The Autoregressive Integrated Moving Average Model, or ARIMA for short is a standard statistical model for time series forecast and analysis. Along with its development, the authors Box and Jenkins also suggest a process for identifying, estimating, and checking models for a specific time series dataset. This process is now referred to as the Box-Jenkins […]
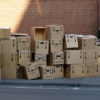