We have arrived at a point where we have implemented and tested the Transformer encoder and decoder separately, and we may now join the two together into a complete model. We will also see how to create padding and look-ahead masks by which we will suppress the input values that will not be considered in the encoder or decoder computations. Our end goal remains to apply the complete model to Natural Language Processing (NLP).
In this tutorial, you will discover how to implement the complete Transformer model and create padding and look-ahead masks.
After completing this tutorial, you will know:
- How to create a padding mask for the encoder and decoder
- How to create a look-ahead mask for the decoder
- How to join the Transformer encoder and decoder into a single model
- How to print out a summary of the encoder and decoder layers
Let’s get started.
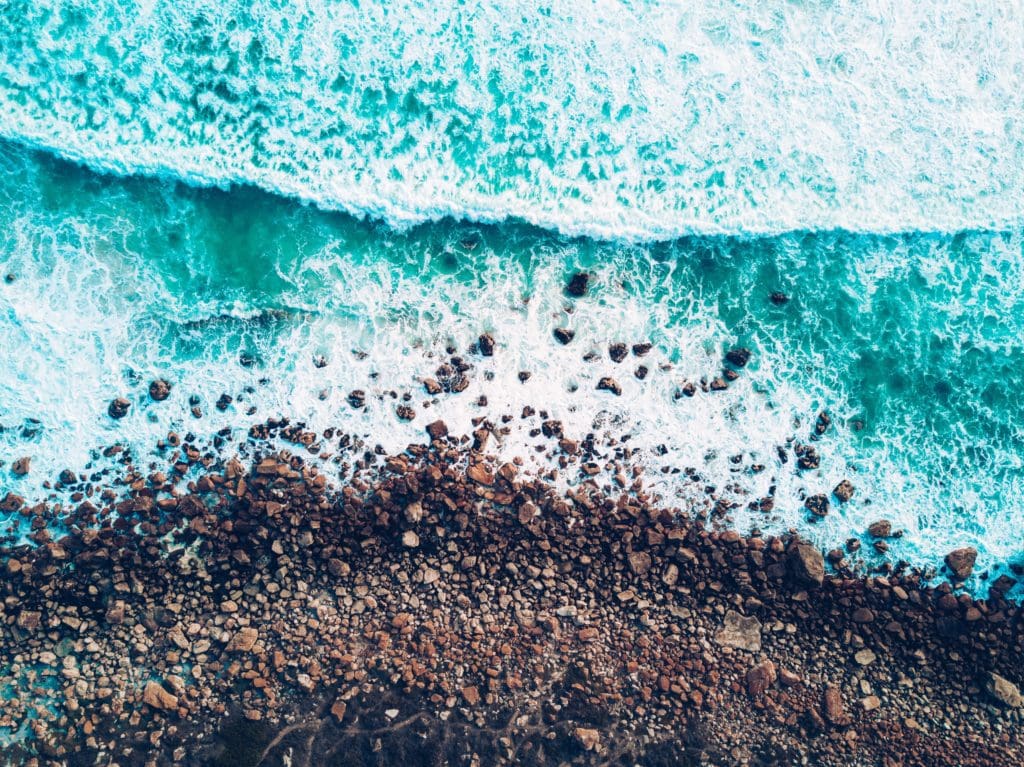
Joining the Transformer encoder and decoder and Masking
Photo by John O’Nolan, some rights reserved.
Tutorial Overview
This tutorial is divided into four parts; they are:
- Recap of the Transformer Architecture
- Masking
- Creating a Padding Mask
- Creating a Look-Ahead Mask
- Joining the Transformer Encoder and Decoder
- Creating an Instance of the Transformer Model
- Printing Out a Summary of the Encoder and Decoder Layers
Prerequisites
For this tutorial, we assume that you are already familiar with:
Recap of the Transformer Architecture
Recall having seen that the Transformer architecture follows an encoder-decoder structure. The encoder, on the left-hand side, is tasked with mapping an input sequence to a sequence of continuous representations; the decoder, on the right-hand side, receives the output of the encoder together with the decoder output at the previous time step to generate an output sequence.
