric.
First, the model can be used to estimate an outcome for each example in the training dataset, then the mean_squared_error() scikit-learn function can be used to calculate the mean squared error for the model.
1 2 3 4 5 6 |
... # predict the training set yhat = model.predict(X) # calculate the error mse = mean_squared_error(y, yhat) print('MSE: %.3f' % mse) |
Tying this all together, the complete example of defining the dataset, fitting the model, and reporting the number of parameters and maximum likelihood estimate of the model is listed below.
1 2 3 4 5 6 7 8 9 10 11 12 13 14 15 16 17 |
# generate a test dataset and fit a linear regression model from sklearn.datasets import make_regression from sklearn.linear_model import LinearRegression from sklearn.metrics import mean_squared_error # generate dataset X, y = make_regression(n_samples=100, n_features=2, noise=0.1) # define and fit the model on all data model = LinearRegression() model.fit(X, y) # number of parameters num_params = len(model.coef_) + 1 print('Number of parameters: %d' % (num_params)) # predict the training set yhat = model.predict(X) # calculate the error mse = mean_squared_error(y, yhat) print('MSE: %.3f' % mse) |
Note: Your results may vary given the stochastic nature of the algorithm or evaluation procedure, or differences in numerical precision. Consider running the example a few times and compare the average outcome.
Running the example first reports the number of parameters in the model as 3, as we expected, then reports the MSE as about 0.01.
1 2 |
Number of parameters: 3 MSE: 0.010 |
Next, we can adapt the example to calculate the AIC for the model.
Skipping the derivation, the AIC calculation for an ordinary least squares linear regression model can be calculated as follows (taken from “A New Look At The Statistical Identification Model“, 1974.):
- AIC = n * LL + 2 * k
Where n is the number of examples in the training dataset, LL is the log-likelihood for the model using the natural logarithm (e.g. the log of the MSE), and k is the number of parameters in the model.
The calculate_aic() function below implements this, taking n, the raw mean squared error (mse), and k as arguments.
1 2 3 4 |
# calculate aic for regression def calculate_aic(n, mse, num_params): aic = n * log(mse) + 2 * num_params return aic |
The example can then be updated to make use of this new function and calculate the AIC for the model.
The complete example is listed below.
1 2 3 4 5 6 7 8 9 10 11 12 13 14 15 16 17 18 19 20 21 22 23 24 25 26 27 |
# calculate akaike information criterion for a linear regression model from math import log from sklearn.datasets import make_regression from sklearn.linear_model import LinearRegression from sklearn.metrics import mean_squared_error # calculate aic for regression def calculate_aic(n, mse, num_params): aic = n * log(mse) + 2 * num_params return aic # generate dataset X, y = make_regression(n_samples=100, n_features=2, noise=0.1) # define and fit the model on all data model = LinearRegression() model.fit(X, y) # number of parameters num_params = len(model.coef_) + 1 print('Number of parameters: %d' % (num_params)) # predict the training set yhat = model.predict(X) # calculate the error mse = mean_squared_error(y, yhat) print('MSE: %.3f' % mse) # calculate the aic aic = calculate_aic(len(y), mse, num_params) print('AIC: %.3f' % aic) |
Running the example reports the number of parameters and MSE as before and then reports the AIC.
Note: Your results may vary given the stochastic nature of the algorithm or evaluation procedure, or differences in numerical precision. Consider running the example a few times and compare the average outcome.
In this case, the AIC is reported to be a value of about -451.616. This value can be minimized in order to choose better models.
1 2 3 |
Number of parameters: 3 MSE: 0.010 AIC: -451.616 |
We can also explore the same example with the calculation of BIC instead of AIC.
Skipping the derivation, the BIC calculation for an ordinary least squares linear regression model can be calculated as follows (taken from here):
- BIC = n * LL + k * log(n)
Where n is the number of examples in the training dataset, LL is the log-likelihood for the model using the natural logarithm (e.g. log of the mean squared error), and k is the number of parameters in the model, and log() is the natural logarithm.
The calculate_bic() function below implements this, taking n, the raw mean squared error (mse), and k as arguments.
1 2 3 4 |
# calculate bic for regression def calculate_bic(n, mse, num_params): bic = n * log(mse) + num_params * log(n) return bic |
The example can then be updated to make use of this new function and calculate the BIC for the model.
The complete example is listed below.
