Boosting is a class of ensemble machine learning algorithms that involve combining the predictions from many weak learners.
A weak learner is a model that is very simple, although has some skill on the dataset. Boosting was a theoretical concept long before a practical algorithm could be developed, and the AdaBoost (adaptive boosting) algorithm was the first successful approach for the idea.
The AdaBoost algorithm involves using very short (one-level) decision trees as weak learners that are added sequentially to the ensemble. Each subsequent model attempts to correct the predictions made by the model before it in the sequence. This is achieved by weighing the training dataset to put more focus on training examples on which prior models made prediction errors.
In this tutorial, you will discover how to develop AdaBoost ensembles for classification and regression.
After completing this tutorial, you will know:
- AdaBoost ensemble is an ensemble created from decision trees added sequentially to the model
- How to use the AdaBoost ensemble for classification and regression with scikit-learn.
- How to explore the effect of AdaBoost model hyperparameters on model performance.
Kick-start your project with my new book Ensemble Learning Algorithms With Python, including step-by-step tutorials and the Python source code files for all examples.
Let’s get started.
- Updated Aug/2020: Added example of grid searching model hyperparameters.
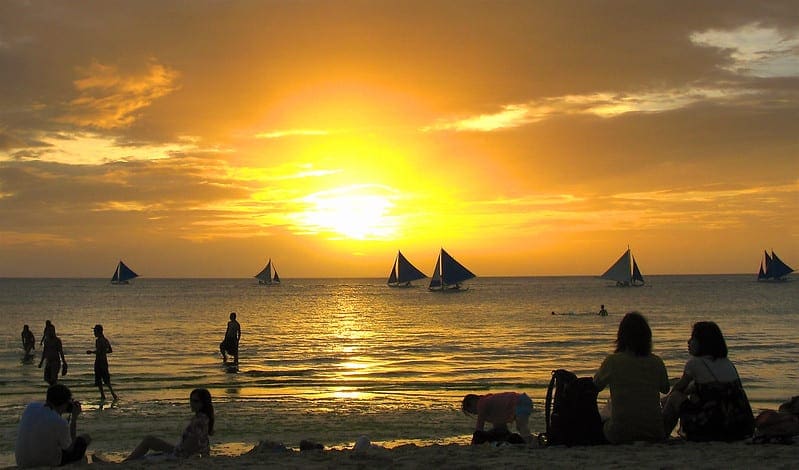
How to Develop an AdaBoost Ensemble in Python
Photo by Ray in Manila, some rights reserved.
Tutorial Overview
This tutorial is divided into four parts; they are:
- AdaBoost Ensemble Algorithm
- AdaBoost Scikit-Learn API
- AdaBoost for Classification
- AdaBoost for Regression
- AdaBoost Hyperparameters
- Explore Number of Trees
- Explore Weak Learner
- Explore Learning Rate
- Explore Alternate Algorithm
- Grid Search AdaBoost Hyperparameters
AdaBoost Ensemble Algorithm
Boosting refers to a class of machine learning ensemble algorithms where models are added sequentially and later models in the sequence correct the predictions made by earlier models in the sequence.
AdaBoost, short for “Adaptive Boosting,” is a boosting ensemble machine learning algorithm, and was one of the first successful boosting approaches.
We call the algorithm AdaBoost because, unlike previous algorithms, it adjusts adaptively to the errors of the weak hypotheses
— A Decision-Theoretic Generalization of on-Line Learning and an Application to Boosting, 1996.
AdaBoost combines the predictions from short one-level decision trees, called decision stumps, although other algorithms can also be used. Decision stump algorithms are used as the AdaBoost algorithm seeks to use many weak models and correct their predictions by adding additional weak models.
The training algorithm involves starting with one decision tree, finding those examples in the training dataset that were misclassified, and adding more weight to those examples. Another tree is trained on the same data, although now weighted by the misclassification errors. This process is repeated until a desired number of trees are added.
If a training data point is misclassified, the weight of that training data point is increased (boosted). A second classifier is built using the new weights, which are no longer equal. Again, misclassified training data have their weights boosted and the procedure is repeated.
— Multi-class AdaBoost, 2009.
The algorithm was developed for classification and involves combining the predictions made by all decision trees in the ensemble. A similar approach was also developed for regression problems where predictions are made by using the average of the decision trees. The contribution of each model to the ensemble prediction is weighted based on the performance of the model on the training dataset.
… the new algorithm needs no prior knowledge of the accuracies of the weak hypotheses. Rather, it adapts to these accuracies and generates a weighted majority hypothesis in which the weight of each weak hypothesis is a function of its accuracy.
— A Decision-Theoretic Generalization of on-Line Learning and an Application to Boosting, 1996.
Now that we are familiar with the AdaBoost algorithm, let’s look at how we can fit AdaBoost models in Python.
Want to Get Started With Ensemble Learning?
Take my free 7-day email crash course now (with sample code).
Click to sign-up and also get a free PDF Ebook version of the course.
AdaBoost Scikit-Learn API
AdaBoost ensembles can be implemented from scratch, although this can be challenging for beginners.
For an example, see the tutorial:
The scikit-learn Python machine learning library provides an implementation of AdaBoost ensembles for machine learning.
It is available in a modern version of the library.
First, confirm that you are using a modern version of the library by running the following script:
1 2 3 |
# check scikit-learn version import sklearn print(sklearn.__version__) |
Running the script will print your version of scikit-learn.
Your version should be the same or higher. If not, you must upgrade your version of the scikit-learn library.
1 |
0.22.1 |
AdaBoost is provided via the AdaBoostRegressor and AdaBoostClassifier classes.