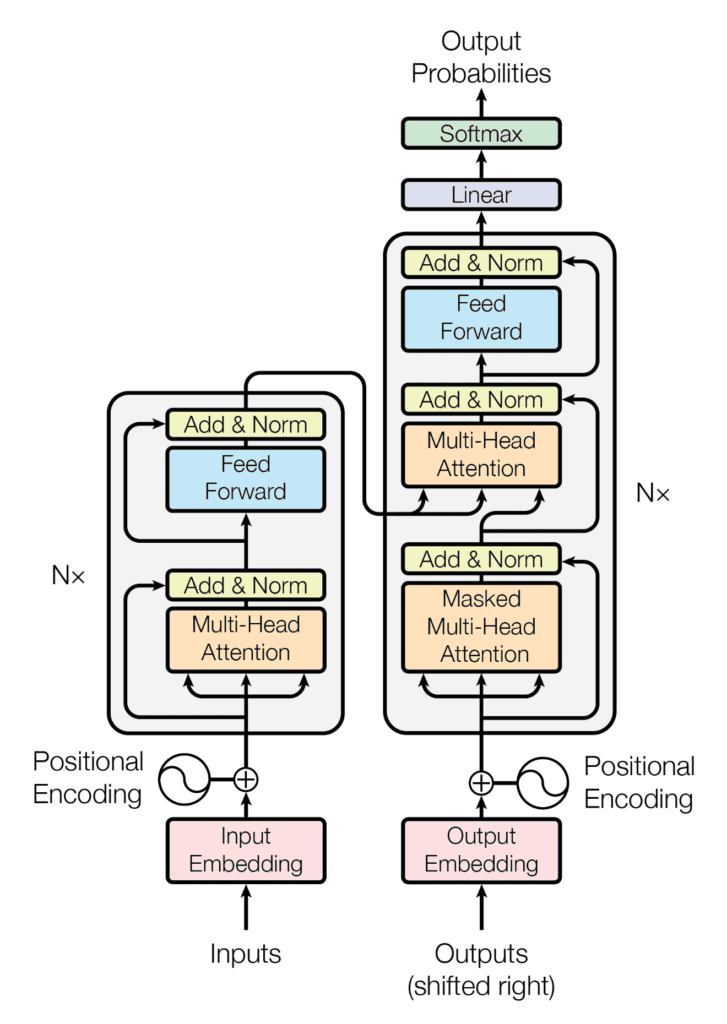
The encoder-decoder structure of the Transformer architecture
Taken from “Attention Is All You Need“
In generating an output sequence, the Transformer does not rely on recurrence and convolutions.
You have seen how to implement the Transformer encoder and decoder separately. In this tutorial, you will join the two into a complete Transformer model and apply padding and look-ahead masking to the input values.
Let’s start first by discovering how to apply masking.
Kick-start your project with my book Building Transformer Models with Attention. It provides self-study tutorials with working code to guide you into building a fully-working transformer model that can
translate sentences from one language to another...
Masking
Creating a Padding Mask
You should already be familiar with the importance of masking the input values before feeding them into the encoder and decoder.
As you will see when you proceed to train the Transformer model, the input sequences fed into the encoder and decoder will first be zero-padded up to a specific sequence length. The importance of having a padding mask is to make sure that these zero values are not processed along with the actual input values by both the encoder and decoder.
Let’s create the following function to generate a padding mask for both the encoder and decoder:
1 2 3 4 5 6 7 8 |
from tensorflow import math, cast, float32 def padding_mask(input): # Create mask which marks the zero padding values in the input by a 1 mask = math.equal(input, 0) mask = cast(mask, float32) return mask |
Upon receiving an input, this function will generate a tensor that marks by a value of one wherever the input contains a value of zero.
Hence, if you input the following array:
1 2 3 4 |
from numpy import array input = array([1, 2, 3, 4, 0, 0, 0]) print(padding_mask(input)) |
Then the output of the padding_mask
function would be the following:
1 |
tf.Tensor([0. 0. 0. 0. 1. 1. 1.], shape=(7,), dtype=float32) |
Creating a Look-Ahead Mask
A look-ahead mask is required to prevent the decoder from attending to succeeding words, such that the prediction for a particular word can only depend on known outputs for the words that come before it.
For this purpose, let’s create the following function to generate a look-ahead mask for the decoder:
1 2 3 4 5 6 7 |
from tensorflow import linalg, ones def lookahead_mask(shape): # Mask out future entries by marking them with a 1.0 mask = 1 - linalg.band_part(ones((shape, shape)), -1, 0) return mask |
You will pass to it the length of the decoder input. Let’s make this length equal to 5, as an example:
1 |
print(lookahead_mask(5)) |
Then the output that the lookahead_mask
function returns is the following:
1 2 3 4 5 6 |
tf.Tensor( [[0. 1. 1. 1. 1.] [0. 0. 1. 1. 1.] [0. 0. 0. 1. 1.] [0. 0. 0. 0. 1.] [0. 0. 0. 0. 0.]], shape=(5, 5), dtype=float32) |
Again, the one values mask out the entries that should not be used. In this manner, the prediction of every word only depends on those that come before it.
Want to Get Started With Building Transformer Models with Attention?
Take my free 12-day email crash course now (with sample code).
Click to sign-up and also get a free PDF Ebook version of the course.
Joining the Transformer Encoder and Decoder
Let’s start by creating the class, TransformerModel
, which inherits from the Model
base class in Keras:
1 2 3 4 5 6 7 8 9 10 11 12 13 |
class TransformerModel(Model): def __init__(self, enc_vocab_size, dec_vocab_size, enc_seq_length, dec_seq_length, h, d_k, d_v, d_model, d_ff_inner, n, rate, **kwargs): super(TransformerModel, self).__init__(**kwargs) # Set up the encoder self.encoder = Encoder(enc_vocab_size, enc_seq_length, h, d_k, d_v, d_model, d_ff_inner, n, rate) # Set up the decoder self.decoder = Decoder(dec_vocab_size, dec_seq_length, h, d_k, d_v, d_model, d_ff_inner, n, rate) # Define the final dense layer self.model_last_layer = Dense(dec_vocab_size) ... |
Our first step in creating the TransformerModel
class is to initialize instances of the Encoder
and Decoder
classes implemented earlier and assign their outputs to the variables, encoder
and decoder
, respectively. If you saved these classes in separate Python scripts, do not forget to import them. I saved my code in the Python scripts encoder.py and decoder.py, so I need to import them accordingly.