1 2 3 4 5 6 7 8 9 10 11 12 13 14 15 16 17 18 19 20 21 22 23 24 25 26 27 |
# calculate bayesian information criterion for a linear regression model from math import log from sklearn.datasets import make_regression from sklearn.linear_model import LinearRegression from sklearn.metrics import mean_squared_error # calculate bic for regression def calculate_bic(n, mse, num_params): bic = n * log(mse) + num_params * log(n) return bic # generate dataset X, y = make_regression(n_samples=100, n_features=2, noise=0.1) # define and fit the model on all data model = LinearRegression() model.fit(X, y) # number of parameters num_params = len(model.coef_) + 1 print('Number of parameters: %d' % (num_params)) # predict the training set yhat = model.predict(X) # calculate the error mse = mean_squared_error(y, yhat) print('MSE: %.3f' % mse) # calculate the bic bic = calculate_bic(len(y), mse, num_params) print('BIC: %.3f' % bic) |
Running the example reports the number of parameters and MSE as before and then reports the BIC.
Note: Your results may vary given the stochastic nature of the algorithm or evaluation procedure, or differences in numerical precision. Consider running the example a few times and compare the average outcome.
In this case, the BIC is reported to be a value of about -450.020, which is very close to the AIC value of -451.616. Again, this value can be minimized in order to choose better models.
1 2 3 |
Number of parameters: 3 MSE: 0.010 BIC: -450.020 |
Further Reading
This section provides more resources on the topic if you are looking to go deeper.
Books
- Chapter 7 Model Assessment and Selection, The Elements of Statistical Learning, 2016.
- Section 1.3 Model Selection, Pattern Recognition and Machine Learning, 2006.
- Section 4.4.1 Model comparison and BIC, Pattern Recognition and Machine Learning, 2006.
- Section 6.6 Minimum Description Length Principle, Machine Learning, 1997.
- Section 5.3.2.4 BIC approximation to log marginal likelihood, Machine Learning: A Probabilistic Perspective, 2012.
- Applied Predictive Modeling, 2013.
- Section 28.3 Minimum description length (MDL), Information Theory, Inference and Learning Algorithms, 2003.
- Section 5.10 The MDL Principle, Data Mining: Practical Machine Learning Tools and Techniques, 4th edition, 2016.
Papers
API
- sklearn.datasets.make_regression API.
- sklearn.linear_model.LinearRegression API.
- sklearn.metrics.mean_squared_error API.
Articles
- Akaike information criterion, Wikipedia.
- Bayesian information criterion, Wikipedia.
- Minimum description length, Wikipedia.
Summary
In this post, you discovered probabilistic statistics for machine learning model selection.
Specifically, you learned:
- Model selection is the challenge of choosing one among a set of candidate models.
- Akaike and Bayesian Information Criterion are two ways of scoring a model based on its log-likelihood and complexity.
- Minimum Description Length provides another scoring method from information theory that can be shown to be equivalent to BIC.
Do you have any questions?
Ask your questions in the comments below and I will do my best to answer.
Hi Jason,
Thank you for this nice post!
I’m wondering how to deal with non-parametric models where the number of parameters depends strongly on the data (tree-based methods).
Best
Good question.
Typically such measures are challenging to use for non-parametric models like decision trees.
Hi Jason,
Instead of using mse, I have used log loss value in the equation of AIC and found good result on my dataset. I have also cross-checked the value with the AIC value found from statsmodel of python (logit). Here I have noticed that log loss value performed better than mse. Can you please explain why has that happened?
Log loss is for classification, e.g. logistic regression. The example in the tutorial is linear regression, e.g. predicting a numerical value and log loss is inappropriate.
For classification algorithms (listed below) other than Logistic Regression, should we always use Log Loss for calculating,the AIC?
List of other classification algorithms:
– k Nearest Neighbor
– SVC
– Naive Bayes
– Linear Discriminant Analysis
– Decision Tree
– Random Forest
I don’t think so.
Each algorithm will require its own AIC calculation to be derived, at least that is my understanding.
Has anyone tried implementing MDL for keras training?
No, sorry.
Hi, I have a question about parameters.
If I use MLP model, How can I get the number of parameters to calculate AIC ?
Thank you
model.summary() can access the number of parameters.
Hi Jason 🙂
I have a one more question.
As you told me, I just run “model.summary()”,
then it said “‘MLPRegressor’ object has no attribute ‘summary’.”
How can I fix this problem?
Thank you !