Both models operate the same way and take the same arguments that influence how the decision trees are created.
Randomness is used in the construction of the model. This means that each time the algorithm is run on the same data, it will produce a slightly different model.
When using machine learning algorithms that have a stochastic learning algorithm, it is good practice to evaluate them by averaging their performance across multiple runs or repeats of cross-validation. When fitting a final model it may be desirable to either increase the number of trees until the variance of the model is reduced across repeated evaluations, or to fit multiple final models and average their predictions.
Let’s take a look at how to develop an AdaBoost ensemble for both classification and regression.
AdaBoost for Classification
In this section, we will look at using AdaBoost for a classification problem.
First, we can use the make_classification() function to create a synthetic binary classification problem with 1,000 examples and 20 input features.
The complete example is listed below.
1 2 3 4 5 6 |
# test classification dataset from sklearn.datasets import make_classification # define dataset X, y = make_classification(n_samples=1000, n_features=20, n_informative=15, n_redundant=5, random_state=6) # summarize the dataset print(X.shape, y.shape) |
Running the example creates the dataset and summarizes the shape of the input and output components.
1 |
(1000, 20) (1000,) |
Next, we can evaluate an AdaBoost algorithm on this dataset.
We will evaluate the model using repeated stratified k-fold cross-validation, with three repeats and 10 folds. We will report the mean and standard deviation of the accuracy of the model across all repeats and folds.
1 2 3 4 5 6 7 8 9 10 11 12 13 14 15 16 |
# evaluate adaboost algorithm for classification from numpy import mean from numpy import std from sklearn.datasets import make_classification from sklearn.model_selection import cross_val_score from sklearn.model_selection import RepeatedStratifiedKFold from sklearn.ensemble import AdaBoostClassifier # define dataset X, y = make_classification(n_samples=1000, n_features=20, n_informative=15, n_redundant=5, random_state=6) # define the model model = AdaBoostClassifier() # evaluate the model cv = RepeatedStratifiedKFold(n_splits=10, n_repeats=3, random_state=1) n_scores = cross_val_score(model, X, y, scoring='accuracy', cv=cv, n_jobs=-1, error_score='raise') # report performance print('Accuracy: %.3f (%.3f)' % (mean(n_scores), std(n_scores))) |
Running the example reports the mean and standard deviation accuracy of the model.
Note: Your results may vary given the stochastic nature of the algorithm or evaluation procedure, or differences in numerical precision. Consider running the example a few times and compare the average outcome.
In this case, we can see the AdaBoost ensemble with default hyperparameters achieves a classification accuracy of about 80 percent on this test dataset.
1 |
Accuracy: 0.806 (0.041) |
We can also use the AdaBoost model as a final model and make predictions for classification.
First, the AdaBoost ensemble is fit on all available data, then the predict() function can be called to make predictions on new data.
The example below demonstrates this on our binary classification dataset.
1 2 3 4 5 6 7 8 9 10 11 12 13 |
# make predictions using adaboost for classification from sklearn.datasets import make_classification from sklearn.ensemble import AdaBoostClassifier # define dataset X, y = make_classification(n_samples=1000, n_features=20, n_informative=15, n_redundant=5, random_state=6) # define the model model = AdaBoostClassifier() # fit the model on the whole dataset model.fit(X, y) # make a single prediction row = [[-3.47224758,1.95378146,0.04875169,-0.91592588,-3.54022468,1.96405547,-7.72564954,-2.64787168,-1.81726906,-1.67104974,2.33762043,-4.30273117,0.4839841,-1.28253034,-10.6704077,-0.7641103,-3.58493721,2.07283886,0.08385173,0.91461126]] yhat = model.predict(row) print('Predicted Class: %d' % yhat[0]) |
Running the example fits the AdaBoost ensemble model on the entire dataset and is then used to make a prediction on a new row of data, as we might when using the model in an application.
1 |
Predicted Class: 0 |
Now that we are familiar with using AdaBoost for classification, let’s look at the API for regression.
AdaBoost for Regression
In this section, we will look at using AdaBoost for a regression problem.
First, we can use the make_regression() function to create a synthetic regression problem with 1,000 examples and 20 input features.
The complete example is listed below.
1 2 3 4 5 6 |
# test regression dataset from sklearn.datasets import make_regression # define dataset X, y = make_regression(n_samples=1000, n_features=20, n_informative=15, noise=0.1, random_state=6) # summarize the dataset print(X.shape, y.shape) |
Running the example creates the dataset and summarizes the shape of the input and output components.
1 |
(1000, 20) (1000,) |
Next, we can evaluate an AdaBoost algorithm on this dataset.
As we did with the last section, we will evaluate the model using repeated k-fold cross-validation, with three repeats and 10 folds. We will report the mean absolute error (MAE) of the model across all repeats and folds. The scikit-learn library makes the MAE negative so that it is maximized instead of minimized. This means that larger negative MAE are better and a perfect model has a MAE of 0.
The complete example is listed below.
1 2 3 4 5 6 7 8 9 10 11 12 13 14 15 16 |
# evaluate adaboost ensemble for regression from numpy import mean from numpy import std from sklearn.datasets import make_regression from sklearn.model_selection import cross_val_score from sklearn.model_selection import RepeatedKFold from sklearn.ensemble import AdaBoostRegressor # define dataset X, y = make_regression(n_samples=1000, n_features=20, n_informative=15, noise=0.1, random_state=6) # define the model model = AdaBoostRegressor() # evaluate the model cv = RepeatedKFold(n_splits=10, n_repeats=3, random_state=1) n_scores = cross_val_score(model, X, y, scoring='neg_mean_absolute_error', cv=cv, n_jobs=-1, error_score='raise') # report performance print('MAE: %.3f (%.3f)' % (mean(n_scores), std(n_scores))) |
Running the example reports the mean and standard deviation accuracy of the model.