You will also include one final dense layer that produces the final output, as in the Transformer architecture of Vaswani et al. (2017).
Next, you shall create the class method, call()
, to feed the relevant inputs into the encoder and decoder.
A padding mask is first generated to mask the encoder input, as well as the encoder output, when this is fed into the second self-attention block of the decoder:
1 2 3 4 5 6 |
... def call(self, encoder_input, decoder_input, training): # Create padding mask to mask the encoder inputs and the encoder outputs in the decoder enc_padding_mask = self.padding_mask(encoder_input) ... |
A padding mask and a look-ahead mask are then generated to mask the decoder input. These are combined together through an element-wise maximum
operation:
1 2 3 4 5 6 |
... # Create and combine padding and look-ahead masks to be fed into the decoder dec_in_padding_mask = self.padding_mask(decoder_input) dec_in_lookahead_mask = self.lookahead_mask(decoder_input.shape[1]) dec_in_lookahead_mask = maximum(dec_in_padding_mask, dec_in_lookahead_mask) ... |
Next, the relevant inputs are fed into the encoder and decoder, and the Transformer model output is generated by feeding the decoder output into one final dense layer:
1 2 3 4 5 6 7 8 9 10 11 |
... # Feed the input into the encoder encoder_output = self.encoder(encoder_input, enc_padding_mask, training) # Feed the encoder output into the decoder decoder_output = self.decoder(decoder_input, encoder_output, dec_in_lookahead_mask, enc_padding_mask, training) # Pass the decoder output through a final dense layer model_output = self.model_last_layer(decoder_output) return model_output |
Combining all the steps gives us the following complete code listing:
1 2 3 4 5 6 7 8 9 10 11 12 13 14 15 16 17 18 19 20 21 22 23 24 25 26 27 28 29 30 31 32 33 34 35 36 37 38 39 40 41 42 43 44 45 46 47 48 49 50 51 52 53 54 55 |
from encoder import Encoder from decoder import Decoder from tensorflow import math, cast, float32, linalg, ones, maximum, newaxis from tensorflow.keras import Model from tensorflow.keras.layers import Dense class TransformerModel(Model): def __init__(self, enc_vocab_size, dec_vocab_size, enc_seq_length, dec_seq_length, h, d_k, d_v, d_model, d_ff_inner, n, rate, **kwargs): super(TransformerModel, self).__init__(**kwargs) # Set up the encoder self.encoder = Encoder(enc_vocab_size, enc_seq_length, h, d_k, d_v, d_model, d_ff_inner, n, rate) # Set up the decoder self.decoder = Decoder(dec_vocab_size, dec_seq_length, h, d_k, d_v, d_model, d_ff_inner, n, rate) # Define the final dense layer self.model_last_layer = Dense(dec_vocab_size) def padding_mask(self, input): # Create mask which marks the zero padding values in the input by a 1.0 mask = math.equal(input, 0) mask = cast(mask, float32) # The shape of the mask should be broadcastable to the shape # of the attention weights that it will be masking later on return mask[:, newaxis, newaxis, :] def lookahead_mask(self, shape): # Mask out future entries by marking them with a 1.0 mask = 1 - linalg.band_part(ones((shape, shape)), -1, 0) return mask def call(self, encoder_input, decoder_input, training): # Create padding mask to mask the encoder inputs and the encoder outputs in the decoder enc_padding_mask = self.padding_mask(encoder_input) # Create and combine padding and look-ahead masks to be fed into the decoder dec_in_padding_mask = self.padding_mask(decoder_input) dec_in_lookahead_mask = self.lookahead_mask(decoder_input.shape[1]) dec_in_lookahead_mask = maximum(dec_in_padding_mask, dec_in_lookahead_mask) # Feed the input into the encoder encoder_output = self.encoder(encoder_input, enc_padding_mask, training) # Feed the encoder output into the decoder decoder_output = self.decoder(decoder_input, encoder_output, dec_in_lookahead_mask, enc_padding_mask, training) # Pass the decoder output through a final dense layer model_output = self.model_last_layer(decoder_output) return model_output |
Note that you have performed a small change to the output that is returned by the padding_mask
function. Its shape is made broadcastable to the shape of the attention weight tensor that it will mask when you train the Transformer model.