Perhaps your model has no layers?
Or do I just insert number of hyper-parameter of MLP model ? (like number of nodes, layers…)
Thank you
I believe AIC requires a specialized calculation for an MLP.
Hi Jason,
Thank you 🙂
Is a specialized calculation the number of parameters using model.summary() ?
No, I mean you will need to check the literature for how to calculate the metric for an MLP in an appropriate manner.
Jason, I’m finding your information and code examples most helpful as I work on my MS degree. Thank you for the time and effort it takes to compose these posts!!
To adapt the linear regression example for logistic regression, the calculation for AIC and BIC (line 9) requires adjustment, correct?
Earlier in this post you define the AIC and BIC calculations for Logistic Regression as:
AIC = -2/N * LL + 2 * k/N
BIC = -2 * LL + log(N) * k
My understanding is line 9 needs replacement with these equations and LL should be replaced with the logistic regression log-likelihood calculation described in your “Gentle Introduction to Logistic Regression” post:
log-likelihood = log(yhat) * y + log(1 – yhat) * (1 – y)
Am I on the right track?
There are many formula for the AIC and BIC metrics.
I tried to provide standard calculations and linked to their source, but they are not the only approaches that I have seen described.
I cannot comment on the best or most correct formula.
El BIC es el mejor criterio para seleccionar el modelo mas optimo de pronostico que el AIC??
It really depends on your project goals. There is never a best anything for all cases.
Hello!
Thank you for the useful article. What I miss is how can MSE stand in place of L despite the fact that a model is better if it has smaller MSE and not larger, like when we deal with L?
Hello again Jason, thank you for good lecture!
Question: Probabilistic model selection include complexity penalty along to error prediction minimization. Ine may ask, why don’t we just focus on test error score? I guess that the reasons for this are:
1) We prefer simple models (easier to interpret)
2) Simpler models normally require less memory, less train/test exectution time.
Is it correct and maybe you can add something to this list?
Regards!
Yes, but more important: simpler explanations are more likely correct and generalize than complex explanations.
Hello: I am an Auxologist trying to develop a model for describing human height growth data from birth to maturity (0 to 21 years ). My model assumes that human growth is due to the combination of n=nine logistic growth components. When I presented the paper at an international conference, I was told that I could use the AiC and BiC method for selecting the best number of logistic growth components needed for these data. Do you agree? Each data set contains 35 height measurements and a plot of the AiC values vs n shows a u-shaped curve, showing a minimum value when n= 6 components. However, when I fit the components to a data set containing two of the same measurements at a particular age (70 measurements), the AiC values and plots change, showing a minimum value when n= 9 components. Can I take this approach? Why does the AiC change when the number of data points change? Would you like to collaborate with me ?
Perhaps. Although you are preparing a descriptive rather than predictive model, e.g. statistics rather than machine learning.
The metrics change when the number of elements change because the number of elements impact the complexity of the model.
Hi Jason,
Nicely articulated indeed!
I got some ambiguity here, btw. Computing statsmodels’s aic(3026) on sklearn boston dataset is showing different result than this manual aic computation(1565).
Any help would be much appreciated!
Perhaps there is a difference in the implementation.
Hi Jason
Thank you for this blog. Could you please provide R code for the calculation of the MDL for linear regression model.
regards
Nkue
Thanks for the suggestion, perhaps in the future.
Or Python code
Thank you, Jason, for this post
I have one question about using AIC to calculate the goodness of fit for neural network and random forest models ? If we can use it, which package to use in R
Best regards
I don’t know an R package off hand, perhaps try a google search.
Thank you Jason
You’re welcome.
Hi Jason.
I’ve built two models.
Model 1- AIC= 8906
Model 2 AIC= – 9501
Is it right to compare negative AIC with positive AIC? Also which AIC above, proves a better model?
Sorry, I don’t interpret results.
H Jason,
Thanks for this great post.
For purposes of calculating BIC for a linear regression model, is the number of parameters strictly the number of terms (in the example above, 1 for the intercept + 2 for the two features = 3) or should it also include the variance, also being estimated in the fit (in which case, 4)?
I’m wondering because this https://en.wikipedia.org/wiki/Bayesian_information_criterion
seems to +1 for the variance.
Perhaps, I based my description on the textbooks listed at the end of the tutorial.
How do you calculate AIC and BIC for Logistic Regression Models in Python?
The above calculations will help directly.