Note: Your results may vary given the stochastic nature of the algorithm or evaluation procedure, or differences in numerical precision. Consider running the example a few times and compare the average outcome.
In this case, we can see the AdaBoost ensemble with default hyperparameters achieves a MAE of about 100.
1 |
MAE: -72.327 (4.041) |
We can also use the AdaBoost model as a final model and make predictions for regression.
First, the AdaBoost ensemble is fit on all available data, then the predict() function can be called to make predictions on new data.
The example below demonstrates this on our regression dataset.
1 2 3 4 5 6 7 8 9 10 11 12 13 |
# adaboost ensemble for making predictions for regression from sklearn.datasets import make_regression from sklearn.ensemble import AdaBoostRegressor # define dataset X, y = make_regression(n_samples=1000, n_features=20, n_informative=15, noise=0.1, random_state=6) # define the model model = AdaBoostRegressor() # fit the model on the whole dataset model.fit(X, y) # make a single prediction row = [[1.20871625,0.88440466,-0.9030013,-0.22687731,-0.82940077,-1.14410988,1.26554256,-0.2842871,1.43929072,0.74250241,0.34035501,0.45363034,0.1778756,-1.75252881,-1.33337384,-1.50337215,-0.45099008,0.46160133,0.58385557,-1.79936198]] yhat = model.predict(row) print('Prediction: %d' % yhat[0]) |
Running the example fits the AdaBoost ensemble model on the entire dataset and is then used to make a prediction on a new row of data, as we might when using the model in an application.
1 |
Prediction: -10 |
Now that we are familiar with using the scikit-learn API to evaluate and use AdaBoost ensembles, let’s look at configuring the model.
AdaBoost Hyperparameters
In this section, we will take a closer look at some of the hyperparameters you should consider tuning for the AdaBoost ensemble and their effect on model performance.
Explore Number of Trees
An important hyperparameter for AdaBoost algorithm is the number of decision trees used in the ensemble.
Recall that each decision tree used in the ensemble is designed to be a weak learner. That is, it has skill over random prediction, but is not highly skillful. As such, one-level decision trees are used, called decision stumps.
The number of trees added to the model must be high for the model to work well, often hundreds, if not thousands.
The number of trees can be set via the “n_estimators” argument and defaults to 50.
The example below explores the effect of the number of trees with values between 10 to 5,000.
1 2 3 4 5 6 7 8 9 10 11 12 13 14 15 16 17 18 19 20 21 22 23 24 25 26 27 28 29 30 31 32 33 34 35 36 37 38 39 40 41 42 43 44 45 46 47 48 |
# explore adaboost ensemble number of trees effect on performance from numpy import mean from numpy import std from sklearn.datasets import make_classification from sklearn.model_selection import cross_val_score from sklearn.model_selection import RepeatedStratifiedKFold from sklearn.ensemble import AdaBoostClassifier from matplotlib import pyplot # get the dataset def get_dataset(): X, y = make_classification(n_samples=1000, n_features=20, n_informative=15, n_redundant=5, random_state=6) return X, y # get a list of models to evaluate def get_models(): models = dict() # define number of trees to consider n_trees = [10, 50, 100, 500, 1000, 5000] for n in n_trees: models[str(n)] = AdaBoostClassifier(n_estimators=n) return models # evaluate a given model using cross-validation def evaluate_model(model, X, y): # define the evaluation procedure cv = RepeatedStratifiedKFold(n_splits=10, n_repeats=3, random_state=1) # evaluate the model and collect the results scores = cross_val_score(model, X, y, scoring='accuracy', cv=cv, n_jobs=-1) return scores # define dataset X, y = get_dataset() # get the models to evaluate models = get_models() # evaluate the models and store results results, names = list(), list() for name, model in models.items(): # evaluate the model scores = evaluate_model(model, X, y) # store the results results.append(scores) names.append(name) # summarize the performance along the way print('>%s %.3f (%.3f)' % (name, mean(scores), std(scores))) # plot model performance for comparison pyplot.boxplot(results, labels=names, showmeans=True) pyplot.show() |
Running the example first reports the mean accuracy for each configured number of decision trees.
Note: Your results may vary given the stochastic nature of the algorithm or evaluation procedure, or differences in numerical precision. Consider running the example a few times and compare the average outcome.
In this case, we can see that that performance improves on this dataset until about 50 trees and declines after that. This might be a sign of the ensemble overfitting the training dataset after additional trees are added.
1 2 3 4 5 6 |
>10 0.773 (0.039) >50 0.806 (0.041) >100 0.801 (0.032) >500 0.793 (0.028) >1000 0.791 (0.032) >5000 0.782 (0.031) |
A box and whisker plot is created for the distribution of accuracy scores for each configured number of trees.
We can see the general trend of model performance and ensemble size.