Creating an Instance of the Transformer Model
You will work with the parameter values specified in the paper, Attention Is All You Need, by Vaswani et al. (2017):
1 2 3 4 5 6 7 8 9 |
h = 8 # Number of self-attention heads d_k = 64 # Dimensionality of the linearly projected queries and keys d_v = 64 # Dimensionality of the linearly projected values d_ff = 2048 # Dimensionality of the inner fully connected layer d_model = 512 # Dimensionality of the model sub-layers' outputs n = 6 # Number of layers in the encoder stack dropout_rate = 0.1 # Frequency of dropping the input units in the dropout layers ... |
As for the input-related parameters, you will work with dummy values for now until you arrive at the stage of training the complete Transformer model. At that point, you will use actual sentences:
1 2 3 4 5 6 7 |
... enc_vocab_size = 20 # Vocabulary size for the encoder dec_vocab_size = 20 # Vocabulary size for the decoder enc_seq_length = 5 # Maximum length of the input sequence dec_seq_length = 5 # Maximum length of the target sequence ... |
You can now create an instance of the TransformerModel
class as follows:
1 2 3 4 |
from model import TransformerModel # Create model training_model = TransformerModel(enc_vocab_size, dec_vocab_size, enc_seq_length, dec_seq_length, h, d_k, d_v, d_model, d_ff, n, dropout_rate) |
The complete code listing is as follows:
1 2 3 4 5 6 7 8 9 10 11 12 13 14 15 16 17 |
enc_vocab_size = 20 # Vocabulary size for the encoder dec_vocab_size = 20 # Vocabulary size for the decoder enc_seq_length = 5 # Maximum length of the input sequence dec_seq_length = 5 # Maximum length of the target sequence h = 8 # Number of self-attention heads d_k = 64 # Dimensionality of the linearly projected queries and keys d_v = 64 # Dimensionality of the linearly projected values d_ff = 2048 # Dimensionality of the inner fully connected layer d_model = 512 # Dimensionality of the model sub-layers' outputs n = 6 # Number of layers in the encoder stack dropout_rate = 0.1 # Frequency of dropping the input units in the dropout layers # Create model training_model = TransformerModel(enc_vocab_size, dec_vocab_size, enc_seq_length, dec_seq_length, h, d_k, d_v, d_model, d_ff, n, dropout_rate) |
Printing Out a Summary of the Encoder and Decoder Layers
You may also print out a summary of the encoder and decoder blocks of the Transformer model. The choice to print them out separately will allow you to be able to see the details of their individual sub-layers. In order to do so, add the following line of code to the __init__()
method of both the EncoderLayer
and DecoderLayer
classes:
1 |
self.build(input_shape=[None, sequence_length, d_model]) |
Then you need to add the following method to the EncoderLayer
class:
1 2 3 |
def build_graph(self): input_layer = Input(shape=(self.sequence_length, self.d_model)) return Model(inputs=[input_layer], outputs=self.call(input_layer, None, True)) |
And the following method to the DecoderLayer
class:
1 2 3 |
def build_graph(self): input_layer = Input(shape=(self.sequence_length, self.d_model)) return Model(inputs=[input_layer], outputs=self.call(input_layer, input_layer, None, None, True)) |
This results in the EncoderLayer
class being modified as follows (the three dots under the call()
method mean that this remains the same as the one that was implemented here):
1 2 3 4 5 6 7 8 9 10 11 12 13 14 15 16 17 18 19 20 21 22 |
from tensorflow.keras.layers import Input from tensorflow.keras import Model class EncoderLayer(Layer): def __init__(self, sequence_length, h, d_k, d_v, d_model, d_ff, rate, **kwargs): super(EncoderLayer, self).__init__(**kwargs) self.build(input_shape=[None, sequence_length, d_model]) self.d_model = d_model self.sequence_length = sequence_length self.multihead_attention = MultiHeadAttention(h, d_k, d_v, d_model) self.dropout1 = Dropout(rate) self.add_norm1 = AddNormalization() self.feed_forward = FeedForward(d_ff, d_model) self.dropout2 = Dropout(rate) self.add_norm2 = AddNormalization() def build_graph(self): input_layer = Input(shape=(self.sequence_length, self.d_model)) return Model(inputs=[input_layer], outputs=self.call(input_layer, None, True)) def call(self, x, padding_mask, training): ... |
Similar changes can be made to the DecoderLayer
class too.