It is really nicely articulated article. I had initially struggled to understand these concepts, but your article made it crystal clear. I wanted to implement new criteria for model selection via GLM based approach – stepwise forward regression using R or Python. Could you please suggest what parameters I can consider for defining criteria. Also in case you have sample code for GLM or stepwise forward regression, it would be great help.
You’re welcome.
Perhaps you can implement the algorithm from a paper or textbook or start with an existing implementation.
Do the methods listed above (make_regression) fit the model(s) by maximum likelihood?
If not, the following principle is ignored:
“The theory of AIC requires that the log-likelihood has been maximized: whereas AIC can be computed for models not fitted by maximum likelihood, their AIC values should not be compared.” (from ‘help(AIC)’ (package ‘stats’))
In other words: When linear models are not fitted by maximum likelihood, their results cannot be compared by measures such AIC and BIC.
Such measures also cannot be used to compare models fitted to a different response (or when the response has been transformed in one case but not the other).
@ Dr. James T. Walker: Also not to the same response considering a different sample size!
make_regression defines a new regression dataset, learn more here:
https://scikit-learn.org/stable/modules/generated/sklearn.datasets.make_regression.html
It’s just the context for the tutorial.
How can your AIC and BIC calculations become negative when there is no negative value in the formula. Log cannot output negative values and I assume that the number of parameters and features are also not able to be negative. So how is that possible?
Hi Nadine…both can be negative. Please review the equations closely.
AIC = -2/N * LL + 2 * k/N
Yes, I know. But this is not how it is implemented here right?
I do not see a minus sign.
Hello Nadine…I am not sure why your view does not show the same equation below:
AIC = –2/N * LL + 2 * k/N
I mean in the code I do not see a minus sign. For AIC is stated:
aic = n * log(mse) + 2 * num_params
And for BIC is stated:
bic = n * log(mse) + num_params * log(n)
So, where is the minus sign?
In the code, there is an alternate form being used.
In the code, there is no minus sign right?
aic = n * log(mse) + 2 * num_params
bic = n * log(mse) + num_params * log(n)
Or am I missing something?
No minus sign in this particular form, however the result can still be negative because log(0.10) = -1
Are AIC and BIC robust measures that will prevent choosing models that overfit to the training data?
@jason and @james nice blog. Can you suggest what should be k or the number of parameters in a Random Forest model when calculating BIC?
Is there any specific formulation to estimate BIC for the random forest model?
Hi Zeshan…You may find the following of interest:
https://medium.com/@analyttica/what-is-bayesian-information-criterion-bic-b3396a894be6
Thank you for your nice post!
Do you think AIC and/or BIC can be used for selecting the best model in standardized major axis (sma) regressions (which considers errors of both x and y variables simultaneously)?
Hi Akihiro…They would both be great options. Have you implemented either or both for your models?
Thank you for your reply.
Calculation of AIC is already implemented in the SMATR package (for standardized major axis (SMA) regression) in R. So I thought, using this package, model selection is possible when I want to select the best SMA model, for example, between
y ~ x
and
y ~ x + group
and
y ~ x * group
where group shows categorical variable.
As the difference between ordinary least square (OLS) regression and SMA is that in the latter case residuals are calculated not only for y direction but also for x, and if residuals are calculated in SMA regression, I thought AIC should also work in selecting models in SMA, However, I could not find a paper/website that says model selection from several different SMA regressions can be possible using AIC.
So do you agree that selecting the best model of SMA using AIC is no problem?
Hi, it’s about model selection using AIC in SMA regressions.
I asked the author of SMATR package in R about this question of mine; he said AIC can be used for selecting the best model of SMA regressions.
Heyy, I have executed the exact codes as above:
aic = n * log(mse) + 2 * num_params
And got results as:
Model1 AIC = -1023.4
Model2 AIC = -730.5
So should I choose Model1 as it’s the least value considering the negative sign, or should I choose Model2 considering the absolute values?
Hi MAR…The following resource may be of interest to you:
https://www.scribbr.com/statistics/akaike-information-criterion/
Dear Dr. Brownlee,
Is the MDL method also called “The minimum message length criterion” ? As far as I know it is not the same. If I’m correct would you recommend a reference to study MML that is as clear as your explanation of AIC, BIC and MDL? Thanks a lot.
Hi MER…The following resource may provide a suitable background for MDL.
https://www.cs.cmu.edu/~aarti/Class/10704/lec13-MDL.pdf