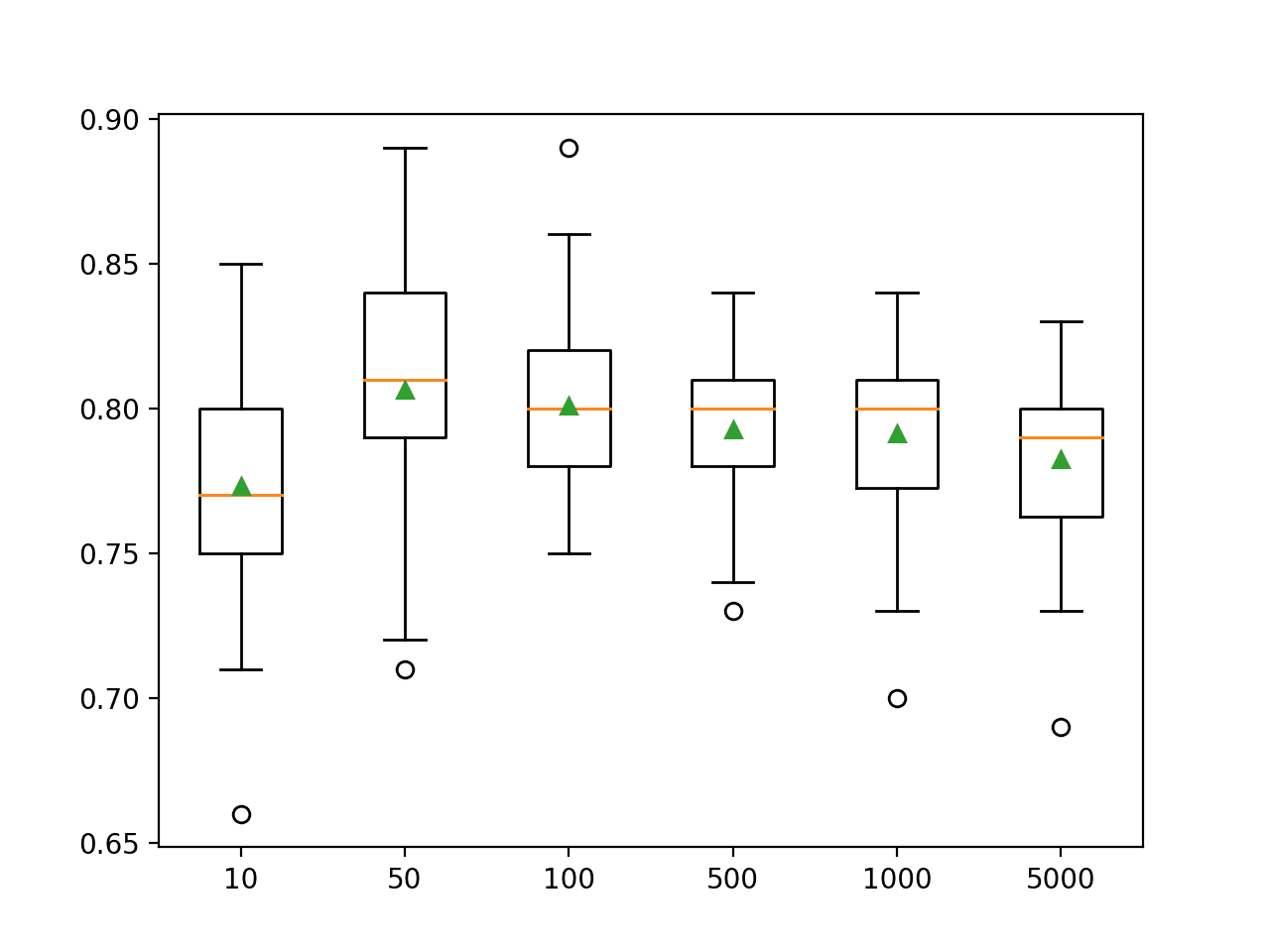
Box Plot of AdaBoost Ensemble Size vs. Classification Accuracy
Explore Weak Learner
A decision tree with one level is used as the weak learner by default.
We can make the models used in the ensemble less weak (more skillful) by increasing the depth of the decision tree.
The example below explores the effect of increasing the depth of the DecisionTreeClassifier weak learner on the AdBoost ensemble.
1 2 3 4 5 6 7 8 9 10 11 12 13 14 15 16 17 18 19 20 21 22 23 24 25 26 27 28 29 30 31 32 33 34 35 36 37 38 39 40 41 42 43 44 45 46 47 48 49 50 51 |
# explore adaboost ensemble tree depth effect on performance from numpy import mean from numpy import std from sklearn.datasets import make_classification from sklearn.model_selection import cross_val_score from sklearn.model_selection import RepeatedStratifiedKFold from sklearn.ensemble import AdaBoostClassifier from sklearn.tree import DecisionTreeClassifier from matplotlib import pyplot # get the dataset def get_dataset(): X, y = make_classification(n_samples=1000, n_features=20, n_informative=15, n_redundant=5, random_state=6) return X, y # get a list of models to evaluate def get_models(): models = dict() # explore depths from 1 to 10 for i in range(1,11): # define base model base = DecisionTreeClassifier(max_depth=i) # define ensemble model models[str(i)] = AdaBoostClassifier(base_estimator=base) return models # evaluate a given model using cross-validation def evaluate_model(model, X, y): # define the evaluation procedure cv = RepeatedStratifiedKFold(n_splits=10, n_repeats=3, random_state=1) # evaluate the model and collect the results scores = cross_val_score(model, X, y, scoring='accuracy', cv=cv, n_jobs=-1) return scores # define dataset X, y = get_dataset() # get the models to evaluate models = get_models() # evaluate the models and store results results, names = list(), list() for name, model in models.items(): # evaluate the model scores = evaluate_model(model, X, y) # store the results results.append(scores) names.append(name) # summarize the performance along the way print('>%s %.3f (%.3f)' % (name, mean(scores), std(scores))) # plot model performance for comparison pyplot.boxplot(results, labels=names, showmeans=True) pyplot.show() |
Running the example first reports the mean accuracy for each configured weak learner tree depth.
Note: Your results may vary given the stochastic nature of the algorithm or evaluation procedure, or differences in numerical precision. Consider running the example a few times and compare the average outcome.