Once you have the necessary changes in place, you can proceed to create instances of the EncoderLayer
and DecoderLayer
classes and print out their summaries as follows:
1 2 3 4 5 6 7 8 |
from encoder import EncoderLayer from decoder import DecoderLayer encoder = EncoderLayer(enc_seq_length, h, d_k, d_v, d_model, d_ff, dropout_rate) encoder.build_graph().summary() decoder = DecoderLayer(dec_seq_length, h, d_k, d_v, d_model, d_ff, dropout_rate) decoder.build_graph().summary() |
The resulting summary for the encoder is the following:
1 2 3 4 5 6 7 8 9 10 11 12 13 14 15 16 17 18 19 20 21 22 23 24 25 26 27 |
Model: "model" __________________________________________________________________________________________________ Layer (type) Output Shape Param # Connected to ================================================================================================== input_1 (InputLayer) [(None, 5, 512)] 0 [] multi_head_attention_18 (Multi (None, 5, 512) 131776 ['input_1[0][0]', HeadAttention) 'input_1[0][0]', 'input_1[0][0]'] dropout_32 (Dropout) (None, 5, 512) 0 ['multi_head_attention_18[0][0]'] add_normalization_30 (AddNorma (None, 5, 512) 1024 ['input_1[0][0]', lization) 'dropout_32[0][0]'] feed_forward_12 (FeedForward) (None, 5, 512) 2099712 ['add_normalization_30[0][0]'] dropout_33 (Dropout) (None, 5, 512) 0 ['feed_forward_12[0][0]'] add_normalization_31 (AddNorma (None, 5, 512) 1024 ['add_normalization_30[0][0]', lization) 'dropout_33[0][0]'] ================================================================================================== Total params: 2,233,536 Trainable params: 2,233,536 Non-trainable params: 0 __________________________________________________________________________________________________ |
While the resulting summary for the decoder is the following:
1 2 3 4 5 6 7 8 9 10 11 12 13 14 15 16 17 18 19 20 21 22 23 24 25 26 27 28 29 30 31 32 33 34 35 |
Model: "model_1" __________________________________________________________________________________________________ Layer (type) Output Shape Param # Connected to ================================================================================================== input_2 (InputLayer) [(None, 5, 512)] 0 [] multi_head_attention_19 (Multi (None, 5, 512) 131776 ['input_2[0][0]', HeadAttention) 'input_2[0][0]', 'input_2[0][0]'] dropout_34 (Dropout) (None, 5, 512) 0 ['multi_head_attention_19[0][0]'] add_normalization_32 (AddNorma (None, 5, 512) 1024 ['input_2[0][0]', lization) 'dropout_34[0][0]', 'add_normalization_32[0][0]', 'dropout_35[0][0]'] multi_head_attention_20 (Multi (None, 5, 512) 131776 ['add_normalization_32[0][0]', HeadAttention) 'input_2[0][0]', 'input_2[0][0]'] dropout_35 (Dropout) (None, 5, 512) 0 ['multi_head_attention_20[0][0]'] feed_forward_13 (FeedForward) (None, 5, 512) 2099712 ['add_normalization_32[1][0]'] dropout_36 (Dropout) (None, 5, 512) 0 ['feed_forward_13[0][0]'] add_normalization_34 (AddNorma (None, 5, 512) 1024 ['add_normalization_32[1][0]', lization) 'dropout_36[0][0]'] ================================================================================================== Total params: 2,365,312 Trainable params: 2,365,312 Non-trainable params: 0 __________________________________________________________________________________________________ |
Further Reading
This section provides more resources on the topic if you are looking to go deeper.
Books
Papers
Summary
In this tutorial, you discovered how to implement the complete Transformer model and create padding and look-ahead masks.
Specifically, you learned:
- How to create a padding mask for the encoder and decoder
- How to create a look-ahead mask for the decoder
- How to join the Transformer encoder and decoder into a single model
- How to print out a summary of the encoder and decoder layers
Do you have any questions?
Ask your questions in the comments below and I will do my best to answer.
Thanks for your great tutorial!
I found some errors when printing out the encoder and decoder structure information.
It seems the reason is in the MultiHeadAttention/reshape_tensor function. Precisely, reshape is not correctly working for tensors with None dimension.
I solved the problem by changing the function as below.
_________________________________________________
def reshape_tensor(self, x, heads, flag):
if flag:
# Tensor shape after reshaping and transposing: (batch_size, heads, seq_length, -1)
x = reshape(x, shape=(shape(x)[0], shape(x)[1], heads, int(x.shape[2]/heads)))
x = transpose(x, perm=(0, 2, 1, 3))
else:
# Reverting the reshaping and transposing operations: (batch_size, seq_length, d_model)
x = transpose(x, perm=(0, 2, 1, 3))
x = reshape(x, shape=(shape(x)[0], shape(x)[1], int(x.shape[2]*x.shape[3])))
return x
_________________________________________________
It seems weird somehow. I want to know if there are any better solutions. Thanks again!
Thank you Helen for your feedback! What specific error messages did you encounter so that we may take note of any code listings that require review.
Thanks for your reply! The error is like below.
in test_transformer
encoder.build_graph().summary()
in build_graph
return Model(inputs=[input_layer], outputs=self.call(input_layer, None, True))
in call
multihead_output = self.multihead_attention(x, x, x, padding_mask)
in call *
return self.W_o(output)
ValueError: The last dimension of the inputs to a Dense layer should be defined. Found None. Full input shape received: (None, 5, None)
Call arguments received by layer “multi_head_attention_18” (type MultiHeadAttention):
\u2022 queries=tf.Tensor(shape=(None, 5, 512), dtype=float32)
\u2022 keys=tf.Tensor(shape=(None, 5, 512), dtype=float32)
\u2022 values=tf.Tensor(shape=(None, 5, 512), dtype=float32)
\u2022 mask=None
The last part of adding graph output is quite confusing.
It invovles many changes in different places.
In the end, I still got some error:
49
50 def build_graph(self):
—> 51 input_layer = Input(shape=(self.sequence_length, self.d_model))
52 return Model(inputs=[input_layer], outputs=self.call(input_layer, None, True))
53 def call(self, x, padding_mask, training):
The collab reproducing the error:
NameError: name ‘Input’ is not defined
I had the same error.
Input layer didn’t imported in import section. Append Input at the end of the first line
from tensorflow.keras.layers import LayerNormalization, Layer, Dense, ReLU, Dropout, Input
I got the following error when building the decoder summary, same as encoder summary
TypeError Traceback (most recent call last)
in
2 # encoder.build_graph().summary()
3
—-> 4 decoder = DecoderLayer(dec_seq_length, h, d_k, d_v, d_model, d_ff, dropout_rate)
5 decoder.build_graph().summary()
TypeError: __init__() takes 7 positional arguments but 8 were given
Hi Michel…I would recommend trying the code in Google Colab.