In this case, we can see that as the depth of the decision trees is increased, the performance of the ensemble is also increased on this dataset.
1 2 3 4 5 6 7 8 9 10 |
>1 0.806 (0.041) >2 0.864 (0.028) >3 0.867 (0.030) >4 0.889 (0.029) >5 0.909 (0.021) >6 0.923 (0.020) >7 0.927 (0.025) >8 0.928 (0.028) >9 0.923 (0.017) >10 0.926 (0.030) |
A box and whisker plot is created for the distribution of accuracy scores for each configured weak learner depth.
We can see the general trend of model performance and weak learner depth.
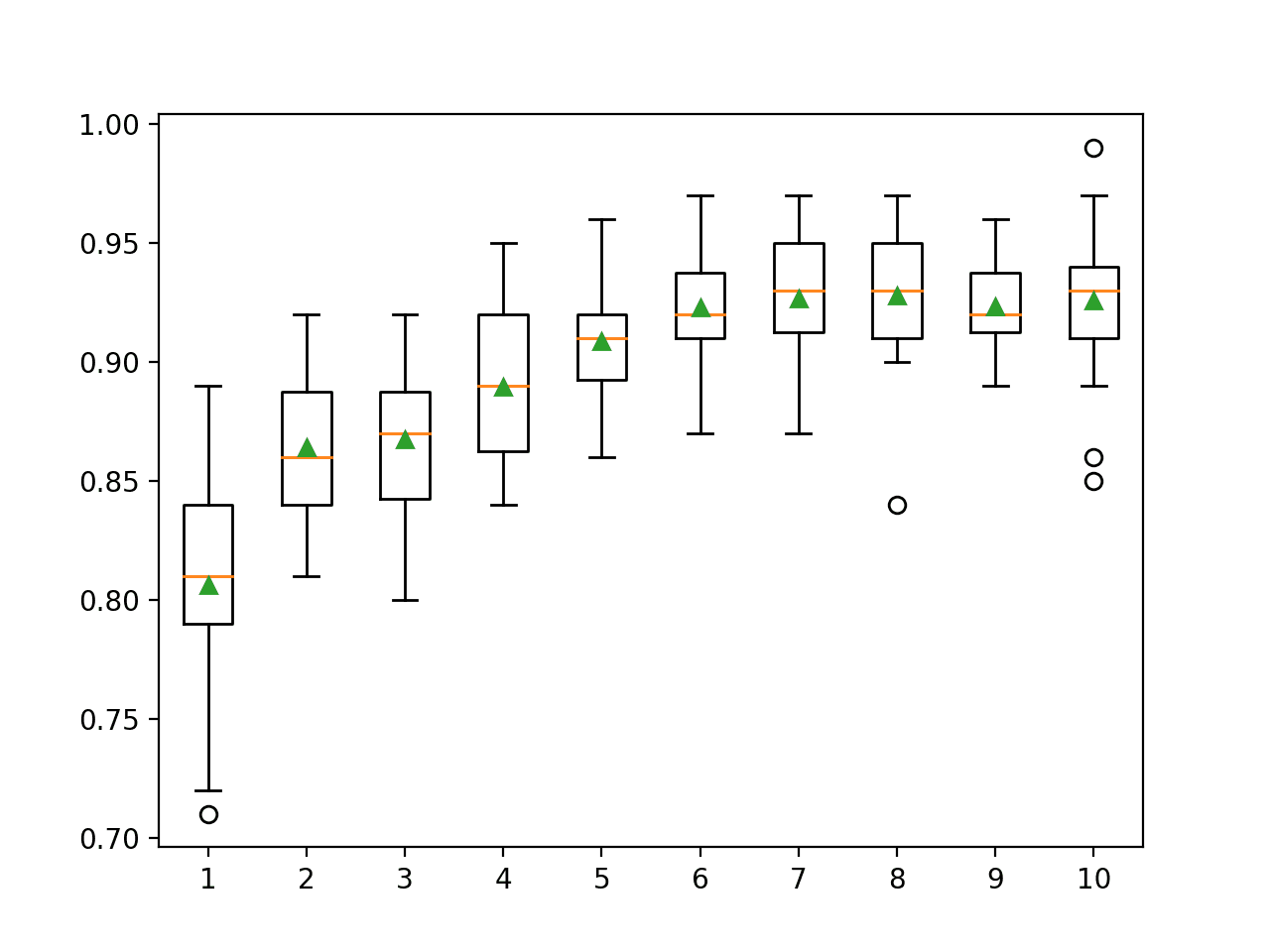
Box Plot of AdaBoost Ensemble Weak Learner Depth vs. Classification Accuracy
Explore Learning Rate
AdaBoost also supports a learning rate that controls the contribution of each model to the ensemble prediction.
This is controlled by the “learning_rate” argument and by default is set to 1.0 or full contribution. Smaller or larger values might be appropriate depending on the number of models used in the ensemble. There is a balance between the contribution of the models and the number of trees in the ensemble.
More trees may require a smaller learning rate; fewer trees may require a larger learning rate. It is common to use values between 0 and 1 and sometimes very small values to avoid overfitting such as 0.1, 0.01 or 0.001.
The example below explores learning rate values between 0.1 and 2.0 in 0.1 increments.
1 2 3 4 5 6 7 8 9 10 11 12 13 14 15 16 17 18 19 20 21 22 23 24 25 26 27 28 29 30 31 32 33 34 35 36 37 38 39 40 41 42 43 44 45 46 47 48 49 50 |
# explore adaboost ensemble learning rate effect on performance from numpy import mean from numpy import std from numpy import arange from sklearn.datasets import make_classification from sklearn.model_selection import cross_val_score from sklearn.model_selection import RepeatedStratifiedKFold from sklearn.ensemble import AdaBoostClassifier from matplotlib import pyplot # get the dataset def get_dataset(): X, y = make_classification(n_samples=1000, n_features=20, n_informative=15, n_redundant=5, random_state=6) return X, y # get a list of models to evaluate def get_models(): models = dict() # explore learning rates from 0.1 to 2 in 0.1 increments for i in arange(0.1, 2.1, 0.1): key = '%.3f' % i models[key] = AdaBoostClassifier(learning_rate=i) return models # evaluate a given model using cross-validation def evaluate_model(model, X, y): # define the evaluation procedure cv = RepeatedStratifiedKFold(n_splits=10, n_repeats=3, random_state=1) # evaluate the model and collect the results scores = cross_val_score(model, X, y, scoring='accuracy', cv=cv, n_jobs=-1) return scores # define dataset X, y = get_dataset() # get the models to evaluate models = get_models() # evaluate the models and store results results, names = list(), list() for name, model in models.items(): # evaluate the model scores = evaluate_model(model, X, y) # store the results results.append(scores) names.append(name) # summarize the performance along the way print('>%s %.3f (%.3f)' % (name, mean(scores), std(scores))) # plot model performance for comparison pyplot.boxplot(results, labels=names, showmeans=True) pyplot.xticks(rotation=45) pyplot.show() |
Running the example first reports the mean accuracy for each configured learning rate.
Note: Your results may vary given the stochastic nature of the algorithm or evaluation procedure, or differences in numerical precision. Consider running the example a few times and compare the average outcome.
In this case, we can see similar values between 0.5 to 1.0 and a decrease in model performance after that.
1 2 3 4 5 6 7 8 9 10 11 12 13 14 15 16 17 18 19 20 |
>0.100 0.767 (0.049) >0.200 0.786 (0.042) >0.300 0.802 (0.040) >0.400 0.798 (0.037) >0.500 0.805 (0.042) >0.600 0.795 (0.031) >0.700 0.799 (0.035) >0.800 0.801 (0.033) >0.900 0.805 (0.032) >1.000 0.806 (0.041) >1.100 0.801 (0.037) >1.200 0.800 (0.030) >1.300 0.799 (0.041) >1.400 0.793 (0.041) >1.500 0.790 (0.040) >1.600 0.775 (0.034) >1.700 0.767 (0.054) >1.800 0.768 (0.040) >1.900 0.736 (0.047) >2.000 0.682 (0.048) |
A box and whisker plot is created for the distribution of accuracy scores for each configured learning rate.
We can see the general trend of decreasing model performance with a learning rate larger than 1.0 on this dataset.
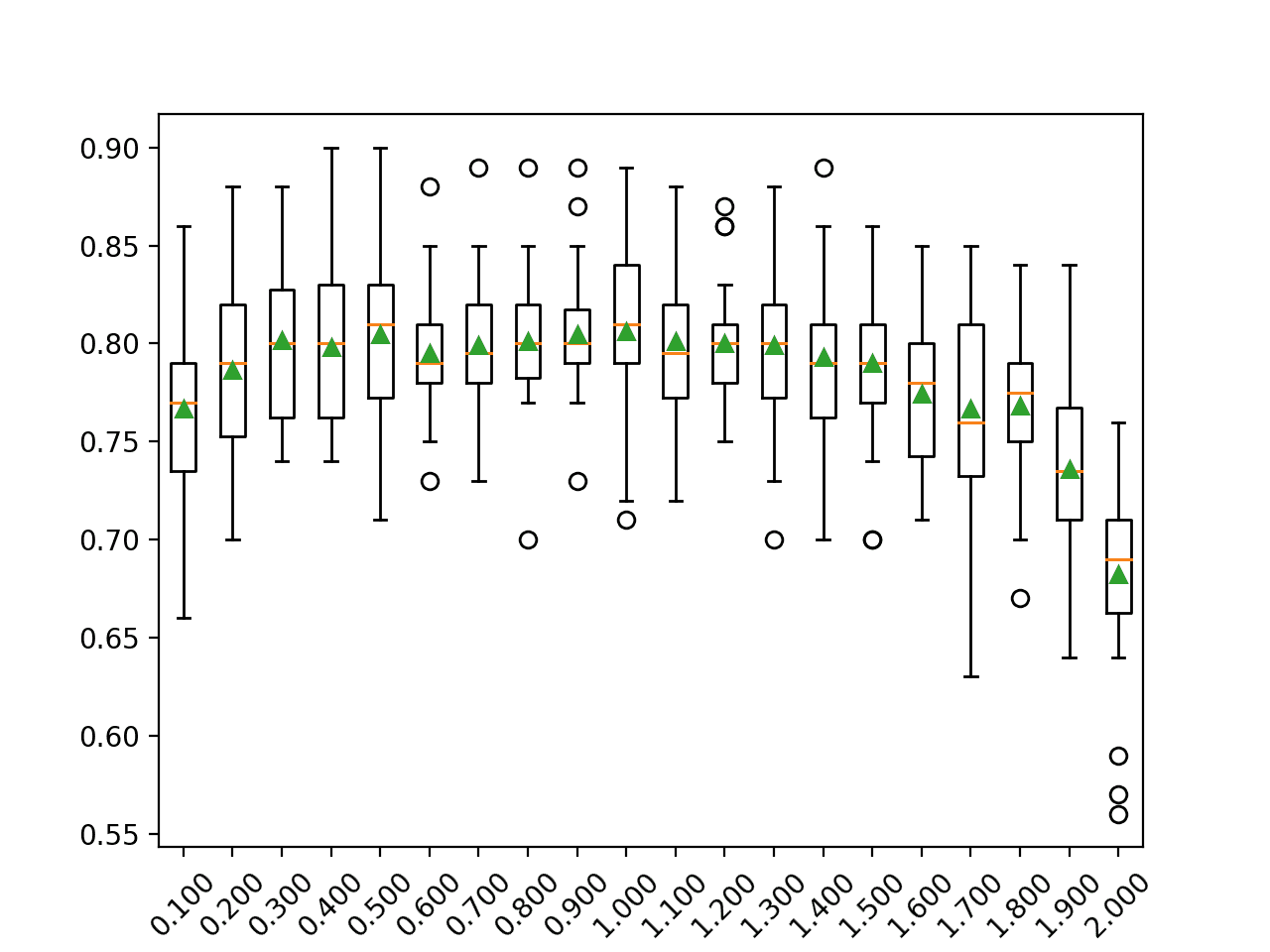
Box Plot of AdaBoost Ensemble Learning Rate vs. Classification Accuracy
Explore Alternate Algorithm
The default algorithm used in the ensemble is a decision tree, although other algorithms can be used.