Check the __init__ function in the DecoderLayer class.
Book version before this chapter don’t have dec_seq_length (only 7 input parameter), but if you look closely at the constructor in this chapter there are multiple changes that are not mentioned in the text:
class EncoderLayer(Layer):
# ——— sequence_length added
def __init__(self, sequence_length, h, d_k, d_v, d_model, d_ff, rate, **kwargs):
super().__init__(**kwargs)
self.build(input_shape=[None, sequence_length, d_model])
# ——– below two lines are added
self.d_model = d_model
self.sequence_length = sequence_length
self.multihead_attention = MultiHeadAttention(h, d_k, d_v, d_model)
self.dropout1 = Dropout(rate)
self.add_norm1 = AddNormalization()
self.feed_forward = FeedForward(d_ff, d_model)
self.dropout2 = Dropout(rate)
If you introduce all these changes, then build_graph will work, but everything else most probably will be broken
ValueError Traceback (most recent call last)
in
2
3 encoder = EncoderLayer(enc_seq_length, h, d_k, d_v, d_model, d_ff, dropout_rate)
—-> 4 encoder.build_graph().summary()
5
6 decoder = DecoderLayer(dec_seq_length, h, d_k, d_v, d_model, d_ff, dropout_rate)
3 frames
/tmp/__autograph_generated_file2sd23ix_.py in tf__call(self, queries, keys, values, mask)
15 try:
16 do_return = True
—> 17 retval_ = ag__.converted_call(ag__.ld(self).W_o, (ag__.ld(output),), None, fscope)
18 except:
19 do_return = False
ValueError: Exception encountered when calling layer “multi_head_attention_155” (type MultiHeadAttention).
in user code:
File “”, line 48, in call *
return self.W_o(output)
File “/usr/local/lib/python3.7/dist-packages/keras/utils/traceback_utils.py”, line 67, in error_handler **
raise e.with_traceback(filtered_tb) from None
File “/usr/local/lib/python3.7/dist-packages/keras/layers/core/dense.py”, line 141, in build
raise ValueError(‘The last dimension of the inputs to a Dense layer ‘
ValueError: The last dimension of the inputs to a Dense layer should be defined. Found None. Full input shape received: (None, 5, None)
Call arguments received by layer “multi_head_attention_155” (type MultiHeadAttention):
• queries=tf.Tensor(shape=(None, 5, 512), dtype=float32)
• keys=tf.Tensor(shape=(None, 5, 512), dtype=float32)
• values=tf.Tensor(shape=(None, 5, 512), dtype=float32)
• mask=None
Hi Michel…Did you type in the code or copy and paste it? You may wish to try it Google Colab.
This error appears due to constructor in the class EncoderLayer changed, but class Encoder still rely upon previous implementation. To fix the problem Encoder-class must take into account new constructor-parameter seq_length.
class Transformer(tf.keras.Model):
def __init__(self, num_layers, d_model, num_heads, dff, input_vocab_size, target_vocab_size, pe_input, pe_target, rate=0.1):
super(Transformer, self).__init__()
self.encoder = Encoder(num_layers, d_model, num_heads, dff, input_vocab_size, pe_input, rate)
self.decoder = Decoder(num_layers, d_model, num_heads, dff, target_vocab_size, pe_target, rate)
self.final_layer = tf.keras.layers.Dense(target_vocab_size)
def call(self, inp, tar, training, enc_padding_mask, look_ahead_mask, dec_padding_mask):
enc_output = self.encoder(inp, training, enc_padding_mask)
dec_output, attention_weights = self.decoder(tar, enc_output, training, look_ahead_mask, dec_padding_mask)
final_output = self.final_layer(dec_output)
return final_output, attention_weights
NOTE: rest of the code for transformer/training of transformer is similar to these blogs
.
.
.
.
.
.
Here is my Transformer class… during training this subclass model, after every 3 epochs I was trying to save the subclass model using this line of code: `trainer.save(‘net’, save_format=’tf’)
but I was getting this error:
TypeError: tf__call() missing 3 required positional arguments: ‘enc_padding_mask’, ‘look_ahead_mask’, and ‘dec_padding_mask’
could you please suggest me the solution of it ?
Hi Shivam…The following discussion may prove beneficial:
https://stackoverflow.com/questions/61631360/self-defined-tensorflow-decoder-typeerror-call-missing-1-required-positio
Hello,
your call function declaration is
def call(self, inp, tar, training, enc_padding_mask, look_ahead_mask, dec_padding_mask):
which has three new mask-parameters.
If rest code is the same as in the book, then Transformer.call() doesn’t have these new parameters.
Hi,
Thanks for a great tutorial! How can I implement this is in transformers package by Hugging face?
Hi Vik…The following resource is a great starting point:
https://machinelearningmastery.com/a-brief-introduction-to-bert/
Hello experts,
While reading this chapter and running many experiments, I noticed a behavior that I’d think is incorrect. Wanted to know your opinion.
Note: in the below explanation
*) loosely following types int vs float
*) loosely using terms “tokens” vs “words”, which is the same in this case
Sorry for that.