The intent is to use very simple models, called weak learners. Also, the scikit-learn implementation requires that any models used must also support weighted samples, as they are how the ensemble is created by fitting models based on a weighted version of the training dataset.
The base model can be specified via the “base_estimator” argument. The base model must also support predicting probabilities or probability-like scores in the case of classification. If the specified model does not support a weighted training dataset, you will see an error message as follows:
1 |
ValueError: KNeighborsClassifier doesn't support sample_weight. |
One example of a model that supports a weighted training is the logistic regression algorithm.
The example below demonstrates an AdaBoost algorithm with a LogisticRegression weak learner.
1 2 3 4 5 6 7 8 9 10 11 12 13 14 15 16 17 |
# evaluate adaboost algorithm with logistic regression weak learner for classification from numpy import mean from numpy import std from sklearn.datasets import make_classification from sklearn.model_selection import cross_val_score from sklearn.model_selection import RepeatedStratifiedKFold from sklearn.ensemble import AdaBoostClassifier from sklearn.linear_model import LogisticRegression # define dataset X, y = make_classification(n_samples=1000, n_features=20, n_informative=15, n_redundant=5, random_state=6) # define the model model = AdaBoostClassifier(base_estimator=LogisticRegression()) # evaluate the model cv = RepeatedStratifiedKFold(n_splits=10, n_repeats=3, random_state=1) n_scores = cross_val_score(model, X, y, scoring='accuracy', cv=cv, n_jobs=-1, error_score='raise') # report performance print('Accuracy: %.3f (%.3f)' % (mean(n_scores), std(n_scores))) |
Running the example reports the mean and standard deviation accuracy of the model.
Note: Your results may vary given the stochastic nature of the algorithm or evaluation procedure, or differences in numerical precision. Consider running the example a few times and compare the average outcome.
In this case, we can see the AdaBoost ensemble with a logistic regression weak model achieves a classification accuracy of about 79 percent on this test dataset.
1 |
Accuracy: 0.794 (0.032) |
Grid Search AdaBoost Hyperparameters
AdaBoost can be challenging to configure as the algorithm as many key hyperparameters that influence the behavior of the model on training data and the hyperparameters interact with each other.
As such, it is a good practice to use a search process to discover a configuration of the model hyperparameters that works well or best for a given predictive modeling problem. Popular search processes include a random search and a grid search.
In this section we will look at grid searching common ranges for the key hyperparameters for the AdaBoost algorithm that you can use as starting point for your own projects. This can be achieving using the GridSearchCV class and specifying a dictionary that maps model hyperparameter names to the values to search.
In this case, we will grid search two key hyperparameters for AdaBoost: the number of trees used in the ensemble and the learning rate. We will use a range of popular well performing values for each hyperparameter.
Each configuration combination will be evaluated using repeated k-fold cross-validation and configurations will be compared using the mean score, in this case, classification accuracy.
The complete example of grid searching the key hyperparameters of the AdaBoost algorithm on our synthetic classification dataset is listed below.
1 2 3 4 5 6 7 8 9 10 11 12 13 14 15 16 17 18 19 20 21 22 23 24 25 26 27 |
# example of grid searching key hyperparameters for adaboost on a classification dataset from sklearn.datasets import make_classification from sklearn.model_selection import RepeatedStratifiedKFold from sklearn.model_selection import GridSearchCV from sklearn.ensemble import AdaBoostClassifier # define dataset X, y = make_classification(n_samples=1000, n_features=20, n_informative=15, n_redundant=5, random_state=6) # define the model with default hyperparameters model = AdaBoostClassifier() # define the grid of values to search grid = dict() grid['n_estimators'] = [10, 50, 100, 500] grid['learning_rate'] = [0.0001, 0.001, 0.01, 0.1, 1.0] # define the evaluation procedure cv = RepeatedStratifiedKFold(n_splits=10, n_repeats=3, random_state=1) # define the grid search procedure grid_search = GridSearchCV(estimator=model, param_grid=grid, n_jobs=-1, cv=cv, scoring='accuracy') # execute the grid search grid_result = grid_search.fit(X, y) # summarize the best score and configuration print("Best: %f using %s" % (grid_result.best_score_, grid_result.best_params_)) # summarize all scores that were evaluated means = grid_result.cv_results_['mean_test_score'] stds = grid_result.cv_results_['std_test_score'] params = grid_result.cv_results_['params'] for mean, stdev, param in zip(means, stds, params): print("%f (%f) with: %r" % (mean, stdev, param)) |
Running the example many take a while depending on your hardware. At the end of the run, the configuration that achieved the best score is reported first, followed by the scores for all other configurations that were considered.