— Padding mask —
let’s assume:
input_seq_len = 9 # words in encoded sentence
input_seq = [[2,3,4,5,0,0,0,0,0]]
In class TransformerModel.padding_mask:
mask = padding_mask(input_seq)
# tensor [[[[0,0,0,0,1,1,1,1,1]]]], shape (1,1,1,9)
# first “1” is a batch
# second and third “1”-s are tf.newaxis
# last dimension is an actual mask
Idea of this mask is to prohibit “0”-token to attend to actual words (2,3,4,5)
Let’s see what is happening in DotProductAttention.call line:
scores += -1e9 * mask
# scores.shape (1, heads, 9, 9)
# “1” is a batch
# 9×9 is an attention score matrix, which shows how each token must attend to another token
When tensorflow multiplies (9, 9)-score to (1, 9)-mask matrices broadcast rule applies. Which produces scores in the following form (* is -1e9):
[x11, x12, x13, x14, *,*,*,*,*]
[x21, x22, x23, x24, *,*,*,*,*]
[x31, x32, x33, x34, *,*,*,*,*]
[x41, x42, x43, x44, *,*,*,*,*]
[x51, x52, x53, x54, *,*,*,*,*]
[x61, x62, x63, x64, *,*,*,*,*]
[x71, x72, x73, x74, *,*,*,*,*]
[x81, x82, x83, x84, *,*,*,*,*]
[x91, x92, x93, x94, *,*,*,*,*]
Observtion 1)
Above matrix allows 5-th, 6-th, 7-th, 8-th and 9-th tokens attend to 1-st, 2-nd, 3-rd and 4-th. I think above matrix must be
[x11, x12, x13, x14, *,*,*,*,*]
[x21, x22, x23, x24, *,*,*,*,*]
[x31, x32, x33, x34, *,*,*,*,*]
[x41, x42, x43, x44, *,*,*,*,*]
[ *, *, *, *, *,*,*,*,*]
[ *, *, *, *, *,*,*,*,*]
[ *, *, *, *, *,*,*,*,*]
[ *, *, *, *, *,*,*,*,*]
[ *, *, *, *, *,*,*,*,*]
Solution 1)
If observation 1 is correct
def PaddingMask(self, input):
mask = tf.equal(input, 0)
mask = tf.cast(mask, tf.float32)
mask = mask[:, tf.newaxis, tf.newaxis, :]
new_mask = tf.maximum(mask, tf.transpose(mask, perm=(0,1,3,2)))
return new_mask
— Lookahead mask combined with padding mask —
Very same setup.
In the TransformerModel.call():
dec_lookahead_mask = tf.maximum(dec_lookahead_mask_pre, dec_padding_mask)
will looks like:
[1,0,0,0,0,0,0,0,0]
[1,1,0,0,0,0,0,0,0]
[1,1,1,0,0,0,0,0,0]
[1,1,1,1,0,0,0,0,0]
[1,1,1,1,0,0,0,0,0]
[1,1,1,1,0,0,0,0,0]
[1,1,1,1,0,0,0,0,0]
[1,1,1,1,0,0,0,0,0]
[1,1,1,1,0,0,0,0,0]
But should be:
[1,0,0,0,0,0,0,0,0]
[1,1,0,0,0,0,0,0,0]
[1,1,1,0,0,0,0,0,0]
[1,1,1,1,0,0,0,0,0]
[0,0,0,0,0,0,0,0,0]
[0,0,0,0,0,0,0,0,0]
[0,0,0,0,0,0,0,0,0]
[0,0,0,0,0,0,0,0,0]
[0,0,0,0,0,0,0,0,0]
Observation 2)
Above mask allows attend zero-tokens to actual words.
Solution 2)
If observation 2 is valid, Solution(1) will fix Observation (2) as well
I totally agree with your observation. Zero tokens should not be allow to attend to actual tokens so score matrix should be blockwise non-zero. However, your solution generates smth like
array([[[[0., 0., 1., 1.],
[0., 0., 1., 1.],
[1., 1., 1., 1.],
[1., 1., 1., 1.]]]], dtype=float32)>
if we have, for example, a sequence [2,4,0,0]. This array does not work in the line
scores = scores + mask * -1e9
in the implementation of masking in dot product attention. The reason is obviously due to softmax operating row-wise: attention scores of zero tokens will ofc change by -1e9, however, all of them uniformly do so and softmax eventually normalizes them to equal values.
In my message I messed up with ones and zeros, but code still correct.
In a padding/lookahead mask 1 means – should be masked.
When 1 multiplies to -1e9 it became very small number. So mask should have ones in the position that must be masked.
scores += -1e9 * mask
# here are shapes:
# (1, 8, 4, 4) + -1e9 * (1, 1, 4, 4) – works ok, due to broadcasting
Hi, Dr Jason Brownlee
i met a problem when run the whole transformer model:
when processed by batch_size,
the encoder_input shape is [batch_size, max_len_eng – 1] after the execution of “encoder_input = trainX_batch[:, 1:]”
the decoder_input shape is [batch_size, max_len_ger – 1] after the execution of “decoder_input = trainY_batch[:, :-1]”
so when max_len_eng is not equal to max_len_ger,
the execution of combining two types of mask by “tensorflow.maximum(dec_in_padding_mask, dec_in_lookahead_mask)” raise an error as follows:
ValueError: Dimensions must be equal, but are 64 and 23 for ‘{{node transformer_model_complete/Maximum}} = Maximum[T=DT_FLOAT](transformer_model_complete/Cast_1, transformer_model_complete/sub)’ with input shapes: [64,23], [23,23].
can you help me to figure it out ?