Note: Your results may vary given the stochastic nature of the algorithm or evaluation procedure, or differences in numerical precision. Consider running the example a few times and compare the average outcome.
In this case, we can see that a configuration with 500 trees and a learning rate of 0.1 performed the best with a classification accuracy of about 81.3 percent.
The model may perform even better with more trees such as 1,000 or 5,000 although these configurations were not tested in this case to ensure that the grid search completed in a reasonable time.
1 2 3 4 5 6 7 8 9 10 11 12 13 14 15 16 17 18 19 20 21 |
Best: 0.813667 using {'learning_rate': 0.1, 'n_estimators': 500} 0.646333 (0.036376) with: {'learning_rate': 0.0001, 'n_estimators': 10} 0.646667 (0.036545) with: {'learning_rate': 0.0001, 'n_estimators': 50} 0.646667 (0.036545) with: {'learning_rate': 0.0001, 'n_estimators': 100} 0.647000 (0.038136) with: {'learning_rate': 0.0001, 'n_estimators': 500} 0.646667 (0.036545) with: {'learning_rate': 0.001, 'n_estimators': 10} 0.647000 (0.038136) with: {'learning_rate': 0.001, 'n_estimators': 50} 0.654333 (0.045511) with: {'learning_rate': 0.001, 'n_estimators': 100} 0.672667 (0.046543) with: {'learning_rate': 0.001, 'n_estimators': 500} 0.648333 (0.042197) with: {'learning_rate': 0.01, 'n_estimators': 10} 0.671667 (0.045613) with: {'learning_rate': 0.01, 'n_estimators': 50} 0.715000 (0.053213) with: {'learning_rate': 0.01, 'n_estimators': 100} 0.767667 (0.045948) with: {'learning_rate': 0.01, 'n_estimators': 500} 0.716667 (0.048876) with: {'learning_rate': 0.1, 'n_estimators': 10} 0.767000 (0.049271) with: {'learning_rate': 0.1, 'n_estimators': 50} 0.784667 (0.042874) with: {'learning_rate': 0.1, 'n_estimators': 100} 0.813667 (0.032092) with: {'learning_rate': 0.1, 'n_estimators': 500} 0.773333 (0.038759) with: {'learning_rate': 1.0, 'n_estimators': 10} 0.806333 (0.040701) with: {'learning_rate': 1.0, 'n_estimators': 50} 0.801000 (0.032491) with: {'learning_rate': 1.0, 'n_estimators': 100} 0.792667 (0.027560) with: {'learning_rate': 1.0, 'n_estimators': 500} |
Further Reading
This section provides more resources on the topic if you are looking to go deeper.
Tutorials
Papers
- A Decision-Theoretic Generalization of on-Line Learning and an Application to Boosting, 1996.
- Multi-class AdaBoost, 2009.
- Improving Regressors using Boosting Techniques, 1997.
APIs
Articles
Summary
In this tutorial, you discovered how to develop AdaBoost ensembles for classification and regression.
Specifically, you learned:
- AdaBoost ensemble is an ensemble created from decision trees added sequentially to the model.
- How to use the AdaBoost ensemble for classification and regression with scikit-learn.
- How to explore the effect of AdaBoost model hyperparameters on model performance.
Do you have any questions?
Ask your questions in the comments below and I will do my best to answer.
nicely explained the concept and how the model behavior changes with respect to individual hyperparameter.
similar explanation with combination of hyperparameter will be more effective. e.g. how the model will behave when learning rate and no of estimators are changed together
Thanks!
Great suggestion.
Hi, I really enjoy your posts and courses.
My question is, we use cross-validation to assess the performance of a given model, but CV won’t improve the model, it will inform on the performance of the model that we are testing, is that right?
If we want to predict new samples we need to use the model that we used for CV and gave the higher mean accuracy (?).
Almost.
CV just evaluates the model. Then we throw away the models.
After we choose a model and config, we fit the model on all data and use it to start making predictions, more details here:
https://machinelearningmastery.com/train-final-machine-learning-model/
Hi Jason, great article as always
How do you set the model parameters to the best found hyper parameters
I tried **hyperParams but in the case of AdaBoost it seems to obliterate the base parameters and only set the best found params
Sorry Mark, I don’t understand your problem/question. Can you please elaborate?
Ignore my last comment, sorted it
You have to set the (in my case) DecisionTreeClassifier(max_depth=3), **hyperParams
Its easy to assume that like other hyper params in other models the ones not being replaced with tuned param’s are left as is but this is not the case with this parameter and AdaBoost.
No problem.
Thank you Jason. Sincerely, I learned a lot following your tutorials.
Just a quick question @Jason please.
I understand you used scikit-learn implementation of the AdaBoost Classifier and in the “Explore Number of trees” section, you stated ” Recall that each decision tree used in the ensemble is designed to be a weak learner. That is, it has skill over random prediction, but is not highly skilful…… As such, one-level decision trees are used, called decision stumps.”
However, in the scikit-learn documentation of AdaBoostClassifier, default trees is 50, (n_estimators=50). How is that a one-level tree /decision stumps?
https://scikit-learn.org/stable/modules/generated/sklearn.ensemble.AdaBoostClassifier.html
50 trees, where each tree is a decision stump added to the ensemble one at a time.
nice explanation sir.
Sir please write a blog on how to use neural networks(CNNs) as weak classifiers in adaboost on image dataset.
I have read many articles on google on adaboost but major articles talks about applying adaboost on tabular dataset and with decision tree as weak classifiers .
Plz help!
Thank you for the feedback and suggestions, Aditi! We greatly appreciate it.