Hi David…The following discussion may be of interest:
https://stackoverflow.com/questions/56302243/keras-valueerror-dimensions-must-be-equal-issue
sorry, James, i don’t think this is related to your posted url.
when masking the encoder input, i follow this tutorial: https://machinelearningmastery.com/joining-the-transformer-encoder-and-decoder-and-masking/
the key part of code is:
# Create and combine padding and look-ahead masks to be fed into the decoder
dec_in_padding_mask = self.padding_mask(decoder_input)
dec_in_lookahead_mask = self.lookahead_mask(decoder_input.shape[1])
dec_in_lookahead_mask = maximum(dec_in_padding_mask, dec_in_lookahead_mask)
in the above three lines, decoder_input’s shape is [batch_size, max_len_source_lang], for example [64, 25]
after executing “dec_in_padding_mask = self.padding_mask(decoder_input)”,
dec_in_padding_mask’s shape is [64, 25]
the decoder_input’s shape is [batch_size, max_len_target_lang – 1], for example [64, 23]
after executing “dec_in_lookahead_mask = self.lookahead_mask(decoder_input.shape[1])”
dec_in_lookahead_mask is a square matrix with shape [23, 23]
so, why can these two different shape tensor sent to maximum function, that’s the error i got when i start trainging the model.
sorry, i type the tensor’s shape wrong, so i write this reply again:
i don’t think this is related to your posted url or i didn’t find the exactly right comment in that stackover problem.
My question is:
when masking the encoder input, i follow this tutorial: https://machinelearningmastery.com/joining-the-transformer-encoder-and-decoder-and-masking/
the key part of code is:
# Create and combine padding and look-ahead masks to be fed into the decoder
dec_in_padding_mask = self.padding_mask(decoder_input)
dec_in_lookahead_mask = self.lookahead_mask(decoder_input.shape[1])
dec_in_lookahead_mask = maximum(dec_in_padding_mask, dec_in_lookahead_mask)
in the above three lines, decoder_input’s shape is [batch_size, max_len_target_lang – 1], for example [64, 23]
after executing “dec_in_padding_mask = self.padding_mask(decoder_input)”,
dec_in_padding_mask’s shape is [64, 23]
the decoder_input’s shape is [batch_size, max_len_target_lang – 1], for example [64, 23]
after executing “dec_in_lookahead_mask = self.lookahead_mask(decoder_input.shape[1])”
dec_in_lookahead_mask is a square matrix with shape [23, 23]
so, why can these two different shape tensor([64, 23] vs [23, 23]) sent to maximum function, that’s the error i got when i start trainging the model.
Hello David, the shapes of the tensors that you are mentioning should be the following:
– decoder_input: [batch_size, dec_seq_length – 1]
– dec_in_padding_mask: [batch_size, 1, 1, dec_seq_length – 1]
– dec_in_lookahead_mask: [batch_size, 1, dec_seq_length – 1, dec_seq_length – 1]
For example, I’m using a dec_seq_length = 12 with a batch_size = 64, hence I have the following tensor shapes:
– decoder_input: [64, 11]
– dec_in_padding_mask: [64, 1, 1, 11]
– dec_in_lookahead_mask: [64, 1, 11, 11]
Can you step into the code to check why your tensor shapes are different from what is expected?
Thanks Cristina for your help. I find that i directly used the demo “padding_mask” function in the front part of this tutorial which only returns “mask” variable, actually, a same name of this function in the final format returns “[:, newaxis, newaxis, :]”, so that’s the reason i cannot maximum those two padded mask tensors.
Thank you for the clarification, David.
I want to provide a quick work-around for people stuck at the last stage: print the model summary.
You can use dummy variables like we did in previous chapters.
batch_size = 64
my_encoder_input = np.random.random((batch_size, enc_seq_length))
my_decoder_input = np.random.random((batch_size, dec_seq_length))
output = training_model(my_encoder_input, my_decoder_input, True)
–> output should have shape (batch_size, dec_seq_length, dec_vocab_size)
Bonus: now that the training_model has been instantiated, you can run
training_model.summary()
and see that our model has more 27 million parameters!
Thank you Florian for you willingness to share with the community!
Excuse me, But my dataset is lists numbers of 3-element lists like: [[],[],[]], [[],[],[]],..
dataset = [[1, 2, 3], [4, 5, 6], [7, 8, 9]]
[[10, 11, 12], [13, 14, 15], [16, 17, 18]], …
it’s a classification problem with 5 classes of 1,2,3,4,5.
How should I use transformers models for this sequence classification problem? I want to use attention method.
Thank you for your help
Hi Mohammad…The following resource may be of interest to you:
https://machinelearningmastery.com/transformer-models-with-attention/